本文实例讲述了python连接oracle数据库的方法,分享给大家供大家参考。具体步骤如下:
一、首先下载驱动:(cx_Oracle)
http://www.python.net/crew/atuining/cx_Oracle/
不过要注意一下版本,根据你的情况加以选择。
二、安装:
首先配置oracle_home环境变量
执行那个exe安装程序就可以了,它会copy一个cx_Oracle.pyd到Libsite-packages目录下。
如果是linux,执行
代码如下:
python setup.py build
python setup.py install
三、执行一段测试程序:
代码如下:
import cx_Oracle
con = cx_Oracle.connect( "xjtu_test", "37343734","xjtu.world")
cursor = con.cursor()
cursor.close()
con.close()
里边connect中的3个参数从左到右分别是:user, pass, TNS。
那个TNS可以用Oracle客户端工具中的Net Configuration Assistant来配置。
四、具体的cx_Oracle API可以参考:
http://www.python.net/crew/atuining/cx_Oracle/html/cx_Oracle.html
五、示例:
代码如下:
>>> import cx_Oracle
>>> conn=cx_Oracle.connect ('scott/tiger@oratest')
>>> curs=conn.cursor ()
>>> sql='select * from emp'
>>> rr=curs.execute (sql)
>>> row=curs.fetchone()
>>> row
(7369, 'SMITH', 'CLERK', 7902, datetime.datetime(1980, 12, 17, 0, 0), 800.0, None, 20)
>>> while row:
(ID,NAME)=(row[0],row[1])
row=curs.fetchone ()
print ID,NAME
7369 SMITH
7499 ALLEN
7521 WARD
7566 JONES
7654 MARTIN
7698 BLAKE
7782 CLARK
7788 SCOTT
7839 KING
7844 TURNER
7876 ADAMS
7900 JAMES
7902 日常
7934 MILLER
如果使用windows平台,执行那段测试代码时你肯定遇到问题了,一般会有以下问题:
① import cx_Oracle 时报告找不到OCI.DLL:
到装了Oracle的机器上找一个,然后copy到Libsite-packages目录下就可以了。
② cx_Oracle.connect 时报告RuntimeError: Unable to acquire Oracle environment handle:
这个比较麻烦,按以下步骤来解决:(可能不需要所有的步骤,我没有确认,不过把以下步骤都执行了,确实问题就解决了)
首先,确认你是在控制台下边来执行这个python脚本的。而不是某些ide,例如:PyDev(它们似乎无法载入os的环境变量)。
其实,在本机安装Oracle(只安客户端工具就可以了)。
最后,添加以下环境变量:(我给出我的,换成你自己的路径就可以了)
代码如下:
ORACLE_HOME=D:OracleOra81
PATH=D:OracleOra81bin;
希望本文所述对大家的Python程序设计有所帮助。
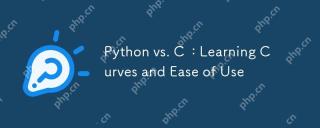
Python is easier to learn and use, while C is more powerful but complex. 1. Python syntax is concise and suitable for beginners. Dynamic typing and automatic memory management make it easy to use, but may cause runtime errors. 2.C provides low-level control and advanced features, suitable for high-performance applications, but has a high learning threshold and requires manual memory and type safety management.
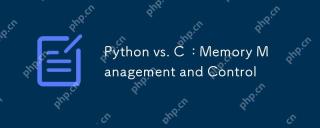
Python and C have significant differences in memory management and control. 1. Python uses automatic memory management, based on reference counting and garbage collection, simplifying the work of programmers. 2.C requires manual management of memory, providing more control but increasing complexity and error risk. Which language to choose should be based on project requirements and team technology stack.
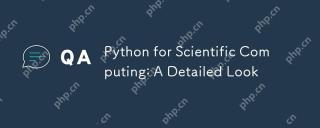
Python's applications in scientific computing include data analysis, machine learning, numerical simulation and visualization. 1.Numpy provides efficient multi-dimensional arrays and mathematical functions. 2. SciPy extends Numpy functionality and provides optimization and linear algebra tools. 3. Pandas is used for data processing and analysis. 4.Matplotlib is used to generate various graphs and visual results.
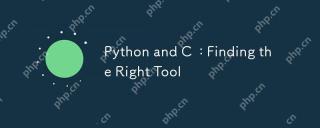
Whether to choose Python or C depends on project requirements: 1) Python is suitable for rapid development, data science, and scripting because of its concise syntax and rich libraries; 2) C is suitable for scenarios that require high performance and underlying control, such as system programming and game development, because of its compilation and manual memory management.
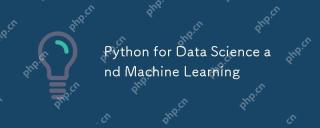
Python is widely used in data science and machine learning, mainly relying on its simplicity and a powerful library ecosystem. 1) Pandas is used for data processing and analysis, 2) Numpy provides efficient numerical calculations, and 3) Scikit-learn is used for machine learning model construction and optimization, these libraries make Python an ideal tool for data science and machine learning.
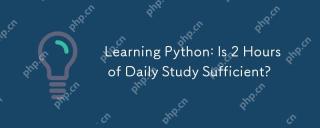
Is it enough to learn Python for two hours a day? It depends on your goals and learning methods. 1) Develop a clear learning plan, 2) Select appropriate learning resources and methods, 3) Practice and review and consolidate hands-on practice and review and consolidate, and you can gradually master the basic knowledge and advanced functions of Python during this period.
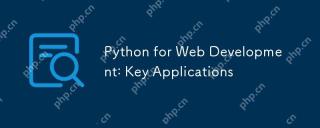
Key applications of Python in web development include the use of Django and Flask frameworks, API development, data analysis and visualization, machine learning and AI, and performance optimization. 1. Django and Flask framework: Django is suitable for rapid development of complex applications, and Flask is suitable for small or highly customized projects. 2. API development: Use Flask or DjangoRESTFramework to build RESTfulAPI. 3. Data analysis and visualization: Use Python to process data and display it through the web interface. 4. Machine Learning and AI: Python is used to build intelligent web applications. 5. Performance optimization: optimized through asynchronous programming, caching and code
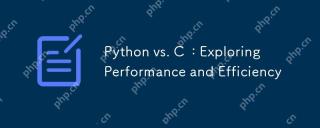
Python is better than C in development efficiency, but C is higher in execution performance. 1. Python's concise syntax and rich libraries improve development efficiency. 2.C's compilation-type characteristics and hardware control improve execution performance. When making a choice, you need to weigh the development speed and execution efficiency based on project needs.


Hot AI Tools

Undresser.AI Undress
AI-powered app for creating realistic nude photos

AI Clothes Remover
Online AI tool for removing clothes from photos.

Undress AI Tool
Undress images for free

Clothoff.io
AI clothes remover

Video Face Swap
Swap faces in any video effortlessly with our completely free AI face swap tool!

Hot Article

Hot Tools

Atom editor mac version download
The most popular open source editor

SublimeText3 Linux new version
SublimeText3 Linux latest version
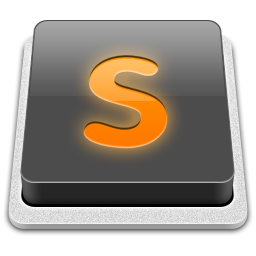
SublimeText3 Mac version
God-level code editing software (SublimeText3)

SublimeText3 English version
Recommended: Win version, supports code prompts!

SAP NetWeaver Server Adapter for Eclipse
Integrate Eclipse with SAP NetWeaver application server.