Written before&The author’s personal understanding
Recent research has emphasized the application prospects of NeRF in autonomous driving environments. However, the complexity of outdoor environments, coupled with restricted viewpoints in driving scenes, complicates the task of accurately reconstructing scene geometry. These challenges often result in reduced reconstruction quality and longer training and rendering durations. To address these challenges, we launched Lightning NeRF. It uses an efficient hybrid scene representation that effectively exploits lidar's geometric priors in autonomous driving scenarios. Lightning NeRF significantly improves NeRF's novel view synthesis performance and reduces computational overhead. Through evaluation on real-world datasets such as KITTI-360, Argoverse2, and our private dataset, we demonstrate that our method not only surpasses the current state-of-the-art in new view synthesis quality, but also improves in training speed Five times faster, and ten times faster rendering.
- Code link: https://github.com/VISION-SJTU/Lightning-NeRF
Detailed explanation of Lightning NeRF
Preliminaries
NeRF is a method of representing scenarios with implicit functions. This implicit Functions are usually parameterized by MLP. It is able to return the color value c and volume density prediction σ of a 3D point x in the scene based on the viewing direction d.
To render pixels, NeRF uses hierarchical volume sampling to generate a series of points along a ray r, and then combines the predicted density and color features at these locations through accumulation.
Although NeRF performs well in new perspective synthesis, its long training time and slow rendering speed are mainly caused by the inefficiency of the sampling strategy. To improve the model's efficiency, we maintain a coarse grid occupancy during training and only sample locations within the occupied volume. This sampling strategy is similar to existing work and helps improve model performance and speed up training.
Hybrid Scene Representation
Hybrid volume representation has been optimized and rendered quickly using compact models. Given this, we adopt a hybrid voxel grid representation to model the radiation field to improve efficiency. Briefly, we explicitly model the volumetric density by storing σ at the mesh vertices, while using a shallow MLP to implicitly decode the color embedding f into the final color c. To handle the boundaryless nature of outdoor environments, we divide the scene representation into two parts, foreground and background, as shown in Figure 2. Specifically, we examine the camera frustum in each frame from the trajectory sequence and define the foreground bounding box such that it tightly wraps all frustums in the aligned coordinate system. The background box is obtained by scaling up the foreground box along each dimension.
Voxel grid representation. A voxel mesh representation explicitly stores scene properties (e.g., density, RGB color, or features) in its mesh vertices to support efficient feature queries. This way, for a given 3D position, we can decode the corresponding attribute via trilinear interpolation:
Foreground. We build two independent feature grids to model the density and color embedding of the foreground region. Specifically, density mesh mapping maps positions into a density scalar σ for volumetric rendering. For color-embedded mesh mapping, we instantiate multiple voxel meshes at different resolution backups via hash tables to obtain finer details with affordable memory overhead. The final color embedding f is obtained by concatenating the outputs at L resolution levels.
Background Although the foreground modeling mentioned previously works for object-level radiation fields, extending it to unbounded outdoor scenes is not trivial. Some related techniques, such as NGP, directly extend their scene bounding box so that the background area can be included, while GANcraft and URF introduce spherical background radiation to deal with this problem. However, the former attempt resulted in a waste of its functionality since most of the area within its scene box was used for the background scene. For the latter scheme, it may not be able to handle complex panoramas in urban scenes (e.g., undulating buildings or complex landscapes) because it simply assumes that the background radiation depends only on the view direction.
For this, we set up an additional background mesh model to keep the resolution of the foreground part unchanged. We adopt the scene parameterization in [9] as the background, which is carefully designed. First, unlike inverse spherical modeling, we use inverse cubic modeling, with ℓ∞ norm, since we use voxel grid representation. Secondly we do not instantiate additional MLP to query the background color to save memory. Specifically, we warp 3D background points into 4D by:
LiDAR Initialization
Using our blending scene Representation, this model saves computation and memory when we query density values directly from an efficient voxel grid representation instead of a computationally intensive MLP. However, given the large-scale nature and complexity of urban scenes, this lightweight representation can easily get stuck in local minima during optimization due to the limited resolution of the density grid. Fortunately, in autonomous driving, most self-driving vehicles (SDVs) are equipped with LiDAR sensors, which provide rough geometric priors for scene reconstruction. To this end, we propose to use lidar point clouds to initialize our density mesh to alleviate the obstacles of joint optimization of scene geometry and radioactivity.
Color Decomposition
The original NeRF used a view-dependent MLP to model color in a radiation field, a simplification of the physical world where radiation Consists of diffuse (view-independent) color and specular (view-dependent) color. Furthermore, since the final output color c is completely entangled with the viewing direction d, it is difficult to render high-fidelity images in unseen views. As shown in Figure 3, our method trained without color decomposition (CD) fails at new view synthesis in the extrapolation setting (i.e., shifting the viewing direction 2 meters to the left based on the training view), while our method in color The decomposed case gives reasonable rendering results.
The final color at the sample location is the sum of these two factors:
Training Loss
We modify the photometric loss using rescaled weights wi to optimize our model to focus on hard samples for fast convergence. The weight coefficient is defined as:
##Picture
experiment
Conclusion
This paper introduces Lightning NeRF, an efficient outdoor scene view synthesis framework that integrates point clouds and images. The proposed method leverages point clouds to quickly initialize a sparse representation of the scene, achieving significant performance and speed enhancements. By modeling the background more efficiently, we reduce the representational strain on the foreground. Finally, through color decomposition, view-related and view-independent colors are modeled separately, which enhances the extrapolation ability of the model. Extensive experiments on various autonomous driving datasets demonstrate that our method outperforms previous state-of-the-art techniques in terms of performance and efficiency.
The above is the detailed content of Born for autonomous driving, Lightning NeRF: 10 times faster. For more information, please follow other related articles on the PHP Chinese website!
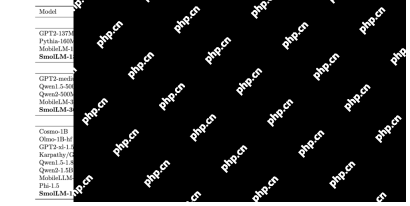
Harness the Power of On-Device AI: Building a Personal Chatbot CLI In the recent past, the concept of a personal AI assistant seemed like science fiction. Imagine Alex, a tech enthusiast, dreaming of a smart, local AI companion—one that doesn't rely
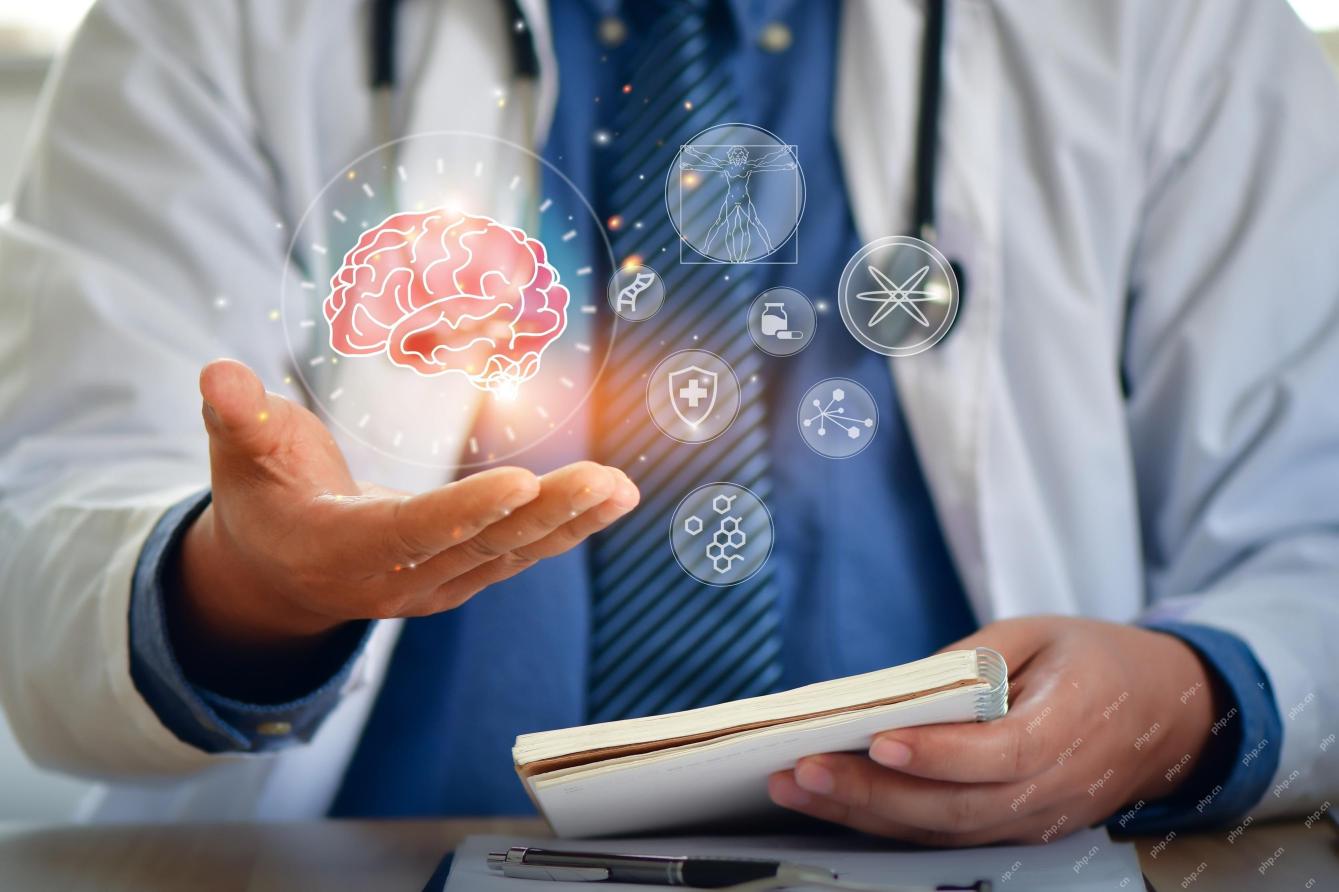
Their inaugural launch of AI4MH took place on April 15, 2025, and luminary Dr. Tom Insel, M.D., famed psychiatrist and neuroscientist, served as the kick-off speaker. Dr. Insel is renowned for his outstanding work in mental health research and techno
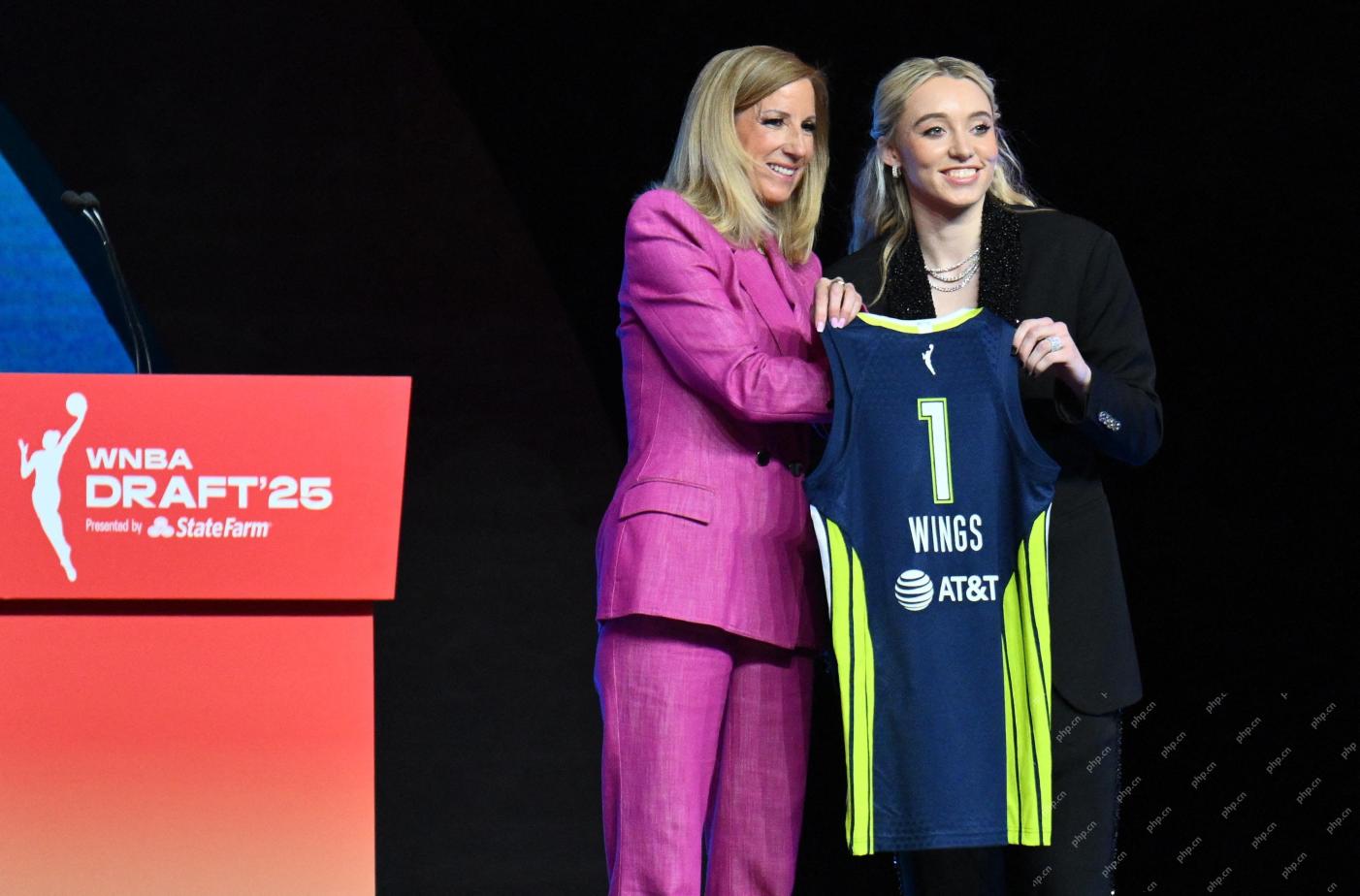
"We want to ensure that the WNBA remains a space where everyone, players, fans and corporate partners, feel safe, valued and empowered," Engelbert stated, addressing what has become one of women's sports' most damaging challenges. The anno
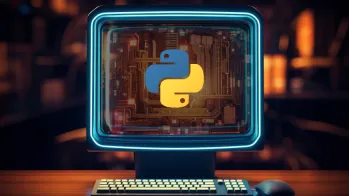
Introduction Python excels as a programming language, particularly in data science and generative AI. Efficient data manipulation (storage, management, and access) is crucial when dealing with large datasets. We've previously covered numbers and st
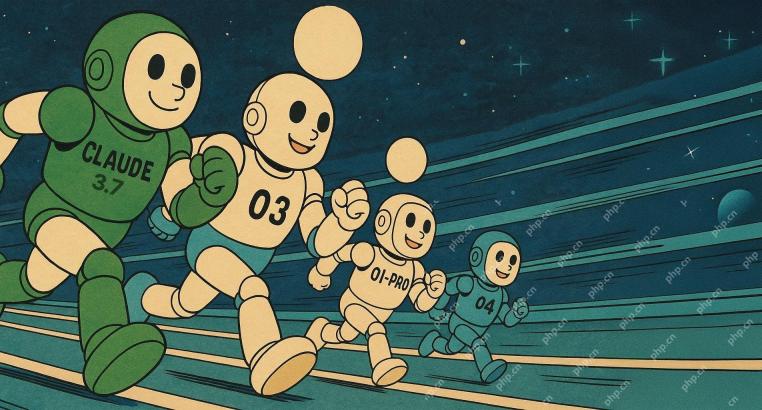
Before diving in, an important caveat: AI performance is non-deterministic and highly use-case specific. In simpler terms, Your Mileage May Vary. Don't take this (or any other) article as the final word—instead, test these models on your own scenario
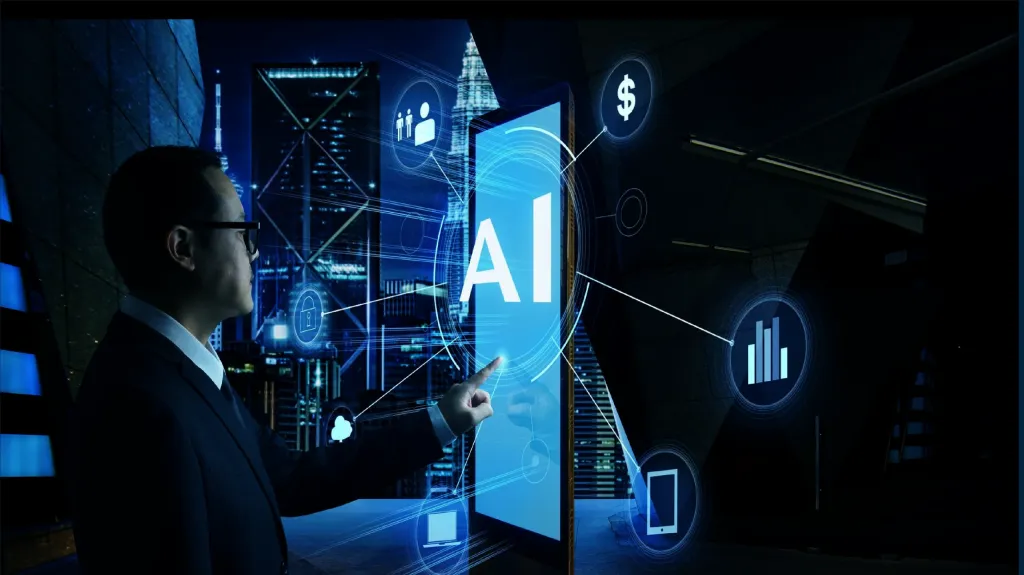
Building a Standout AI/ML Portfolio: A Guide for Beginners and Professionals Creating a compelling portfolio is crucial for securing roles in artificial intelligence (AI) and machine learning (ML). This guide provides advice for building a portfolio
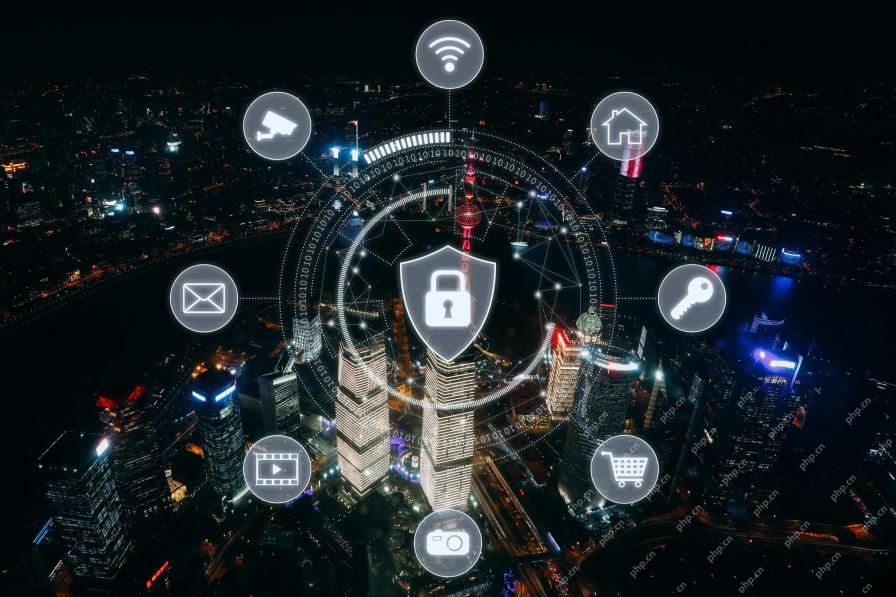
The result? Burnout, inefficiency, and a widening gap between detection and action. None of this should come as a shock to anyone who works in cybersecurity. The promise of agentic AI has emerged as a potential turning point, though. This new class
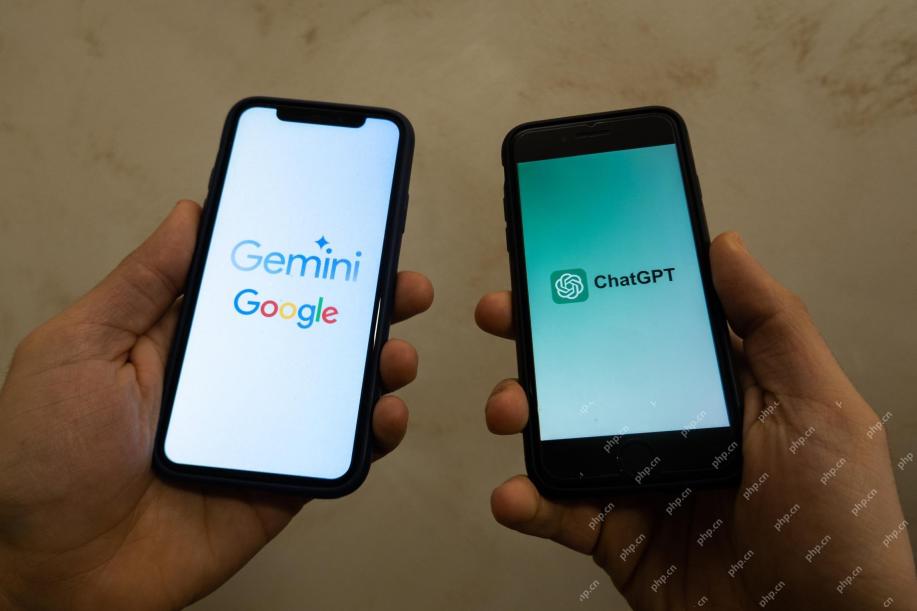
Immediate Impact versus Long-Term Partnership? Two weeks ago OpenAI stepped forward with a powerful short-term offer, granting U.S. and Canadian college students free access to ChatGPT Plus through the end of May 2025. This tool includes GPT‑4o, an a


Hot AI Tools

Undresser.AI Undress
AI-powered app for creating realistic nude photos

AI Clothes Remover
Online AI tool for removing clothes from photos.

Undress AI Tool
Undress images for free

Clothoff.io
AI clothes remover

AI Hentai Generator
Generate AI Hentai for free.

Hot Article

Hot Tools

DVWA
Damn Vulnerable Web App (DVWA) is a PHP/MySQL web application that is very vulnerable. Its main goals are to be an aid for security professionals to test their skills and tools in a legal environment, to help web developers better understand the process of securing web applications, and to help teachers/students teach/learn in a classroom environment Web application security. The goal of DVWA is to practice some of the most common web vulnerabilities through a simple and straightforward interface, with varying degrees of difficulty. Please note that this software

PhpStorm Mac version
The latest (2018.2.1) professional PHP integrated development tool

SublimeText3 English version
Recommended: Win version, supports code prompts!

SecLists
SecLists is the ultimate security tester's companion. It is a collection of various types of lists that are frequently used during security assessments, all in one place. SecLists helps make security testing more efficient and productive by conveniently providing all the lists a security tester might need. List types include usernames, passwords, URLs, fuzzing payloads, sensitive data patterns, web shells, and more. The tester can simply pull this repository onto a new test machine and he will have access to every type of list he needs.
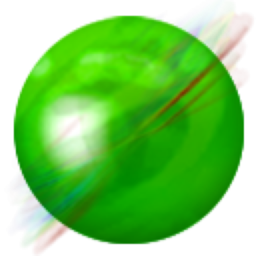
ZendStudio 13.5.1 Mac
Powerful PHP integrated development environment