


Recently, led by Professor Yan Shuicheng, the Kunlun Wanwei 2050 Global Research Institute, National University of Singapore, and Nanyang Technological University of Singapore teams jointly released and open sourced itVitron universal pixel-level visual multi-modal large language model.
This is a heavy-duty general visual multi-modal model that supports a series of visual tasks from visual understanding to visual generation, from low level to high level, solving problems. The image / long-standing problem in the large language model industry provides a pixel-level pixel-level solution that comprehensively unifies the understanding, generation, segmentation, and editing of static images and dynamic video content. The general vision multi-modal large model lays the foundation for the ultimate form of the next generation general vision large model, and also marks the step towards general artificial intelligence(#AGI)Another big step.
Vitron, as a unified pixel-level visual multi-modal large language model, achieves comprehensive support for visual tasks from low-level to high-level ,Able to handle complex visual tasks, andunderstand and generate image and video content, providing powerful visual understanding and task execution capabilities. At the same time, Vitron supports continuous operations with users, enabling flexible human-computer interaction, demonstrating the great potential towards a more unified visual multi-modal universal model.
Vitron-related papers, codes and Demo have all been made public. They are comprehensive, technological innovation, The unique advantages and potential demonstrated in human-computer interaction and application potential not only promote the development of multi-modal large models, but also provide a new direction for future visual large model research.
Kunlun Wanwei2050 Global Research Institute has always been committed to building a excellent company for the future world Scientific research institutions, together with the scientific communitycross" singularity", Explore the unknown world,create a better future. Previously, Kunlun Wanwei2050 Global Research Institute has released and open sourced the digital agent research and development toolkitAgentStudio, In the future, the institute will continue to promote artificial intelligencetechnical breakthroughs, and contribute to China'sartificial intelligence ecological constructionContribute. The current development of visual large language models (LLMs) has made gratifying progress. The community increasingly believes that building more general and powerful multimodal large models (MLLMs) will be the only way to achieve general artificial intelligence (AGI). However, there are still some key challenges in the process of moving towards a multi-modal general model (Generalist). For example, a large part of the work does not achieve fine-grained pixel-level visual understanding, or lacks unified support for images and videos. Or the support for various visual tasks is insufficient, and it is far from a universal large model.
In order to fill this gap, recently, the Kunlun Worldwide 2050 Global Research Institute, the National University of Singapore, and the Nanyang Technological University of Singapore team jointly released the open source Vitron universal pixel-level visual multi-modal large language model. Vitron supports a series of visual tasks from visual understanding to visual generation, from low level to high level, including comprehensive understanding, generation, segmentation and editing of static images and dynamic video content.
Vitron comprehensively describes the functional support for four major vision-related tasks. and its key advantages. Vitron also supports continuous operation with users to achieve flexible human-machine interaction. This project demonstrates the great potential for a more unified vision multi-modal general model, laying the foundation for the ultimate form of the next generation of general vision large models.Vitron related papers, codes, and demos are now all public.
- Paper link: https://is.gd/aGu0VV
- Open source code: https://github.com/SkyworkAI/Vitron
0
. The ultimate unified multi-modal language model In recent years, large language models (LLMs) have demonstrated unprecedented powerful capabilities, and they have been gradually verified as the technical route to AGI. Multimodal large language models (MLLMs) are developing rapidly in many communities and are rapidly emerging. By introducing modules that can perform visual perception, pure language-based LLMs are extended to MLLMs. Many MLLMs that are powerful and excellent in image understanding have been developed. , such as BLIP-2, LLaVA, MiniGPT-4, etc. At the same time, MLLMs focusing on video understanding have also been launched, such as VideoChat, Video-LLaMA, Video-LLaVA, etc. Subsequently, researchers mainly tried to further expand the capabilities of MLLMs from two dimensions. On the one hand, researchers are trying to deepen MLLMs' understanding of vision, transitioning from rough instance-level understanding to pixel-level fine-grained understanding of images, so as to achieve visual region positioning (Regional Grounding) capabilities, such as GLaMM, PixelLM, NExT-Chat and MiniGPT-v2 etc. On the other hand, researchers try to expand the visual functions that MLLMs can support. Some research has begun to study how MLLMs not only understand input visual signals, but also support the generation of output visual content. For example, MLLMs such as GILL and Emu can flexibly generate image content, and GPT4Video and NExT-GPT realize video generation. At present, the artificial intelligence community has gradually reached a consensus that the future trend of visual MLLMs will inevitably develop in the direction of highly unified and stronger capabilities. However, despite the numerous MLLMs developed by the community, a clear gap still exists. The above table simply summarizes the capabilities of existing visual MLLM (only representatively includes some models, and the coverage is incomplete). To bridge these gaps, the team proposes Vitron, a general pixel-level visual MLLM. 02. Vitron system architecture: three key modules Vitron overall framework As shown below. Vitron adopts a similar architecture to existing related MLLMs, including three key parts: 1) front-end visual & language encoding module, 2) central LLM understanding and text generation module, and 3) back-end user response and module calls for visual control module. 03. VitronThree stages of model training Based on the above architecture, Vitron is trained and fine-tuned to give it powerful visual understanding and task execution capabilities. Model training mainly includes three different stages. 1) User response output , directly reply to the user's input. 2) Module name, indicating the function or task to be performed. 3) Call the command to trigger the meta-instruction of the task module. 4) Region (optional output) that specifies fine-grained visual features required for certain tasks, such as in video tracking or visual editing, where backend modules require this information. For regions, based on LLM's pixel-level understanding, a bounding box described by coordinates will be output. 04. Evaluation Experiment Researchers conducted extensive experimental evaluations on 22 common benchmark data sets and 12 image/video vision tasks based on Vitron. Vitron demonstrates strong capabilities in four major visual task groups (segmentation, understanding, content generation and editing), while at the same time it has flexible human-computer interaction capabilities. The following representatively shows some qualitative comparison results: Results of image referring image segmentation Results of image referring expression comprehension. Results on video QA. Image editing results For more detailed experimental content and details, please move here step thesis. 05. Future Directions Overall, this work demonstrates The huge potential of developing a unified visual multi-modal general large model has laid a new form for the research of the next generation of visual large models and taken the first step in this direction. Although the Vitron system proposed by the team shows strong general capabilities, it still has its own limitations. The following researchers list some directions that could be further explored in the future. The Vitron system still uses a semi-joint, semi-agent approach to call external tools. Although this call-based method facilitates the expansion and replacement of potential modules, it also means that the back-end modules of this pipeline structure do not participate in the joint learning of the front-end and LLM core modules. This limitation is not conducive to the overall learning of the system, which means that the performance upper limit of different vision tasks will be limited by the back-end modules. Future work should integrate various vision task modules into a unified unit. Achieving unified understanding and output of images and videos while supporting generation and editing capabilities through a single generative paradigm remains a challenge. Currently, a promising approach is to combine modularity-persistent tokenization to improve the unification of the system on different inputs and outputs and various tasks. Unlike previous models that focused on a single vision task (e.g., Stable Diffusion and SEEM), Vitron aims to facilitate The in-depth interaction between LLM and users is similar to OpenAI’s DALL-E series, Midjourney, etc. in the industry. Achieving optimal user interactivity is one of the core goals of this work. Vitron leverages existing language-based LLMs, combined with appropriate instruction adjustments, to achieve a certain level of interactivity. For example, the system can flexibly respond to any expected message input by the user and produce corresponding visual operation results without requiring the user input to exactly match the back-end module conditions. However, this work still has a lot of room for improvement in terms of enhancing interactivity. For example, drawing inspiration from the closed-source Midjourney system, no matter what decision LLM makes at each step, the system should actively provide feedback to users to ensure that its actions and decisions are consistent with user intentions. Modal capabilities Currently, Vitron integrates a 7B Vicuna model, which may have the ability to understand language, images and videos Certain restrictions will apply. Future exploration directions could be to develop a comprehensive end-to-end system, such as expanding the scale of the model to achieve a more thorough and comprehensive understanding of vision. Furthermore, efforts should be made to enable LLM to fully unify the understanding of image and video modalities.
The above is the detailed content of Under the leadership of Yan Shuicheng, Kunlun Wanwei 2050 Global Research Institute jointly released Vitron with NUS and NTU, establishing the ultimate form of general visual multi-modal large models.. For more information, please follow other related articles on the PHP Chinese website!
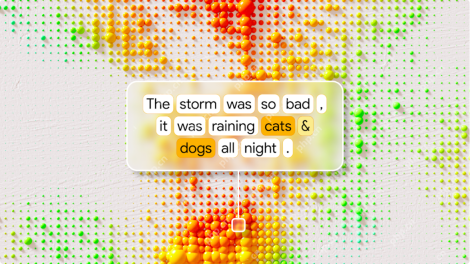
Exploring the Inner Workings of Language Models with Gemma Scope Understanding the complexities of AI language models is a significant challenge. Google's release of Gemma Scope, a comprehensive toolkit, offers researchers a powerful way to delve in
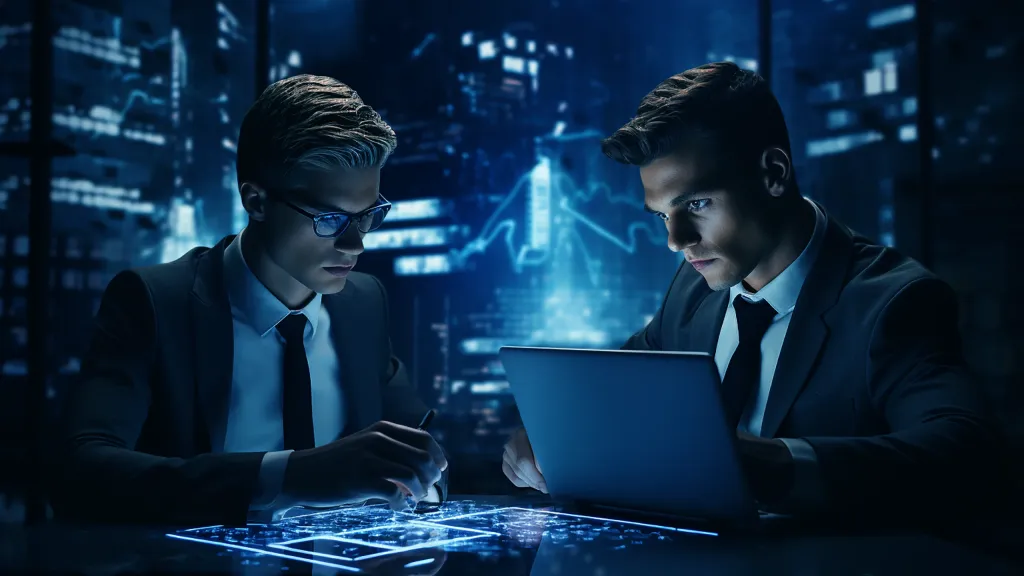
Unlocking Business Success: A Guide to Becoming a Business Intelligence Analyst Imagine transforming raw data into actionable insights that drive organizational growth. This is the power of a Business Intelligence (BI) Analyst – a crucial role in gu

SQL's ALTER TABLE Statement: Dynamically Adding Columns to Your Database In data management, SQL's adaptability is crucial. Need to adjust your database structure on the fly? The ALTER TABLE statement is your solution. This guide details adding colu
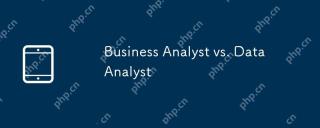
Introduction Imagine a bustling office where two professionals collaborate on a critical project. The business analyst focuses on the company's objectives, identifying areas for improvement, and ensuring strategic alignment with market trends. Simu
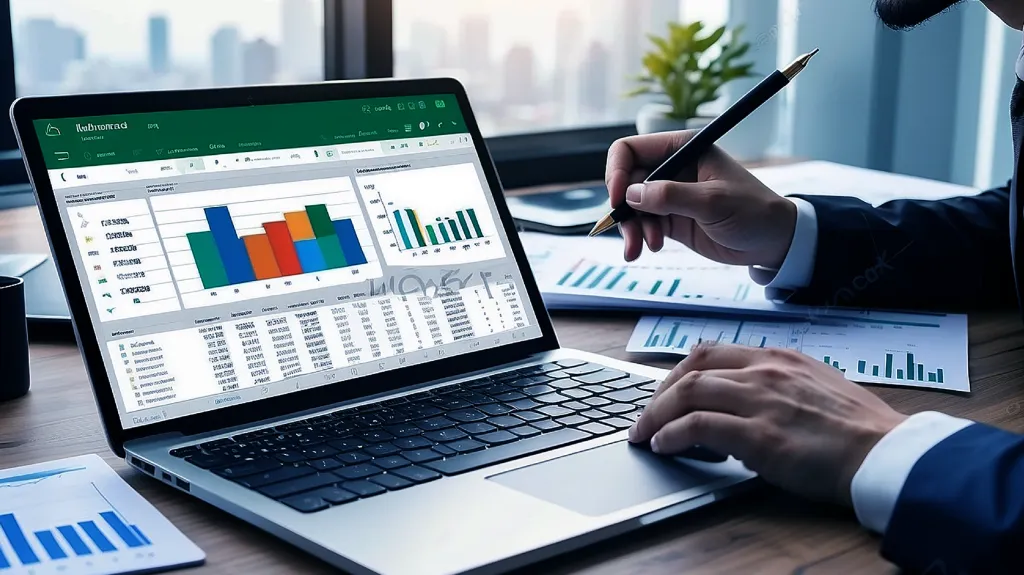
Excel data counting and analysis: detailed explanation of COUNT and COUNTA functions Accurate data counting and analysis are critical in Excel, especially when working with large data sets. Excel provides a variety of functions to achieve this, with the COUNT and COUNTA functions being key tools for counting the number of cells under different conditions. Although both functions are used to count cells, their design targets are targeted at different data types. Let's dig into the specific details of COUNT and COUNTA functions, highlight their unique features and differences, and learn how to apply them in data analysis. Overview of key points Understand COUNT and COU
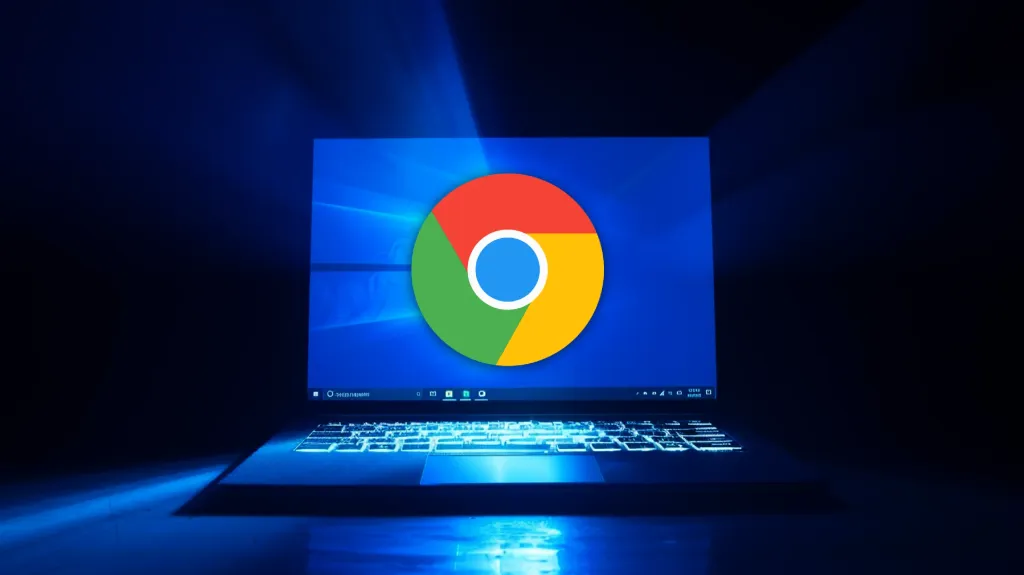
Google Chrome's AI Revolution: A Personalized and Efficient Browsing Experience Artificial Intelligence (AI) is rapidly transforming our daily lives, and Google Chrome is leading the charge in the web browsing arena. This article explores the exciti
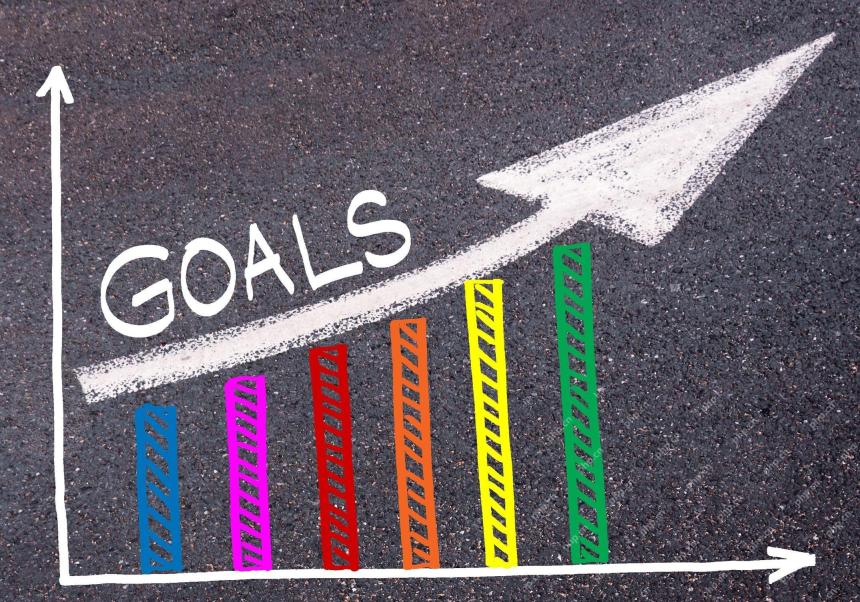
Reimagining Impact: The Quadruple Bottom Line For too long, the conversation has been dominated by a narrow view of AI’s impact, primarily focused on the bottom line of profit. However, a more holistic approach recognizes the interconnectedness of bu
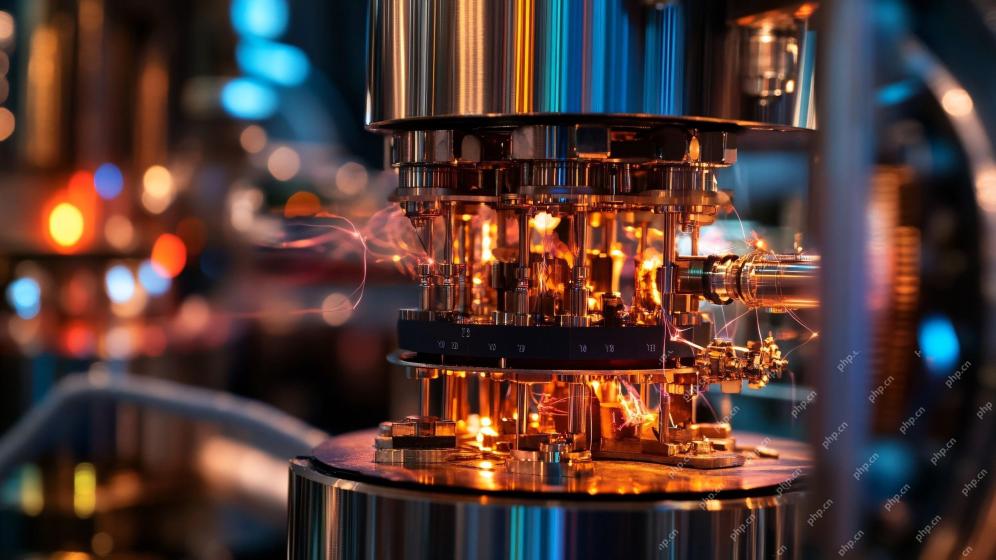
Things are moving steadily towards that point. The investment pouring into quantum service providers and startups shows that industry understands its significance. And a growing number of real-world use cases are emerging to demonstrate its value out


Hot AI Tools

Undresser.AI Undress
AI-powered app for creating realistic nude photos

AI Clothes Remover
Online AI tool for removing clothes from photos.

Undress AI Tool
Undress images for free

Clothoff.io
AI clothes remover

AI Hentai Generator
Generate AI Hentai for free.

Hot Article

Hot Tools
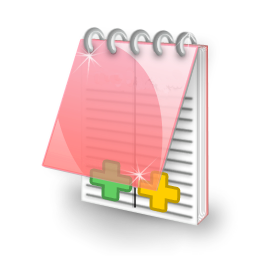
EditPlus Chinese cracked version
Small size, syntax highlighting, does not support code prompt function
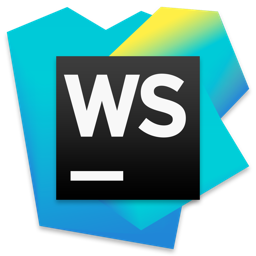
WebStorm Mac version
Useful JavaScript development tools

Safe Exam Browser
Safe Exam Browser is a secure browser environment for taking online exams securely. This software turns any computer into a secure workstation. It controls access to any utility and prevents students from using unauthorized resources.

SublimeText3 English version
Recommended: Win version, supports code prompts!

Zend Studio 13.0.1
Powerful PHP integrated development environment