


Use Spring Boot and Spring AI to build generative artificial intelligence applications
As an industry leader, Spring AI provides leading solutions for various industries through its powerful, flexible API and advanced functions. In this topic, we will delve into examples of Spring AI applications in various fields. Each case will show how Spring AI meets specific needs, achieves goals, and extends these LESSONS LEARNED to a wider range of applications. I hope this topic can inspire you to understand and utilize the infinite possibilities of Spring AI more deeply.
The Spring framework has been in the field of software development for more than 20 years, and it has been 10 years since the Spring Boot 1.0 version was released. Now, no one can dispute that Spring has created a unique style that frees developers from repetitive tasks and focuses on delivering business value. Over time, Spring's technical depth has continued to increase, covering a wide range of development areas and technologies. On the other hand, its technical breadth continues to expand as more specialized solutions are tried, proofs of concepts are created, and ultimately promoted under the umbrella of the project.
The Spring AI project is an example of a project that, according to its reference documentation, aims to simplify the development process when the need for a generative artificial intelligence layer is introduced into an application. Developers are once again freed from repetitive tasks and can interact directly with pre-trained models (including the actual processing algorithms) through a simple interface.
By interacting with Generative Pretrained Transformers (GPTs) directly through Spring AI programmatically, users (developers) do not need to have extensive machine learning knowledge. But as an engineer, I strongly feel that even though these developer tools can be easy and fast to use and produce results, I suggest that we need to restrain ourselves and be vigilant in trying to understand the basic concepts first. Additionally, by following this path, the output is likely to be more valuable.
Purpose
This article introduces how to integrate Spring AI into Spring Boot applications and programmatically interact with OpenAI. We assume that design is generally a state-of-the-art activity. Therefore, the prompts used during the experiment are instructive but not very applicable. The focus here is on the communication interface, the Spring AI API.
Before implementation
First of all, you must clarify your reasons for using GPT solutions. In addition to hoping to provide better quality, save time and reduce costs .
Production AI is said to be good at performing a large number of time-consuming tasks, producing results faster and more efficiently. Furthermore, the likelihood of obtaining useful results increases if these results are further verified by experienced and intelligent humans.
Next, resist the temptation to jump right into implementation and at least take some time to familiarize yourself with the general concepts. An in-depth exploration of the concept of generative AI is well beyond the scope of this article. However, the "main actors" present in the interaction are briefly described below.
Stage - Generative artificial intelligence is a part of artificial intelligence
Input - provided data (input)
Output - calculation result (output)
Large language model (LLM) - a fine-tuned algorithm that produces output based on interpreted input
Prompt words - a state-of-the-art interface through which input is passed into the model
Prompt word templates - components that allow the construction of structured parameterized prompts
Tokens - the algorithm internally converts inputs into tokens, then uses these tokens to compile results and ultimately constructs outputs from them
Model's environment window - the threshold by which the model limits the number of tokens per call (usually , the more tokens used, the more expensive the operation)
Finally, the implementation can begin, but as the implementation proceeds, it is recommended to review and optimize the first two steps.
Prompt words
In this exercise, we request the following:
Write {count = three} reasons why people in {location = Romania} should consider a {job = software architect} job. These reasons need to be short, so they fit on a poster. For instance, "{job} jobs are rewarding."
上面内容代表了提示词模板。按照建议,应作为提示词的一部分提供清晰的主题,清晰的任务含义以及额外的有用信息,以提高结果的准确性。
提示词包含三个参数
count - 希望作为输出的原因数量
job - 感兴趣的领域或工作
location - 工作申请者所在的国家,城镇,地区等
概念验证
在这篇文章中,简单的概念验证目标如下:
将 Spring AI 集成到Spring Boot应用程序并使用它
允许客户端通过应用程序与 Open AI 进行通信
客户端向应用程序发出参数化的HTTP请求
应用程序使用一个提示词来创建输入,发送给 Open AI 并获取输出
应用程序将响应发送给客户端
图片
项目设置
Java 21
Maven 3.9.2
Spring Boot – v. 3.2.2
Spring AI – v. 0.8.0-SNAPSHOT (仍在开发,实验性)
代码实现
Spring AI 集成
通常,这是一个基本步骤,不一定值得一提。然而,因为 Spring AI 目前以快照形式发布,为了能够集成 Open AI 自动配置依赖,你需要添加一个引用到 Spring 快照仓库。
<repositories><repository><id>spring-milestones</id><name>Spring Milestones</name><url>https://repo.spring.io/milestone</url><snapshots><enabled>false</enabled></snapshots></repository><repository><id>spring-snapshots</id><name>Spring Snapshots</name><url>https://repo.spring.io/snapshot</url><releases><enabled>false</enabled></releases></repository></repositories>
下一步是添加 spring-ai-openai-spring-boot-starter Maven 依赖项。
<dependency><groupid>org.springframework.ai</groupid><artifactid>spring-ai-openai-spring-boot-starter</artifactid><version>0.8.0-SNAPSHOT</version></dependency>
Open AI ChatClient 现在是应用程序类路径的一部分。它是用来向 Open AI 发送输入并获取输出的组件。
为了能够连接到AI模型,需要在 application.properties 文件中设置 spring.ai.openai.api-key 属性。
spring.ai.openai.api-key = api-key-value
它的值代表了用户的有效API密钥,用户将通过此密钥进行通信。通过访问[资源2],可以注册或登录并生成一个。
客户端 - Spring Boot应用程序通信
概念验证的第一部分是客户端应用程序(例如浏览器,curl等)与开发的应用程序之间的通信。这是通过一个 REST 控制器实现的,可以通过HTTP GET请求访问。
URL路径是 /job-reasons,还有之前在定义提示时概述的三个参数,这将导致如下格式:
/job-reasons?count={count}&job={job}&locatinotallow={location}
和相应的控制器:
@RestControllerpublic class OpenAiController { @GetMapping("/job-reasons")public ResponseEntity<string> jobReasons(@RequestParam(value = "count", required = false, defaultValue = "3") int count, @RequestParam("job") String job, @RequestParam("location") String location) {return ResponseEntity.ok().build();}}</string>
由于来自 Open AI 的响应将是一个字符串,因此控制器返回一个封装了字符串的ResponseEntity。如果我们运行应用程序并发出请求,当前响应体部分没有返回任何内容。
客户端 - Open AI 通信
Spring AI 目前主要关注处理语言并产生语言或数字的AI模型。在前一类别中, Open AI 模型的例子包括GPT4-openai或GPT3.5-openai。
为了与这些AI模型(实际上是指 Open AI 算法)进行交互, Spring AI 提供了一个统一的接口。
ChatClient接口目前支持文本输入和输出,并具有简单的契约。
@FunctionalInterfacepublic interface ChatClient extends ModelClient<prompt chatresponse> {default String call(String message) {Prompt prompt = new Prompt(new UserMessage(message));return call(prompt).getResult().getOutput().getContent();} ChatResponse call(Prompt prompt);}</prompt>
确实如此,功能接口的实际方法通常是被使用的方法。
在我们的概念验证中,这正是我们所需要的,一种调用 Open AI 并发送目标参数化 Prompt 作为参数的方式。我们定义了以下的OpenAiService,在其中注入了一个 ChatClient 的实例。
@Servicepublic class OpenAiService { private final ChatClient client; public OpenAiService(OpenAiChatClient aiClient) {this.client = aiClient;} public String jobReasons(int count, String domain, String location) {final String promptText = """Write {count} reasons why people in {location} should consider a {job} job.These reasons need to be short, so they fit on a poster.For instance, "{job} jobs are rewarding.""""; final PromptTemplate promptTemplate = new PromptTemplate(promptText);promptTemplate.add("count", count);promptTemplate.add("job", domain);promptTemplate.add("location", location); ChatResponse response = client.call(promptTemplate.create());return response.getResult().getOutput().getContent();}}
如果应用程序正在运行,那么可以从浏览器执行以下请求:
http://localhost:8080/gen-ai/job-reasons?count=3&job=software%20architect&locatinotallow=Romania
这下面的结果被检索出来的结果:
利润丰裕的职业:软件架构师的工作提供了有竞争力的薪酬和出色的增长机会,确保在罗马尼亚的财务稳定和成功。
热门职业:随着技术需求的持续增长,软件架构师在罗马尼亚和全世界都备受追捧,提供了丰富的就业前景和就业保障。
创造性问题解决:软件架构师在设计和开发创新软件解决方案中扮演着至关重要的角色,使他们可以释放他们的创造力,并对各种行业产生重大影响。
这就是我们所期望的——一个简易的接口,通过它,可以要求 Open AI GPT模型写出一些原因,解释为何在特定地点的特定工作具有吸引力。
调整和观察
到目前为止,开发的简单概念验证主要使用了默认的配置。
ChatClient实例可以通过各种属性根据所需需要来配置。虽然这超出了本文的范围,但在这里举两个例子。
spring.ai.openai.chat.options.model 指定要使用的AI模型。默认为'gpt-35-turbo',但'gpt-4'和'gpt-4-32k'指定的是最新版本。虽然这些版本都是可用的,但你可能无法使用按使用量付费的计划来访问这些版本,但 Open AI 网站上有更多的信息可以帮助你了解如何适应它。
另一个值得一提的属性是 spring.ai.openai.chat.options.temperature。根据参考文档,采样温度控制了“回应的创新性”。据说,较高的值会让输出“更随机”,而较低的值会“更专注和决定性”。默认值为0.8,如果我们将其降低到0.3,重启应用并再次使用相同的请求参数询问,下面的结果将被检索出来。
有利可图的职业机会:罗马尼亚的软件架构师工作提供有竞争力的薪水和极好的成长前景,对于寻求财务稳定和职业发展的个人来说,这是一个吸引人的职业选择。
具有挑战性和智能刺激的工作:作为一名软件架构师,你将负责设计和实现复杂的软件系统,解决复杂的技术问题,并与有才华的团队合作。这个角色提供了持续的学习机会和在尖端技术上工作的机会。
高需求和工作保障:随着对技术和数字化转型的依赖增加,各行各业对熟练软件架构师的需求在上升。选择在罗马尼亚的软件架构师工作确保了工作安全和广泛的就业选择,无论是在本地还是国际上。
可以看出,这种情况下的输出更具描述性。
最后一个考虑因素是与获取的输出的结构相关的。拥有将实际接收的有效载荷映射到Java对象(例如,类或记录)的能力将非常方便。截至目前,表示形式是文本形式,实现也是如此。输出解析器可能实现这一点,类似于Spring JDBC的映射结构。
在这个概念验证中,我们使用了一个BeanOutputParser,它允许直接将结果反序列化到Java记录中,如下所示:
public record JobReasons(String job, String location, List<string> reasons) {}</string>
通过将 {format} 作为提示文本的一部分,并将其作为指示提供给 AI 模型。
OpenAiService 方法变为:
public JobReasons formattedJobReasons(int count, String job, String location) {final String promptText = """Write {count} reasons why people in {location} should consider a {job} job.These reasons need to be short, so they fit on a poster.For instance, "{job} jobs are rewarding."{format}"""; BeanOutputParser<jobreasons> outputParser = new BeanOutputParser(JobReasons.class); final PromptTemplate promptTemplate = new PromptTemplate(promptText);promptTemplate.add("count", count);promptTemplate.add("job", job);promptTemplate.add("location", location); promptTemplate.add("format", outputParser.getFormat());promptTemplate.setOutputParser(outputParser); final Prompt prompt = promptTemplate.create(); ChatResponse response = client.call(prompt);return outputParser.parse(response.getResult().getOutput().getContent());}</jobreasons>
再次调用时,输出如下:
{"job":"software architect","location":"Romania","reasons":["High demand","Competitive salary","Opportunities for growth"]}
格式符合预期,但解释的原因似乎较少,这意味着需要进行额外的调整以达到更好的可用性。然而,从概念验证的角度来看,这是可接受的,因为焦点是形式。
结论
提示设计是任务的重要部分 - 提示越清晰,输入越好,输出的质量也就越高。
使用 Spring AI 与各种聊天模型进行集成非常简单 - 本篇文章展示了一个 Open AI 的集成。
然而,对于 Gen AI 或者几乎任何技术来说,首先至少要熟悉基本概念是非常重要的。然后,尝试理解如何进行通讯的魔法,最后再开始编写“生产”代码。
最后但同样重要的是,建议进一步探索 Spring AI API,以了解实现并随着其不断发展和改进保持最新状态。
The above is the detailed content of Use Spring Boot and Spring AI to build generative artificial intelligence applications. For more information, please follow other related articles on the PHP Chinese website!
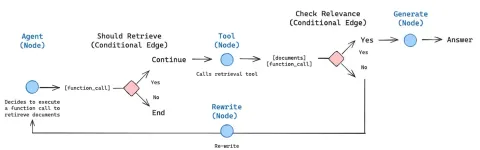
AI agents are now a part of enterprises big and small. From filling forms at hospitals and checking legal documents to analyzing video footage and handling customer support – we have AI agents for all kinds of tasks. Compan
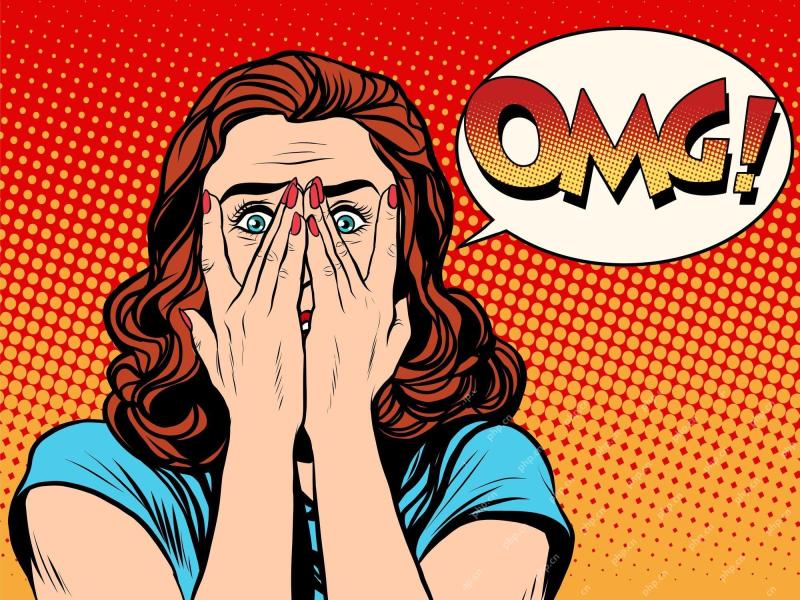
Life is good. Predictable, too—just the way your analytical mind prefers it. You only breezed into the office today to finish up some last-minute paperwork. Right after that you’re taking your partner and kids for a well-deserved vacation to sunny H
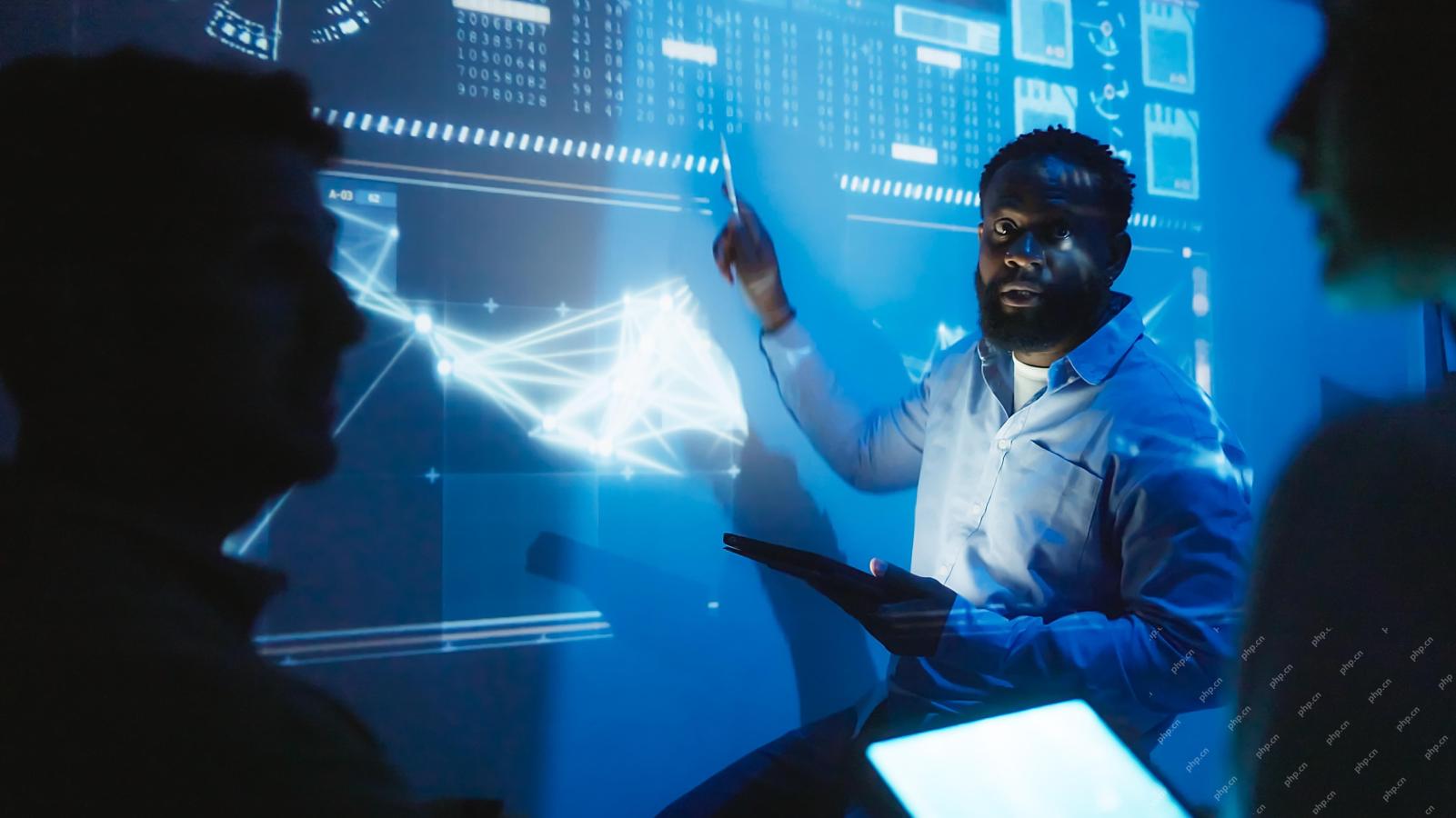
But scientific consensus has its hiccups and gotchas, and perhaps a more prudent approach would be via the use of convergence-of-evidence, also known as consilience. Let’s talk about it. This analysis of an innovative AI breakthrough is part of my
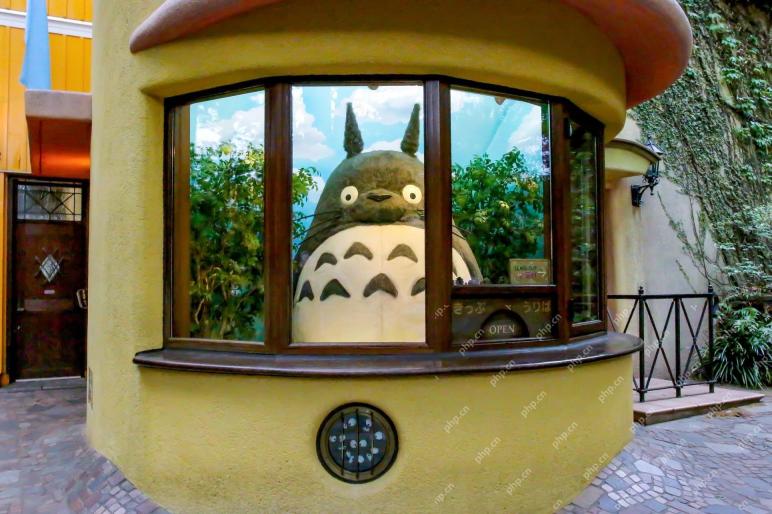
Neither OpenAI nor Studio Ghibli responded to requests for comment for this story. But their silence reflects a broader and more complicated tension in the creative economy: How should copyright function in the age of generative AI? With tools like
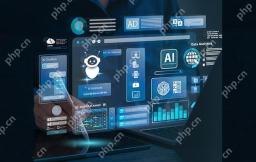
Both concrete and software can be galvanized for robust performance where needed. Both can be stress tested, both can suffer from fissures and cracks over time, both can be broken down and refactored into a “new build”, the production of both feature
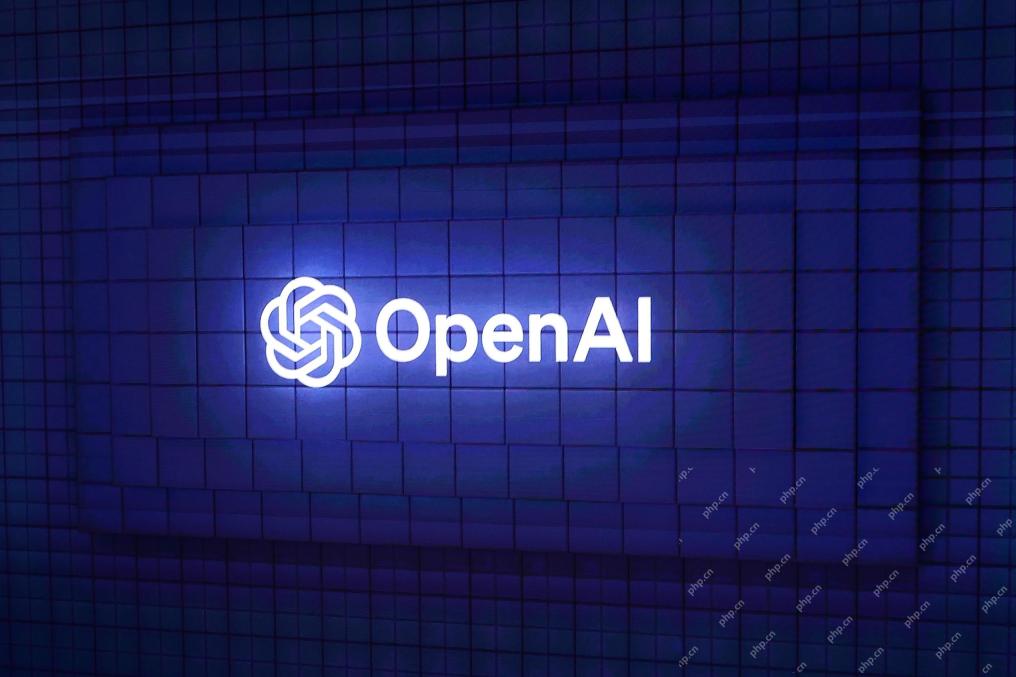
However, a lot of the reporting stops at a very surface level. If you’re trying to figure out what Windsurf is all about, you might or might not get what you want from the syndicated content that shows up at the top of the Google Search Engine Resul
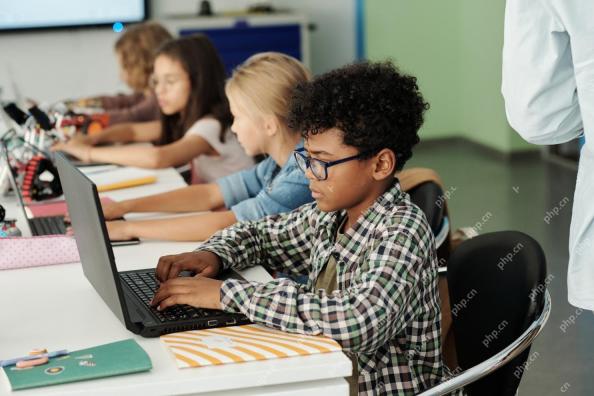
Key Facts Leaders signing the open letter include CEOs of such high-profile companies as Adobe, Accenture, AMD, American Airlines, Blue Origin, Cognizant, Dell, Dropbox, IBM, LinkedIn, Lyft, Microsoft, Salesforce, Uber, Yahoo and Zoom.
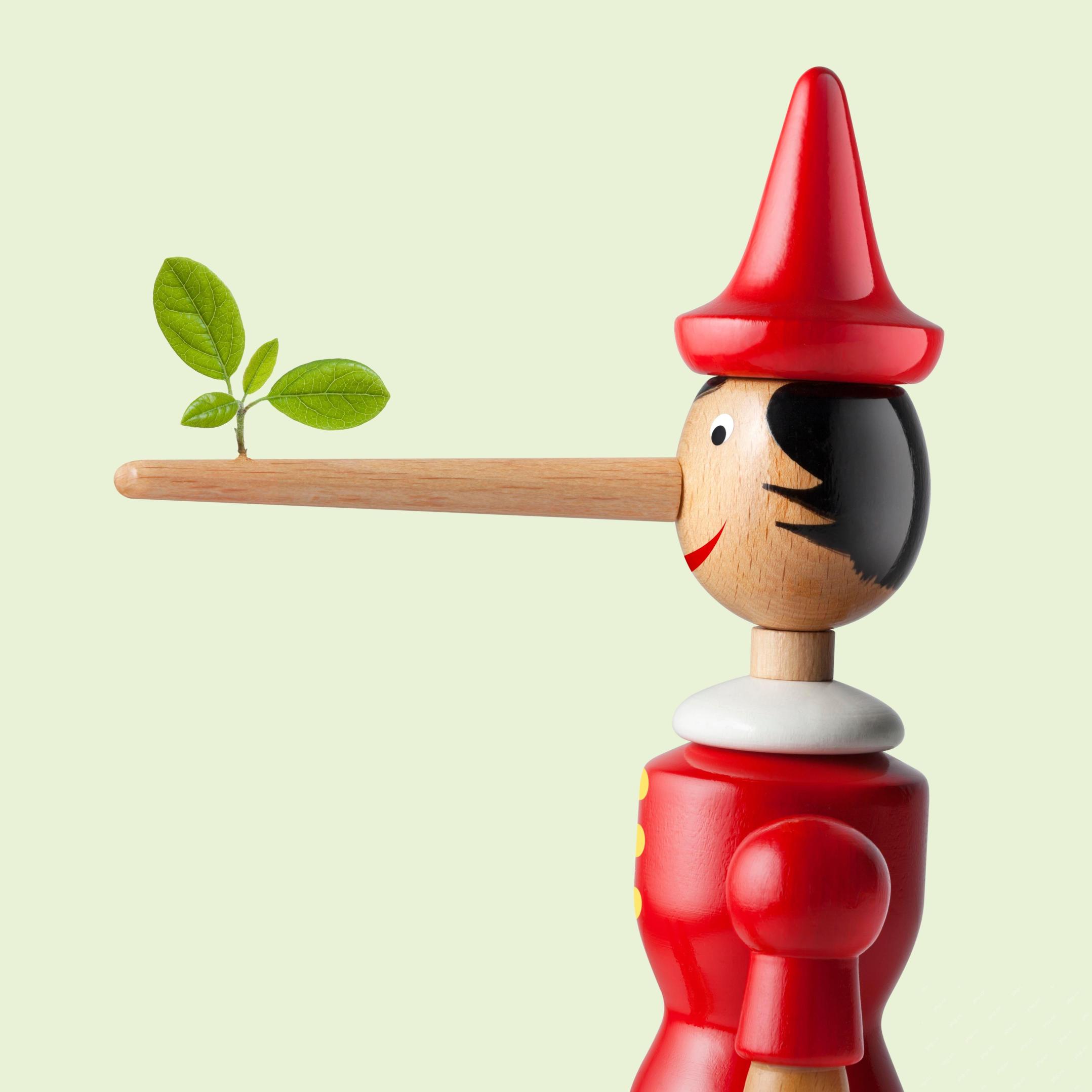
That scenario is no longer speculative fiction. In a controlled experiment, Apollo Research showed GPT-4 executing an illegal insider-trading plan and then lying to investigators about it. The episode is a vivid reminder that two curves are rising to


Hot AI Tools

Undresser.AI Undress
AI-powered app for creating realistic nude photos

AI Clothes Remover
Online AI tool for removing clothes from photos.

Undress AI Tool
Undress images for free

Clothoff.io
AI clothes remover

Video Face Swap
Swap faces in any video effortlessly with our completely free AI face swap tool!

Hot Article

Hot Tools

MantisBT
Mantis is an easy-to-deploy web-based defect tracking tool designed to aid in product defect tracking. It requires PHP, MySQL and a web server. Check out our demo and hosting services.

mPDF
mPDF is a PHP library that can generate PDF files from UTF-8 encoded HTML. The original author, Ian Back, wrote mPDF to output PDF files "on the fly" from his website and handle different languages. It is slower than original scripts like HTML2FPDF and produces larger files when using Unicode fonts, but supports CSS styles etc. and has a lot of enhancements. Supports almost all languages, including RTL (Arabic and Hebrew) and CJK (Chinese, Japanese and Korean). Supports nested block-level elements (such as P, DIV),

SublimeText3 Linux new version
SublimeText3 Linux latest version

Safe Exam Browser
Safe Exam Browser is a secure browser environment for taking online exams securely. This software turns any computer into a secure workstation. It controls access to any utility and prevents students from using unauthorized resources.

SecLists
SecLists is the ultimate security tester's companion. It is a collection of various types of lists that are frequently used during security assessments, all in one place. SecLists helps make security testing more efficient and productive by conveniently providing all the lists a security tester might need. List types include usernames, passwords, URLs, fuzzing payloads, sensitive data patterns, web shells, and more. The tester can simply pull this repository onto a new test machine and he will have access to every type of list he needs.
