Spark Streaming and Flink are both stream processing frameworks with different features: Programming model: Spark Streaming is based on the Spark RDD model, while Flink has its own streaming API. State management: Flink has built-in state management, while Spark Streaming requires an external solution. Fault tolerance: Flink is based on snapshots, while Spark Streaming is based on checkpoints. Scalability: Flink is based on streaming operator chains, while Spark Streaming is based on cluster scaling. In real-time data aggregation use cases, Flink generally performs better than Spark Streaming because it provides better throughput and latency.
Spark Streaming and Flink: Comparison of Stream Processing Frameworks
Introduction
Stream processing frameworks are powerful tools for processing real-time data. Spark Streaming and Flink are two leading stream processing frameworks with excellent performance and capabilities for handling large-scale data streams. This article will compare the main features of these two frameworks and demonstrate their differences in practical applications through practical cases.
Feature comparison
Feature | Spark Streaming | Flink |
---|---|---|
Programming model | Spark core RDD model | own streaming API |
Status Management | Difficult to manage, requires external solution | Built-in state management |
Fault tolerance | Checkpoint based | Based on snapshot |
Scalability | Based on cluster expansion | Based on stream operator chain |
Community support | Large and active | Active and constantly developing |
##Practical cases
Use Case: Real-time Data Aggregation
We consider a use case of real-time data aggregation, where streaming data from sensors needs to be continuously aggregated to calculate an average.Spark Streaming implementation
import org.apache.spark.streaming.{StreamingContext, Seconds} import org.apache.spark.streaming.StreamingContext._ import org.apache.spark.sql.SparkSession // 创建 SparkSession 和 StreamingContext val spark = SparkSession.builder().master("local[*]").appName("StreamingAggregation").getOrCreate() val ssc = new StreamingContext(spark.sparkContext, Seconds(1)) // 从文件数据流中创建 DStream val lines = ssc.textFileStream("sensor_data.txt") // 提取传感器 ID 和数值 val values = lines.map(line => (line.split(",")(0), line.split(",")(1).toDouble)) // 计算每分钟平均值 val windowedCounts = values.window(Seconds(60), Seconds(60)).mapValues(v => (v, 1)).reduceByKey((a, b) => (a._1 + b._1, a._2 + b._2)) val averages = windowedCounts.map(pair => (pair._1, pair._2._1 / pair._2._2)) // 打印结果 averages.foreachRDD(rdd => rdd.foreach(println)) // 启动 StreamingContext ssc.start() ssc.awaitTermination()
Flink implementation
import org.apache.flink.streaming.api.environment.StreamExecutionEnvironment; public class FlinkStreamingAggregation { public static void main(String[] args) throws Exception { // 创建 StreamExecutionEnvironment StreamExecutionEnvironment env = StreamExecutionEnvironment.getExecutionEnvironment(); // 从文件数据流中创建 DataStream DataStream<String> lines = env.readTextFile("sensor_data.txt"); // 提取传感器 ID 和数值 DataStream<Tuple2<String, Double>> values = lines .flatMap(s -> Arrays.stream(s.split(",")) .map(v -> new Tuple2<>(v.split("_")[0], Double.parseDouble(v.split("_")[1]))) .iterator()); // 计算每分钟平均值 DataStream<Tuple2<String, Double>> averages = values .keyBy(0) .timeWindow(Time.seconds(60), Time.seconds(60)) .reduce((a, b) -> new Tuple2<>(a.f0, (a.f1 + b.f1) / 2)); // 打印结果 averages.print(); // 执行 Pipeline env.execute("StreamingAggregation"); } }
Performance comparison
In real-time data aggregation use cases, Flink is often considered to be superior to Spark Streaming in terms of performance. This is because Flink’s streaming API and scalability based on streaming operator chains provide better throughput and latency.Conclusion
Spark Streaming and Flink are both powerful stream processing frameworks with their own advantages and disadvantages. Depending on the specific requirements of your application, choosing the right framework is crucial. If you require a high degree of customization and integration with the Spark ecosystem, Spark Streaming may be a good choice. On the other hand, if you need high performance, built-in state management, and scalability, Flink is more suitable. Through the comparison of actual cases, we can more intuitively understand the performance and application of these two frameworks in actual scenarios.The above is the detailed content of Comparison between Spark Streaming and Flink. For more information, please follow other related articles on the PHP Chinese website!
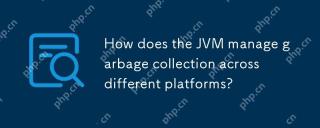
JVMmanagesgarbagecollectionacrossplatformseffectivelybyusingagenerationalapproachandadaptingtoOSandhardwaredifferences.ItemploysvariouscollectorslikeSerial,Parallel,CMS,andG1,eachsuitedfordifferentscenarios.Performancecanbetunedwithflagslike-XX:NewRa
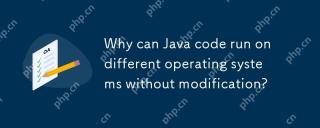
Java code can run on different operating systems without modification, because Java's "write once, run everywhere" philosophy is implemented by Java virtual machine (JVM). As the intermediary between the compiled Java bytecode and the operating system, the JVM translates the bytecode into specific machine instructions to ensure that the program can run independently on any platform with JVM installed.
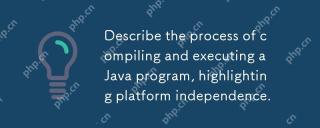
The compilation and execution of Java programs achieve platform independence through bytecode and JVM. 1) Write Java source code and compile it into bytecode. 2) Use JVM to execute bytecode on any platform to ensure the code runs across platforms.
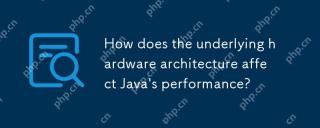
Java performance is closely related to hardware architecture, and understanding this relationship can significantly improve programming capabilities. 1) The JVM converts Java bytecode into machine instructions through JIT compilation, which is affected by the CPU architecture. 2) Memory management and garbage collection are affected by RAM and memory bus speed. 3) Cache and branch prediction optimize Java code execution. 4) Multi-threading and parallel processing improve performance on multi-core systems.
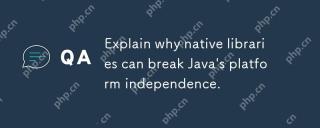
Using native libraries will destroy Java's platform independence, because these libraries need to be compiled separately for each operating system. 1) The native library interacts with Java through JNI, providing functions that cannot be directly implemented by Java. 2) Using native libraries increases project complexity and requires managing library files for different platforms. 3) Although native libraries can improve performance, they should be used with caution and conducted cross-platform testing.
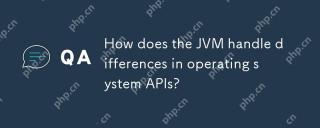
JVM handles operating system API differences through JavaNativeInterface (JNI) and Java standard library: 1. JNI allows Java code to call local code and directly interact with the operating system API. 2. The Java standard library provides a unified API, which is internally mapped to different operating system APIs to ensure that the code runs across platforms.
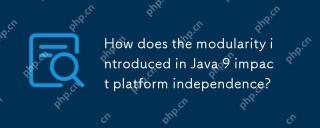
modularitydoesnotdirectlyaffectJava'splatformindependence.Java'splatformindependenceismaintainedbytheJVM,butmodularityinfluencesapplicationstructureandmanagement,indirectlyimpactingplatformindependence.1)Deploymentanddistributionbecomemoreefficientwi
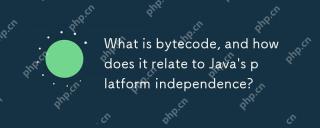
BytecodeinJavaistheintermediaterepresentationthatenablesplatformindependence.1)Javacodeiscompiledintobytecodestoredin.classfiles.2)TheJVMinterpretsorcompilesthisbytecodeintomachinecodeatruntime,allowingthesamebytecodetorunonanydevicewithaJVM,thusfulf


Hot AI Tools

Undresser.AI Undress
AI-powered app for creating realistic nude photos

AI Clothes Remover
Online AI tool for removing clothes from photos.

Undress AI Tool
Undress images for free

Clothoff.io
AI clothes remover

Video Face Swap
Swap faces in any video effortlessly with our completely free AI face swap tool!

Hot Article

Hot Tools
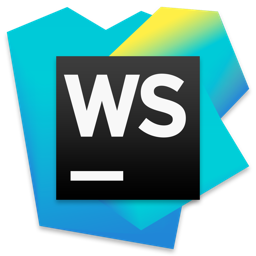
WebStorm Mac version
Useful JavaScript development tools

DVWA
Damn Vulnerable Web App (DVWA) is a PHP/MySQL web application that is very vulnerable. Its main goals are to be an aid for security professionals to test their skills and tools in a legal environment, to help web developers better understand the process of securing web applications, and to help teachers/students teach/learn in a classroom environment Web application security. The goal of DVWA is to practice some of the most common web vulnerabilities through a simple and straightforward interface, with varying degrees of difficulty. Please note that this software
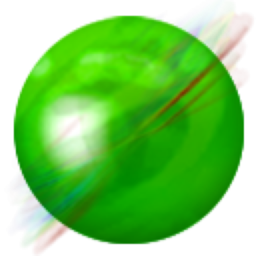
ZendStudio 13.5.1 Mac
Powerful PHP integrated development environment

Atom editor mac version download
The most popular open source editor
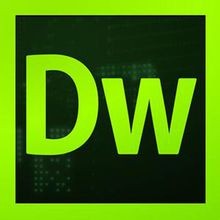
Dreamweaver CS6
Visual web development tools
