


More than 13 times faster than manual work, 'robot + AI' discovers the best electrolyte for batteries and accelerates materials research
Editor | Ziluo
The traditional material research and development model mainly relies on "trial and error" experimental methods or accidental discoveries, and its research and development process generally lasts 10-20 years.
Data-driven methods based on machine learning (ML) can accelerate the design of new materials for clean energy technologies. However, its practical application in materials research is still limited due to the lack of large-scale high-fidelity experimental databases.
Recently, research teams from the Pacific Northwest National Laboratory and Argonne National Laboratory in the United States designed a highly automated workflow that combines a high-throughput experimental platform with the most advanced active learning algorithms to Efficient screening of binary organic solvents with optimal solubility for the anolyte. The goal of this research is to improve the performance and stability of energy storage systems to promote the widespread application of renewable energy. Traditionally, research involving anolytes usually requires a lot of trial and error experiments, which is time-consuming and labor-intensive. Using this automated workflow, researchers can more quickly screen out suitable binary compounds. In addition to an efficient workflow designed to develop high-performance redox flow batteries, this machine learning-guided High-throughput robotic platforms provide a powerful and versatile approach to accelerate the discovery of functional materials.
The reviewer commented: "This study shows that an AI-guided robotic platform can effectively find non-intuitive combinations of solvents and electrolytes in energy applications. This work has important implications for the battery community."
The research is titled "
An integrated high-throughput robotic platform and active learning approach for accelerated discovery of optimal electrolyte formulations" and was published in "Nature Communications" on March 29, 2024 "superior.
Paper link:
for It is crucial to ensure the development of clean energy technology applications and achieve deep decarbonization of electricity, so designing materials with targeted functional properties of tools is critical to developing clean energy technology applications and achieving deep decarbonization of electricity. Traditional trial-and-error methods are costly and time-consuming, so design tools are inherently expensive and time-saving.
The solubility of redox active molecules is an important factor in determining the energy density of redox flow batteries (RFB). However, electrolyte materials discovery is limited by the lack of experimental solubility data sets that are critical to exploit data-driven approaches.
Nonetheless, the development of highly soluble redox-active organic molecules (ROMs) for non-aqueous RFBs (NRFBs) remains a daunting task due to the lack of standardization of organic solvent systems and application-relevant experimental solubility data. task.
By utilizing the automated high-throughput experiment (HTE) platform, the reliability and efficiency of the "excess solute" solubility measurement method can be improved and the NRFB's solubility database can be constructed. However, even with HTE systems, the diversity of potential solvent mixtures makes the screening process more time-consuming and expensive.
Active learning (AL), and specifically Bayesian optimization (BO), has proven to be a reliable method to accelerate the search for electrolytes needed for energy storage applications. Therefore, a closed-loop experimental workflow guided by BO can be used to minimize HTE execution.
ML-guided high-throughput experimental robotic platformHere, researchers used 2,1,3-benzothiadiazole (2,1,3 -benzothiadiazole (BTZ), a high-performance anolyte with a high degree of delocalized charge density and good chemical stability, serves as a model ROM. The focus is on studying its solubility in various organic solvents, demonstrating the potential of a machine learning-guided high-throughput experiment (HTE) robotic platform to accelerate NRFB electrolyte discovery.
Illustration: Schematic diagram of the closed-loop electrolyte screening process based on a high-throughput experimental platform guided by machine learning (ML). (Source: paper)
Specifically, the researchers designed a closed-loop solvent screening workflow consisting of two connected modules, HTE and BO. The HTE module performs sample preparation and solubility measurements via a high-throughput robotic platform. The BO component consists of a surrogate model and an acquisition function, which together act as an oracle, making solubility predictions and suggesting new solvents for evaluation.
The workflow is shown in the figure below, the specific steps are:More than 13 times faster than manual sample processing The automated platform can prepare saturated solutions with solute excess and quantitative nuclear magnetic resonance (qNMR) with minimal manual intervention ) sample. With the automated HTE workflow, the total experimental time to complete the solubility measurements of 42 samples was approximately 27 hours (~39 minutes/sample, less time per sample when running more samples). This is more than 13 times faster than manually processing samples using the "excess solute" method (approximately 525 minutes per sample). In addition to the speed increase provided by the HTE system, research also placed great emphasis on controlling experimental conditions, such as temperature (20°C) and stabilization time (8 hours), to ensure accurate measurement of BTZ solubility in various organic solvents . Illustration: Overview of the automated high-throughput experiment (HTE) platform. (Source: Paper) Based on a literature review and consideration of solvent properties, the researchers listed 22 potential candidate solvents for BTZ. Then, an additional 2079 binary solvents were further enumerated by combining these 22 single solvents in pairs, each with 9 different volume fractions. Table: List of 22 candidate organic solvents and their physical and chemical properties. (Source: paper) #The platform identifies multiple solvents from a comprehensive library of more than 2000 potential solvents, with prototype redox active molecules2,1,3 -The solubility threshold of benzothiadiazole exceeds 6.20 M. Notably, the comprehensive strategy required solubility assessment for less than 10% of drug candidates, highlighting the efficiency of the new approach. Illustration: Identification of required electrolytes via Bayesian Optimization (BO). (Source: paper) The research results also show that binary solvent mixtures, especially those incorporating 1,4-dioxane (1,4-dioxane), help improve the BTZ solubility. In conclusion, the study demonstrates an ML-guided HTE platform for electrolyte screening, where ML predictions and automated experiments work together to effectively screen binary organic solvents with optimal solubility for BTZ. This research not only helps connect the fields of data science and traditional experimental science, but also lays the foundation for the future development of an autonomous platform dedicated to battery electrolyte screening.
The above is the detailed content of More than 13 times faster than manual work, 'robot + AI' discovers the best electrolyte for batteries and accelerates materials research. For more information, please follow other related articles on the PHP Chinese website!
![Can't use ChatGPT! Explaining the causes and solutions that can be tested immediately [Latest 2025]](https://img.php.cn/upload/article/001/242/473/174717025174979.jpg?x-oss-process=image/resize,p_40)
ChatGPT is not accessible? This article provides a variety of practical solutions! Many users may encounter problems such as inaccessibility or slow response when using ChatGPT on a daily basis. This article will guide you to solve these problems step by step based on different situations. Causes of ChatGPT's inaccessibility and preliminary troubleshooting First, we need to determine whether the problem lies in the OpenAI server side, or the user's own network or device problems. Please follow the steps below to troubleshoot: Step 1: Check the official status of OpenAI Visit the OpenAI Status page (status.openai.com) to see if the ChatGPT service is running normally. If a red or yellow alarm is displayed, it means Open
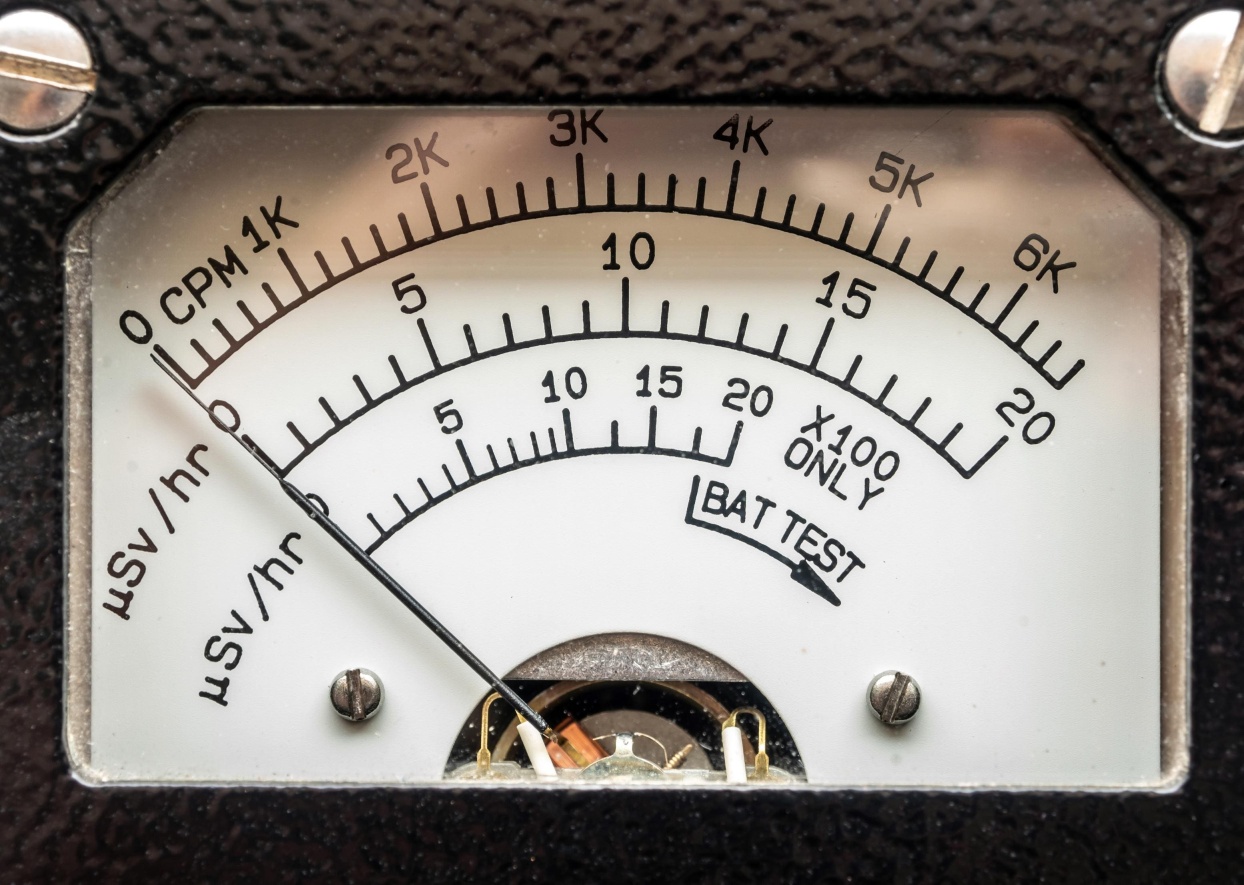
On 10 May 2025, MIT physicist Max Tegmark told The Guardian that AI labs should emulate Oppenheimer’s Trinity-test calculus before releasing Artificial Super-Intelligence. “My assessment is that the 'Compton constant', the probability that a race to
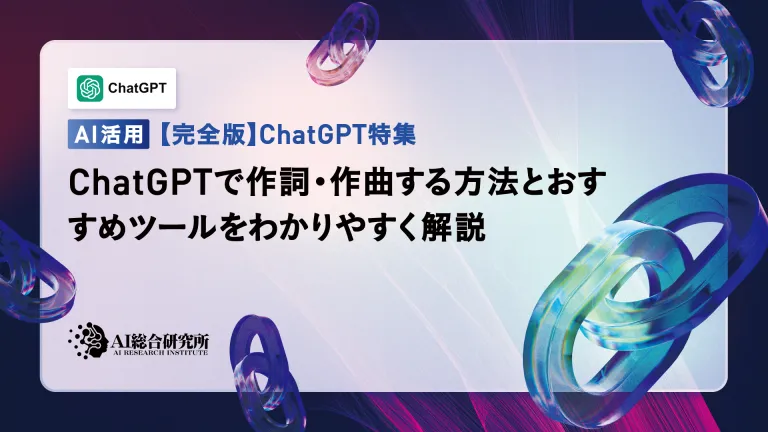
AI music creation technology is changing with each passing day. This article will use AI models such as ChatGPT as an example to explain in detail how to use AI to assist music creation, and explain it with actual cases. We will introduce how to create music through SunoAI, AI jukebox on Hugging Face, and Python's Music21 library. Through these technologies, everyone can easily create original music. However, it should be noted that the copyright issue of AI-generated content cannot be ignored, and you must be cautious when using it. Let’s explore the infinite possibilities of AI in the music field together! OpenAI's latest AI agent "OpenAI Deep Research" introduces: [ChatGPT]Ope
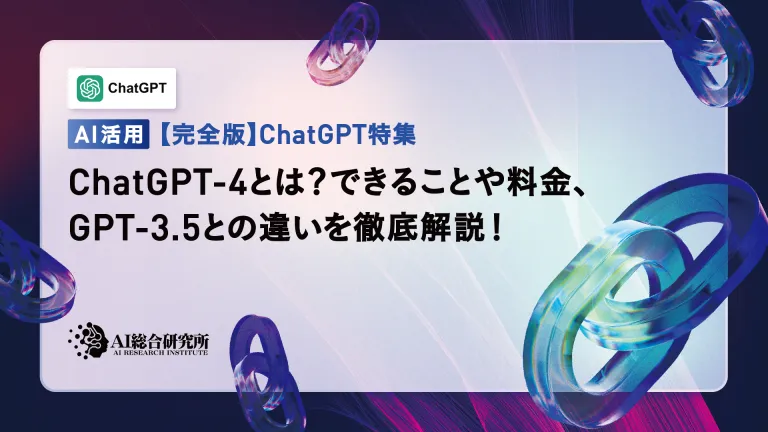
The emergence of ChatGPT-4 has greatly expanded the possibility of AI applications. Compared with GPT-3.5, ChatGPT-4 has significantly improved. It has powerful context comprehension capabilities and can also recognize and generate images. It is a universal AI assistant. It has shown great potential in many fields such as improving business efficiency and assisting creation. However, at the same time, we must also pay attention to the precautions in its use. This article will explain the characteristics of ChatGPT-4 in detail and introduce effective usage methods for different scenarios. The article contains skills to make full use of the latest AI technologies, please refer to it. OpenAI's latest AI agent, please click the link below for details of "OpenAI Deep Research"
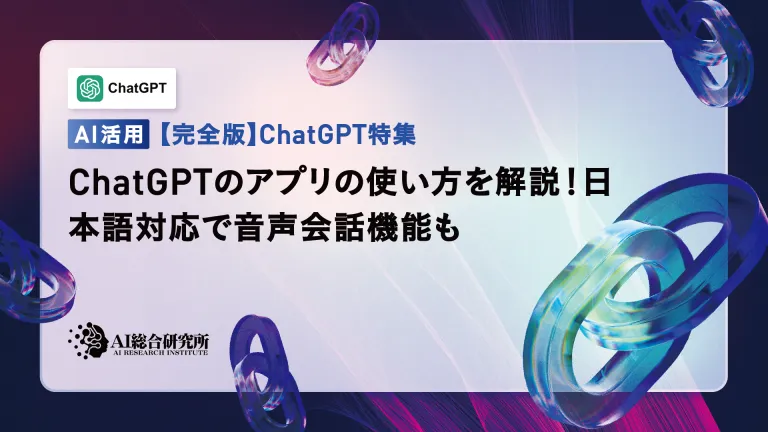
ChatGPT App: Unleash your creativity with the AI assistant! Beginner's Guide The ChatGPT app is an innovative AI assistant that handles a wide range of tasks, including writing, translation, and question answering. It is a tool with endless possibilities that is useful for creative activities and information gathering. In this article, we will explain in an easy-to-understand way for beginners, from how to install the ChatGPT smartphone app, to the features unique to apps such as voice input functions and plugins, as well as the points to keep in mind when using the app. We'll also be taking a closer look at plugin restrictions and device-to-device configuration synchronization
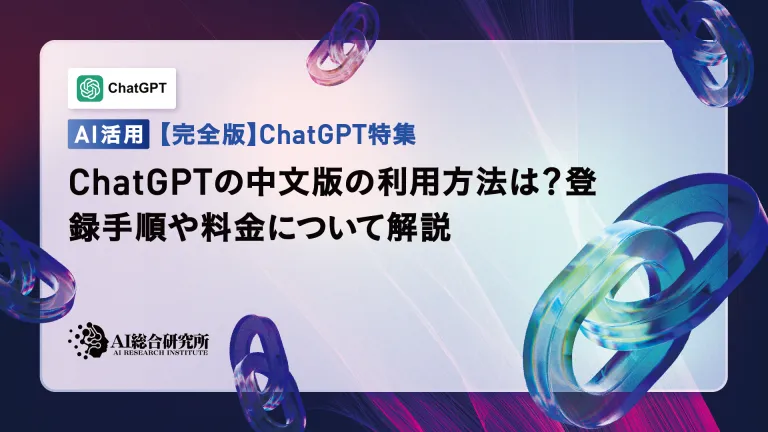
ChatGPT Chinese version: Unlock new experience of Chinese AI dialogue ChatGPT is popular all over the world, did you know it also offers a Chinese version? This powerful AI tool not only supports daily conversations, but also handles professional content and is compatible with Simplified and Traditional Chinese. Whether it is a user in China or a friend who is learning Chinese, you can benefit from it. This article will introduce in detail how to use ChatGPT Chinese version, including account settings, Chinese prompt word input, filter use, and selection of different packages, and analyze potential risks and response strategies. In addition, we will also compare ChatGPT Chinese version with other Chinese AI tools to help you better understand its advantages and application scenarios. OpenAI's latest AI intelligence
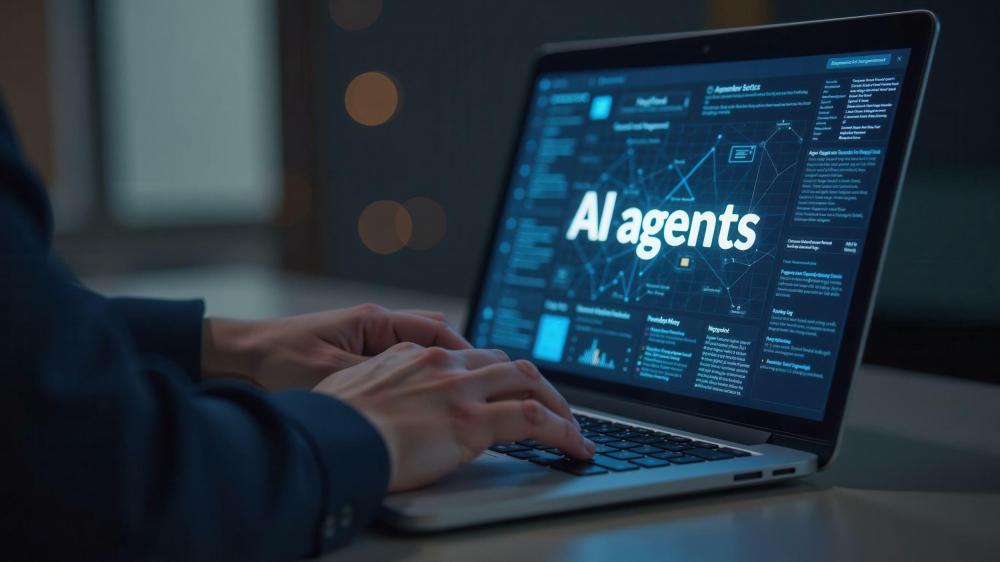
These can be thought of as the next leap forward in the field of generative AI, which gave us ChatGPT and other large-language-model chatbots. Rather than simply answering questions or generating information, they can take action on our behalf, inter
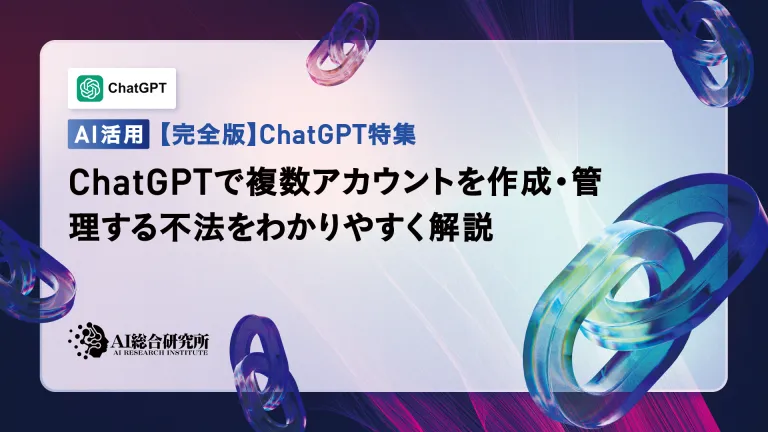
Efficient multiple account management techniques using ChatGPT | A thorough explanation of how to use business and private life! ChatGPT is used in a variety of situations, but some people may be worried about managing multiple accounts. This article will explain in detail how to create multiple accounts for ChatGPT, what to do when using it, and how to operate it safely and efficiently. We also cover important points such as the difference in business and private use, and complying with OpenAI's terms of use, and provide a guide to help you safely utilize multiple accounts. OpenAI


Hot AI Tools

Undresser.AI Undress
AI-powered app for creating realistic nude photos

AI Clothes Remover
Online AI tool for removing clothes from photos.

Undress AI Tool
Undress images for free

Clothoff.io
AI clothes remover

Video Face Swap
Swap faces in any video effortlessly with our completely free AI face swap tool!

Hot Article

Hot Tools

SublimeText3 Linux new version
SublimeText3 Linux latest version

MantisBT
Mantis is an easy-to-deploy web-based defect tracking tool designed to aid in product defect tracking. It requires PHP, MySQL and a web server. Check out our demo and hosting services.

Zend Studio 13.0.1
Powerful PHP integrated development environment

SAP NetWeaver Server Adapter for Eclipse
Integrate Eclipse with SAP NetWeaver application server.
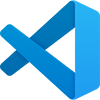
VSCode Windows 64-bit Download
A free and powerful IDE editor launched by Microsoft
