


Natural Language Processing Meets Python: An Algorithmic Journey
Natural Language Processing (NLP) is a branch of computer science that deals with how computers understand and generate human language. python is a popular programming language that provides a rich set of libraries and tools to simplify NLP tasks. This article will explore common algorithms used for NLP in Python, focusing on text classification, sentiment analysis, and machine translation.
Text Categorization
Text classification algorithms assign text documents to a set of predefined categories. In Python, perform text classification using the following algorithm:
- Naive Bayes: A probabilistic algorithm that assumes that features are independent of each other. It's simple and effective, especially useful for small data sets.
- Support Vector Machine (SVM): A classification algorithm that creates hyperplanes to separate different categories. SVM performs well in handling high-dimensional data.
- Random Forest: A decision tree-based algorithm that improves accuracy by classifying multiple trees and combining their predictions. Random forests are suitable for big data sets and can handle missing data.
Sentiment Analysis
Sentiment analysis algorithms determine the mood or emotion in text. In Python, popular algorithms for sentiment analysis include:
- Sentiment Analysis Dictionary: A vocabulary lookup-based approach that uses a predefined sentiment dictionary to map words to emotions. For example, "happy" and "satisfied" are classified as positive emotions, while "sadness" and "angry" are classified as negative emotions.
- Machine learning algorithms: Such as support vector machines and naive Bayes, models can be trained to predict emotions in text. These algorithms use training data sets with known emotion labels.
- Deep learning model: For example, convolutional Neural network (CNN), which can extract features of text and predict its emotion. Deep Learning Models perform well in processing large amounts of text data.
machine translation
Machine translation algorithms translate text from one language into another. In Python, algorithms used for machine translation include:
- Statistical Machine Translation (SMT): An algorithm based on statistical methods that uses large corpora to learn correspondences between languages. SMT excels at short sentences and phrases.
- Neural Machine Translation (NMT): An algorithm based on a neural network that takes an entire sentence as input and directly generates a translation output. NMT can outperform SMT in terms of quality and fluidity.
- Transformer: An NMT model that utilizes a self-attention mechanism to capture long-term dependencies in text. TransfORMer is particularly effective at handling long sentences and complex syntax.
in conclusion
Python provides a variety of algorithms for performing NLP tasks, including text classification, sentiment analysis, and machine translation. Naive Bayes, Support Vector Machines, and Random Forests are commonly used algorithms for text classification, while sentiment analysis dictionaries, Machine Learning algorithms, and deep learning models are used for sentiment analysis. Finally, Statistical Machine Translation, Neural Machine Translation and Transformer are used for machine translation. By leveraging these algorithms, we can create powerful NLP applications that understand and interact with human language.
The above is the detailed content of Natural Language Processing Meets Python: An Algorithmic Journey. For more information, please follow other related articles on the PHP Chinese website!
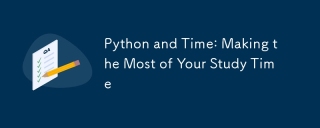
To maximize the efficiency of learning Python in a limited time, you can use Python's datetime, time, and schedule modules. 1. The datetime module is used to record and plan learning time. 2. The time module helps to set study and rest time. 3. The schedule module automatically arranges weekly learning tasks.
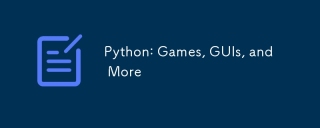
Python excels in gaming and GUI development. 1) Game development uses Pygame, providing drawing, audio and other functions, which are suitable for creating 2D games. 2) GUI development can choose Tkinter or PyQt. Tkinter is simple and easy to use, PyQt has rich functions and is suitable for professional development.
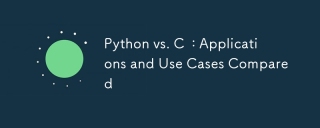
Python is suitable for data science, web development and automation tasks, while C is suitable for system programming, game development and embedded systems. Python is known for its simplicity and powerful ecosystem, while C is known for its high performance and underlying control capabilities.
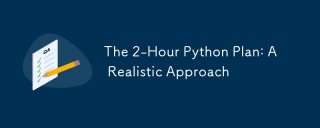
You can learn basic programming concepts and skills of Python within 2 hours. 1. Learn variables and data types, 2. Master control flow (conditional statements and loops), 3. Understand the definition and use of functions, 4. Quickly get started with Python programming through simple examples and code snippets.
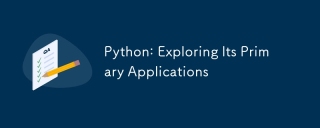
Python is widely used in the fields of web development, data science, machine learning, automation and scripting. 1) In web development, Django and Flask frameworks simplify the development process. 2) In the fields of data science and machine learning, NumPy, Pandas, Scikit-learn and TensorFlow libraries provide strong support. 3) In terms of automation and scripting, Python is suitable for tasks such as automated testing and system management.
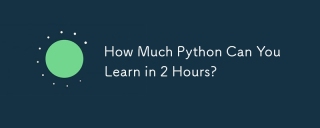
You can learn the basics of Python within two hours. 1. Learn variables and data types, 2. Master control structures such as if statements and loops, 3. Understand the definition and use of functions. These will help you start writing simple Python programs.
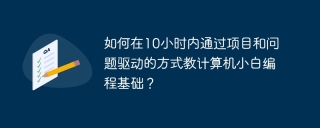
How to teach computer novice programming basics within 10 hours? If you only have 10 hours to teach computer novice some programming knowledge, what would you choose to teach...
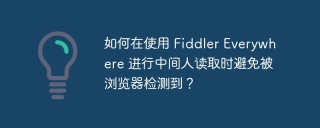
How to avoid being detected when using FiddlerEverywhere for man-in-the-middle readings When you use FiddlerEverywhere...


Hot AI Tools

Undresser.AI Undress
AI-powered app for creating realistic nude photos

AI Clothes Remover
Online AI tool for removing clothes from photos.

Undress AI Tool
Undress images for free

Clothoff.io
AI clothes remover

AI Hentai Generator
Generate AI Hentai for free.

Hot Article

Hot Tools
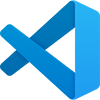
VSCode Windows 64-bit Download
A free and powerful IDE editor launched by Microsoft
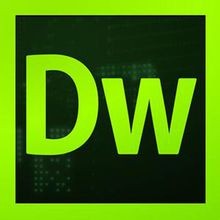
Dreamweaver CS6
Visual web development tools
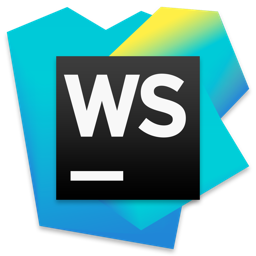
WebStorm Mac version
Useful JavaScript development tools

Safe Exam Browser
Safe Exam Browser is a secure browser environment for taking online exams securely. This software turns any computer into a secure workstation. It controls access to any utility and prevents students from using unauthorized resources.

Zend Studio 13.0.1
Powerful PHP integrated development environment