Implement robust AI governance to democratize data
The emergence of GenAI has accelerated the pace of unlocking the potential of data, providing opportunities for new insights and better decisions. However, achieving broader data access requires a comprehensive data governance strategy. Those enterprises that can strike a balance between data democratization and rigorous data governance will differentiate themselves in the market by unlocking unique data-driven insights.
According to Gartner, more than 80% of enterprises will use GenAI APIs and models or deploy GenAI-enabled applications in production by 2026, up from less than 5% last year. GenAI's natural language interface allows non-technical users, from department heads to frontline workers, to more easily access and use data. This levels the playing field in access to information and skills, which Gartner calls “one of the most disruptive trends of this decade.”
If companies are to avoid increased risks to privacy, security and data quality, democratizing data in this way makes strong governance even more critical, which means knowing exactly what you have Data, where it resides, who has access to it and how each type of user is allowed to use it, but how does a business enforce total control without stifling innovation?
At a higher level, the favored approach is to consolidate data into a comprehensive repository that can be shared easily and securely among different teams and workgroups. By unifying data, enterprises can centralize management and expand access to data while minimizing complexity and optimizing costs. This centralized approach to storing data helps ensure data consistency and accuracy and avoids problems caused by data duplication and inconsistency. Additionally, this also helps improve data security and protect privacy, as access control and monitoring measures can be more easily implemented. Therefore, it is very important for enterprises to establish a unified data repository.
In practice, this may bring some challenges because data sovereignty regulations require that certain data must be stored in specific country or region. Faced with this situation, enterprises need to work to eliminate data silos and implement a consistent governance framework across their data platforms.
In addition, some specific methods and technologies can help ensure that enterprises can maintain effective governance while maintaining security as GenAI expands access to data. These approaches include basic governance practices that apply across a variety of settings, but become especially critical as GenAI drives further democratization of data access.
Granular Controls for Privacy and Compliance
As employee access to data increases, so does the risk of data breaches and personally identifiable information (PII) being accessed by unauthorized users. . Therefore, implementing strict access control policies and using anonymization and identification technologies are critical to ensure compliance and protect data from inappropriate access.
In our new Data Trends 2024 report analyzing Snowflake Data Cloud trends, we noticed a significant increase in the use of governance capabilities that provide granular control over data while also appropriately Available to more users for more use cases, for example, usage of applied masks or row access policies increased 98% in the 12 months ended January 31, 2024 compared to the same period last year , at the same time, the number of columns assigned masking policies increased by 97%.
However, it is worth noting that the total number of queries run against policy-protected objects rose by 142%. This number is significant because it shows that good data governance is not about saying "no" and restricting data usage. Despite seeing an increase in governance through the use of labeling and blocking policies, the report notes that the amount of work being done using this data is rising rapidly.
In some cases, employees may wish to inspect a dataset to which they cannot be granted direct access. In such cases, differential privacy is a powerful technique as it allows users to view the dataset by schema to share and explore data sets without revealing any individual user’s PII. Taking this a step further, data clean rooms allow multiple parties to collaborate on data without disclosing the raw data to each other. Data clean rooms are typically used to share data between different businesses, but we are seeing the technology being used internally to meet growing demand. regulatory and privacy needs, it can be an effective technique for exploring PII data in the context of GenAI interfaces.
Consistent, coordinated security
Security should be built into the fabric of the data platform, rather than trying to fix it later for individual data sets and users, and the technology that supports conversational interfaces should not be replicated identity and other core permissions on data, which results in a fragile setup. If two or more systems are tracking who has access to which data, the potential for errors and unauthorized access increases significantly.
Technologies that play a key role in protecting data for GenAI use cases include continuous risk monitoring and protection, role-based access control (RBAC) and fine-grained authorization policies. Role-based tags and tag-based masking policies allow you to protect data at the column level by assigning a masking policy to a tag and then setting the tag on one or more database objects.
Data silos are the enemy of good governance
Storing copies or fragments of data in disparate systems makes it difficult to track who has access to what information and to maintain consistency in access and control policies Extremely difficult, which is why data silos are the enemy of strong governance.
Data silos also make it difficult to ensure that employees are querying the most current and accurate data, which can lead to costly mistakes. To achieve broad access to data through GenAI, enterprises need a single source of truth to ensure all employees are viewing the same information and that controls and policies can be applied and updated across all data.
Ensure data quality for accurate results
Even if you eliminate silos and have the appropriate permissions, there is no guarantee that the information your employees are accessing is correct. The data quality framework is based on applying to tables Configurable data quality rules for a specific column or set of columns to help detect quality issues and ensure accurate information.
Additionally, by now we all know that GenAI can sometimes hallucinate and produce answers that are actually unfounded, which is unacceptable for enterprise use. Enterprises can solve this problem by combining large language models (LLMs) with data sources they know they can trust, such as internal customer databases or vetted data sets from trusted third-party providers.
These trusted data sources can be merged using processes that require LLM customization (such as fine-tuning) or do not require LLM customization (such as just-in-time engineering or retrieval-augmented generation (RAG)). Whatever the case, these technologies help ensure employees receive accurate, high-quality results while adhering to the governance standards built into the on-premises cloud environment.
The power of data access and universal search
An important aspect of GenAI governance is making it easy for employees to find the right data sets and data products to help them with their analysis. One reason why artificial intelligence is so powerful is that It allows employees to interact with data without going through a central team, but this requires those employees to know what data is available to them and how to find it.
The search function provides this functionality, allowing users to find and query datasets and data products. This search function itself can be powered by LLM, making data search more intuitive - this is what we have developed at Snowflake, As part of our universal search.
Governance is the foundation of data democratization
Business users are eager to make wider use of their organization’s data, and GenAI finally makes this possible. Thanks to LLMS and natural language processing, employees in areas such as finance, HR, sales and operations can now formulate questions specific to their role and get the answers they need to make more informed decisions.
But to meet the security and compliance needs of the enterprise, this can only happen in an environment with strong governance. The stronger the governance, the more freely your employees can browse the data without giving the company Bringing additional risks, GenAI opens the door to true data democratization, and good governance is the foundation to make it possible.
The above is the detailed content of Implement robust AI governance to democratize data. For more information, please follow other related articles on the PHP Chinese website!
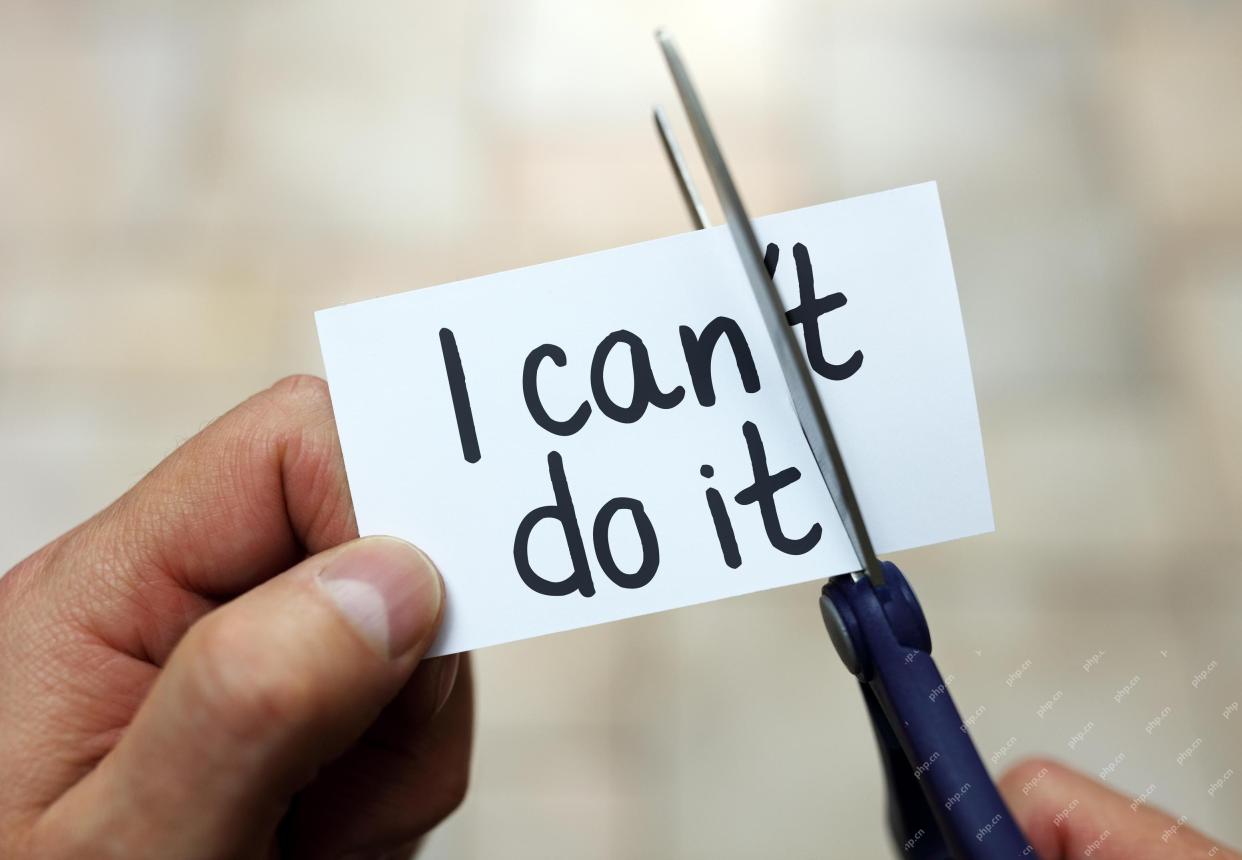
This article explores the growing concern of "AI agency decay"—the gradual decline in our ability to think and decide independently. This is especially crucial for business leaders navigating the increasingly automated world while retainin
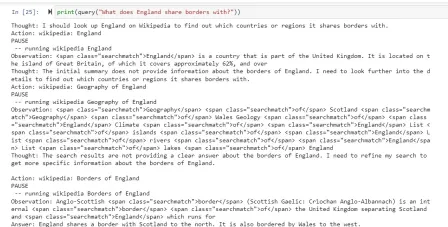
Ever wondered how AI agents like Siri and Alexa work? These intelligent systems are becoming more important in our daily lives. This article introduces the ReAct pattern, a method that enhances AI agents by combining reasoning an
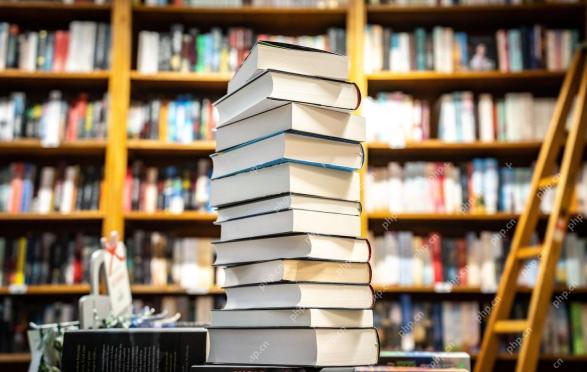
"I think AI tools are changing the learning opportunities for college students. We believe in developing students in core courses, but more and more people also want to get a perspective of computational and statistical thinking," said University of Chicago President Paul Alivisatos in an interview with Deloitte Nitin Mittal at the Davos Forum in January. He believes that people will have to become creators and co-creators of AI, which means that learning and other aspects need to adapt to some major changes. Digital intelligence and critical thinking Professor Alexa Joubin of George Washington University described artificial intelligence as a “heuristic tool” in the humanities and explores how it changes
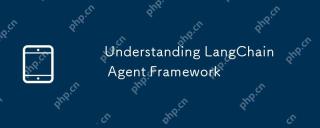
LangChain is a powerful toolkit for building sophisticated AI applications. Its agent architecture is particularly noteworthy, allowing developers to create intelligent systems capable of independent reasoning, decision-making, and action. This expl
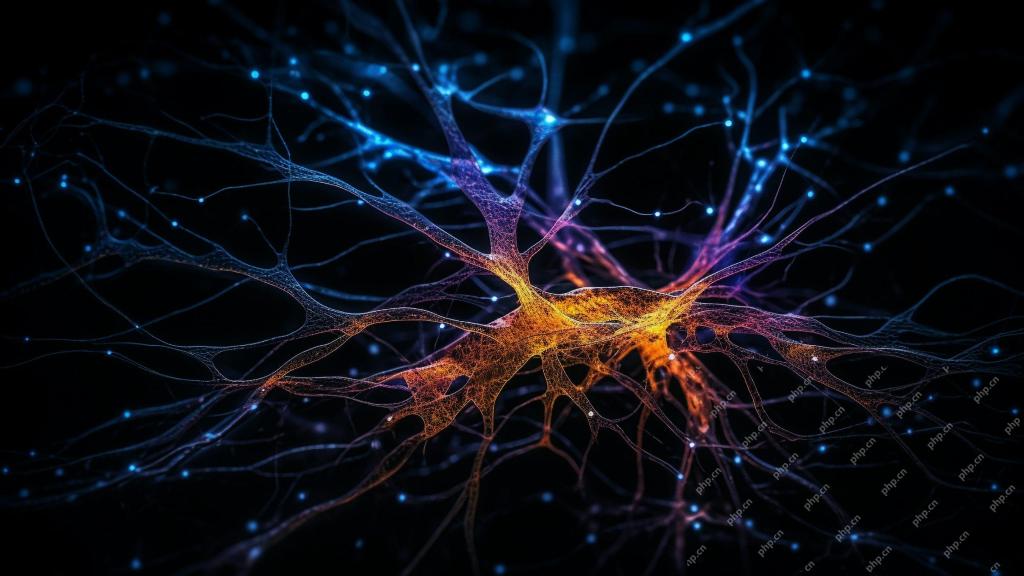
Radial Basis Function Neural Networks (RBFNNs): A Comprehensive Guide Radial Basis Function Neural Networks (RBFNNs) are a powerful type of neural network architecture that leverages radial basis functions for activation. Their unique structure make
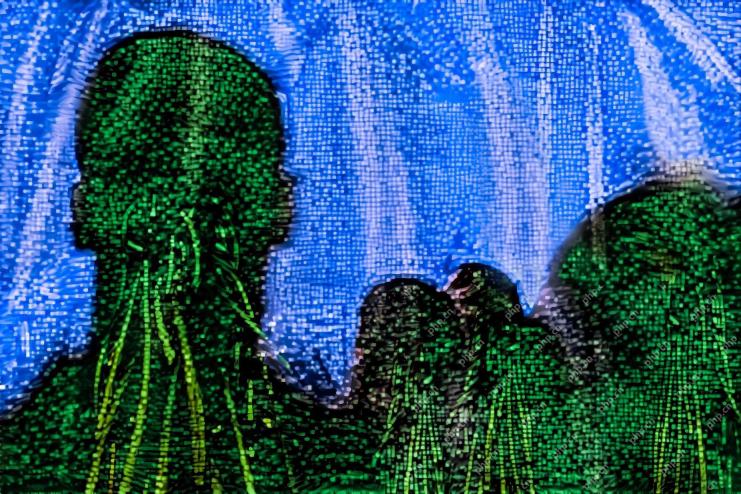
Brain-computer interfaces (BCIs) directly link the brain to external devices, translating brain impulses into actions without physical movement. This technology utilizes implanted sensors to capture brain signals, converting them into digital comman
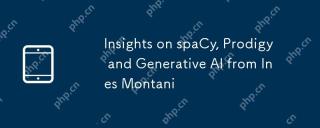
This "Leading with Data" episode features Ines Montani, co-founder and CEO of Explosion AI, and co-developer of spaCy and Prodigy. Ines offers expert insights into the evolution of these tools, Explosion's unique business model, and the tr
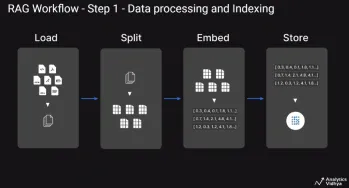
This article explores Retrieval Augmented Generation (RAG) systems and how AI agents can enhance their capabilities. Traditional RAG systems, while useful for leveraging custom enterprise data, suffer from limitations such as a lack of real-time dat


Hot AI Tools

Undresser.AI Undress
AI-powered app for creating realistic nude photos

AI Clothes Remover
Online AI tool for removing clothes from photos.

Undress AI Tool
Undress images for free

Clothoff.io
AI clothes remover

Video Face Swap
Swap faces in any video effortlessly with our completely free AI face swap tool!

Hot Article

Hot Tools
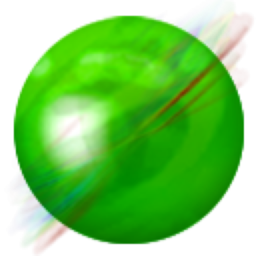
ZendStudio 13.5.1 Mac
Powerful PHP integrated development environment

mPDF
mPDF is a PHP library that can generate PDF files from UTF-8 encoded HTML. The original author, Ian Back, wrote mPDF to output PDF files "on the fly" from his website and handle different languages. It is slower than original scripts like HTML2FPDF and produces larger files when using Unicode fonts, but supports CSS styles etc. and has a lot of enhancements. Supports almost all languages, including RTL (Arabic and Hebrew) and CJK (Chinese, Japanese and Korean). Supports nested block-level elements (such as P, DIV),

Atom editor mac version download
The most popular open source editor
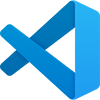
VSCode Windows 64-bit Download
A free and powerful IDE editor launched by Microsoft

Zend Studio 13.0.1
Powerful PHP integrated development environment