


CLIP long text capability is unlocked, and the performance of image retrieval tasks is significantly improved!
Some key details can also be captured. Shanghai Jiao Tong University and Shanghai AI Laboratory proposed a new framework Long-CLIP.
△The brown text is the key detail that distinguishes the two images
Long-CLIP is based on maintaining the original feature space of CLIP, in the downstream such as image generation Plug and play in the task to achieve fine-grained image generation of long text.
Long text-image retrieval increased by 20%, short text-image retrieval increased by 6%.
Unlocking CLIP long text capabilities
CLIP aligns visual and text modalities and has powerful zero-shot generalization capabilities. Therefore, CLIP is widely used in various multi-modal tasks, such as image classification, text image retrieval, image generation, etc.
But a major drawback of CLIP is the lack of long text capabilities.
First of all, due to the use of absolute position encoding, the text input length of CLIP is limited to 677 tokens. Not only that, experiments have proven that the real effective length of CLIP is even less than 20 tokens, which is far from enough to represent fine-grained information. However, to overcome this limitation, researchers have proposed a solution. By introducing specific tags in the text input, the model can focus on the important parts. The position and number of these tokens in the input are determined in advance and will not exceed 20 tokens. In this way, CLIP is able to
Long text missing on the text side also limits the capabilities of the visual side when processing text input. Since it only contains short text, CLIP's visual encoder will only extract the most important components of an image, while ignoring various details. This is very detrimental to fine-grained tasks such as cross-modal retrieval.
At the same time, the lack of long text also makes CLIP adopt a simple modeling method similar to bag-of-feature (BOF), which does not have complex capabilities such as causal reasoning.
In response to this problem, researchers proposed the Long-CLIP model.
Specifically proposed two major strategies: Knowledge-Preserving Stretching of Positional Embedding (Knowledge-Preserving Stretching of Positional Embedding) and fine-tuning strategy of adding core component alignment (Primary Component Matching).
Knowledge-preserving positional encoding expansion
A simple method to extend the input length and enhance long text capabilities is to first interpolate the positional encoding at a fixed ratio λ1 , and then fine-tune it with long text.
Researchers found that the training degree of different position encodings of CLIP is different. Since the training text is likely to be mainly short text, the lower position coding is more fully trained and can accurately represent the absolute position, while the higher position coding can only represent its approximate relative position. Therefore, the cost of interpolating codes at different positions is different.
Based on the above observations, the researcher retained the first 20 position codes, and for the remaining 57 position codes, interpolated with a larger ratio λ2 , the calculation formula It can be expressed as:
Experiments show that compared with direct interpolation, this strategy can significantly improve the performance on various tasks while supporting a longer total length.
Add fine-tuning of core attribute alignment
Merely introducing long-text fine-tuning will lead the model into another misunderstanding, that is, including all details equally. To address this problem, researchers introduced the strategy of core attribute alignment in fine-tuning.
Specifically, researchers use the principal component analysis (PCA) algorithm to extract core attributes from fine-grained image features, filter the remaining attributes and reconstruct coarse-grained image features, and combine them with generalized of short text. This strategy requires that the model not only contains more details (fine-grained alignment), but also identifies and models the most core attributes (core component extraction and coarse-grained alignment).
△Add the fine-tuning process of core attribute alignment
Plug and play in various multi-modal tasks
In pictures and texts In fields such as retrieval and image generation, Long-CLIP can replace CLIP plug-and-play.
For example, in image and text retrieval, Long-CLIP can capture more fine-grained information in image and text modes, thereby enhancing the ability to distinguish similar images and text, and greatly improving the performance of image and text retrieval.
Whether it is in traditional short text retrieval (COCO, Flickr30k) or long text retrieval tasks, Long-CLIP has significantly improved the recall rate.
△Short text-image retrieval experimental results
Paper link: https://arxiv.org/abs/2403.15378
Code link: https://github.com/beichenzbc/Long-CLIP
The above is the detailed content of Shanghai Jiao Tong University's new framework unlocks CLIP long text capabilities, grasps the details of multi-modal generation, and significantly improves image retrieval capabilities. For more information, please follow other related articles on the PHP Chinese website!
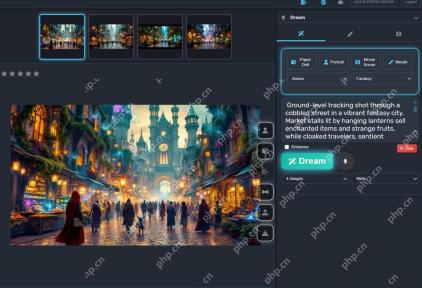
Upheaval Games: Revolutionizing Game Development with AI Agents Upheaval, a game development studio comprised of veterans from industry giants like Blizzard and Obsidian, is poised to revolutionize game creation with its innovative AI-powered platfor
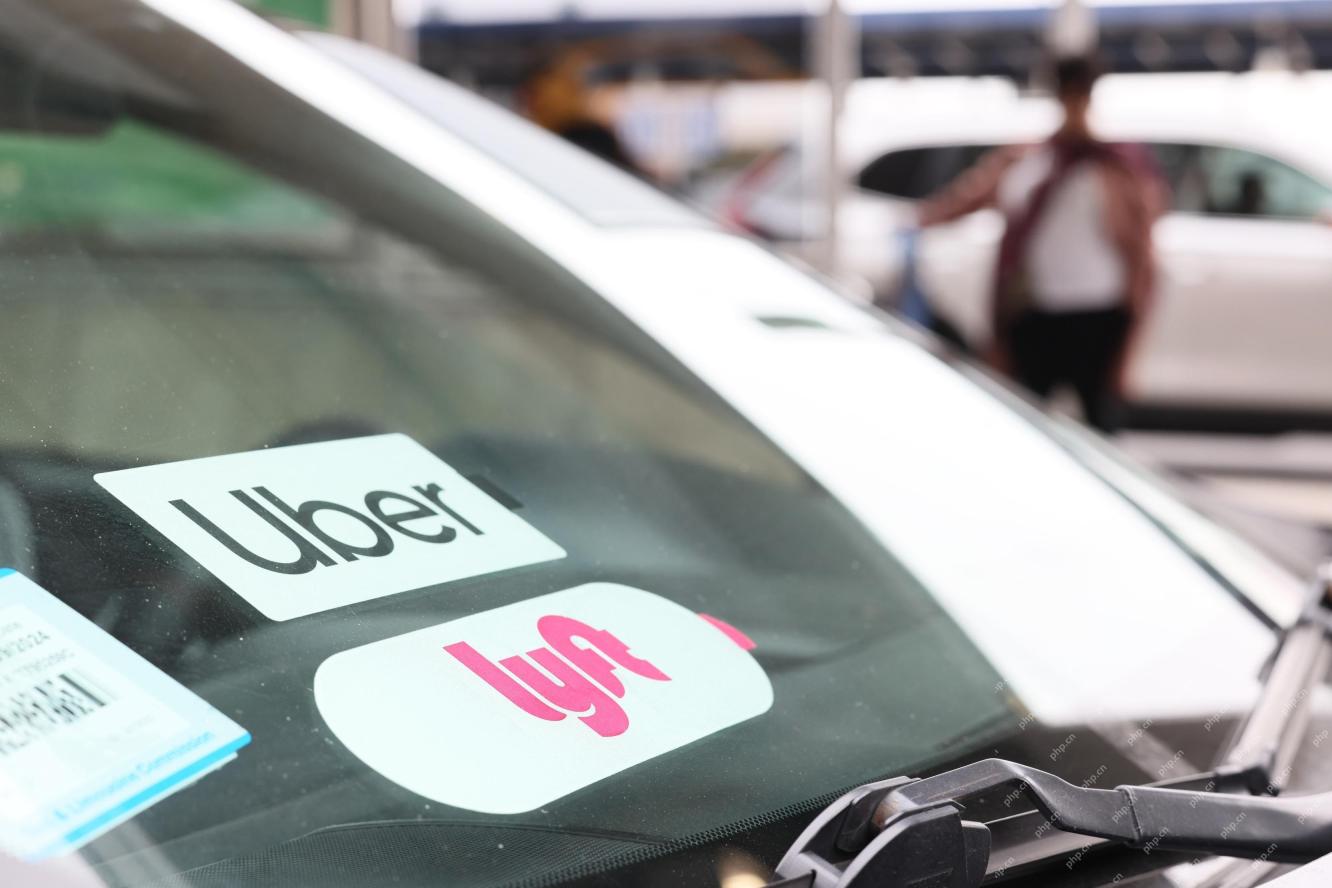
Uber's RoboTaxi Strategy: A Ride-Hail Ecosystem for Autonomous Vehicles At the recent Curbivore conference, Uber's Richard Willder unveiled their strategy to become the ride-hail platform for robotaxi providers. Leveraging their dominant position in
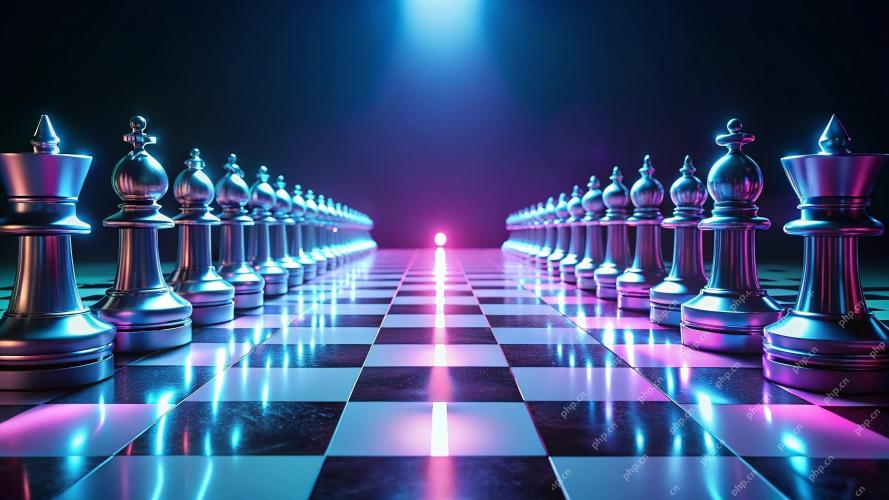
Video games are proving to be invaluable testing grounds for cutting-edge AI research, particularly in the development of autonomous agents and real-world robots, even potentially contributing to the quest for Artificial General Intelligence (AGI). A
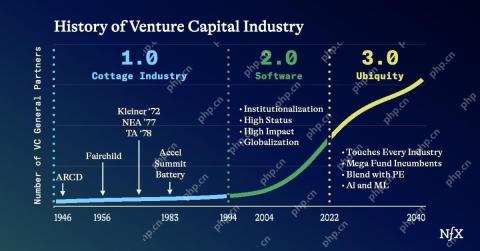
The impact of the evolving venture capital landscape is evident in the media, financial reports, and everyday conversations. However, the specific consequences for investors, startups, and funds are often overlooked. Venture Capital 3.0: A Paradigm
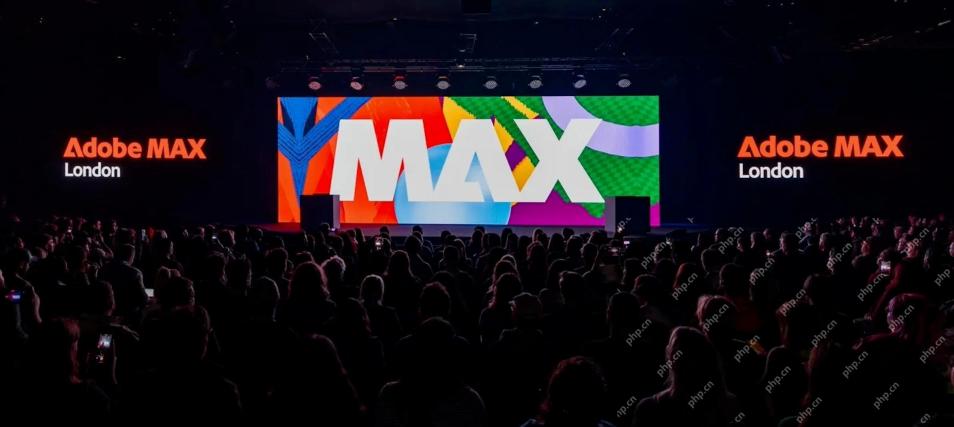
Adobe MAX London 2025 delivered significant updates to Creative Cloud and Firefly, reflecting a strategic shift towards accessibility and generative AI. This analysis incorporates insights from pre-event briefings with Adobe leadership. (Note: Adob
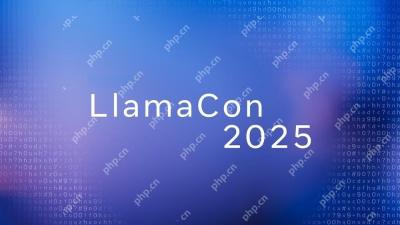
Meta's LlamaCon announcements showcase a comprehensive AI strategy designed to compete directly with closed AI systems like OpenAI's, while simultaneously creating new revenue streams for its open-source models. This multifaceted approach targets bo
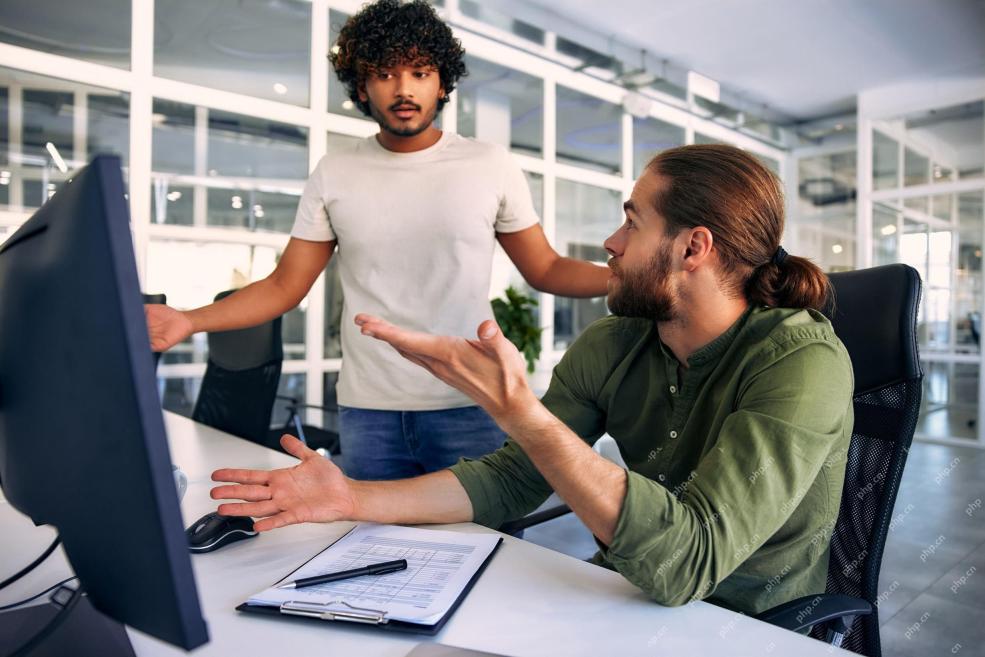
There are serious differences in the field of artificial intelligence on this conclusion. Some insist that it is time to expose the "emperor's new clothes", while others strongly oppose the idea that artificial intelligence is just ordinary technology. Let's discuss it. An analysis of this innovative AI breakthrough is part of my ongoing Forbes column that covers the latest advancements in the field of AI, including identifying and explaining a variety of influential AI complexities (click here to view the link). Artificial intelligence as a common technology First, some basic knowledge is needed to lay the foundation for this important discussion. There is currently a large amount of research dedicated to further developing artificial intelligence. The overall goal is to achieve artificial general intelligence (AGI) and even possible artificial super intelligence (AS)
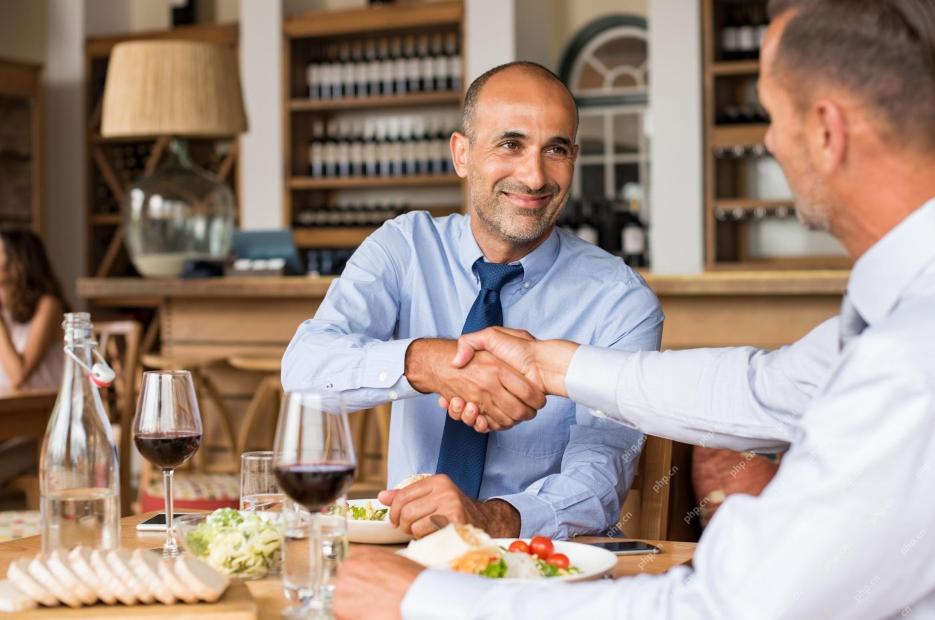
The effectiveness of a company's AI model is now a key performance indicator. Since the AI boom, generative AI has been used for everything from composing birthday invitations to writing software code. This has led to a proliferation of language mod


Hot AI Tools

Undresser.AI Undress
AI-powered app for creating realistic nude photos

AI Clothes Remover
Online AI tool for removing clothes from photos.

Undress AI Tool
Undress images for free

Clothoff.io
AI clothes remover

Video Face Swap
Swap faces in any video effortlessly with our completely free AI face swap tool!

Hot Article

Hot Tools

DVWA
Damn Vulnerable Web App (DVWA) is a PHP/MySQL web application that is very vulnerable. Its main goals are to be an aid for security professionals to test their skills and tools in a legal environment, to help web developers better understand the process of securing web applications, and to help teachers/students teach/learn in a classroom environment Web application security. The goal of DVWA is to practice some of the most common web vulnerabilities through a simple and straightforward interface, with varying degrees of difficulty. Please note that this software

Atom editor mac version download
The most popular open source editor
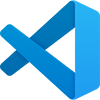
VSCode Windows 64-bit Download
A free and powerful IDE editor launched by Microsoft
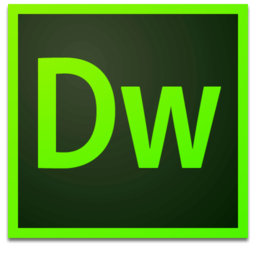
Dreamweaver Mac version
Visual web development tools
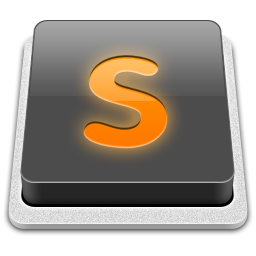
SublimeText3 Mac version
God-level code editing software (SublimeText3)
