Translator | Chen Jun
Reviewer | Chonglou
If someone tells you, software When development and operations teams can effortlessly work together, streamline processes, and increase productivity, you'll definitely think of DevOps. Today, people expect it to harness the power of artificial intelligence (AI) to revolutionize every decision and more easily help educate new DevOps professionals. From a deeper perspective, whether it is automating daily tasks, optimizing asset allocation, or predicting potential problems, artificial intelligence can have a revolutionary impact on DevOps workflows.
learn. To realize the unlimited opportunities of artificial intelligence in DevOps, you need to consider improving team communication efficiency, reducing fault recovery time, and enhancing resilience.
How to integrate artificial intelligence into DevOps?
DevOps teams can think about and implement various new examples of openness from the following aspects: improvements in continuous integration and continuous delivery tools, increases in automated test coverage, infrastructure as code Practice, application of containerization technology, and adoption of cloud native architecture.
CI/CD Pipeline
Artificial intelligence enables enterprises to achieve visibility into their CI/CD processes and control. Using artificial intelligence, enterprises can quickly analyze historical data that has been built, tested and deployed to discover potential failure points and predict possible problems. For example, AI can analyze MySQL's query logs to identify inefficient database queries that impact application performance.
AI-driven systems can also proactively implement preventive measures to minimize the risk of potentially costly delays, failures, and outages during the integration and deployment stages. In addition, AI-driven systems can help optimize resource allocation in CI/CD pipelines, such as leveraging advanced machine learning models (also known as MLOps models) to predict workload and resource needs. In this sense, AI-driven systems can dynamically adjust the coordination of computing power, storage and network resources. This ensures that teams can build and deploy efficiently without wasting valuable resources or running into performance bottlenecks.
Predictive Analytics
In DevOps, the ability to predict and prevent disruption often means the difference between success and catastrophic failure. In response, AI-driven predictive analytics can keep teams one step ahead of potential disruptions. Therefore, AI-driven predictive analytics can allow teams to better respond to disruptions and stay one step ahead of them.
Predictive analytics typically use advanced algorithms and machine learning models to analyze massive amounts of data from various sources, including: application logs, system indicators, and historical event reports. They can then identify patterns and correlations in this data, detect anomalies and provide early warning of impending system failure or performance degradation. This enables teams to take proactive preventive measures before an issue escalates into a full-blown outage.
In addition, AI can continuously analyze data from various infrastructure components such as servers, networks, and storage systems to identify failures or capacity constraints before they occur. Potential hardware failure.
AI-driven code review
Manual operations often involve human error and take too long. In this regard, artificial intelligence tools can analyze the code base at a speed that humans cannot achieve, thereby quickly and at scale identifying potential issues such as: performance bottlenecks, code that does not meet best practices or internal standards, security responsibilities, and coding style. .
At the same time, more and more tools can provide developers with operational intelligence and suggested action plans, thereby significantly reducing code while solving discovered problems. Defects introduced into the library and accumulated technical debt risks.
In a broader sense, the following models and tools can also propose code base optimization measures:
- DeepSeek-Coder6.7B/33BPhind-CodeLlama v2
- ##Deepseek 67b
- CodeCapybara
- GPT-4-1106
The above model has achieved good results in terms of automation and simplified quality control. Effect. By using these solutions appropriately, DevOps teams can accelerate delivery cycles, reduce the risk of costly post-deployment issues, and ensure comprehensive quality control at all times.
Automatic security checks
In order to avoid the occurrence of vulnerabilities, implementing and executing appropriate security measures may often slow down the normal development cycle. And artificial intelligence can just simplify the process and improve efficiency. Automated security checks driven by artificial intelligence, unlike traditional static security solutions, have the ability to continuously learn and "grow" to adapt to various emerging threats by analyzing the patterns and techniques used by malicious actors.
At the same time, the artificial intelligence-driven automated security check function can be seamlessly integrated into the DevOps workflow to achieve continuous improvement at all stages of the software development life cycle (SDLC). security monitoring and verification.
Feedback and Optimization
Although automating various tasks and processes is an important part of artificial intelligence, an overlooked function is, It also improves the feedback loop between operations, end users and DevOps teams. Being good at sifting through large amounts of data, these tools are ideal for analyzing things like system logs, user behavior, application performance metrics, and direct feedback from end customers.
In addition, these tools can also use natural language processing (NLP, Natural Language Processing) and machine learning to identify patterns and trends to point out application performance, usability and overall user satisfaction. areas that need improvement. Moreover, this intelligent analysis allows the development team to prioritize modifications and enhancements based on real user needs and system performance, so that the product can better meet user expectations and run according to actual conditions.
Tools and Techniques for Integrating Artificial Intelligence into DevOps
The integration of Artificial Intelligence and DevOps has given rise to a series of tools and techniques designed to increase automation and efficiency. tool. While many organizations may default to the popular Google Cloud, a growing number of DevOps teams are looking at alternatives to discover AI-powered ones that are more affordable and better suited for specific jobs. Streaming service. For example, Oracle and Alibaba Cloud have become increasingly popular in this field, and their artificial intelligence capabilities are iterating month by month.
Code Review and Quality Assurance
We can consider using solutions such as DeepCode, Codacy and SonarSource to analyze the code base using machine learning algorithms , identify potential vulnerabilities, code defects, and violations of best practices, and then optimize the existing code analysis and review process.
And in terms of testing and quality assurance, Applitools, Functionize and ## Artificial intelligence-driven tools such as #Mabl can automatically create and execute tests through visual machine learning technology. Of course, if you choose to use a locally hosted large model it may require specialized training to specialize it in DevOps tasks (especially CI/CD). In addition, in terms of infrastructure management and monitoring, artificial intelligence-enhanced platforms such as Moogsoft and Dynatrace can provide advanced anomaly detection and root cause analysis services, through real-time analysis of operating data, To predict and prevent potential system failures.
DevOps tools for non-technical people
Currently, a common misconception is that AI-driven DevOps tools are only for those with huge "Specially available" for large enterprises with resources and complex software development needs. This is not the case. AI-powered solutions like Harness and CodeGuru are ideal for smaller teams because of their flexibility. The fact that small IT teams are often constantly operating at full capacity means that they need to use a variety of open source artificial intelligence tools for DevOps tasks that can be customized to their specific needs.
Best Practices for Integrating Artificial Intelligence into DevOps
Momentum continues to build as we integrate Artificial Intelligence into DevOps , enterprises can unleash the full potential of AI-driven DevOps automation through the following best practices to mitigate potential challenges:
- OK Clear goals and metrics: Teams must first identify specific goals they want to achieve by integrating AI in the DevOps cycle. Whether it’s increasing deployment frequency, improving code quality, reducing failure rates, or speeding up incident response times, clear goals can help teams choose the right AI tools and technologies.
- Start small and iterate: Rather than trying to overhaul DevOps processes, identify specific areas where AI may bring immediate value. Teams should start with pilot projects or proof-of-concepts and gradually expand AI integration as they gain experience and confidence.
- Ensure data quality and management: Since AI algorithms rely heavily on data, teams must establish and improve data governance practices in a timely manner. Only when the quality, integrity and accessibility of data are effectively guaranteed will it become easier to implement processes such as data cleaning, verification and management.
Summary
To sum up, artificial intelligence has increasingly been integrated into the broader DevOps framework and has brought new challenges to the daily life of DevOps. Significant changes in processing methods and efficiency. Especially in CI/CD, predictive analytics powered by AI will help DevOps teams continuously change customer service pipelines and optimize resource allocation while staying ahead of the curve. It is no exaggeration to say that if enterprises want to effectively gain competitive advantage, integrating artificial intelligence into DevOps is not only a possibility, but also an inevitable choice.
Translator Introduction
Julian Chen, 51CTO community editor, has more than ten years of experience in IT project implementation and is good at Implement management and control of internal and external resources and risks, and focus on disseminating network and information security knowledge and experience.
Original title: Next-Gen DevOps: Integrate AI for Enhanced Workflow Automation By Alexander T. Williams
The above is the detailed content of Integrate AI into DevOps to enhance workflow automation. For more information, please follow other related articles on the PHP Chinese website!
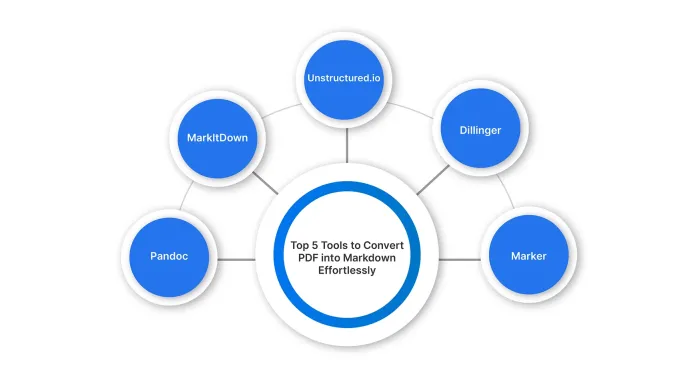
Different formats, such as PPTX, DOCX, or PDF, to Markdown converter is an essential tool for content writers, developers, and documentation specialists. Having the right tools makes all the difference when converting any type of
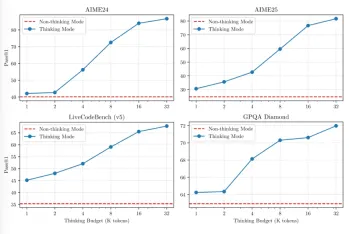
Qwen has been silently adding one model after the other. Each of its models comes packed with features so big and sizes so quantized that they are just impossible to ignore. After QvQ, Qwen2.5-VL, and Qwen2.5-Omni this year, the
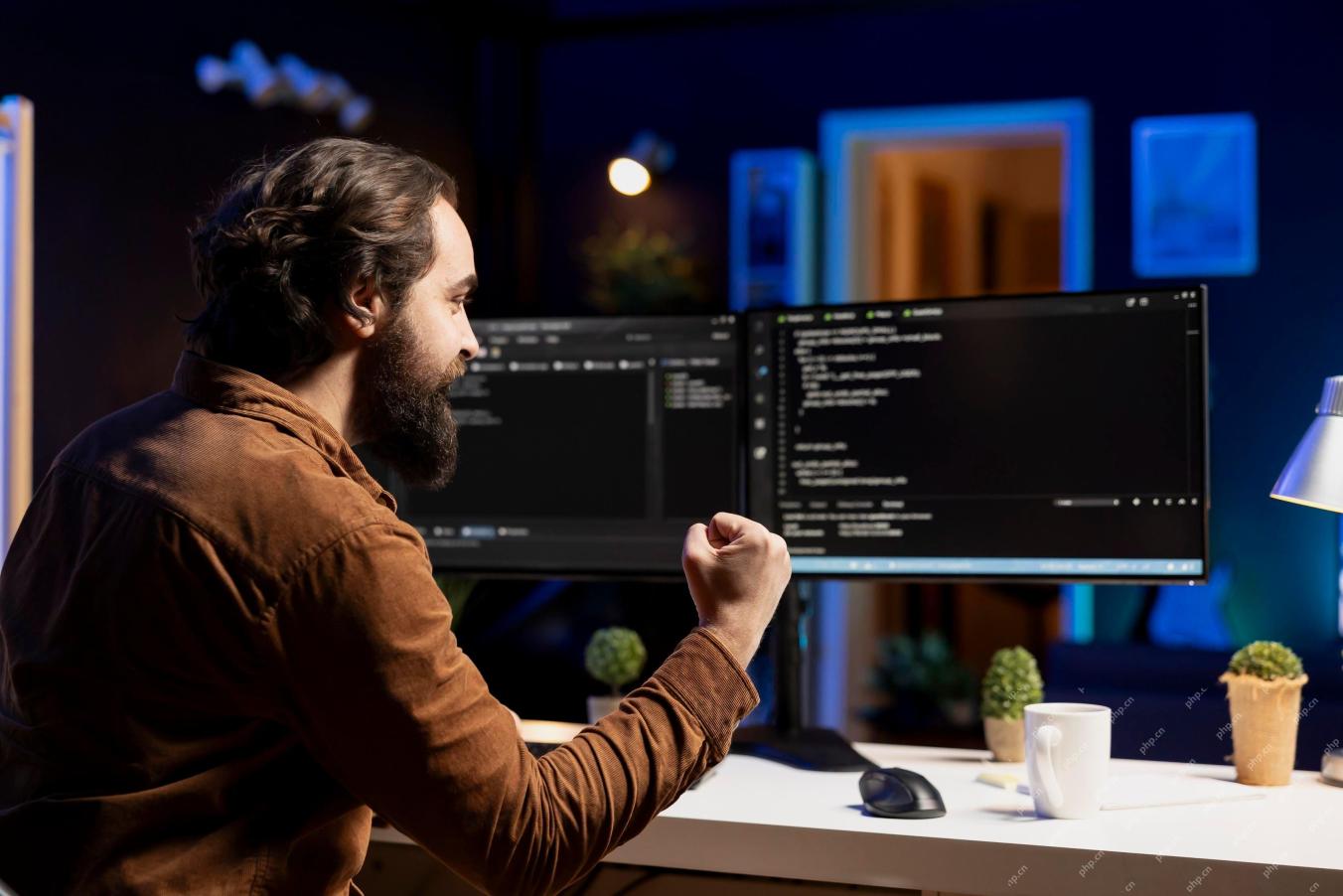
Let's discuss the rising use of "vibes" as an evaluation metric in the AI field. This analysis is part of my ongoing Forbes column on AI advancements, exploring complex aspects of AI development (see link here). Vibes in AI Assessment Tradi
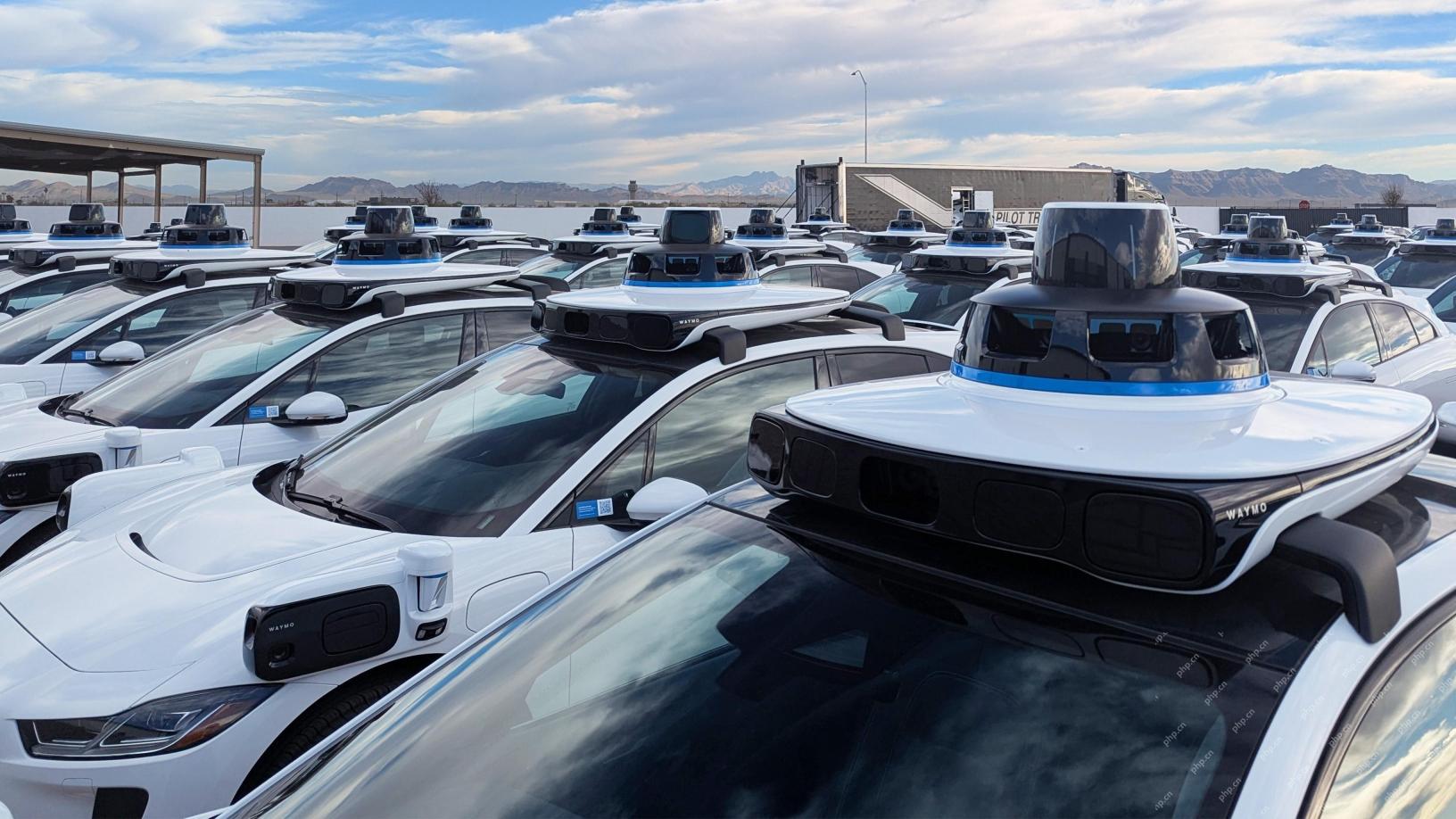
Waymo's Arizona Factory: Mass-Producing Self-Driving Jaguars and Beyond Located near Phoenix, Arizona, Waymo operates a state-of-the-art facility producing its fleet of autonomous Jaguar I-PACE electric SUVs. This 239,000-square-foot factory, opened
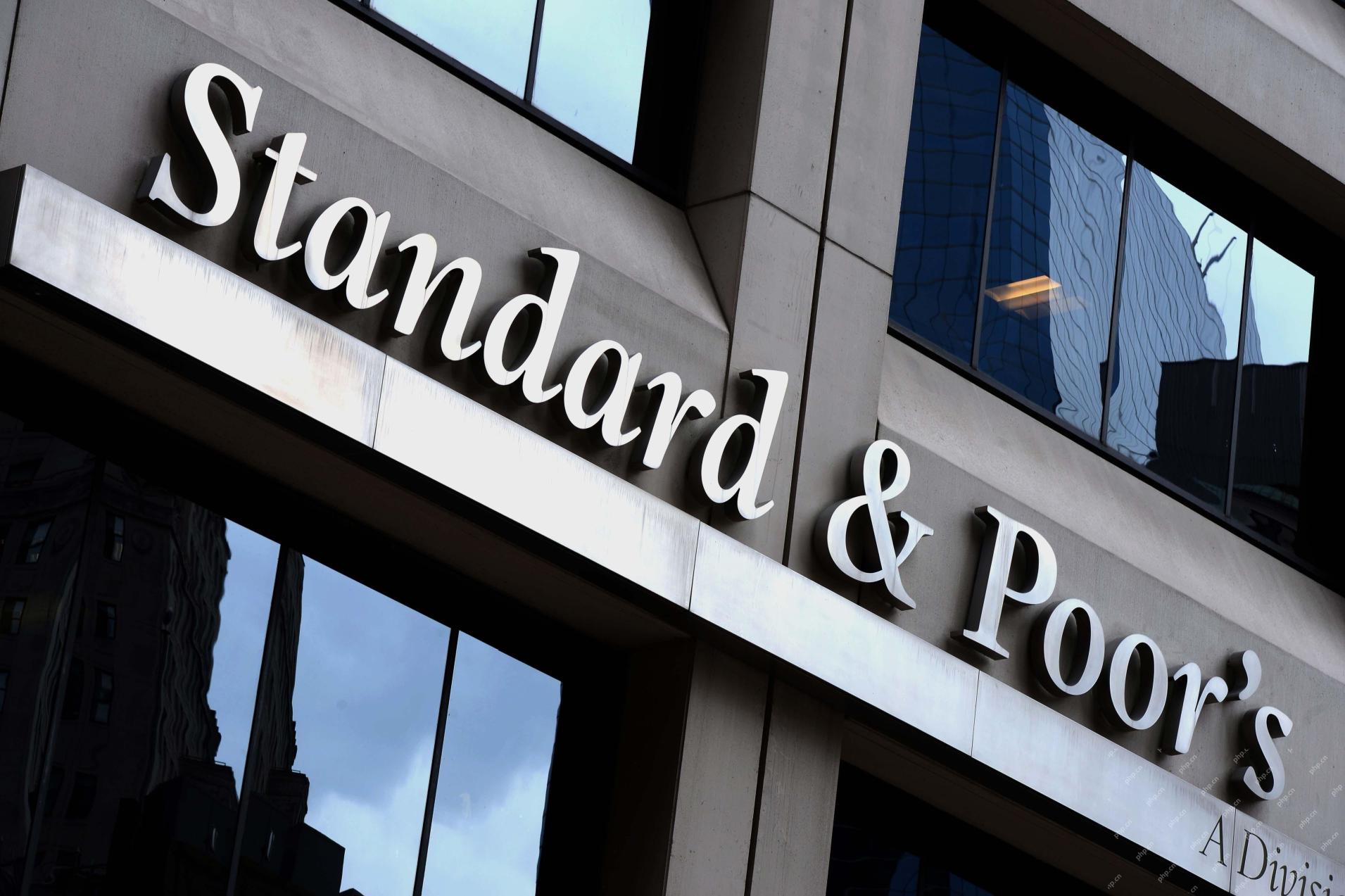
S&P Global's Chief Digital Solutions Officer, Jigar Kocherlakota, discusses the company's AI journey, strategic acquisitions, and future-focused digital transformation. A Transformative Leadership Role and a Future-Ready Team Kocherlakota's role
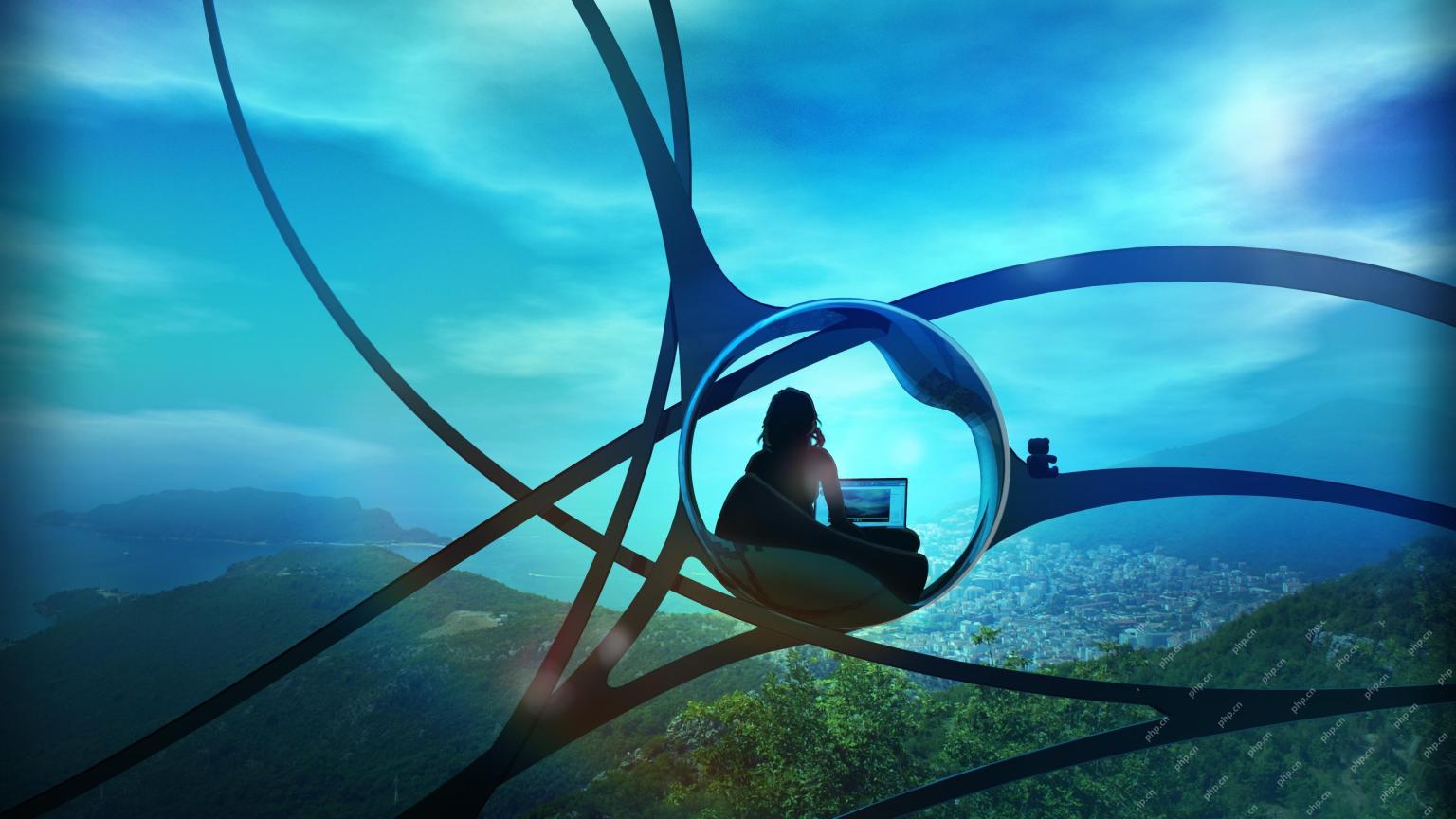
From Apps to Ecosystems: Navigating the Digital Landscape The digital revolution extends far beyond social media and AI. We're witnessing the rise of "everything apps"—comprehensive digital ecosystems integrating all aspects of life. Sam A
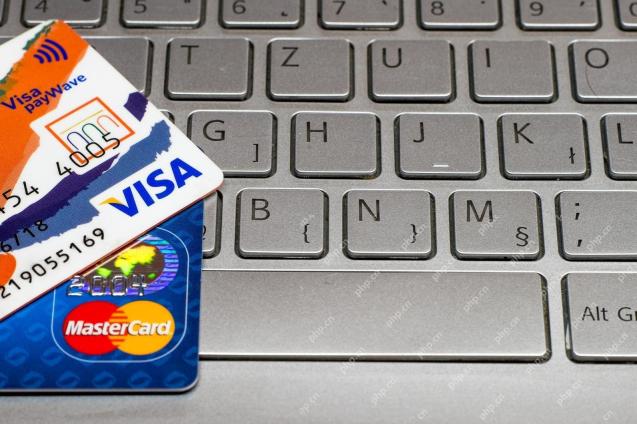
Mastercard's Agent Pay: AI-Powered Payments Revolutionize Commerce While Visa's AI-powered transaction capabilities made headlines, Mastercard has unveiled Agent Pay, a more advanced AI-native payment system built on tokenization, trust, and agentic
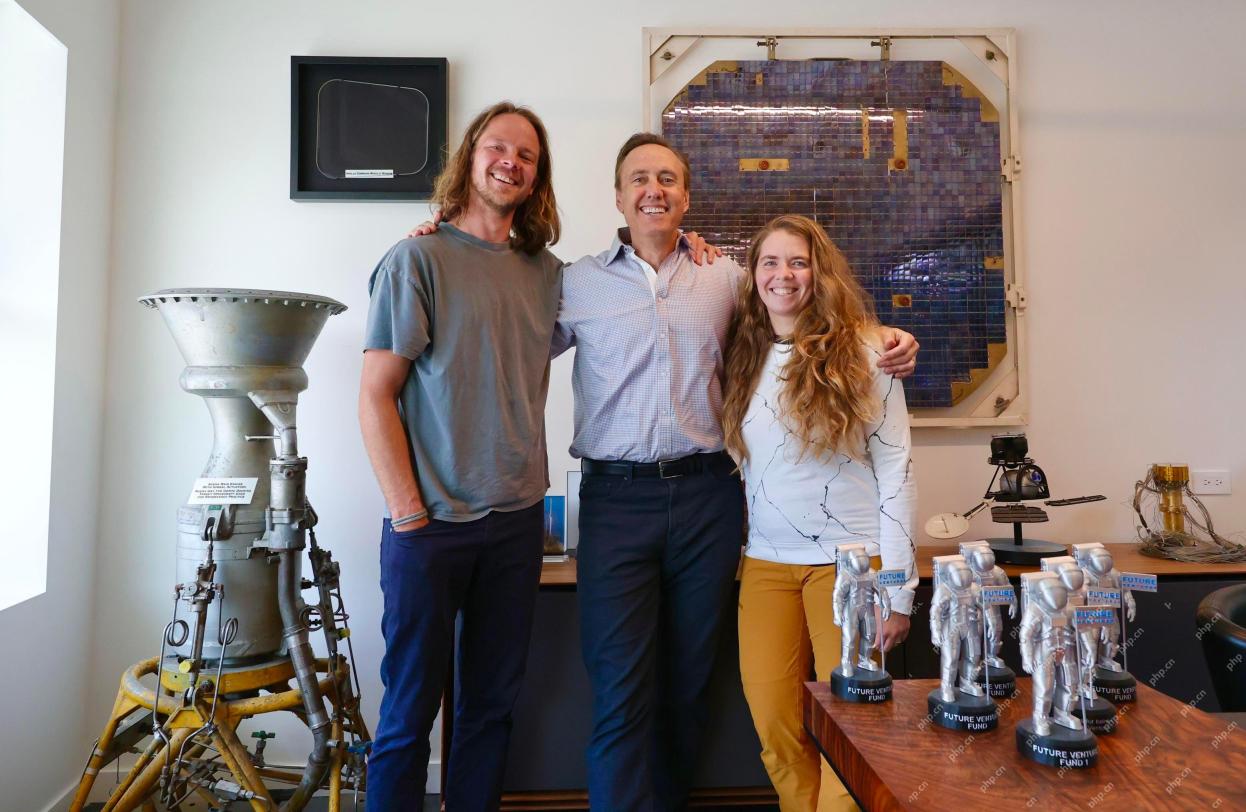
Future Ventures Fund IV: A $200M Bet on Novel Technologies Future Ventures recently closed its oversubscribed Fund IV, totaling $200 million. This new fund, managed by Steve Jurvetson, Maryanna Saenko, and Nico Enriquez, represents a significant inv


Hot AI Tools

Undresser.AI Undress
AI-powered app for creating realistic nude photos

AI Clothes Remover
Online AI tool for removing clothes from photos.

Undress AI Tool
Undress images for free

Clothoff.io
AI clothes remover

Video Face Swap
Swap faces in any video effortlessly with our completely free AI face swap tool!

Hot Article

Hot Tools

mPDF
mPDF is a PHP library that can generate PDF files from UTF-8 encoded HTML. The original author, Ian Back, wrote mPDF to output PDF files "on the fly" from his website and handle different languages. It is slower than original scripts like HTML2FPDF and produces larger files when using Unicode fonts, but supports CSS styles etc. and has a lot of enhancements. Supports almost all languages, including RTL (Arabic and Hebrew) and CJK (Chinese, Japanese and Korean). Supports nested block-level elements (such as P, DIV),

Safe Exam Browser
Safe Exam Browser is a secure browser environment for taking online exams securely. This software turns any computer into a secure workstation. It controls access to any utility and prevents students from using unauthorized resources.

SublimeText3 Chinese version
Chinese version, very easy to use
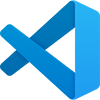
VSCode Windows 64-bit Download
A free and powerful IDE editor launched by Microsoft

DVWA
Damn Vulnerable Web App (DVWA) is a PHP/MySQL web application that is very vulnerable. Its main goals are to be an aid for security professionals to test their skills and tools in a legal environment, to help web developers better understand the process of securing web applications, and to help teachers/students teach/learn in a classroom environment Web application security. The goal of DVWA is to practice some of the most common web vulnerabilities through a simple and straightforward interface, with varying degrees of difficulty. Please note that this software
