YOLO (You Only Look Once) has been a leading real-time object detection framework, with each iteration improving upon the previous versions. The latest version YOLO v12 introduces advancements that significantly enhance accuracy while maintaining real-time processing speeds. This article explores the key innovations in YOLO v12, highlighting how it surpasses the previous versions while minimizing computational costs without compromising detection efficiency.
Table of contents
- What’s New in YOLO v12?
- Key Improvements Over Previous Versions
- Computational Efficiency Enhancements
- YOLO v12 Model Variants
- Let’s compare YOLO v11 and YOLO v12 Models
- Expert Opinions on YOLOv11 and YOLOv12
- Conclusion
What’s New in YOLO v12?
Previously, YOLO models relied on Convolutional Neural Networks (CNNs) for object detection due to their speed and efficiency. However, YOLO v12 makes use of attention mechanisms, a concept widely known and used in Transformer models which allow it to recognize patterns more effectively. While attention mechanisms have originally been slow for real-time object detection, YOLO v12 somehow successfully integrates them while maintaining YOLO’s speed, leading to an Attention-Centric YOLO framework.
Key Improvements Over Previous Versions
1. Attention-Centric Framework
YOLO v12 combines the power of attention mechanisms with CNNs, resulting in a model that is both faster and more accurate. Unlike its predecessors which relied solely on CNNs, YOLO v12 introduces optimized attention modules to improve object recognition without adding unnecessary latency.
2. Superior Performance Metrics
Comparing performance metrics across different YOLO versions and real-time detection models reveals that YOLO v12 achieves higher accuracy while maintaining low latency.
- The mAP (Mean Average Precision) values on datasets like COCO show YOLO v12 outperforming YOLO v11 and YOLO v10 while maintaining comparable speed.
- The model achieves a remarkable 40.6% accuracy (mAP) while processing images in just 1.64 milliseconds on an Nvidia T4 GPU. This performance is superior to YOLO v10 and YOLO v11 without sacrificing speed.
3. Outperforming Non-YOLO Models
YOLO v12 surpasses previous YOLO versions; it also outperforms other real-time object detection frameworks, such as RT-Det and RT-Det v2. These alternative models have higher latency yet fail to match YOLO v12’s accuracy.
Computational Efficiency Enhancements
One of the major concerns with integrating attention mechanisms into YOLO models was their high computational cost (Attention Mechanism) and memory inefficiency. YOLO v12 addresses these issues through several key innovations:
1. Flash Attention for Memory Efficiency
Traditional attention mechanisms consume a large amount of memory, making them impractical for real-time applications. YOLO v12 introduces Flash Attention, a technique that reduces memory consumption and speeds up inference time.
2. Area Attention for Lower Computation Cost
To further optimize efficiency, YOLO v12 employs Area Attention, which focuses only on relevant regions of an image instead of processing the entire feature map. This technique dramatically reduces computation costs while retaining accuracy.
3. R-ELAN for Optimized Feature Processing
YOLO v12 also introduces R-ELAN (Re-Engineered ELAN), which optimizes feature propagation making the model more efficient in handling complex object detection tasks without increasing computational demands.
YOLO v12 Model Variants
YOLO v12 comes in five different variants, catering to different applications:
- N (Nano) & S (Small): Designed for real-time applications where speed is crucial.
- M (Medium): Balances accuracy and speed, suitable for general-purpose tasks.
- L (Large) & XL (Extra Large): Optimized for high-precision tasks where accuracy is prioritized over speed.
Also read:
- A Step-by-Step Introduction to the Basic Object Detection Algorithms (Part 1)
- A Practical Implementation of the Faster R-CNN Algorithm for Object Detection (Part 2)
- A Practical Guide to Object Detection using the Popular YOLO Framework – Part III (with Python codes)
Let’s compare YOLO v11 and YOLO v12 Models
We’ll be experimenting with YOLO v11 and YOLO v12 small models to understand their performance across various tasks like object counting, heatmaps, and speed estimation.
1. Object Counting
YOLO v11
import cv2 from ultralytics import solutions cap = cv2.VideoCapture("highway.mp4") assert cap.isOpened(), "Error reading video file" w, h, fps = (int(cap.get(cv2.CAP_PROP_FRAME_WIDTH)), int(cap.get(cv2.CAP_PROP_FRAME_HEIGHT)), int(cap.get(cv2.CAP_PROP_FPS))) # Define region points region_points = [(20, 1500), (1080, 1500), (1080, 1460), (20, 1460)] # Lower rectangle region counting # Video writer (MP4 format) video_writer = cv2.VideoWriter("object_counting_output.mp4", cv2.VideoWriter_fourcc(*"mp4v"), fps, (w, h)) # Init ObjectCounter counter = solutions.ObjectCounter( show=False, # Disable internal window display region=region_points, model="yolo11s.pt", ) # Process video while cap.isOpened(): success, im0 = cap.read() if not success: print("Video frame is empty or video processing has been successfully completed.") break im0 = counter.count(im0) # Resize to fit screen (optional — scale down for large videos) im0_resized = cv2.resize(im0, (640, 360)) # Adjust resolution as needed # Show the resized frame cv2.imshow("Object Counting", im0_resized) video_writer.write(im0) # Press 'q' to exit if cv2.waitKey(1) & 0xFF == ord('q'): break cap.release() video_writer.release() cv2.destroyAllWindows()
Output
YOLO v12
import cv2 from ultralytics import solutions cap = cv2.VideoCapture("highway.mp4") assert cap.isOpened(), "Error reading video file" w, h, fps = (int(cap.get(cv2.CAP_PROP_FRAME_WIDTH)), int(cap.get(cv2.CAP_PROP_FRAME_HEIGHT)), int(cap.get(cv2.CAP_PROP_FPS))) # Define region points region_points = [(20, 1500), (1080, 1500), (1080, 1460), (20, 1460)] # Lower rectangle region counting # Video writer (MP4 format) video_writer = cv2.VideoWriter("object_counting_output.mp4", cv2.VideoWriter_fourcc(*"mp4v"), fps, (w, h)) # Init ObjectCounter counter = solutions.ObjectCounter( show=False, # Disable internal window display region=region_points, model="yolo12s.pt", ) # Process video while cap.isOpened(): success, im0 = cap.read() if not success: print("Video frame is empty or video processing has been successfully completed.") break im0 = counter.count(im0) # Resize to fit screen (optional — scale down for large videos) im0_resized = cv2.resize(im0, (640, 360)) # Adjust resolution as needed # Show the resized frame cv2.imshow("Object Counting", im0_resized) video_writer.write(im0) # Press 'q' to exit if cv2.waitKey(1) & 0xFF == ord('q'): break cap.release() video_writer.release() cv2.destroyAllWindows()
Output
2. Heatmaps
YOLO v11
import cv2 from ultralytics import solutions cap = cv2.VideoCapture("mall_arial.mp4") assert cap.isOpened(), "Error reading video file" w, h, fps = (int(cap.get(x)) for x in (cv2.CAP_PROP_FRAME_WIDTH, cv2.CAP_PROP_FRAME_HEIGHT, cv2.CAP_PROP_FPS)) # Video writer video_writer = cv2.VideoWriter("heatmap_output_yolov11.mp4", cv2.VideoWriter_fourcc(*"mp4v"), fps, (w, h)) # In case you want to apply object counting + heatmaps, you can pass region points. # region_points = [(20, 400), (1080, 400)] # Define line points # region_points = [(20, 400), (1080, 400), (1080, 360), (20, 360)] # Define region points # region_points = [(20, 400), (1080, 400), (1080, 360), (20, 360), (20, 400)] # Define polygon points # Init heatmap heatmap = solutions.Heatmap( show=True, # Display the output model="yolo11s.pt", # Path to the YOLO11 model file colormap=cv2.COLORMAP_PARULA, # Colormap of heatmap # region=region_points, # If you want to do object counting with heatmaps, you can pass region_points # classes=[0, 2], # If you want to generate heatmap for specific classes i.e person and car. # show_in=True, # Display in counts # show_out=True, # Display out counts # line_width=2, # Adjust the line width for bounding boxes and text display ) # Process video while cap.isOpened(): success, im0 = cap.read() if not success: print("Video frame is empty or video processing has been successfully completed.") break im0 = heatmap.generate_heatmap(im0) im0_resized = cv2.resize(im0, (w, h)) video_writer.write(im0_resized) cap.release() video_writer.release() cv2.destroyAllWindows()
Output
YOLO v12
import cv2 from ultralytics import solutions cap = cv2.VideoCapture("mall_arial.mp4") assert cap.isOpened(), "Error reading video file" w, h, fps = (int(cap.get(x)) for x in (cv2.CAP_PROP_FRAME_WIDTH, cv2.CAP_PROP_FRAME_HEIGHT, cv2.CAP_PROP_FPS)) # Video writer video_writer = cv2.VideoWriter("heatmap_output_yolov12.mp4", cv2.VideoWriter_fourcc(*"mp4v"), fps, (w, h)) # In case you want to apply object counting + heatmaps, you can pass region points. # region_points = [(20, 400), (1080, 400)] # Define line points # region_points = [(20, 400), (1080, 400), (1080, 360), (20, 360)] # Define region points # region_points = [(20, 400), (1080, 400), (1080, 360), (20, 360), (20, 400)] # Define polygon points # Init heatmap heatmap = solutions.Heatmap( show=True, # Display the output model="yolo12s.pt", # Path to the YOLO11 model file colormap=cv2.COLORMAP_PARULA, # Colormap of heatmap # region=region_points, # If you want to do object counting with heatmaps, you can pass region_points # classes=[0, 2], # If you want to generate heatmap for specific classes i.e person and car. # show_in=True, # Display in counts # show_out=True, # Display out counts # line_width=2, # Adjust the line width for bounding boxes and text display ) # Process video while cap.isOpened(): success, im0 = cap.read() if not success: print("Video frame is empty or video processing has been successfully completed.") break im0 = heatmap.generate_heatmap(im0) im0_resized = cv2.resize(im0, (w, h)) video_writer.write(im0_resized) cap.release() video_writer.release() cv2.destroyAllWindows()
Output
3. Speed Estimation
YOLO v11
import cv2 from ultralytics import solutions import numpy as np cap = cv2.VideoCapture("cars_on_road.mp4") assert cap.isOpened(), "Error reading video file" # Capture video properties w = int(cap.get(cv2.CAP_PROP_FRAME_WIDTH)) h = int(cap.get(cv2.CAP_PROP_FRAME_HEIGHT)) fps = int(cap.get(cv2.CAP_PROP_FPS)) # Video writer video_writer = cv2.VideoWriter("speed_management_yolov11.mp4", cv2.VideoWriter_fourcc(*"mp4v"), fps, (w, h)) # Define speed region points (adjust for your video resolution) speed_region = [(300, h - 200), (w - 100, h - 200), (w - 100, h - 270), (300, h - 270)] # Initialize SpeedEstimator speed = solutions.SpeedEstimator( show=False, # Disable internal window display model="yolo11s.pt", # Path to the YOLO model file region=speed_region, # Pass region points # classes=[0, 2], # Optional: Filter specific object classes (e.g., cars, trucks) # line_width=2, # Optional: Adjust the line width ) # Process video while cap.isOpened(): success, im0 = cap.read() if not success: print("Video frame is empty or video processing has been successfully completed.") break # Estimate speed and draw bounding boxes out = speed.estimate_speed(im0) # Draw the speed region on the frame cv2.polylines(out, [np.array(speed_region)], isClosed=True, color=(0, 255, 0), thickness=2) # Resize the frame to fit the screen im0_resized = cv2.resize(out, (1280, 720)) # Resize for better screen fit # Show the resized frame cv2.imshow("Speed Estimation", im0_resized) video_writer.write(out) # Press 'q' to exit if cv2.waitKey(1) & 0xFF == ord('q'): break cap.release() video_writer.release() cv2.destroyAllWindows()
Output
YOLO v12
import cv2 from ultralytics import solutions import numpy as np cap = cv2.VideoCapture("cars_on_road.mp4") assert cap.isOpened(), "Error reading video file" # Capture video properties w = int(cap.get(cv2.CAP_PROP_FRAME_WIDTH)) h = int(cap.get(cv2.CAP_PROP_FRAME_HEIGHT)) fps = int(cap.get(cv2.CAP_PROP_FPS)) # Video writer video_writer = cv2.VideoWriter("speed_management_yolov12.mp4", cv2.VideoWriter_fourcc(*"mp4v"), fps, (w, h)) # Define speed region points (adjust for your video resolution) speed_region = [(300, h - 200), (w - 100, h - 200), (w - 100, h - 270), (300, h - 270)] # Initialize SpeedEstimator speed = solutions.SpeedEstimator( show=False, # Disable internal window display model="yolo12s.pt", # Path to the YOLO model file region=speed_region, # Pass region points # classes=[0, 2], # Optional: Filter specific object classes (e.g., cars, trucks) # line_width=2, # Optional: Adjust the line width ) # Process video while cap.isOpened(): success, im0 = cap.read() if not success: print("Video frame is empty or video processing has been successfully completed.") break # Estimate speed and draw bounding boxes out = speed.estimate_speed(im0) # Draw the speed region on the frame cv2.polylines(out, [np.array(speed_region)], isClosed=True, color=(0, 255, 0), thickness=2) # Resize the frame to fit the screen im0_resized = cv2.resize(out, (1280, 720)) # Resize for better screen fit # Show the resized frame cv2.imshow("Speed Estimation", im0_resized) video_writer.write(out) # Press 'q' to exit if cv2.waitKey(1) & 0xFF == ord('q'): break cap.release() video_writer.release() cv2.destroyAllWindows()
Output
Also Read: Top 30+ Computer Vision Models For 2025
Expert Opinions on YOLOv11 and YOLOv12
Muhammad Rizwan Munawar — Computer Vision Engineer at Ultralytics
“YOLOv12 introduces flash attention, which enhances accuracy, but it requires careful CUDA setup. It’s a solid step forward, especially for complex detection tasks, though YOLOv11 remains faster for real-time needs. In short, choose YOLOv12 for accuracy and YOLOv11 for speed.”
Linkedin Post – Is YOLOv12 really a state-of-the-art model? ?
Muhammad Rizwan, recently tested YOLOv11 and YOLOv12 side by side to break down their real-world performance. His findings highlight the trade-offs between the two models:
- Frames Per Second (FPS): YOLOv11 maintains an average of 40 FPS, while YOLOv12 lags behind at 30 FPS. This makes YOLOv11 the better choice for real-time applications where speed is critical, such as traffic monitoring or live video feeds.
- Training Time: YOLOv12 takes about 20% longer to train than YOLOv11. On a small dataset with 130 training images and 43 validation images, YOLOv11 completed training in 0.009 hours, while YOLOv12 needed 0.011 hours. While this might seem minor for small datasets, the difference becomes significant for larger-scale projects.
- Accuracy: Both models achieved similar accuracy after fine-tuning for 10 epochs on the same dataset. YOLOv12 didn’t dramatically outperform YOLOv11 in terms of accuracy, suggesting the newer model’s improvements lie more in architectural enhancements than raw detection precision.
- Flash Attention: YOLOv12 introduces flash attention, a powerful mechanism that speeds up and optimizes attention layers. However, there’s a catch — this feature isn’t natively supported on the CPU, and enabling it with CUDA requires careful version-specific setup. For teams without powerful GPUs or those working on edge devices, this can become a roadblock.
The PC specifications used for testing:
- GPU: NVIDIA RTX 3050
- CPU: Intel Core-i5-10400 @2.90GHz
- RAM: 64 GB
The model specifications:
- Model = YOLO11n.pt and YOLOv12n.pt
- Image size = 640 for inference
Conclusion
YOLO v12 marks a significant leap forward in real-time object detection, combining CNN speed with Transformer-like attention mechanisms. With improved accuracy, lower computational costs, and a range of model variants, YOLO v12 is poised to redefine the landscape of real-time vision applications. Whether for autonomous vehicles, security surveillance, or medical imaging, YOLO v12 sets a new standard for real-time object detection efficiency.
What’s Next?
- YOLO v13 Possibilities: Will future versions push the attention mechanisms even further?
- Edge Device Optimization: Can Flash Attention or Area Attention be optimized for lower-power devices?
To help you better understand the differences, I’ve attached some code snippets and output results in the comparison section. These examples illustrate how both YOLOv11 and YOLOv12 perform in real-world scenarios, from object counting to speed estimation and heatmaps. I’m excited to see how you guys perceive this new release! Are the improvements in accuracy and attention mechanisms enough to justify the trade-offs in speed? Or do you think YOLOv11 still holds its ground for most applications?
Ce qui précède est le contenu détaillé de. pour plus d'informations, suivez d'autres articles connexes sur le site Web de PHP en chinois!
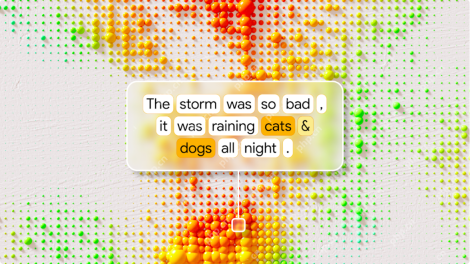
Explorer le fonctionnement interne des modèles de langue avec Gemma Scope Comprendre les complexités des modèles de langue IA est un défi important. La sortie de Google de Gemma Scope, une boîte à outils complète, offre aux chercheurs un moyen puissant de plonger
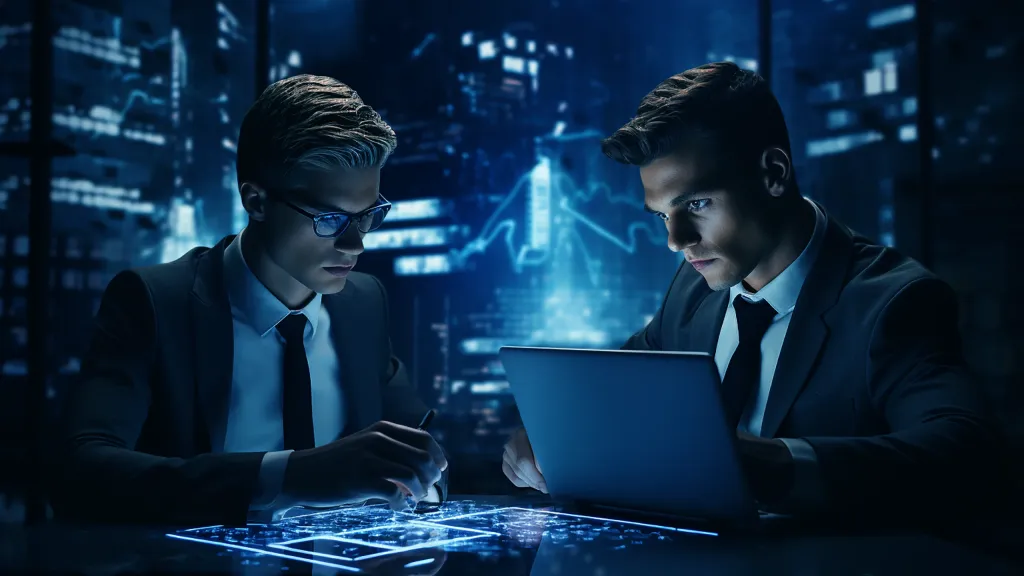
Déverrouiller le succès de l'entreprise: un guide pour devenir un analyste de Business Intelligence Imaginez transformer les données brutes en informations exploitables qui stimulent la croissance organisationnelle. C'est le pouvoir d'un analyste de Business Intelligence (BI) - un rôle crucial dans GU

Instruction ALTER TABLE de SQL: Ajout de colonnes dynamiquement à votre base de données Dans la gestion des données, l'adaptabilité de SQL est cruciale. Besoin d'ajuster votre structure de base de données à la volée? L'énoncé de la table alter est votre solution. Ce guide détaille l'ajout de Colu
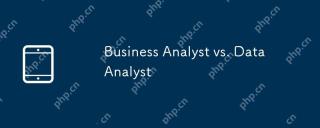
Introduction Imaginez un bureau animé où deux professionnels collaborent sur un projet critique. L'analyste commercial se concentre sur les objectifs de l'entreprise, l'identification des domaines d'amélioration et la garantie d'alignement stratégique sur les tendances du marché. Simulé
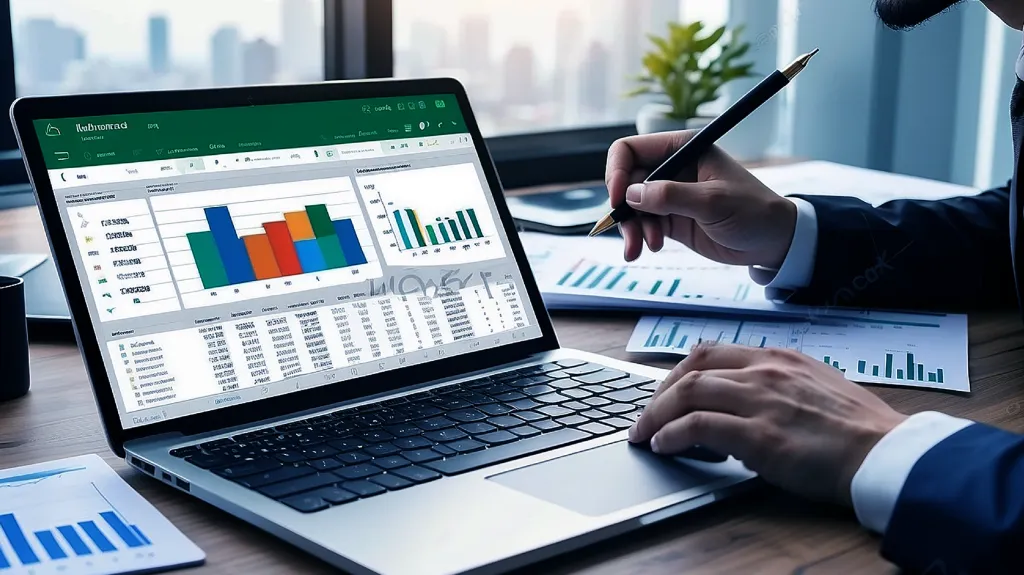
Excel Counting and Analysis: Explication détaillée du nombre et des fonctions de compte Le comptage et l'analyse des données précises sont essentiels dans Excel, en particulier lorsque vous travaillez avec de grands ensembles de données. Excel fournit une variété de fonctions pour y parvenir, les fonctions Count et Count sont des outils clés pour compter le nombre de cellules dans différentes conditions. Bien que les deux fonctions soient utilisées pour compter les cellules, leurs cibles de conception sont ciblées sur différents types de données. Faisons des détails spécifiques du comptage et des fonctions de coude, mettons en évidence leurs caractéristiques et différences uniques et apprenez à les appliquer dans l'analyse des données. Aperçu des points clés Comprendre le nombre et le cou
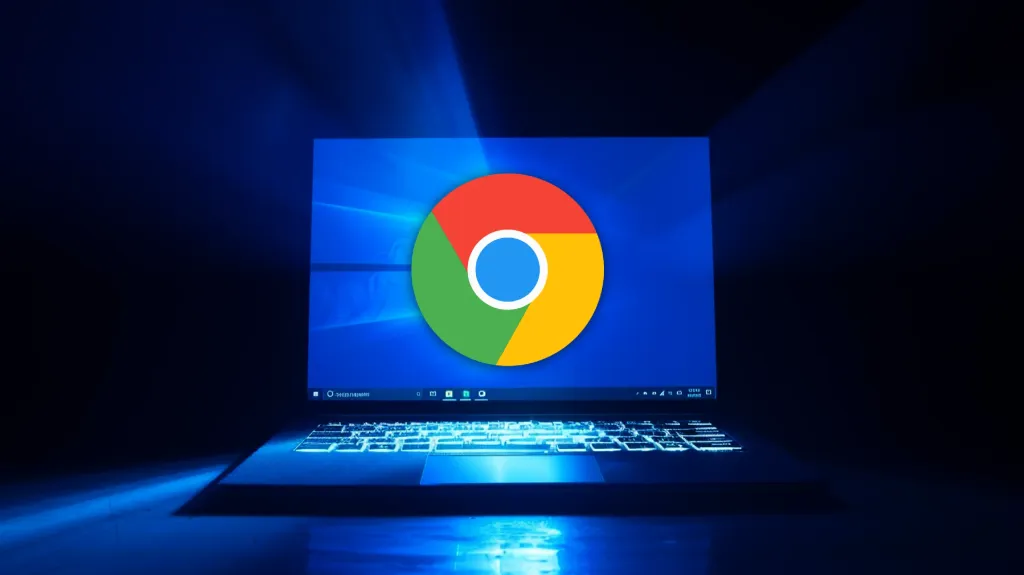
La révolution de l'IA de Google Chrome: une expérience de navigation personnalisée et efficace L'intelligence artificielle (IA) transforme rapidement notre vie quotidienne, et Google Chrome mène la charge dans l'arène de navigation Web. Cet article explore les exciti
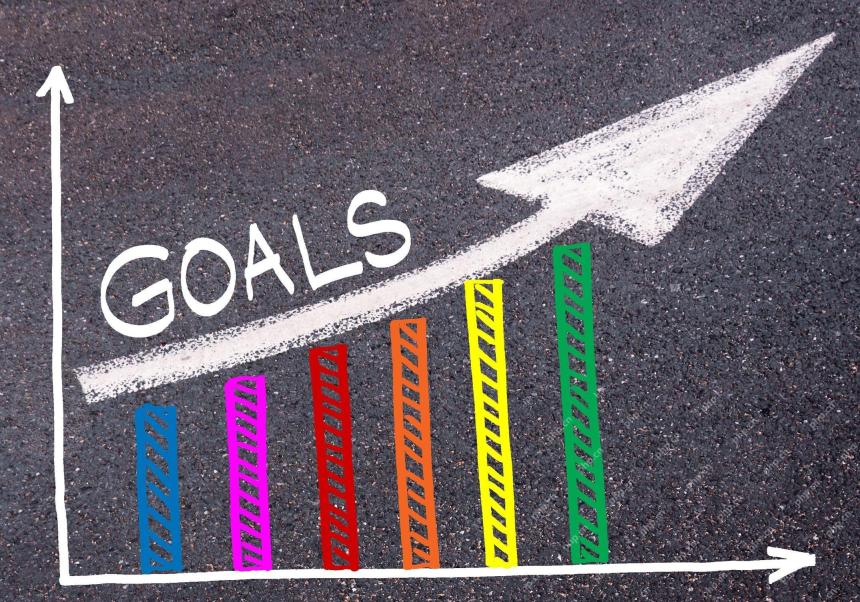
Réinventuation d'impact: le quadruple bas Pendant trop longtemps, la conversation a été dominée par une vision étroite de l’impact de l’IA, principalement axée sur le résultat du profit. Cependant, une approche plus holistique reconnaît l'interconnexion de BU
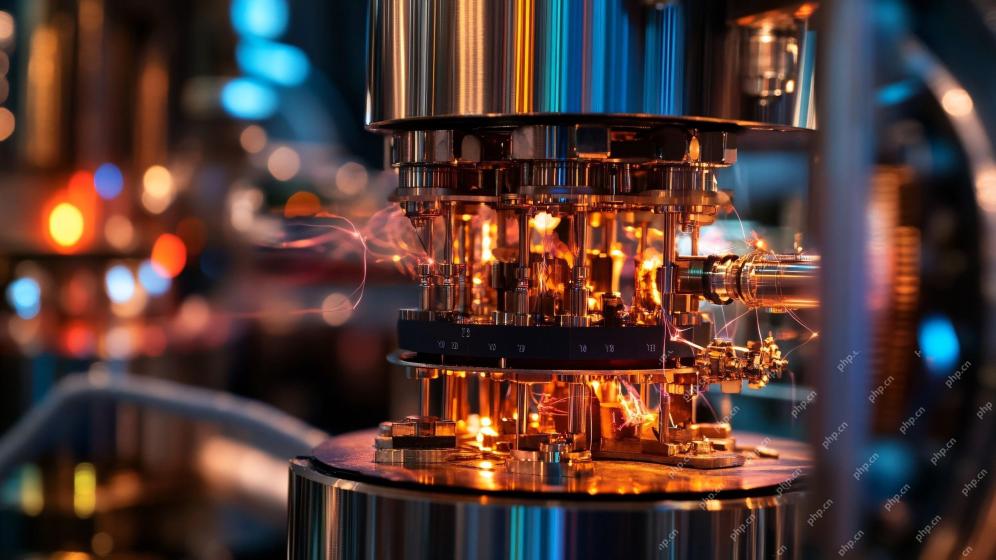
Les choses évoluent régulièrement vers ce point. L'investissement affluant dans les prestataires de services quantiques et les startups montre que l'industrie comprend son importance. Et un nombre croissant de cas d'utilisation réels émergent pour démontrer sa valeur


Outils d'IA chauds

Undresser.AI Undress
Application basée sur l'IA pour créer des photos de nu réalistes

AI Clothes Remover
Outil d'IA en ligne pour supprimer les vêtements des photos.

Undress AI Tool
Images de déshabillage gratuites

Clothoff.io
Dissolvant de vêtements AI

AI Hentai Generator
Générez AI Hentai gratuitement.

Article chaud

Outils chauds
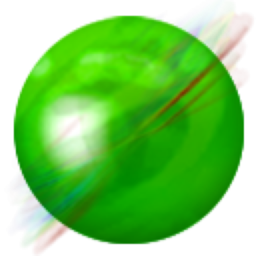
ZendStudio 13.5.1 Mac
Puissant environnement de développement intégré PHP

Envoyer Studio 13.0.1
Puissant environnement de développement intégré PHP
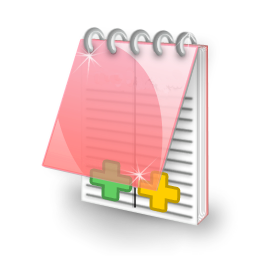
Version crackée d'EditPlus en chinois
Petite taille, coloration syntaxique, ne prend pas en charge la fonction d'invite de code

Navigateur d'examen sécurisé
Safe Exam Browser est un environnement de navigation sécurisé permettant de passer des examens en ligne en toute sécurité. Ce logiciel transforme n'importe quel ordinateur en poste de travail sécurisé. Il contrôle l'accès à n'importe quel utilitaire et empêche les étudiants d'utiliser des ressources non autorisées.
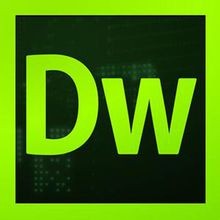
Dreamweaver CS6
Outils de développement Web visuel