


LLM performance is improved by up to 60%! Google ICLR 2024 masterpiece: Let the large language model learn the 'language of graphs'
In the field of computer science, a graph structure consists of nodes (representing entities) and edges (representing relationships between entities).
Pictures are everywhere.
The Internet can be regarded as a huge network, and search engines use graphical methods to organize and display information.
LLMs are mainly trained on regular text, so converting graphs into text understandable by LLMs is a challenging task because graph structures are fundamentally different from text.
At ICLR 2024, a team from Google explored how to transform graph data into a form suitable for LLMs to understand.
Paper address: https://openreview.net/pdf?id=IuXR1CCrSi
The process of encoding graphs into text using two different methods and feeding text and questions back to LLM
They also developed a tool called GraphQA A benchmark that explores approaches to solving different graph reasoning problems and shows how to formulate these problems in a way that is conducive to LLM solving graph-related problems.
Using the correct method, LLMs can improve the performance of graphics tasks by up to 60%.
GraphOA: An "exam" for LLMs
First, the Google team designed the GraphQA benchmark, which can be viewed as An exam designed to assess LLM's ability to address specific graphics problems.
GraphOA ensures diversity in breadth and number of connections by using multiple types of graphs to find possible biases in LLMs when processing graphs and make the entire process closer to LLMs situations that may be encountered in practical applications.
A framework for inference on LLMs using GraphIQA
Although the task is simple, such as checking whether an edge existence, counting the number of nodes or edges, etc., but these tasks require LLMs to understand the relationship between nodes and edges, which is crucial for more complex graph reasoning.
At the same time, the team also explored how to convert graphs into text that LLMs can process, such as solving the following two key issues:
Node encoding: How do we represent a single node? Nodes can include simple integers, common names (people, characters), and letters.
Edge Coding: How do we describe the relationships between nodes? Methods can include parentheses, phrases (such as "are friends"), and symbolic representations (such as arrows).
In the end, the researchers systematically combined various node and edge encoding methods to produce functions like those shown in the figure below.
Example of graphical encoding function
How do LLMs perform?
The research team conducted three key experiments on GraphOA:
- Testing the ability of LLMs to handle graphics tasks
- Test the impact of the size of LLMs on performance
- Test the impact of different graph shapes on performance
In Chapter In one experiment, LLMs performed mediocrely. On most basic tasks, LLMs performed no better than random guessing.
But the encoding method significantly affects the results. As shown in the figure below, in most cases, "incident" encoding performs well in most tasks. Choosing the appropriate encoding function can greatly improve the accuracy of the task.
Comparison of various graph encoder functions based on different task accuracy
In the second test, the researchers tested the same on different sized models graphics tasks.
In terms of conclusion, in graphical inference tasks, larger models perform better,
However, interestingly, in In the "edge existence" task (determining whether two nodes in the graph are connected), scale is not as important as in other tasks.
Even the largest LLM cannot always beat simple baseline solutions on the cycle checking problem (determining whether a cycle exists in the graph). This shows that LLMs still have room for improvement on certain graph tasks.
The impact of model capacity on graph reasoning tasks of PaLM 2-XXS, XS, S and L
In the third test, the researchers used GraphOA to generate graphs with different structures for analysis as to whether the graph structure would affect the ability of LMMs to solve problems.
# Examples of graphs generated by GraphQA’s different graph generators. ER, BA, SBM and SFN are Erdős-Rényi, Barabási-Albert, stochastic block model and scale-free network respectively.
The results show that the structure of the graph has a great impact on the performance of LLMs.
For example, in a task asking whether cycles exist, LLMs perform well in closely connected graphs (where cycles are common) but not in path graphs (where cycles are common). never happens).
But at the same time, providing some mixed samples helps LLMs adapt. For example, in the cycle detection task, the researchers added some examples that contain cycles and some that do not contain cycles in the prompts as less Examples of sample learning improve the performance of LLMs in this way.
Compare different graph generators on different graph tasks. The main observation is that graph structure has a significant impact on the performance of LLM. ER, BA, SBM and SFN refer to Erdős-Rényi, Barabási-Albert, stochastic block model and scale-free network respectively.
This is just the beginning of letting LLMs understand graphs
In the paper, the Google team initially explored how to best represent graphs as text so that LLMs can understand them.
With the help of correct coding techniques, the accuracy of LLMs on graph problems is significantly improved (from about 5% to over 60% improvement).
At the same time, three main influencing factors were also determined, which are the encoding method of converting graphics into text, the task types of different graphics, and the density structure of graphics.
This is just the beginning for LLMs to understand graphs. With the help of the new benchmark GraphQA, we look forward to further research to explore more possibilities of LLMs.
The above is the detailed content of LLM performance is improved by up to 60%! Google ICLR 2024 masterpiece: Let the large language model learn the 'language of graphs'. For more information, please follow other related articles on the PHP Chinese website!
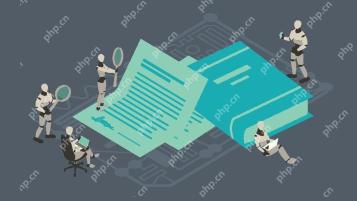
The legal tech revolution is gaining momentum, pushing legal professionals to actively embrace AI solutions. Passive resistance is no longer a viable option for those aiming to stay competitive. Why is Technology Adoption Crucial? Legal professional
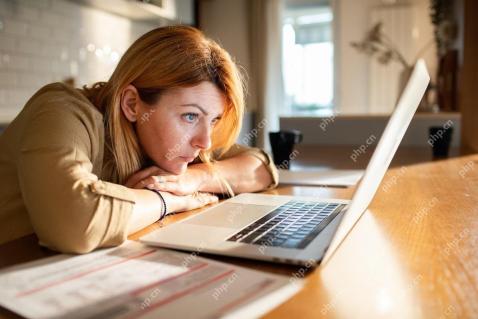
Many assume interactions with AI are anonymous, a stark contrast to human communication. However, AI actively profiles users during every chat. Every prompt, every word, is analyzed and categorized. Let's explore this critical aspect of the AI revo
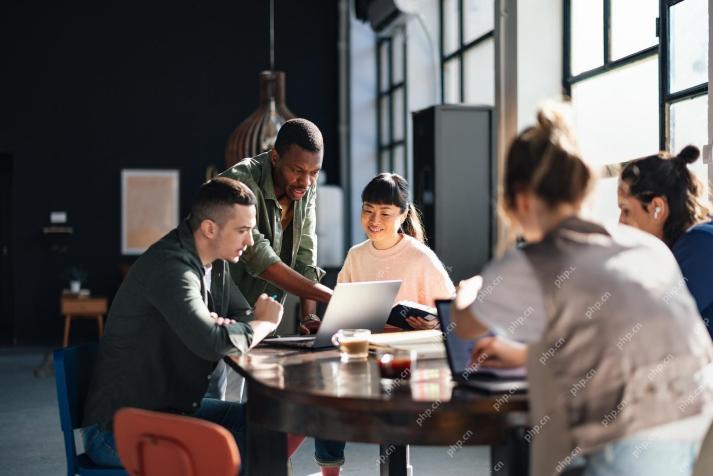
A successful artificial intelligence strategy cannot be separated from strong corporate culture support. As Peter Drucker said, business operations depend on people, and so does the success of artificial intelligence. For organizations that actively embrace artificial intelligence, building a corporate culture that adapts to AI is crucial, and it even determines the success or failure of AI strategies. West Monroe recently released a practical guide to building a thriving AI-friendly corporate culture, and here are some key points: 1. Clarify the success model of AI: First of all, we must have a clear vision of how AI can empower business. An ideal AI operation culture can achieve a natural integration of work processes between humans and AI systems. AI is good at certain tasks, while humans are good at creativity and judgment
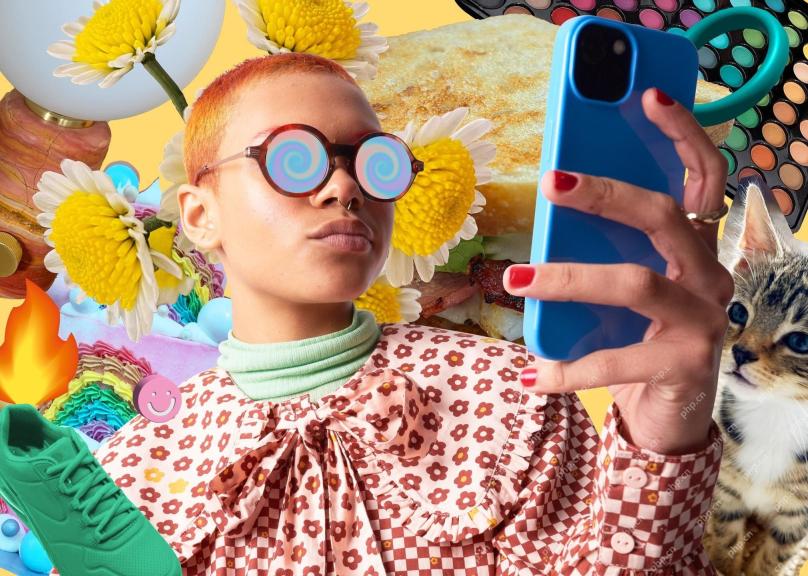
Meta upgrades AI assistant application, and the era of wearable AI is coming! The app, designed to compete with ChatGPT, offers standard AI features such as text, voice interaction, image generation and web search, but has now added geolocation capabilities for the first time. This means that Meta AI knows where you are and what you are viewing when answering your question. It uses your interests, location, profile and activity information to provide the latest situational information that was not possible before. The app also supports real-time translation, which completely changed the AI experience on Ray-Ban glasses and greatly improved its usefulness. The imposition of tariffs on foreign films is a naked exercise of power over the media and culture. If implemented, this will accelerate toward AI and virtual production
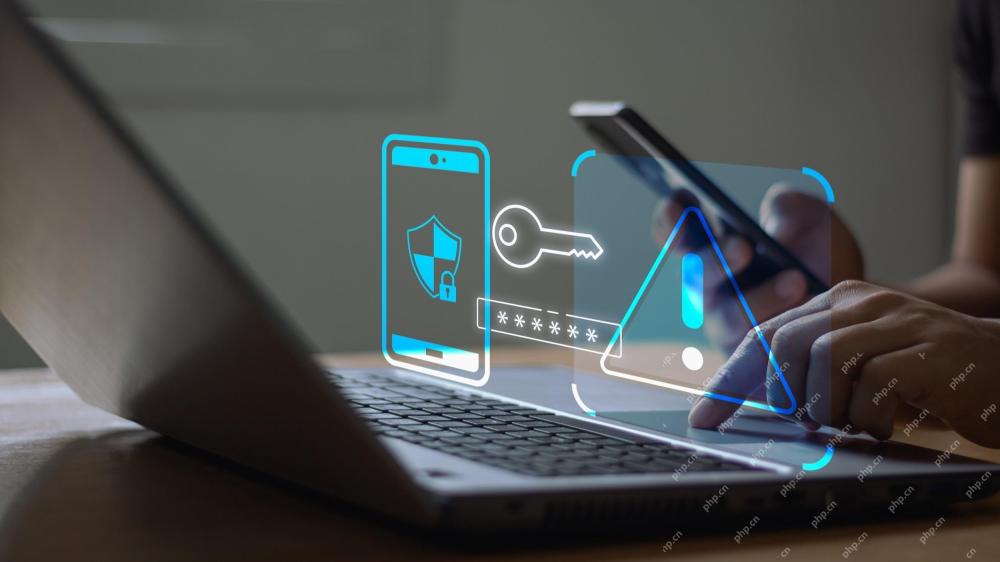
Artificial intelligence is revolutionizing the field of cybercrime, which forces us to learn new defensive skills. Cyber criminals are increasingly using powerful artificial intelligence technologies such as deep forgery and intelligent cyberattacks to fraud and destruction at an unprecedented scale. It is reported that 87% of global businesses have been targeted for AI cybercrime over the past year. So, how can we avoid becoming victims of this wave of smart crimes? Let’s explore how to identify risks and take protective measures at the individual and organizational level. How cybercriminals use artificial intelligence As technology advances, criminals are constantly looking for new ways to attack individuals, businesses and governments. The widespread use of artificial intelligence may be the latest aspect, but its potential harm is unprecedented. In particular, artificial intelligence
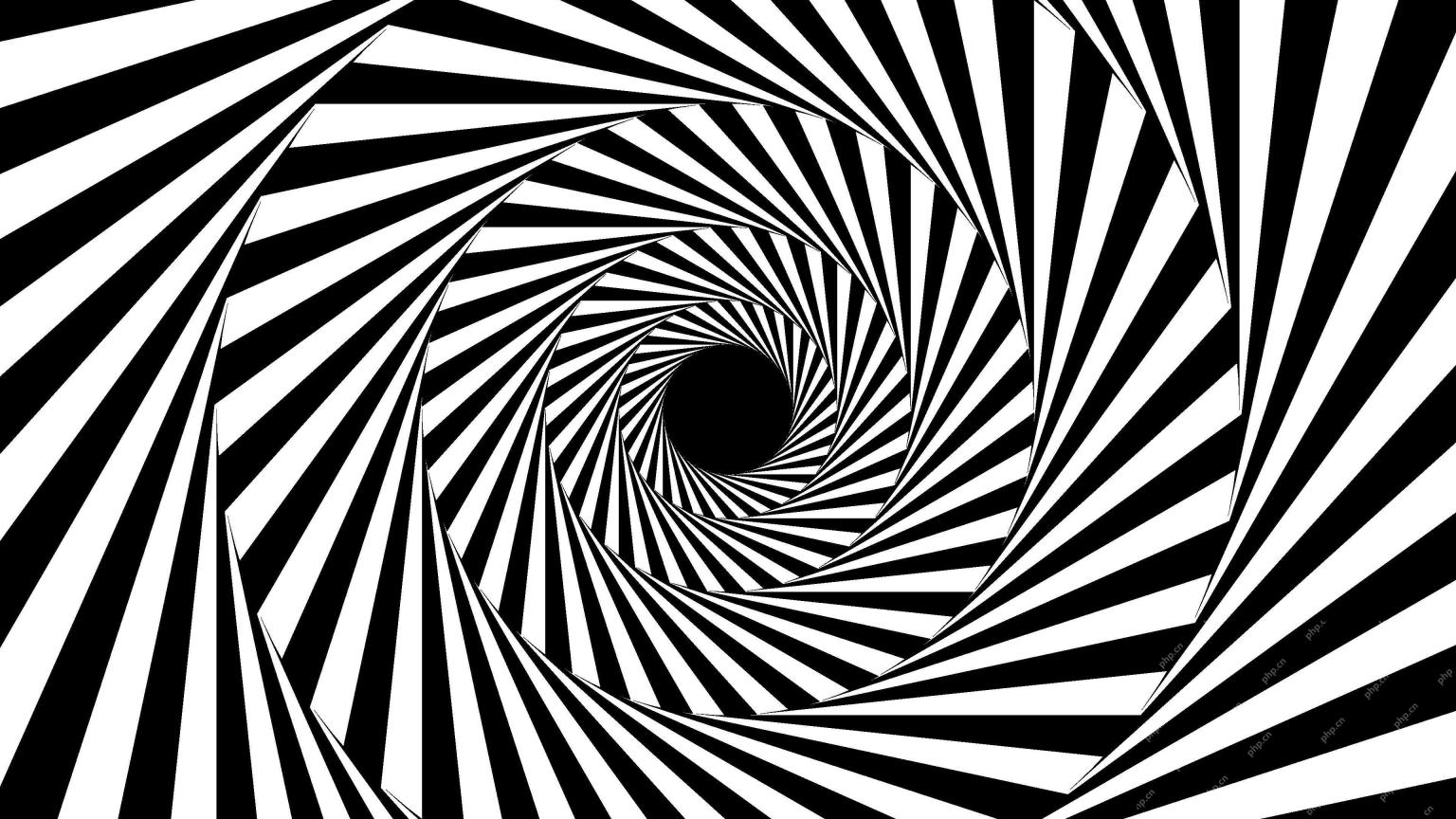
The intricate relationship between artificial intelligence (AI) and human intelligence (NI) is best understood as a feedback loop. Humans create AI, training it on data generated by human activity to enhance or replicate human capabilities. This AI
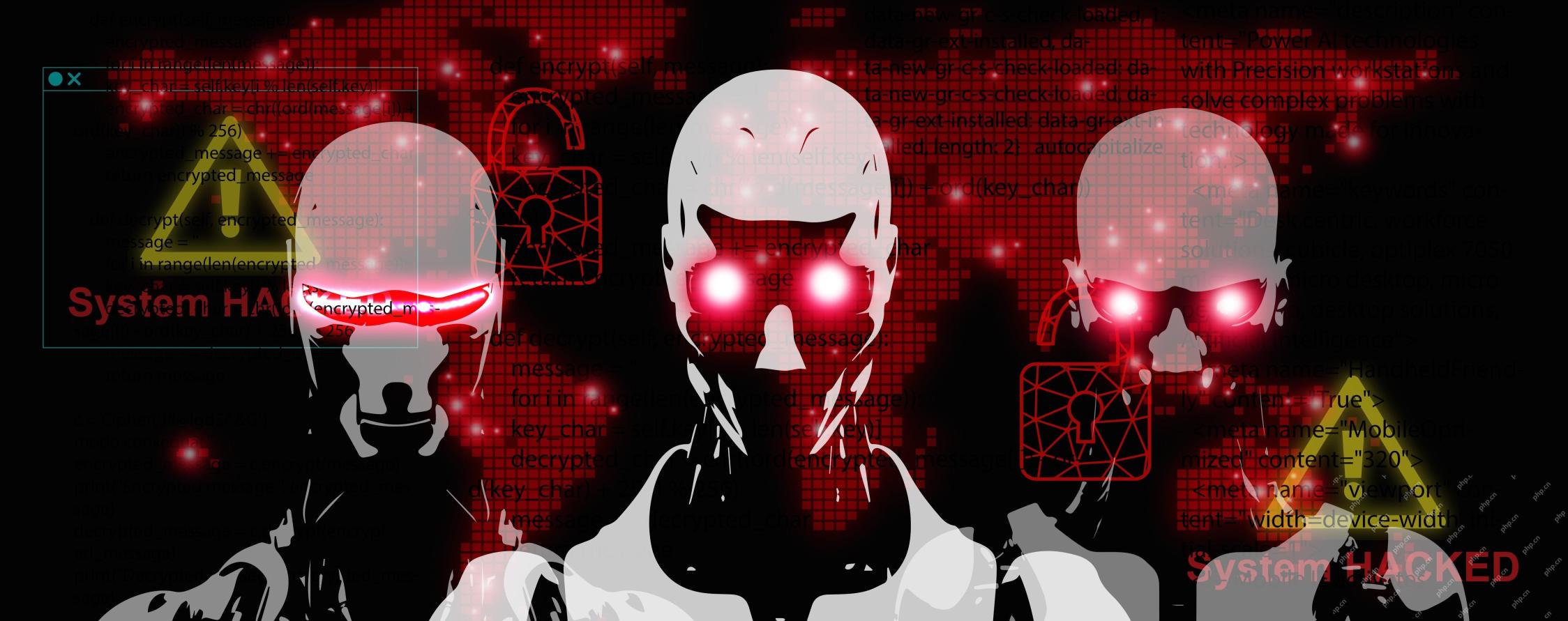
Anthropic's recent statement, highlighting the lack of understanding surrounding cutting-edge AI models, has sparked a heated debate among experts. Is this opacity a genuine technological crisis, or simply a temporary hurdle on the path to more soph
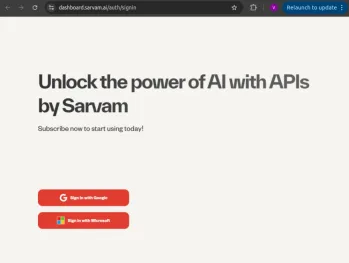
India is a diverse country with a rich tapestry of languages, making seamless communication across regions a persistent challenge. However, Sarvam’s Bulbul-V2 is helping to bridge this gap with its advanced text-to-speech (TTS) t


Hot AI Tools

Undresser.AI Undress
AI-powered app for creating realistic nude photos

AI Clothes Remover
Online AI tool for removing clothes from photos.

Undress AI Tool
Undress images for free

Clothoff.io
AI clothes remover

Video Face Swap
Swap faces in any video effortlessly with our completely free AI face swap tool!

Hot Article

Hot Tools

Safe Exam Browser
Safe Exam Browser is a secure browser environment for taking online exams securely. This software turns any computer into a secure workstation. It controls access to any utility and prevents students from using unauthorized resources.
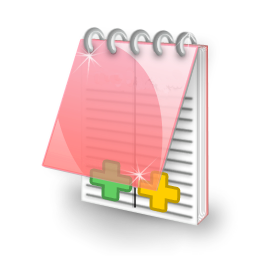
EditPlus Chinese cracked version
Small size, syntax highlighting, does not support code prompt function
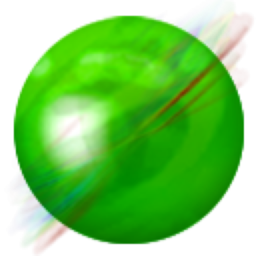
ZendStudio 13.5.1 Mac
Powerful PHP integrated development environment

PhpStorm Mac version
The latest (2018.2.1) professional PHP integrated development tool
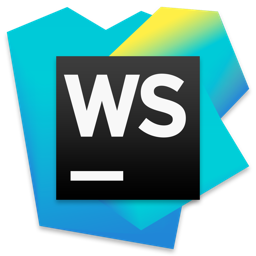
WebStorm Mac version
Useful JavaScript development tools
