OpenSearch は、Elasticsearch のオープンソース代替品であり、大規模なデータセット 簡単に。このブログでは、Python を使用して OpenSearch で基本的な CRUD (作成、読み取り、更新、削除) 操作を実行する方法をデモします。
前提条件:
- Python 3.7+
- Docker を使用してローカルにインストールされた OpenSearch
- RESTful API に関する知識
ステップ 1: Docker を使用して OpenSearch をローカルにセットアップする
始めるには、ローカル OpenSearch インスタンスが必要です。以下は、OpenSearch と OpenSearch Dashboards を起動する単純な docker-compose.yml ファイルです。
version: '3' services: opensearch-test-node-1: image: opensearchproject/opensearch:2.13.0 container_name: opensearch-test-node-1 environment: - cluster.name=opensearch-test-cluster - node.name=opensearch-test-node-1 - discovery.seed_hosts=opensearch-test-node-1,opensearch-test-node-2 - cluster.initial_cluster_manager_nodes=opensearch-test-node-1,opensearch-test-node-2 - bootstrap.memory_lock=true - "OPENSEARCH_JAVA_OPTS=-Xms512m -Xmx512m" - "DISABLE_INSTALL_DEMO_CONFIG=true" - "DISABLE_SECURITY_PLUGIN=true" ulimits: memlock: soft: -1 hard: -1 nofile: soft: 65536 hard: 65536 volumes: - opensearch-test-data1:/usr/share/opensearch/data ports: - 9200:9200 - 9600:9600 networks: - opensearch-test-net opensearch-test-node-2: image: opensearchproject/opensearch:2.13.0 container_name: opensearch-test-node-2 environment: - cluster.name=opensearch-test-cluster - node.name=opensearch-test-node-2 - discovery.seed_hosts=opensearch-test-node-1,opensearch-test-node-2 - cluster.initial_cluster_manager_nodes=opensearch-test-node-1,opensearch-test-node-2 - bootstrap.memory_lock=true - "OPENSEARCH_JAVA_OPTS=-Xms512m -Xmx512m" - "DISABLE_INSTALL_DEMO_CONFIG=true" - "DISABLE_SECURITY_PLUGIN=true" ulimits: memlock: soft: -1 hard: -1 nofile: soft: 65536 hard: 65536 volumes: - opensearch-test-data2:/usr/share/opensearch/data networks: - opensearch-test-net opensearch-test-dashboards: image: opensearchproject/opensearch-dashboards:2.13.0 container_name: opensearch-test-dashboards ports: - 5601:5601 expose: - "5601" environment: - 'OPENSEARCH_HOSTS=["http://opensearch-test-node-1:9200","http://opensearch-test-node-2:9200"]' - "DISABLE_SECURITY_DASHBOARDS_PLUGIN=true" networks: - opensearch-test-net volumes: opensearch-test-data1: opensearch-test-data2: networks: opensearch-test-net:
次のコマンドを実行して OpenSearch インスタンスを起動します:docker-compose up
OpenSearch には http://localhost:9200 からアクセスできます。
ステップ 2: Python 環境をセットアップする
python -m venv .venv source .venv/bin/activate pip install opensearch-py
また、プロジェクトを次のように構造化します:
├── interfaces.py ├── main.py ├── searchservice.py ├── docker-compose.yml
ステップ 3: インターフェイスとリソースの定義 (interfaces.py)
interfaces.py ファイルで、Resource クラスと Resources クラスを定義します。これらは、OpenSearch でさまざまなリソース タイプ (この場合はユーザー) を動的に処理するのに役立ちます。
from dataclasses import dataclass, field @dataclass class Resource: name: str def __post_init__(self) -> None: self.name = self.name.lower() @dataclass class Resources: users: Resource = field(default_factory=lambda: Resource("Users"))
ステップ 4: OpenSearch (searchservice.py) を使用した CRUD 操作
searchservice.py では、必要な操作の概要を示す抽象クラス SearchService を定義します。次に、HTTPOpenSearchService クラスはこれらの CRUD メソッドを実装し、OpenSearch クライアントと対話します。
# coding: utf-8 import abc import logging import typing as t from dataclasses import dataclass from uuid import UUID from interfaces import Resource, Resources from opensearchpy import NotFoundError, OpenSearch resources = Resources() class SearchService(abc.ABC): def search( self, kinds: t.List[Resource], tenants_id: UUID, companies_id: UUID, query: t.Dict[str, t.Any], ) -> t.Dict[t.Literal["hits"], t.Dict[str, t.Any]]: raise NotImplementedError def delete_index( self, kind: Resource, tenants_id: UUID, companies_id: UUID, data: t.Dict[str, t.Any], ) -> None: raise NotImplementedError def index( self, kind: Resource, tenants_id: UUID, companies_id: UUID, data: t.Dict[str, t.Any], ) -> t.Dict[str, t.Any]: raise NotImplementedError def delete_document( self, kind: Resource, tenants_id: UUID, companies_id: UUID, document_id: str, ) -> t.Optional[t.Dict[str, t.Any]]: raise NotImplementedError def create_index( self, kind: Resource, tenants_id: UUID, companies_id: UUID, data: t.Dict[str, t.Any], ) -> None: raise NotImplementedError @dataclass(frozen=True) class HTTPOpenSearchService(SearchService): client: OpenSearch def _gen_index( self, kind: Resource, tenants_id: UUID, companies_id: UUID, ) -> str: return ( f"tenant_{str(UUID(str(tenants_id)))}" f"_company_{str(UUID(str(companies_id)))}" f"_kind_{kind.name}" ) def index( self, kind: Resource, tenants_id: UUID, companies_id: UUID, data: t.Dict[str, t.Any], ) -> t.Dict[str, t.Any]: self.client.index( index=self._gen_index(kind, tenants_id, companies_id), body=data, id=data.get("id"), ) return data def delete_index( self, kind: Resource, tenants_id: UUID, companies_id: UUID, ) -> None: try: index = self._gen_index(kind, tenants_id, companies_id) if self.client.indices.exists(index): self.client.indices.delete(index) except NotFoundError: pass def create_index( self, kind: Resource, tenants_id: UUID, companies_id: UUID, ) -> None: body: t.Dict[str, t.Any] = {} self.client.indices.create( index=self._gen_index(kind, tenants_id, companies_id), body=body, ) def search( self, kinds: t.List[Resource], tenants_id: UUID, companies_id: UUID, query: t.Dict[str, t.Any], ) -> t.Dict[t.Literal["hits"], t.Dict[str, t.Any]]: return self.client.search( index=",".join( [self._gen_index(kind, tenants_id, companies_id) for kind in kinds] ), body={"query": query}, ) def delete_document( self, kind: Resource, tenants_id: UUID, companies_id: UUID, document_id: str, ) -> t.Optional[t.Dict[str, t.Any]]: try: response = self.client.delete( index=self._gen_index(kind, tenants_id, companies_id), id=document_id, ) return response except Exception as e: logging.error(f"Error deleting document: {e}") return None
ステップ 5: メイン (main.py) に CRUD を実装する
main.py では、次の方法を示します。
OpenSearch で
main.py- インデックス を作成します。
- サンプル ユーザー データを使用してドキュメントにインデックスを付けます。
クエリに基づいてドキュメントを- 検索します。
ID を使用してドキュメントを- 削除します。
# coding=utf-8 import logging import os import typing as t from uuid import uuid4 import searchservice from interfaces import Resources from opensearchpy import OpenSearch resources = Resources() logging.basicConfig(level=logging.INFO) search_service = searchservice.HTTPOpenSearchService( client=OpenSearch( hosts=[ { "host": os.getenv("OPENSEARCH_HOST", "localhost"), "port": os.getenv("OPENSEARCH_PORT", "9200"), } ], http_auth=( os.getenv("OPENSEARCH_USERNAME", ""), os.getenv("OPENSEARCH_PASSWORD", ""), ), use_ssl=False, verify_certs=False, ), ) tenants_id: str = "f0835e2d-bd68-406c-99a7-ad63a51e9ef9" companies_id: str = "bf58c749-c90a-41e2-b66f-6d98aae17a6c" search_str: str = "frank" document_id_to_delete: str = str(uuid4()) fake_data: t.List[t.Dict[str, t.Any]] = [ {"id": document_id_to_delete, "name": "Franklin", "tech": "python,node,golang"}, {"id": str(uuid4()), "name": "Jarvis", "tech": "AI"}, {"id": str(uuid4()), "name": "Parry", "tech": "Golang"}, {"id": str(uuid4()), "name": "Steve", "tech": "iOS"}, {"id": str(uuid4()), "name": "Frank", "tech": "node"}, ] search_service.delete_index( kind=resources.users, tenants_id=tenants_id, companies_id=companies_id ) search_service.create_index( kind=resources.users, tenants_id=tenants_id, companies_id=companies_id, ) for item in fake_data: search_service.index( kind=resources.users, tenants_id=tenants_id, companies_id=companies_id, data=dict(tenants_id=tenants_id, companies_id=companies_id, **item), ) search_query: t.Dict[str, t.Any] = { "bool": { "must": [], "must_not": [], "should": [], "filter": [ {"term": {"tenants_id.keyword": tenants_id}}, {"term": {"companies_id.keyword": companies_id}}, ], } } search_query["bool"]["must"].append( { "multi_match": { "query": search_str, "type": "phrase_prefix", "fields": ["name", "tech"], } } ) search_results = search_service.search( kinds=[resources.users], tenants_id=tenants_id, companies_id=companies_id, query=search_query, ) final_result = search_results.get("hits", {}).get("hits", []) for item in final_result: logging.info(["Item -> ", item.get("_source", {})]) deleted_result = search_service.delete_document( kind=resources.users, tenants_id=tenants_id, companies_id=companies_id, document_id=document_id_to_delete, ) logging.info(["Deleted result -> ", deleted_result])
ステップ 6: プロジェクトの実行
ドッカー構成Python main.py
結果:
見つかったレコードと削除されたレコードの情報を出力する必要があります。
ステップ 7: 結論
このブログでは、Docker を使用してローカルで OpenSearch をセットアップし、CRUD 操作を実行する方法をデモしました。 🎜>パイソン。 OpenSearch は、大規模なデータセットの管理とクエリを実行するための強力でスケーラブルなソリューションを提供します。このガイドは OpenSearch と ダミー データ の統合に焦点を当てていますが、実際のアプリケーションでは、OpenSearch は の高速化のための 読み取り最適化ストア としてよく使用されます。 データの取得。このような場合、プライマリ データベースと OpenSearch の両方を同時に更新することでデータの一貫性を確保するために、さまざまな インデックス付け戦略 を実装するのが一般的です。 これにより、OpenSearch
がプライマリ データ ソースと同期した状態を維持し、パフォーマンス と 精度最適化 します。 > データ取得中。 参考文献:
https://github.com/FranklinThaker/opensearch-integration-example
以上がPython で OpenSearch を使用した CRUD 操作をマスターする: 実践ガイドの詳細内容です。詳細については、PHP 中国語 Web サイトの他の関連記事を参照してください。
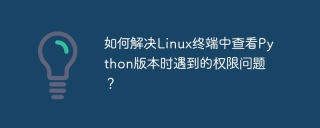
LinuxターミナルでPythonバージョンを表示する際の許可の問題の解決策PythonターミナルでPythonバージョンを表示しようとするとき、Pythonを入力してください...
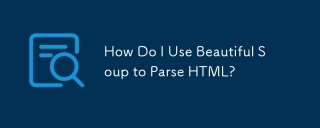
この記事では、Pythonライブラリである美しいスープを使用してHTMLを解析する方法について説明します。 find()、find_all()、select()、およびget_text()などの一般的な方法は、データ抽出、多様なHTML構造とエラーの処理、および代替案(SEL
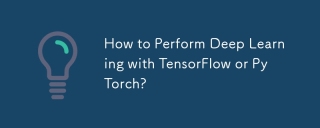
この記事では、深い学習のためにTensorflowとPytorchを比較しています。 関連する手順、データの準備、モデルの構築、トレーニング、評価、展開について詳しく説明しています。 特に計算グラップに関して、フレームワーク間の重要な違い
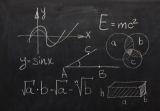
Pythonの統計モジュールは、強力なデータ統計分析機能を提供して、生物統計やビジネス分析などのデータの全体的な特性を迅速に理解できるようにします。データポイントを1つずつ見る代わりに、平均や分散などの統計を見て、無視される可能性のある元のデータの傾向と機能を発見し、大きなデータセットをより簡単かつ効果的に比較してください。 このチュートリアルでは、平均を計算し、データセットの分散の程度を測定する方法を説明します。特に明記しない限り、このモジュールのすべての関数は、単に平均を合計するのではなく、平均()関数の計算をサポートします。 浮動小数点数も使用できます。 ランダムをインポートします インポート統計 fractiから
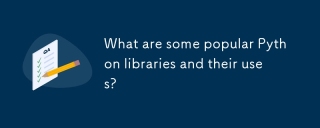
この記事では、numpy、pandas、matplotlib、scikit-learn、tensorflow、django、flask、and requestsなどの人気のあるPythonライブラリについて説明し、科学的コンピューティング、データ分析、視覚化、機械学習、Web開発、Hの使用について説明します。
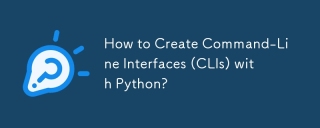
この記事では、コマンドラインインターフェイス(CLI)の構築に関するPython開発者をガイドします。 Typer、Click、Argparseなどのライブラリを使用して、入力/出力の処理を強調し、CLIの使いやすさを改善するためのユーザーフレンドリーな設計パターンを促進することを詳述しています。
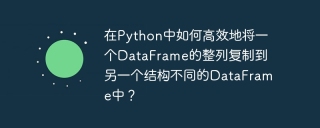
PythonのPandasライブラリを使用する場合、異なる構造を持つ2つのデータフレーム間で列全体をコピーする方法は一般的な問題です。 2つのデータがあるとします...
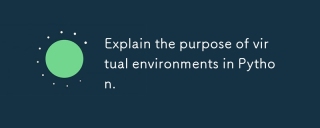
この記事では、Pythonにおける仮想環境の役割について説明し、プロジェクトの依存関係の管理と競合の回避に焦点を当てています。プロジェクト管理の改善と依存関係の問題を減らすための作成、アクティベーション、およびメリットを詳しく説明しています。


ホットAIツール

Undresser.AI Undress
リアルなヌード写真を作成する AI 搭載アプリ

AI Clothes Remover
写真から衣服を削除するオンライン AI ツール。

Undress AI Tool
脱衣画像を無料で

Clothoff.io
AI衣類リムーバー

AI Hentai Generator
AIヘンタイを無料で生成します。

人気の記事

ホットツール

mPDF
mPDF は、UTF-8 でエンコードされた HTML から PDF ファイルを生成できる PHP ライブラリです。オリジナルの作者である Ian Back は、Web サイトから「オンザフライ」で PDF ファイルを出力し、さまざまな言語を処理するために mPDF を作成しました。 HTML2FPDF などのオリジナルのスクリプトよりも遅く、Unicode フォントを使用すると生成されるファイルが大きくなりますが、CSS スタイルなどをサポートし、多くの機能強化が施されています。 RTL (アラビア語とヘブライ語) や CJK (中国語、日本語、韓国語) を含むほぼすべての言語をサポートします。ネストされたブロックレベル要素 (P、DIV など) をサポートします。

SecLists
SecLists は、セキュリティ テスターの究極の相棒です。これは、セキュリティ評価中に頻繁に使用されるさまざまな種類のリストを 1 か所にまとめたものです。 SecLists は、セキュリティ テスターが必要とする可能性のあるすべてのリストを便利に提供することで、セキュリティ テストをより効率的かつ生産的にするのに役立ちます。リストの種類には、ユーザー名、パスワード、URL、ファジング ペイロード、機密データ パターン、Web シェルなどが含まれます。テスターはこのリポジトリを新しいテスト マシンにプルするだけで、必要なあらゆる種類のリストにアクセスできるようになります。

ゼンドスタジオ 13.0.1
強力な PHP 統合開発環境

Safe Exam Browser
Safe Exam Browser は、オンライン試験を安全に受験するための安全なブラウザ環境です。このソフトウェアは、あらゆるコンピュータを安全なワークステーションに変えます。あらゆるユーティリティへのアクセスを制御し、学生が無許可のリソースを使用するのを防ぎます。

PhpStorm Mac バージョン
最新(2018.2.1)のプロフェッショナル向けPHP統合開発ツール

ホットトピック



