


Finally, someone investigated the overfitting of small models: two-thirds of them had data pollution, and Microsoft Phi-3 and Mixtral 8x22B were named
Improving the reasoning capabilities of large language models is one of the most important directions of current research. In this type of task, many small models recently released seem to perform well, and Can handle this type of task well. For example, Microsoft's Phi-3, Mistral 8x22B and other models.
Researchers pointed out that there is a key problem in the current field of large model research: many studies fail to accurately benchmark the capabilities of existing LLMs. This suggests that we need to spend more time evaluating and testing the current LLM capability level.
This is because most current research uses GSM8k, MATH, MBPP, HumanEval, SWEBench and other test sets as benchmarks. Since the model is trained on a large data set scraped from the Internet, the training data set may contain samples that are highly similar to the questions in the benchmark.
This kind of contamination may cause the model's reasoning ability to be incorrectly evaluated - They may simply be confused by the question during the training process and happen to recite the correct answer.
Just now, a paper by Scale AI conducted an in-depth survey of the most popular large models, including OpenAI’s GPT-4, Gemini, Claude, Mistral, Llama, Phi, Abdin Models with different parameter quantities under other series.
The test results confirmed a widespread suspicion: many models were contaminated by benchmark data.
- ##Paper title: A Careful Examination of Large Language Model Performance on Grade School Arithmetic
- Paper link: https://arxiv.org/pdf/2405.00332
Especially the Mistral and Phi model series, which are known for their small quantity and high quality. According to the test results of GSM1k, almost all versions of them show consistent evidence of overfitting.
Further analysis found that there is a positive correlation between the probability of the model generating GSM8k samples and its performance gap between GSM8k and GSM1k (correlation coefficient r^2 = 0.32). This strongly suggests that the main cause of overfitting is that the model partially misjudges the samples in GSM8k.
Furthermore, all models, including the most overfitted model, were still able to successfully generalize to new elementary school math problems, although sometimes with lower success rates than indicated by their baseline data.
Scale AI does not currently plan to publicly release GSM1k to prevent similar data contamination issues from occurring in the future. They plan to conduct regular ongoing evaluations of all major open and closed source LLMs, and will also open source the evaluation code so that subsequent studies can replicate the results in the paper.
GSM1k contains 1250 elementary school math problems. These problems can be solved with only basic mathematical reasoning. Scale AI showed each human annotator 3 sample questions from GSM8k and asked them to ask new questions of similar difficulty, resulting in the GSM1k data set. The researchers asked the human annotators not to use any advanced mathematical concepts and only use basic arithmetic (addition, subtraction, multiplication and division) to formulate questions. Like GSM8k, the solutions to all problems are positive integers. No language model was used in the construction of the GSM1k dataset. In order to avoid the data pollution problem of the GSM1k dataset, Scale AI will not publicly release the dataset at present, but will open source the GSM1k evaluation framework, which is based on EleutherAI's LM Evaluation Harness. But Scale AI promises that will release the complete GSM1k data set under the MIT license after reaching one of the following two conditions: (1) There are three based on different pre-training bases The open source model of model lineage reaches 95% accuracy on GSM1k; (2) by the end of 2025. At that point, it is likely that elementary school mathematics will no longer be a valid benchmark for assessing LLM performance. To evaluate proprietary models, researchers will publish data sets via an API. The reason for this release approach is that the authors believe that LLM vendors generally do not use API data points to train model models. Nonetheless, if GSM1k data is leaked through the API, the authors of the paper have also retained data points that do not appear in the final GSM1k data set. These backup data points will be released with GSM1k when the above conditions are met. They hope that future benchmark releases will follow a similar pattern - not releasing them publicly at first, but pre-promising to release them at a future date or when certain conditions are met to prevent manipulation. In addition, although Scale AI strives to ensure maximum consistency between GSM8k and GSM1k. But the test set of GSM8k has been publicly released and widely used for model testing, so GSM1k and GSM8k are only approximations under ideal conditions. The following evaluation results are obtained when the distributions of GSM8k and GSM1k are not exactly the same. Evaluation results To evaluate the model, the researchers used the LM Evaluation Harness branch of EleutherAI and used the default settings. The running prompts for the GSM8k and GSM1k problems are the same. Five samples are randomly selected from the GSM8k training set, which is also the standard configuration in this field (see Appendix B for complete prompt information). All open source models are evaluated at a temperature of 0 to ensure repeatability. The LM Assessment Kit extracts the last numeric answer in the response and compares it to the correct answer. Therefore, model responses that produce "correct" answers in a format that does not match the sample will be marked as incorrect. For open source models, if the model is compatible with the library, vLLM will be used to accelerate model inference, otherwise the standard HuggingFace library will be used for inference by default. Closed-source models are queried via the LiteLLM library, which unifies the API call format for all evaluated proprietary models. All API model results are from queries between April 16 and April 28, 2024, and use default settings. In terms of the models evaluated, we selected them based on popularity, and also evaluated several lesser-known models that were ranked high on OpenLLMLeaderboard. Interestingly, the researchers found evidence of Goodhart's law in the process: many models performed much worse on GSM1k than GSM8k, indicating that they were mainly catering to GSM8k benchmark, rather than truly improving model inference capabilities. The performance of all models is shown in Appendix D below. In order to make a fair comparison, the researchers divided the models according to their performance on GSM8k, and compared them with other models that performed similarly. The models were compared (Figure 5, Figure 6, Figure 7). What conclusions were drawn? Although the researchers provided objective evaluation results of multiple models, they also stated that interpreting the evaluation results, like interpreting dreams, is often a very subjective task. In the last part of the paper, they elaborate on four implications of the above evaluation in a more subjective way: Conclusion 1: Some model families are systematically overfitting While it is often difficult to draw conclusions from a single data point or model version, examining a family of models and observing patterns of overfitting can make more definitive statements. Some model families, including Phi and Mistral, show a tendency for stronger system performance on GSM8k than on GSM1k in almost every model version and size. There are other model families such as Yi, Xwin, Gemma and CodeLlama that also display this pattern to a lesser extent. Conclusion 2: Other models, especially cutting-edge models, show no signs of overfitting Many models show small overfitting in all performance areas Signs of fit, particularly for all leading or near-leading models including the proprietary Mistral Large, appear to perform similarly on GSM8k and GSM1k. In this regard, the researchers put forward two possible hypotheses: 1) Frontier models have sufficiently advanced reasoning capabilities, so even if the GSM8k problem has already appeared in their training set, they can generalize to new problems; 2) Frontier models Model builders may be more cautious about data contamination. While it’s impossible to look at each model’s training set and determine these assumptions, one piece of evidence supporting the former is that Mistral Large is the only model in the Mistral series that shows no signs of overfitting. The assumption that Mistral only ensures that its largest model is free from data contamination seems unlikely, so researchers favor that a sufficiently powerful LLM will also learn basic inference capabilities during training. If a model learns to reason well enough to solve a problem of a given difficulty, it will be able to generalize to new problems even if GSM8k is present in its training set. Conclusion 3: The overfitting model still has the ability to reason One of the worries of many researchers about model overfitting is that the model cannot perform reasoning, and Just memorize the answers in the training data, but the results of this paper do not support this hypothesis. The fact that a model is overfit does not mean that its inference capabilities are poor, it simply means that it is not as good as the benchmark indicates. In fact, researchers have found that many overfitted models are still capable of reasoning and solving new problems. For example, Phi-3's accuracy dropped by almost 10% between GSM8k and GSM1k, but it still correctly solved more than 68% of GSM1k problems - problems that certainly did not appear in its training distribution. This performance is similar to larger models such as dbrx-instruct, which contain almost 35 times the number of parameters. Likewise, even accounting for overfitting, the Mistral model remains one of the strongest open source models. This provides more evidence for the conclusion of this article that a sufficiently powerful model can learn basic inference even if the benchmark data accidentally leaks into the training distribution, which is likely to happen with most overfit models. Conclusion 4: Data pollution may not be a complete explanation of overfitting A priori, natural hypothesis is that the main cause of overfitting is data Contamination, for example, the test set is leaked during the pre-training or instruction fine-tuning part of creating the model. Previous research has shown that models assign higher log-likelihoods to the data they have seen during training (Carlini et al. [2023]). The researchers tested the hypothesis that data contamination is the cause of overfitting by measuring the probability of the model generating samples from the GSM8k test set and comparing its degree of overfitting compared to GSM8k and GSM1k. Researchers say data pollution may not be the entire cause. They observed this with several outliers. A closer look at these outliers reveals that the model with the lowest log-likelihood per character (Mixtral-8x22b) and the model with the highest log-likelihood per character (Mixtral-8x22b-Instruct) are not just variants of the same model , and has a similar degree of overfitting. More interestingly, the most overfitted model (Math-Shepherd-Mistral-7B-RL (Yu et al. [2023])) has a relatively low log-likelihood per character (Math Shepherd using synthetic data Train reward models on process-level data). Thus, the researchers hypothesized that the reward modeling process may have leaked information about the correct inference chains for GSM8k, even though the issues themselves never appeared in the dataset. Finally, they found that the Llema model had high log-likelihood and minimal overfitting. Since these models are open source and their training data is known, several instances of the GSM8k problem appear in the training corpus, as described in the Llema paper. However, the authors found that these few instances did not lead to severe overfitting. The existence of these outliers suggests that overfitting on GSM8k is not purely due to data contamination, but may be caused by other indirect means, such as the model builder collecting data with similar properties to the baseline as training data, or based on Performance on the benchmark selects the final model checkpoint, even though the model itself may not have seen the GSM8k dataset at any point during training. The opposite is also true: a small amount of data contamination does not necessarily lead to overfitting. For more research details, please refer to the original paper.
The above is the detailed content of Finally, someone investigated the overfitting of small models: two-thirds of them had data pollution, and Microsoft Phi-3 and Mixtral 8x22B were named. For more information, please follow other related articles on the PHP Chinese website!
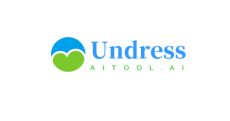
https://undressaitool.ai/ is Powerful mobile app with advanced AI features for adult content. Create AI-generated pornographic images or videos now!
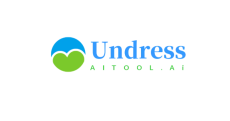
Tutorial on using undressAI to create pornographic pictures/videos: 1. Open the corresponding tool web link; 2. Click the tool button; 3. Upload the required content for production according to the page prompts; 4. Save and enjoy the results.
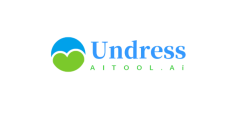
The official address of undress AI is:https://undressaitool.ai/;undressAI is Powerful mobile app with advanced AI features for adult content. Create AI-generated pornographic images or videos now!
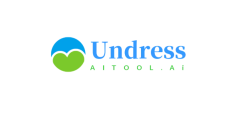
Tutorial on using undressAI to create pornographic pictures/videos: 1. Open the corresponding tool web link; 2. Click the tool button; 3. Upload the required content for production according to the page prompts; 4. Save and enjoy the results.
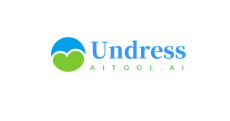
The official address of undress AI is:https://undressaitool.ai/;undressAI is Powerful mobile app with advanced AI features for adult content. Create AI-generated pornographic images or videos now!
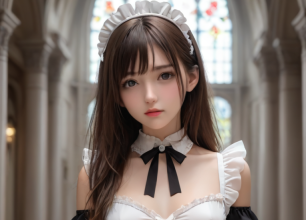
Tutorial on using undressAI to create pornographic pictures/videos: 1. Open the corresponding tool web link; 2. Click the tool button; 3. Upload the required content for production according to the page prompts; 4. Save and enjoy the results.
![[Ghibli-style images with AI] Introducing how to create free images with ChatGPT and copyright](https://img.php.cn/upload/article/001/242/473/174707263295098.jpg?x-oss-process=image/resize,p_40)
The latest model GPT-4o released by OpenAI not only can generate text, but also has image generation functions, which has attracted widespread attention. The most eye-catching feature is the generation of "Ghibli-style illustrations". Simply upload the photo to ChatGPT and give simple instructions to generate a dreamy image like a work in Studio Ghibli. This article will explain in detail the actual operation process, the effect experience, as well as the errors and copyright issues that need to be paid attention to. For details of the latest model "o3" released by OpenAI, please click here⬇️ Detailed explanation of OpenAI o3 (ChatGPT o3): Features, pricing system and o4-mini introduction Please click here for the English version of Ghibli-style article⬇️ Create Ji with ChatGPT
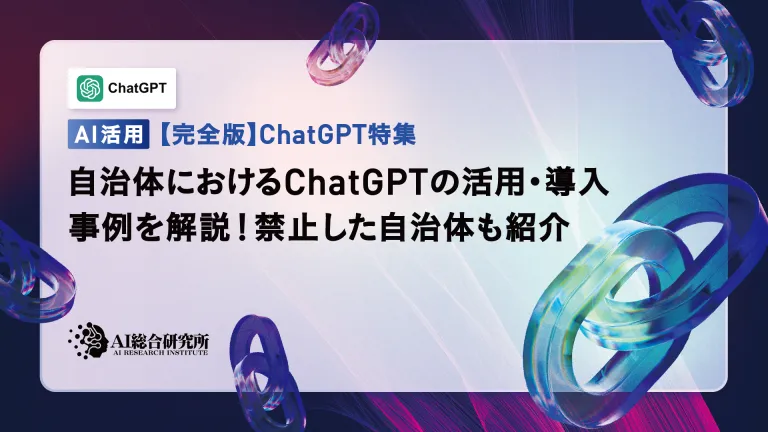
As a new communication method, the use and introduction of ChatGPT in local governments is attracting attention. While this trend is progressing in a wide range of areas, some local governments have declined to use ChatGPT. In this article, we will introduce examples of ChatGPT implementation in local governments. We will explore how we are achieving quality and efficiency improvements in local government services through a variety of reform examples, including supporting document creation and dialogue with citizens. Not only local government officials who aim to reduce staff workload and improve convenience for citizens, but also all interested in advanced use cases.


Hot AI Tools

Undresser.AI Undress
AI-powered app for creating realistic nude photos

AI Clothes Remover
Online AI tool for removing clothes from photos.

Undress AI Tool
Undress images for free

Clothoff.io
AI clothes remover

Video Face Swap
Swap faces in any video effortlessly with our completely free AI face swap tool!

Hot Article

Hot Tools

MantisBT
Mantis is an easy-to-deploy web-based defect tracking tool designed to aid in product defect tracking. It requires PHP, MySQL and a web server. Check out our demo and hosting services.
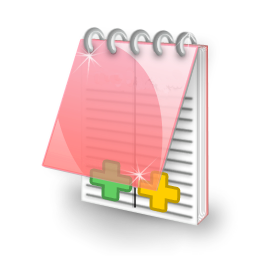
EditPlus Chinese cracked version
Small size, syntax highlighting, does not support code prompt function
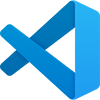
VSCode Windows 64-bit Download
A free and powerful IDE editor launched by Microsoft
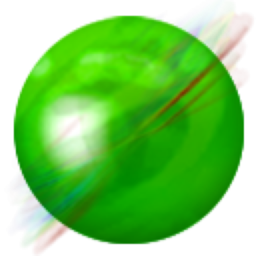
ZendStudio 13.5.1 Mac
Powerful PHP integrated development environment

PhpStorm Mac version
The latest (2018.2.1) professional PHP integrated development tool
