Achetez-moi un café☕
*Mon message explique MS COCO.
CocoDetection() peut utiliser l'ensemble de données MS COCO comme indiqué ci-dessous :
*Mémos :
- Le 1er argument est root(Required-Type:str ou pathlib.Path) :
*Mémos :
- C'est le chemin vers les images.
- Un chemin absolu ou relatif est possible.
- Le 2ème argument est annFile(Required-Type:str ou pathlib.Path) :
*Mémos :
- C'est le chemin d'accès aux annotations.
- Un chemin absolu ou relatif est possible.
- Le 3ème argument est transform(Optional-Default:None-Type:callable).
- Le 4ème argument est target_transform(Optional-Default:None-Type:callable).
- Le 5ème argument est transforms(Optional-Default:None-Type:callable).
from torchvision.datasets import CocoDetection cap_train2014_data = CocoDetection( root="data/coco/imgs/train2014", annFile="data/coco/anns/trainval2014/captions_train2014.json" ) cap_train2014_data = CocoDetection( root="data/coco/imgs/train2014", annFile="data/coco/anns/trainval2014/captions_train2014.json", transform=None, target_transform=None, transforms=None ) ins_train2014_data = CocoDetection( root="data/coco/imgs/train2014", annFile="data/coco/anns/trainval2014/instances_train2014.json" ) pk_train2014_data = CocoDetection( root="data/coco/imgs/train2014", annFile="data/coco/anns/trainval2014/person_keypoints_train2014.json" ) len(cap_train2014_data), len(ins_train2014_data), len(pk_train2014_data) # (82783, 82783, 82783) cap_val2014_data = CocoDetection( root="data/coco/imgs/val2014", annFile="data/coco/anns/trainval2014/captions_val2014.json" ) ins_val2014_data = CocoDetection( root="data/coco/imgs/val2014", annFile="data/coco/anns/trainval2014/instances_val2014.json" ) pk_val2014_data = CocoDetection( root="data/coco/imgs/val2014", annFile="data/coco/anns/trainval2014/person_keypoints_val2014.json" ) len(cap_val2014_data), len(ins_val2014_data), len(pk_val2014_data) # (40504, 40504, 40504) test2014_data = CocoDetection( root="data/coco/imgs/test2014", annFile="data/coco/anns/test2014/test2014.json" ) test2015_data = CocoDetection( root="data/coco/imgs/test2015", annFile="data/coco/anns/test2015/test2015.json" ) testdev2015_data = CocoDetection( root="data/coco/imgs/test2015", annFile="data/coco/anns/test2015/test-dev2015.json" ) len(test2014_data), len(test2015_data), len(testdev2015_data) # (40775, 81434, 20288) cap_train2014_data # Dataset CocoDetection # Number of datapoints: 82783 # Root location: data/coco/imgs/train2014 cap_train2014_data.root # 'data/coco/imgs/train2014' print(cap_train2014_data.transform) # None print(cap_train2014_data.target_transform) # None print(cap_train2014_data.transforms) # None cap_train2014_data[0] # (<pil.image.image image mode="RGB" size="640x480">, # [{'image_id': 9, 'id': 661611, # 'caption': 'Closeup of bins of food that include broccoli and bread.'}, # {'image_id': 9, 'id': 661977, # 'caption': 'A meal is presented in brightly colored plastic trays.'}, # {'image_id': 9, 'id': 663627, # 'caption': 'there are containers filled with different kinds of foods'}, # {'image_id': 9, 'id': 666765, # 'caption': 'Colorful dishes holding meat, vegetables, fruit, and bread.'}, # {'image_id': 9, 'id': 667602, # 'caption': 'A bunch of trays that have different food.'}]) cap_train2014_data[1] # (<pil.image.image image mode="RGB" size="640x426">, # [{'image_id': 25, 'id': 122312, # 'caption': 'A giraffe eating food from the top of the tree.'}, # {'image_id': 25, 'id': 127076, # 'caption': 'A giraffe standing up nearby a tree '}, # {'image_id': 25, 'id': 127238, # 'caption': 'A giraffe mother with its baby in the forest.'}, # {'image_id': 25, 'id': 133058, # 'caption': 'Two giraffes standing in a tree filled area.'}, # {'image_id': 25, 'id': 133676, # 'caption': 'A giraffe standing next to a forest filled with trees.'}]) cap_train2014_data[2] # (<pil.image.image image mode="RGB" size="640x428">, # [{'image_id': 30, 'id': 695774, # 'caption': 'A flower vase is sitting on a porch stand.'}, # {'image_id': 30, 'id': 696557, # 'caption': 'White vase with different colored flowers sitting inside of it. '}, # {'image_id': 30, 'id': 699041, # 'caption': 'a white vase with many flowers on a stage'}, # {'image_id': 30, 'id': 701216, # 'caption': 'A white vase filled with different colored flowers.'}, # {'image_id': 30, 'id': 702428, # 'caption': 'A vase with red and white flowers outside on a sunny day.'}]) ins_train2014_data[0] # (<pil.image.image image mode="RGB" size="640x480">, # [{'segmentation': [[500.49, 473.53, 599.73, ..., 20.49, 473.53]], # 'area': 120057.13925, 'iscrowd': 0, 'image_id': 9, # 'bbox': [1.08, 187.69, 611.59, 285.84], 'category_id': 51, # 'id': 1038967}, # {'segmentation': ..., 'category_id': 51, 'id': 1039564}, # ..., # {'segmentation': ..., 'category_id': 55, 'id': 1914001}]) ins_train2014_data[1] # (<pil.image.image image mode="RGB" size="640x426">, # [{'segmentation': [[437.52, 353.33, 437.87, ..., 437.87, 357.19]], # 'area': 19686.597949999996, 'iscrowd': 0, 'image_id': 25, # 'bbox': [385.53, 60.03, 214.97, 297.16], 'category_id': 25, # 'id': 598548}, # {'segmentation': [[99.26, 405.72, 133.57, ..., 97.77, 406.46]], # 'area': 2785.8475500000004, 'iscrowd': 0, 'image_id': 25, # 'bbox': [53.01, 356.49, 132.03, 55.19], 'category_id': 25, # 'id': 599491}]) ins_train2014_data[2] # (<pil.image.image image mode="RGB" size="640x428">, # [{'segmentation': [[267.38, 330.14, 281.81, ..., 269.3, 329.18]], # 'area': 47675.66289999999, 'iscrowd': 0, 'image_id': 30, # 'bbox': [204.86, 31.02, 254.88, 324.12], 'category_id': 64, # 'id': 291613}, # {'segmentation': [[394.34, 155.81, 403.96, ..., 393.38, 157.73]], # 'area': 16202.798250000003, 'iscrowd': 0, 'image_id': 30, # 'bbox': [237.56, 155.81, 166.4, 195.25], 'category_id': 86, # 'id': 1155486}]) pk_train2014_data[0] # (<pil.image.image image mode="RGB" size="640x480">, []) pk_train2014_data[1] # (<pil.image.image image mode="RGB" size="640x426">, []) pk_train2014_data[2] # (<pil.image.image image mode="RGB" size="640x428">, []) cap_val2014_data[0] # (<pil.image.image image mode="RGB" size="640x478">, # [{'image_id': 42, 'id': 641613, # 'caption': 'This wire metal rack holds several pairs of shoes and sandals'}, # {'image_id': 42, 'id': 645309, # 'caption': 'A dog sleeping on a show rack in the shoes.'}, # {'image_id': 42, 'id': 650217, # 'caption': 'Various slides and other footwear rest in a metal basket outdoors.'}, # {'image_id': 42, # 'id': 650868, # 'caption': 'A small dog is curled up on top of the shoes'}, # {'image_id': 42, # 'id': 652383, # 'caption': 'a shoe rack with some shoes and a dog sleeping on them'}]) cap_val2014_data[1] # (<pil.image.image image mode="RGB" size="565x640">, # [{'image_id': 73, 'id': 593422, # 'caption': 'A motorcycle parked in a parking space next to another motorcycle.'}, # {'image_id': 73, 'id': 746071, # 'caption': 'An old motorcycle parked beside other motorcycles with a brown leather seat.'}, # {'image_id': 73, 'id': 746170, # 'caption': 'Motorcycle parked in the parking lot of asphalt.'}, # {'image_id': 73, 'id': 746914, # 'caption': 'A close up view of a motorized bicycle, sitting in a rack. '}, # {'image_id': 73, 'id': 748185, # 'caption': 'The back tire of an old style motorcycle is resting in a metal stand. '}]) cap_val2014_data[2] # (<pil.image.image image mode="RGB" size="640x426">, # [{'image_id': 74, 'id': 145996, # 'caption': 'A picture of a dog laying on the ground.'}, # {'image_id': 74, 'id': 146710, # 'caption': 'Dog snoozing by a bike on the edge of a cobblestone street'}, # {'image_id': 74, 'id': 149398, # 'caption': 'The white dog lays next to the bicycle on the sidewalk.'}, # {'image_id': 74, 'id': 149638, # 'caption': 'a white dog is sleeping on a street and a bicycle'}, # {'image_id': 74, 'id': 150181, # 'caption': 'A puppy rests on the street next to a bicycle.'}]) ins_val2014_data[0] # (<pil.image.image image mode="RGB" size="640x478">, # [{'segmentation': [[382.48, 268.63, 330.24, ..., 394.09, 264.76]], # 'area': 53481.5118, 'iscrowd': 0, 'image_id': 42, # 'bbox': [214.15, 41.29, 348.26, 243.78], 'category_id': 18, # 'id': 1817255}]) ins_val2014_data[1] # (<pil.image.image image mode="RGB" size="565x640">, # [{'segmentation': [[134.36, 145.55, 117.02, ..., 138.69, 141.22]], # 'area': 172022.43864999997, 'iscrowd': 0, 'image_id': 73, # 'bbox': [13.0, 22.75, 535.98, 609.67], 'category_id': 4, # 'id': 246920}, # {'segmentation': [[202.28, 4.97, 210.57, 26.53, ..., 192.33, 3.32]], # 'area': 52666.3402, 'iscrowd': 0, 'image_id': 73, # 'bbox': [1.66, 3.32, 268.6, 271.91], 'category_id': 4, # 'id': 2047387}]) ins_val2014_data[2] # (<pil.image.image image mode="RGB" size="640x426">, # [{'segmentation': [[321.02, 321.0, 314.25, ..., 320.57, 322.86]], # 'area': 18234.62355, 'iscrowd': 0, 'image_id': 74, # 'bbox': [61.87, 276.25, 296.42, 103.18], 'category_id': 18, # 'id': 1774}, # {'segmentation': ..., 'category_id': 2, 'id': 128367}, # ... # {'segmentation': ..., 'category_id': 1, 'id': 1751664}]) pk_val2014_data[0] # (<pil.image.image image mode="RGB" size="640x478">, []) pk_val2014_data[1] # (<pil.image.image image mode="RGB" size="565x640">, []) pk_val2014_data[2] # (<pil.image.image image mode="RGB" size="640x426">, # [{'segmentation': [[301.32, 93.96, 305.72, ..., 299.67, 94.51]], # 'num_keypoints': 0, 'area': 638.7158, 'iscrowd': 0, # 'keypoints': [0, 0, 0, 0, ..., 0, 0], 'image_id': 74, # 'bbox': [295.55, 93.96, 18.42, 58.83], 'category_id': 1, # 'id': 195946}, # {'segmentation': ..., 'category_id': 1, 'id': 253933}, # ... # {'segmentation': ..., 'category_id': 1, 'id': 1751664}]) test2014_data[0] # (<pil.image.image image mode="RGB" size="640x480">, []) test2014_data[1] # (<pil.image.image image mode="RGB" size="480x640">, []) test2014_data[2] # (<pil.image.image image mode="RGB" size="480x640">, []) test2015_data[0] # (<pil.image.image image mode="RGB" size="640x480">, []) test2015_data[1] # (<pil.image.image image mode="RGB" size="480x640">, []) test2015_data[2] # (<pil.image.image image mode="RGB" size="480x640">, []) testdev2015_data[0] # (<pil.image.image image mode="RGB" size="640x480">, []) testdev2015_data[1] # (<pil.image.image image mode="RGB" size="480x640">, []) testdev2015_data[2] # (<pil.image.image image mode="RGB" size="640x427">, []) import matplotlib.pyplot as plt from matplotlib.patches import Polygon, Rectangle import torch def show_images(data, main_title=None): file = data.root.split('/')[-1] if data[0][1] and "caption" in data[0][1][0]: if file == "train2014": plt.figure(figsize=(14, 5)) plt.suptitle(t=main_title, y=0.9, fontsize=14) x_axis = 0.02 x_axis_incr = 0.325 fs = 10.5 elif file == "val2014": plt.figure(figsize=(14, 6.5)) plt.suptitle(t=main_title, y=0.94, fontsize=14) x_axis = 0.01 x_axis_incr = 0.32 fs = 9.4 for i, (im, ann) in zip(range(1, 4), data): plt.subplot(1, 3, i) plt.imshow(X=im) plt.title(label=ann[0]["image_id"]) y_axis = 0.0 for j in range(0, 5): plt.figtext(x=x_axis, y=y_axis, fontsize=fs, s=f'{ann[j]["id"]}:\n{ann[j]["caption"]}') if file == "train2014": y_axis -= 0.1 elif file == "val2014": y_axis -= 0.07 x_axis += x_axis_incr if i == 2 and file == "val2014": x_axis += 0.06 plt.tight_layout() plt.show() elif data[0][1] and "segmentation" in data[0][1][0]: if file == "train2014": fig, axes = plt.subplots(nrows=1, ncols=3, figsize=(14, 4)) elif file == "val2014": fig, axes = plt.subplots(nrows=1, ncols=3, figsize=(14, 5)) fig.suptitle(t=main_title, y=1.0, fontsize=14) for (im, anns), axis in zip(data, axes.ravel()): for ann in anns: for seg in ann['segmentation']: seg_tsors = torch.tensor(seg).split(2) seg_lists = [seg_tsor.tolist() for seg_tsor in seg_tsors] poly = Polygon(xy=seg_lists, facecolor="lightgreen", alpha=0.7) axis.add_patch(p=poly) px = [] py = [] for j, v in enumerate(seg): if j%2 == 0: px.append(v) else: py.append(v) axis.plot(px, py, color='yellow') x, y, w, h = ann['bbox'] rect = Rectangle(xy=(x, y), width=w, height=h, linewidth=3, edgecolor='r', facecolor='none', zorder=2) axis.add_patch(p=rect) axis.imshow(X=im) axis.set_title(label=anns[0]["image_id"]) fig.tight_layout() plt.show() elif not data[0][1]: if file == "train2014": plt.figure(figsize=(14, 5)) plt.suptitle(t=main_title, y=0.9, fontsize=14) elif file == "val2014": plt.figure(figsize=(14, 5)) plt.suptitle(t=main_title, y=1.05, fontsize=14) elif file == "test2014" or "test2015": plt.figure(figsize=(14, 8)) plt.suptitle(t=main_title, y=0.9, fontsize=14) for i, (im, _) in zip(range(1, 4), data): plt.subplot(1, 3, i) plt.imshow(X=im) plt.tight_layout() plt.show() show_images(data=cap_train2014_data, main_title="cap_train2014_data") show_images(data=ins_train2014_data, main_title="ins_train2014_data") show_images(data=pk_train2014_data, main_title="pk_train2014_data") show_images(data=cap_val2014_data, main_title="cap_val2014_data") show_images(data=ins_val2014_data, main_title="ins_val2014_data") show_images(data=pk_val2014_data, main_title="pk_val2014_data") show_images(data=test2014_data, main_title="test2014_data") show_images(data=test2015_data, main_title="test2015_data") show_images(data=testdev2015_data, main_title="testdev2015_data") </pil.image.image></pil.image.image></pil.image.image></pil.image.image></pil.image.image></pil.image.image></pil.image.image></pil.image.image></pil.image.image></pil.image.image></pil.image.image></pil.image.image></pil.image.image></pil.image.image></pil.image.image></pil.image.image></pil.image.image></pil.image.image></pil.image.image></pil.image.image></pil.image.image></pil.image.image></pil.image.image></pil.image.image></pil.image.image></pil.image.image></pil.image.image>
Ce qui précède est le contenu détaillé de. pour plus d'informations, suivez d'autres articles connexes sur le site Web de PHP en chinois!
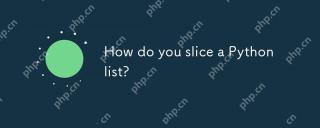
SlitingyPapyThonListIsDoneUsingTheSyntaxList [Démarrage: arrêt: étape] .He'showitworks: 1) startisheindexofthefirStelementoinclude.2) stopisTheIndexoftheFirstelementsoexclude.3) StepistheincrementBetweenselans.it'susefulfactingPortationSoListShsandCanusegeg
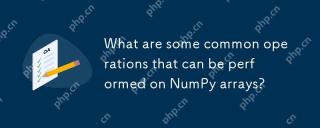
NumpyAllowsForvariousOperations ONARRAYS: 1) BasicarithmeticLikeaddition, Soustraction, Multiplication, anddivision; 2) AdvancedOperationSuchasmatrixMultiplication; 3) Element-Wiseoperations withoutExplicitloop
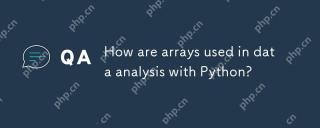
ArraySinpython, en particulier ThroughNumpyandPandas, aressentialfordataanalysis, offingspeeedAfficiency.1) numpyarrayablefficienthandlingoflargedatasetsandComplexOperationsLikEMoVingAverages.2)
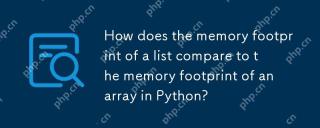
ListsandNumpyArraysInpythonHaveDidifferentMemoryfootprints: listsaRemoreFlexibles Butlessmemory économe, tandis que la liste de résensés est-ce qui
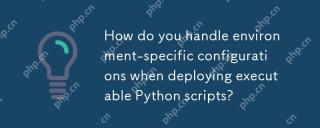
ToenSurepythonscriptsBeHavecorrectlyAcrossDevelopment, mise en scène et production, catégories de type: 1) EnvironmentVariblesForsImplesettings, 2) ConfigurationFilesForComplexsetups et3) dynamicloadingforadaptability.eachMethodoffersNebeneFitsAndreCeresca
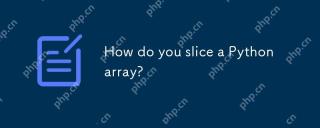
La syntaxe de base pour le découpage de la liste Python est la liste [Démarrage: arrêt: étape]. 1.Start est le premier index d'élément inclus, 2.STOP est le premier indice d'élément exclu et 3.StEP détermine la taille de l'étape entre les éléments. Les tranches sont non seulement utilisées pour extraire les données, mais aussi pour modifier et inverser les listes.
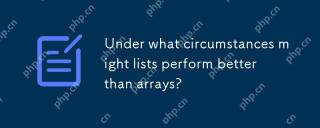
ListesoutPerformarRaySin: 1) dynamicingizingandfrequentinSertions / Deletions, 2) StoringheteroGeneousData, and3) MemoryEfficiencyForsparsedata, butmayhaveslightperformanceCostSincertorations.
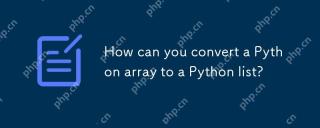
Toconvertapythonarraytoalist, usethelist () Constructororageneratorexpression.1) ImportTheArrayModuleandCreateArray.2) Uselist (Arr) ou [Xforxinarr] à Convertittoalist, considérant la performance et le domaine de l'émie-efficacité pour les étages.


Outils d'IA chauds

Undresser.AI Undress
Application basée sur l'IA pour créer des photos de nu réalistes

AI Clothes Remover
Outil d'IA en ligne pour supprimer les vêtements des photos.

Undress AI Tool
Images de déshabillage gratuites

Clothoff.io
Dissolvant de vêtements AI

Video Face Swap
Échangez les visages dans n'importe quelle vidéo sans effort grâce à notre outil d'échange de visage AI entièrement gratuit !

Article chaud

Outils chauds

Bloc-notes++7.3.1
Éditeur de code facile à utiliser et gratuit
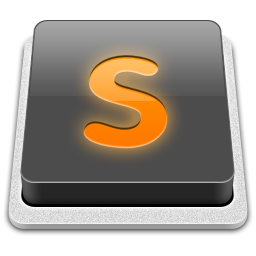
SublimeText3 version Mac
Logiciel d'édition de code au niveau de Dieu (SublimeText3)

Listes Sec
SecLists est le compagnon ultime du testeur de sécurité. Il s'agit d'une collection de différents types de listes fréquemment utilisées lors des évaluations de sécurité, le tout en un seul endroit. SecLists contribue à rendre les tests de sécurité plus efficaces et productifs en fournissant facilement toutes les listes dont un testeur de sécurité pourrait avoir besoin. Les types de listes incluent les noms d'utilisateur, les mots de passe, les URL, les charges utiles floues, les modèles de données sensibles, les shells Web, etc. Le testeur peut simplement extraire ce référentiel sur une nouvelle machine de test et il aura accès à tous les types de listes dont il a besoin.

SublimeText3 version chinoise
Version chinoise, très simple à utiliser
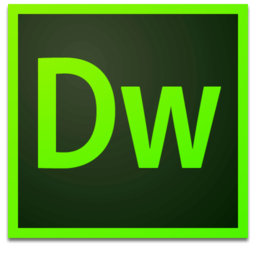
Dreamweaver Mac
Outils de développement Web visuel
