Introduction
In the era of information explosion, data has become one of the most valuable assets of an enterprise. However, if a large amount of data cannot be effectively classified and classified, it will become disordered and chaotic, data security cannot be effectively guaranteed, and its true data value cannot be exerted. Therefore, data classification and grading have become crucial for both data security and data value. This article will discuss the importance of data classification and classification, and introduce how to use machine learning to achieve intelligent classification and classification of data.
1. The importance of data classification and grading
Data classification and grading is the process of classifying and sorting data according to certain rules and standards. It can help enterprises better manage data and improve data confidentiality, availability, integrity and accessibility, thereby better supporting business decision-making and development. The following is the importance of data classification and grading: 1. Confidentiality: By classifying and grading data, data can be encrypted and permissions controlled according to different levels of sensitivity to ensure data security. 2. Availability: Through data classification and grading, we can better understand the importance and urgency of data, thereby rationally allocating resources and formulating backup strategies to ensure timely availability of data. 3. Integrity: Through data classification and grading, data can be effectively verified and verified to ensure the integrity of the data
Improve data utilization: By classifying and grading data, we can more accurately understand the nature and characteristics of the data, thereby making better use of data for analysis and mining, and improving the value and utilization of data.
Reduce data management costs: When the amount of data is large and disordered, the cost of data management and maintenance is often high. By classifying and grading data, data can be managed in an orderly manner, reducing unnecessary duplication of work and reducing data management costs.
Strengthen data security protection: Data classification and grading can provide different levels of targeted protection based on the sensitivity of the data to avoid unauthorized access. Access or disclosure by authorized personnel.
Data sharing and cooperation: On the basis of classification and grading, formulate corresponding authority management mechanisms. According to different categories and levels Authorize data to meet sharing and cooperation, and strengthen information communication.
Support business decisions: Data is an important basis for supporting business decisions. By classifying and grading data, the meaning and relevance of the data can be better understood, providing more reliable support and reference for business decisions.
2. Machine learning and data classification and grading
1. Supervised learning
Supervision Formula learning is a machine learning method that uses known inputs and outputs to train a model. In data classification and grading, supervised learning can train models through labeled data samples and achieve intelligent classification and grading. Supervised learning uses labeled data samples to train models and achieve intelligent classification and classification, which can be applied in data classification and classification.
Text classification: In text data processing, supervised learning can train models through labeled text data samples to achieve text Automatic classification, such as sentiment analysis, topic recognition, etc.
Image recognition: In image data processing, supervised learning can train the model through labeled image data samples to achieve image Automatic classification, such as object recognition, face recognition, etc.
Audio recognition: In audio data processing, supervised learning can train the model through labeled audio data samples to achieve audio Automatic classification, such as speech recognition, music classification, etc.
2. Unsupervised learning
Unsupervised learning is a machine learning method that does not rely on labeled data for training. In data classification and grading, unsupervised learning can classify and classify based on the characteristics and structure of the data itself, thereby achieving intelligent classification and grading. The following is the application of unsupervised learning in data classification and classification:
Cluster analysis: In cluster analysis, unsupervised learning Learning can divide data samples into different categories through the similarities between data samples to achieve automatic classification of data, such as user grouping, product classification, etc.
Association rule mining: In association rule mining, unsupervised learning can classify and classify data by discovering the association between data samples to achieve data classification. Automatic classification, such as shopping basket analysis, recommendation system, etc.
Anomaly detection: In anomaly detection, unsupervised learning can perform classification and classification by discovering abnormal behaviors among data samples. , to achieve automatic classification of data, such as network security monitoring, fraud detection, etc.
3. Semi-supervised learning
Semi-supervised learning is a type of machine learning that combines supervised learning and unsupervised learning method. In data classification and grading, semi-supervised learning can train models with a small number of labeled data samples and a large number of unlabeled data samples, thereby achieving intelligent classification and grading. The following is the application of semi-supervised learning in data classification and grading:
Semi-supervised text classification: In text data processing, semi-supervised learning Supervised learning can train the model through a small number of labeled text data samples and a large number of unlabeled text data samples to achieve automatic text classification.
Semi-supervised image classification: In image data processing, semi-supervised learning can be achieved through a small number of labeled image data samples and a large number of Unlabeled image data samples are used to train the model to achieve automatic classification of images.
Semi-supervised anomaly detection: In anomaly detection, semi-supervised learning can be achieved through a small number of labeled normal data samples and a large number of Unlabeled data samples are used to train the model to achieve automatic classification of abnormal data.
4. Matching of business scenarios and AI training methods
In practical applications, choose the appropriate AI training method to match the business scenario is crucial. The following are some suggestions for matching business scenarios with AI training methods:
For business scenarios that already have a large amount of labeled data, you can choose a supervised learning method for training to achieve efficient data classification. Grading.
For business scenarios that lack labeled data but have a large amount of unlabeled data, you can choose an unsupervised learning method for training, and classify and classify based on the characteristics and structure of the data itself.
For business scenarios with both a small amount of labeled data and a large amount of unlabeled data, you can choose a semi-supervised learning method for training, making full use of labeled data and unlabeled data to achieve intelligence Classification and grading.
For data classification and classification requirements in specific business fields, you can choose targeted AI training methods for training, such as text classification models in the field of natural language processing and image classification models in the field of computer vision. wait.
5. Cooperation between AI and humans
Although AI plays an important role in data classification and grading, AI cannot completely replace humans. Classification and grading. Human expertise and experience remain irreplaceable in some situations. Therefore, the cooperation between AI and humans is crucial to achieve efficient data classification and classification. Here are some ways in which AI and humans collaborate in data classification and grading:
Human experts participate in labeling data: In supervised learning , human experts can participate in labeling data and provide high-quality labeled samples, thereby improving the training effect of the model.
Manual review and adjustment results: After the AI model is classified and graded, humans can review and adjust the results and correct the model if possible existing errors to improve the accuracy of classification and grading.
Continuous optimization model: As business needs and data characteristics change, AI models need to be continuously optimized and updated. Humans can adjust and optimize the model based on actual conditions to better adapt to business scenarios.
3. Conclusion
Data classification and grading are an important part of data management and analysis, and are of great significance to the development of enterprises. By selecting appropriate AI training methods to match business scenarios, and combining human professional knowledge and experience, intelligent classification and classification of data can be achieved, and data security, utilization and management efficiency can be improved, thereby providing strong support for the development of enterprises. .
The above is the detailed content of AI-assisted data classification and classification. For more information, please follow other related articles on the PHP Chinese website!
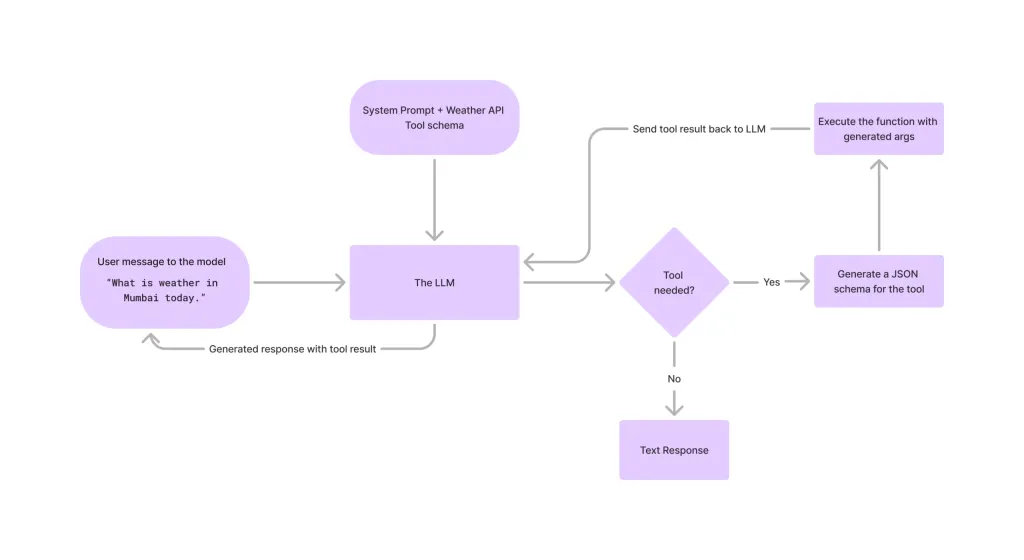
Large language models (LLMs) have surged in popularity, with the tool-calling feature dramatically expanding their capabilities beyond simple text generation. Now, LLMs can handle complex automation tasks such as dynamic UI creation and autonomous a
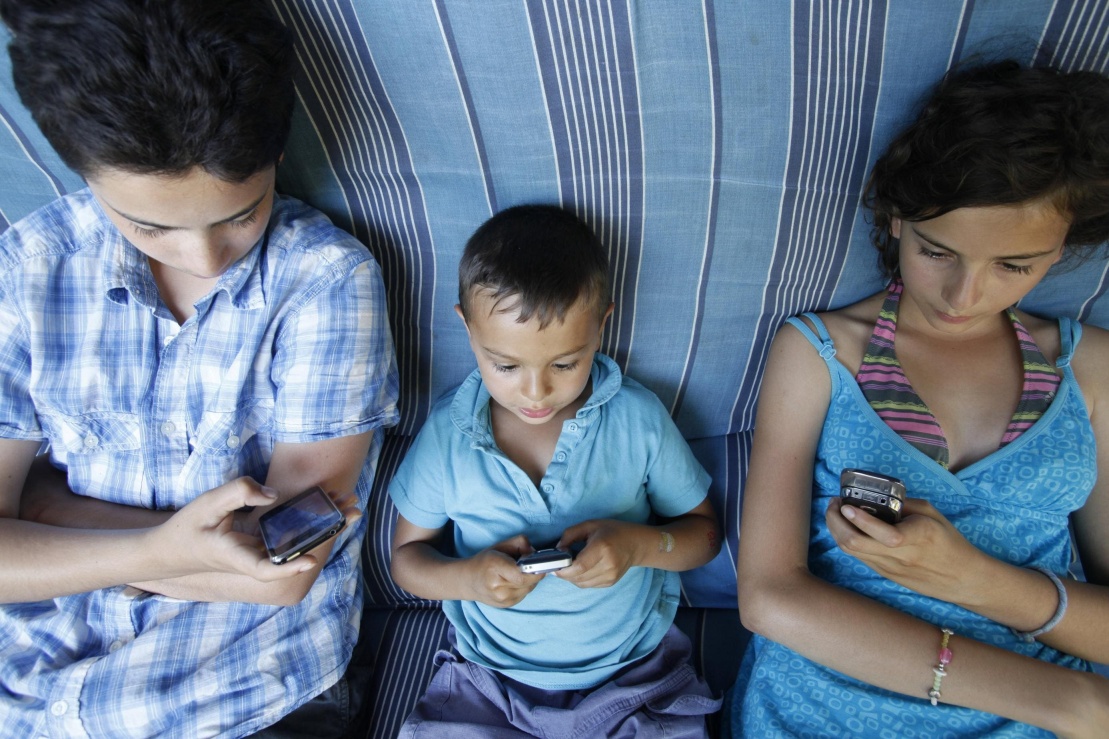
Can a video game ease anxiety, build focus, or support a child with ADHD? As healthcare challenges surge globally — especially among youth — innovators are turning to an unlikely tool: video games. Now one of the world’s largest entertainment indus
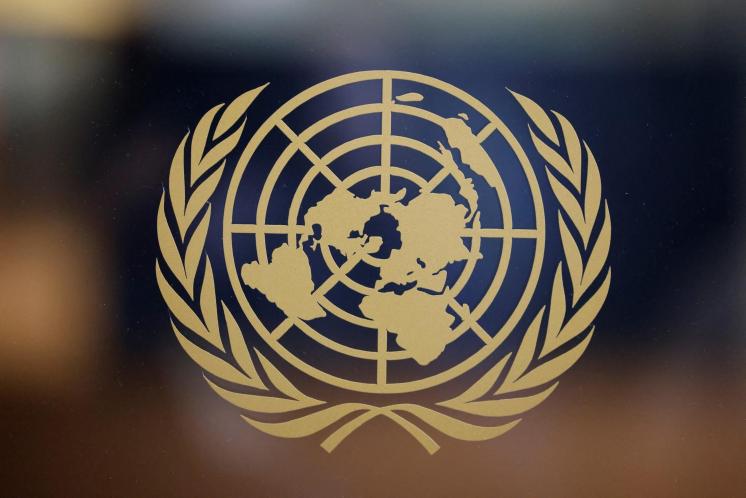
“History has shown that while technological progress drives economic growth, it does not on its own ensure equitable income distribution or promote inclusive human development,” writes Rebeca Grynspan, Secretary-General of UNCTAD, in the preamble.
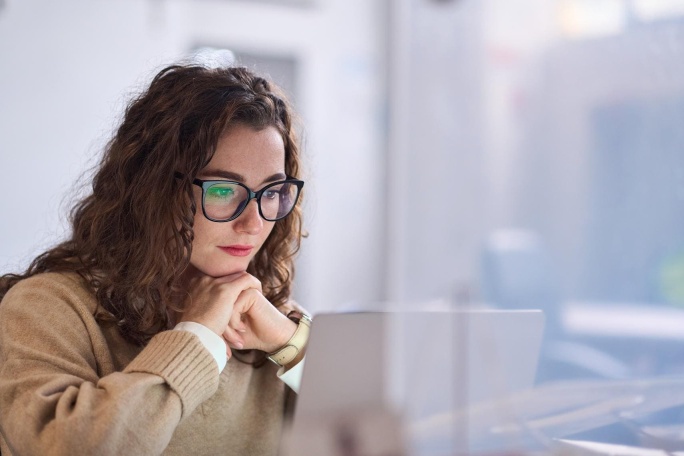
Easy-peasy, use generative AI as your negotiation tutor and sparring partner. Let’s talk about it. This analysis of an innovative AI breakthrough is part of my ongoing Forbes column coverage on the latest in AI, including identifying and explaining
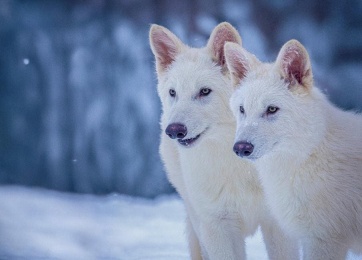
The TED2025 Conference, held in Vancouver, wrapped its 36th edition yesterday, April 11. It featured 80 speakers from more than 60 countries, including Sam Altman, Eric Schmidt, and Palmer Luckey. TED’s theme, “humanity reimagined,” was tailor made
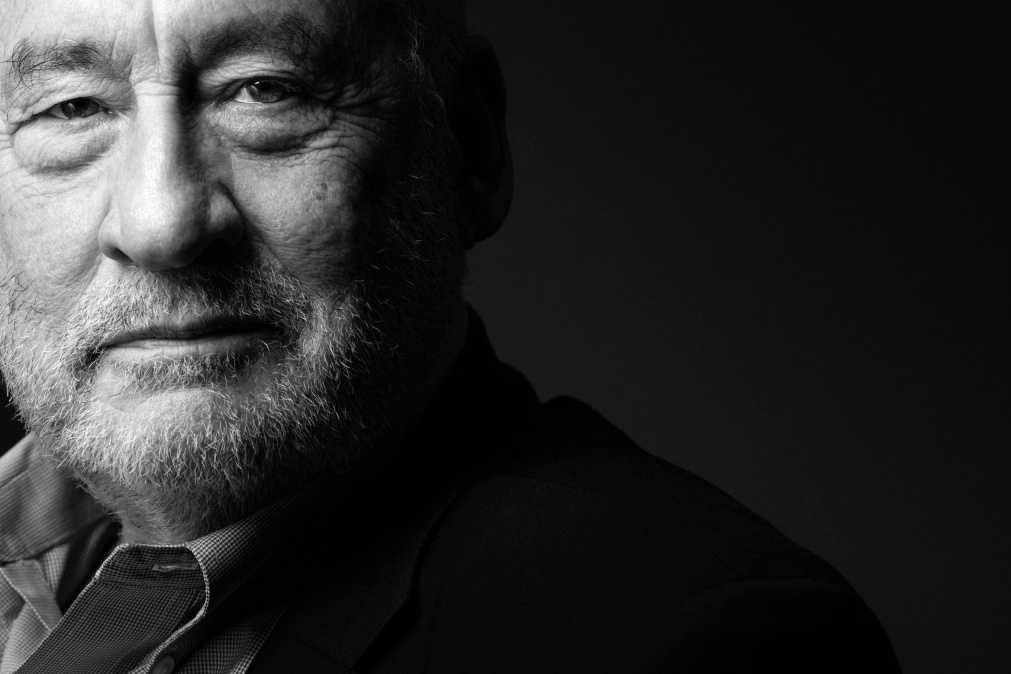
Joseph Stiglitz is renowned economist and recipient of the Nobel Prize in Economics in 2001. Stiglitz posits that AI can worsen existing inequalities and consolidated power in the hands of a few dominant corporations, ultimately undermining economic
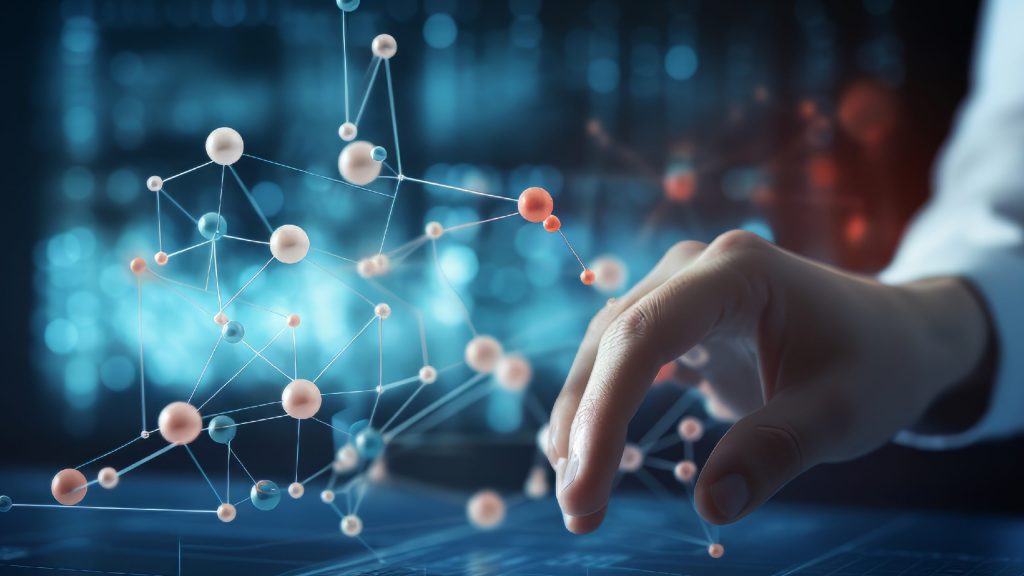
Graph Databases: Revolutionizing Data Management Through Relationships As data expands and its characteristics evolve across various fields, graph databases are emerging as transformative solutions for managing interconnected data. Unlike traditional
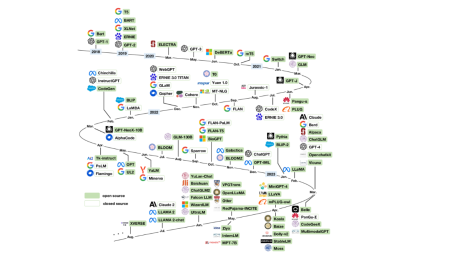
Large Language Model (LLM) Routing: Optimizing Performance Through Intelligent Task Distribution The rapidly evolving landscape of LLMs presents a diverse range of models, each with unique strengths and weaknesses. Some excel at creative content gen


Hot AI Tools

Undresser.AI Undress
AI-powered app for creating realistic nude photos

AI Clothes Remover
Online AI tool for removing clothes from photos.

Undress AI Tool
Undress images for free

Clothoff.io
AI clothes remover

AI Hentai Generator
Generate AI Hentai for free.

Hot Article

Hot Tools
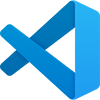
VSCode Windows 64-bit Download
A free and powerful IDE editor launched by Microsoft
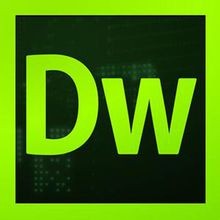
Dreamweaver CS6
Visual web development tools
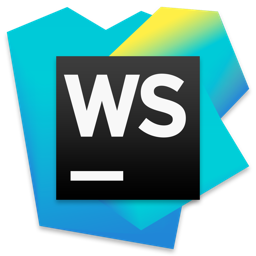
WebStorm Mac version
Useful JavaScript development tools

Safe Exam Browser
Safe Exam Browser is a secure browser environment for taking online exams securely. This software turns any computer into a secure workstation. It controls access to any utility and prevents students from using unauthorized resources.

Zend Studio 13.0.1
Powerful PHP integrated development environment