In the field of technological innovation, artificial intelligence (AI) is one of the most transformative and promising developments of our time. Artificial intelligence has revolutionized many industries, from healthcare and finance to transportation and entertainment, with its ability to analyze large amounts of data, learn from patterns, and make intelligent decisions. However, despite its remarkable progress, AI also faces significant limitations and challenges that prevent it from reaching its full potential. In this article, we will delve into the top ten limitations of artificial intelligence, revealing the limitations faced by developers, researchers, and practitioners in this field. By understanding these challenges, it is possible to navigate the complexities of AI development, reduce risks, and pave the way for responsible and ethical advancement of AI technology.
Limited data availability:
The development of artificial intelligence depends on the adequacy of data. One of the basic requirements for training artificial intelligence models is access to large and diverse data sets. However, in many cases, relevant data may be scarce, incomplete, or biased, hindering the performance and generalization capabilities of AI systems.
Data bias and quality issues:
Artificial intelligence algorithms are susceptible to biases and inaccuracies present in training data, leading to biased results and flawed decision-making processes. Historical data, social stereotypes, or human annotation errors can create biases that lead to unfair or discriminatory outcomes, especially in sensitive applications such as healthcare, criminal justice, and finance. Addressing data bias and ensuring data quality are ongoing challenges in AI development.
Lack of explainability:
"Black box" is a term commonly used to refer to most artificial intelligence models, especially deep learning models. Because its decision-making process is inherently complex and arcane. The key to winning the trust and recognition of users and stakeholders is understanding how AI models make predictions or provide recommendations.
Overfitting and generalization:
An artificial intelligence model trained on a specific data set can easily break away from actual scenarios or unseen data examples, a practice called overfitting. combine. The consequences of this phenomenon include poor performance, unreliable predictions, and the failure of practical AI systems to function properly.
Computing resources and scalability:
Training artificial intelligence models requires a lot of computing, including GPUs, CPUs, and TPUs, while deployment requires large distributed resource pools.
Ethical and Social Impact:
The use of artificial intelligence technology raises ethical principles and social issues such as privacy, security, fairness (or justice), and the concept of accountability or transparency. The problem is that these technologies could lead to biased unemployment policies evolving into autonomous robots with advanced weapons systems, in addition to state-monitoring methods, creating significant difficulties for regulators, policymakers, and communities at large.
Lack of domain expertise and background understanding:
Artificial intelligence systems cannot perform efficiently in areas that require specialized domain knowledge or background understanding. Understanding the nuances, subtleties, and context-specific information is challenging for AI algorithms, especially in dynamic and complex environments.
Security Vulnerabilities and Adversarial Attacks:
AI systems are vulnerable to a variety of security threats and adversarial attacks, in which malicious actors manipulate input or exploit vulnerabilities to trick or corrupt AI models . Adversarial attacks can lead to incorrect navigation predictions, system failures, or privacy leaks, thereby undermining the trust and reliability of AI systems.
Continuous learning and adaptation:
Artificial intelligence systems often need to continuously learn and adapt to remain effective in dynamic and changing environments. However, updating and retraining AI models with new data or changing environments can be challenging and resource-intensive.
Regulatory and Legal Compliance:
Artificial Intelligence technologies are subject to various regulatory frameworks, legal requirements and industry standards governing their development, deployment and use. Compliance with regulations such as GDPR, HIPAA and CCPA, as well as industry-specific standards and guidelines, is critical to ensuring responsible and ethical use of AI.
In short, although artificial intelligence holds great promise in advancing technology and solving complex problems, it is not without limitations and challenges. From data availability and bias to explainability and security, addressing the top ten limitations of AI is critical to realizing its full potential while mitigating potential risks and ensuring responsible development and deployment.
The above is the detailed content of Ten limitations of artificial intelligence. For more information, please follow other related articles on the PHP Chinese website!
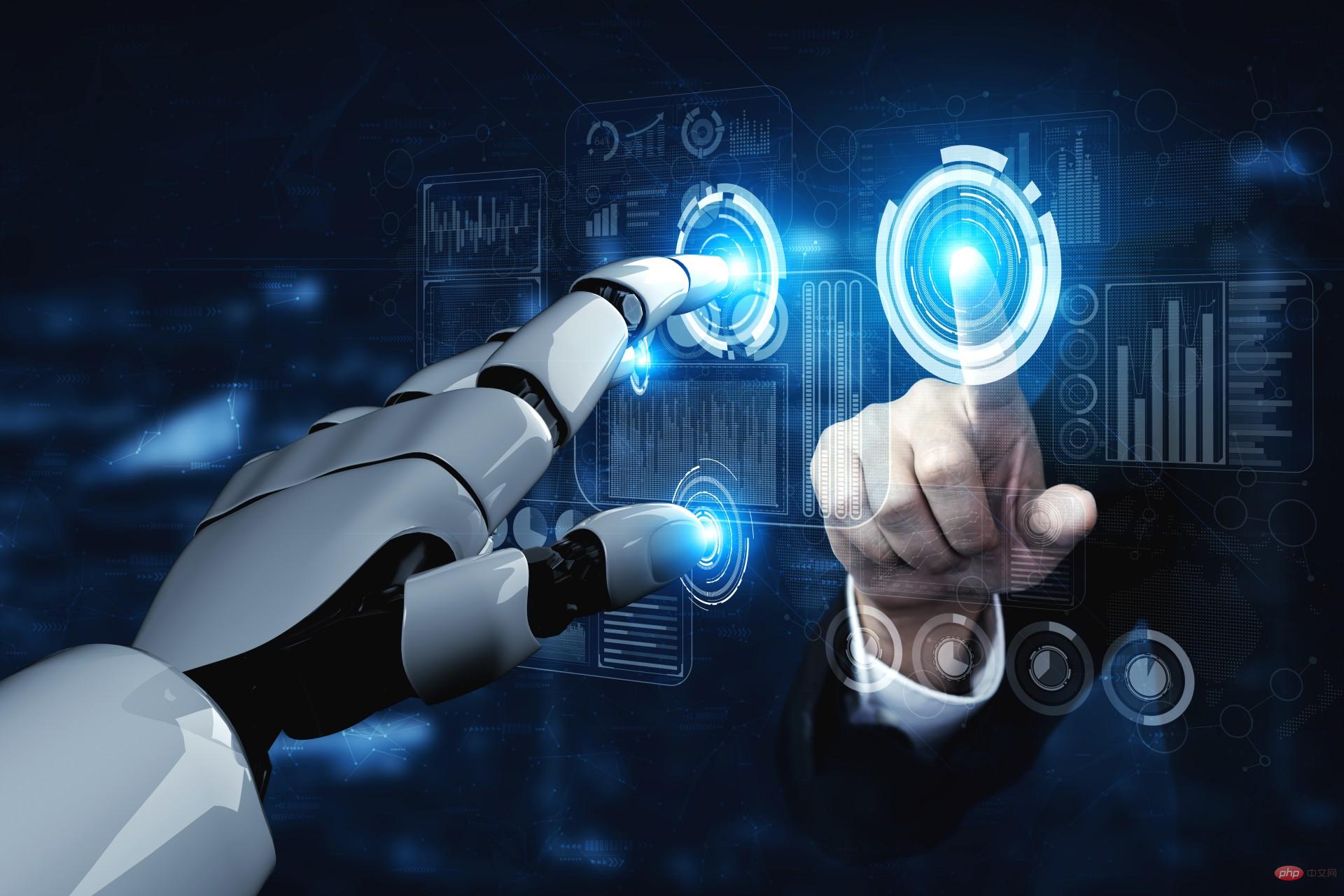
机器学习是一个不断发展的学科,一直在创造新的想法和技术。本文罗列了2023年机器学习的十大概念和技术。 本文罗列了2023年机器学习的十大概念和技术。2023年机器学习的十大概念和技术是一个教计算机从数据中学习的过程,无需明确的编程。机器学习是一个不断发展的学科,一直在创造新的想法和技术。为了保持领先,数据科学家应该关注其中一些网站,以跟上最新的发展。这将有助于了解机器学习中的技术如何在实践中使用,并为自己的业务或工作领域中的可能应用提供想法。2023年机器学习的十大概念和技术:1. 深度神经网

本文将详细介绍用来提高机器学习效果的最常见的超参数优化方法。 译者 | 朱先忠审校 | 孙淑娟简介通常,在尝试改进机器学习模型时,人们首先想到的解决方案是添加更多的训练数据。额外的数据通常是有帮助(在某些情况下除外)的,但生成高质量的数据可能非常昂贵。通过使用现有数据获得最佳模型性能,超参数优化可以节省我们的时间和资源。顾名思义,超参数优化是为机器学习模型确定最佳超参数组合以满足优化函数(即,给定研究中的数据集,最大化模型的性能)的过程。换句话说,每个模型都会提供多个有关选项的调整“按钮
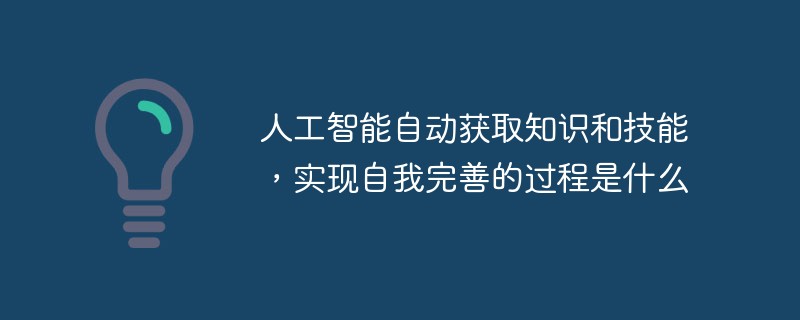
实现自我完善的过程是“机器学习”。机器学习是人工智能核心,是使计算机具有智能的根本途径;它使计算机能模拟人的学习行为,自动地通过学习来获取知识和技能,不断改善性能,实现自我完善。机器学习主要研究三方面问题:1、学习机理,人类获取知识、技能和抽象概念的天赋能力;2、学习方法,对生物学习机理进行简化的基础上,用计算的方法进行再现;3、学习系统,能够在一定程度上实现机器学习的系统。
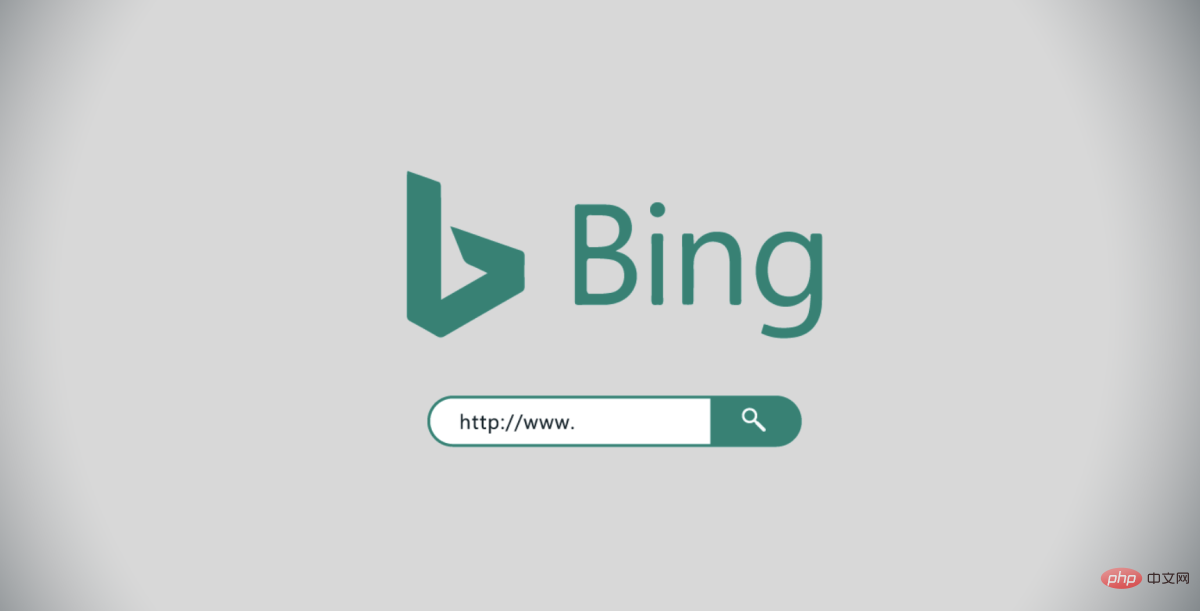
截至3月20日的数据显示,自微软2月7日推出其人工智能版本以来,必应搜索引擎的页面访问量增加了15.8%,而Alphabet旗下的谷歌搜索引擎则下降了近1%。 3月23日消息,外媒报道称,分析公司Similarweb的数据显示,在整合了OpenAI的技术后,微软旗下的必应在页面访问量方面实现了更多的增长。截至3月20日的数据显示,自微软2月7日推出其人工智能版本以来,必应搜索引擎的页面访问量增加了15.8%,而Alphabet旗下的谷歌搜索引擎则下降了近1%。这些数据是微软在与谷歌争夺生
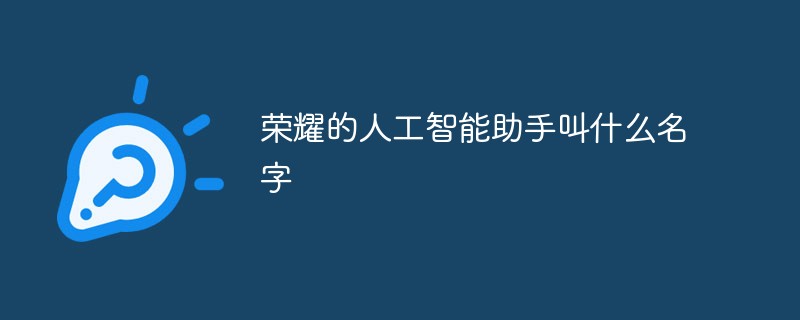
荣耀的人工智能助手叫“YOYO”,也即悠悠;YOYO除了能够实现语音操控等基本功能之外,还拥有智慧视觉、智慧识屏、情景智能、智慧搜索等功能,可以在系统设置页面中的智慧助手里进行相关的设置。
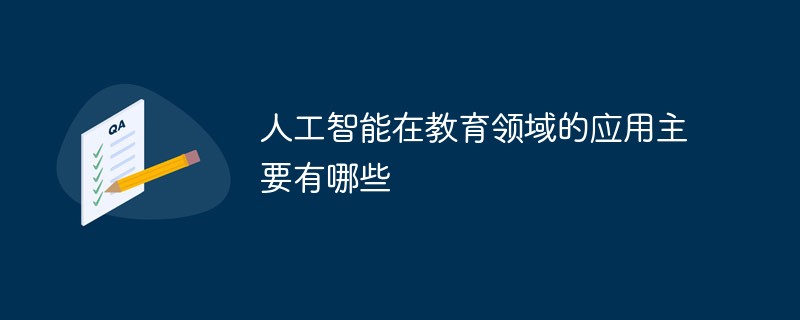
人工智能在教育领域的应用主要有个性化学习、虚拟导师、教育机器人和场景式教育。人工智能在教育领域的应用目前还处于早期探索阶段,但是潜力却是巨大的。
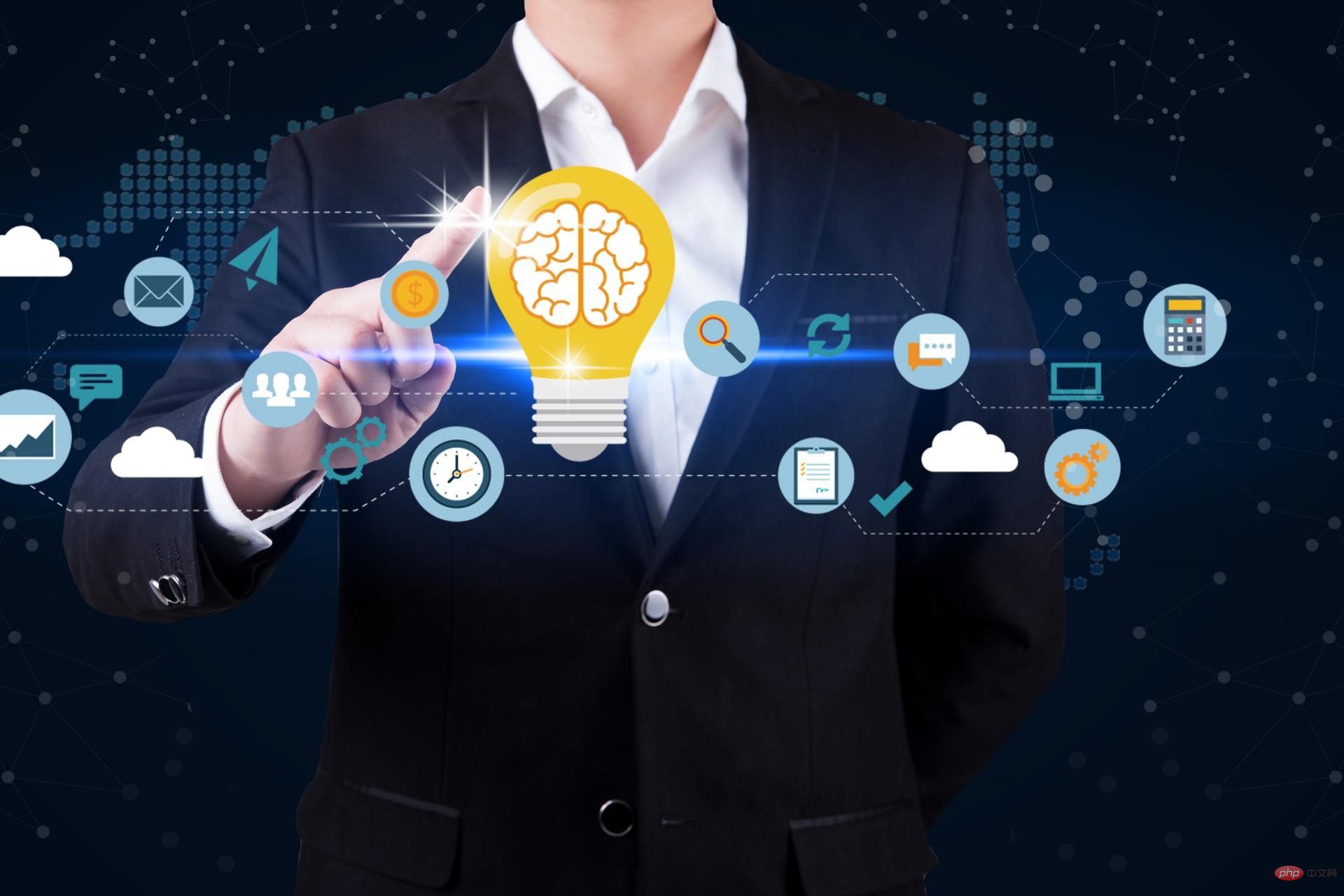
阅读论文可以说是我们的日常工作之一,论文的数量太多,我们如何快速阅读归纳呢?自从ChatGPT出现以后,有很多阅读论文的服务可以使用。其实使用ChatGPT API非常简单,我们只用30行python代码就可以在本地搭建一个自己的应用。 阅读论文可以说是我们的日常工作之一,论文的数量太多,我们如何快速阅读归纳呢?自从ChatGPT出现以后,有很多阅读论文的服务可以使用。其实使用ChatGPT API非常简单,我们只用30行python代码就可以在本地搭建一个自己的应用。使用 Python 和 C
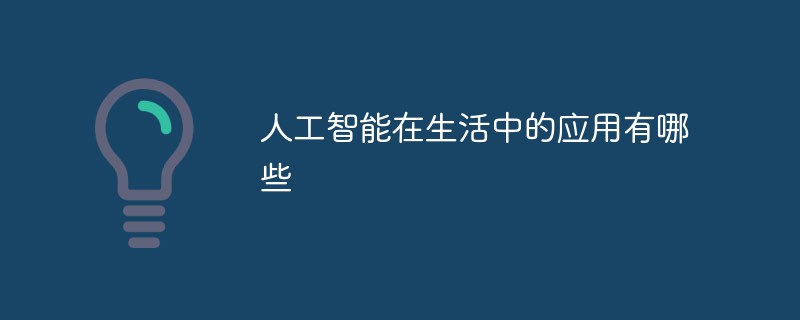
人工智能在生活中的应用有:1、虚拟个人助理,使用者可通过声控、文字输入的方式,来完成一些日常生活的小事;2、语音评测,利用云计算技术,将自动口语评测服务放在云端,并开放API接口供客户远程使用;3、无人汽车,主要依靠车内的以计算机系统为主的智能驾驶仪来实现无人驾驶的目标;4、天气预测,通过手机GPRS系统,定位到用户所处的位置,在利用算法,对覆盖全国的雷达图进行数据分析并预测。


Hot AI Tools

Undresser.AI Undress
AI-powered app for creating realistic nude photos

AI Clothes Remover
Online AI tool for removing clothes from photos.

Undress AI Tool
Undress images for free

Clothoff.io
AI clothes remover

AI Hentai Generator
Generate AI Hentai for free.

Hot Article

Hot Tools
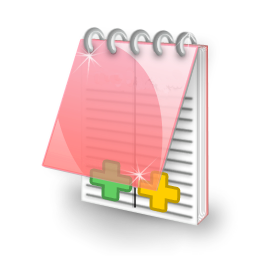
EditPlus Chinese cracked version
Small size, syntax highlighting, does not support code prompt function

SublimeText3 Linux new version
SublimeText3 Linux latest version
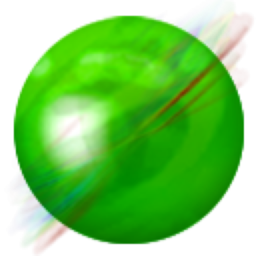
ZendStudio 13.5.1 Mac
Powerful PHP integrated development environment

Notepad++7.3.1
Easy-to-use and free code editor

SublimeText3 English version
Recommended: Win version, supports code prompts!
