


New work by Yan Shuicheng/Cheng Mingming! DiT training, the core component of Sora, is accelerated by 10 times, and Masked Diffusion Transformer V2 is open source
As one of Sora’s compelling core technologies, DiT utilizes Diffusion Transformer to scale the generative model to a larger scale to achieve outstanding image generation effects.
However, larger model sizes cause training costs to skyrocket.
The research team of Yan Shuicheng and Cheng Mingming from Sea AI Lab, Nankai University, and Kunlun Wanwei 2050 Research Institute proposed a new model called Masked Diffusion Transformer at the ICCV 2023 conference. This model uses mask modeling technology to speed up the training of Diffusion Transformer by learning semantic representation information, and achieves SoTA effects in the field of image generation. This innovation brings new breakthroughs to the development of image generation models and provides researchers with a more efficient training method. By combining expertise and technology from different fields, the research team successfully proposed a solution that increases training speed and improves generation results. Their work has contributed important innovative ideas to the development of the field of artificial intelligence and provided useful inspiration for future research and practice
Picture
Paper address: https://arxiv.org/abs/2303.14389
GitHub address: https://github.com/sail-sg/MDT
Recently, Masked Diffusion Transformer V2 once again refreshed SoTA, increasing the training speed by more than 10 times compared to DiT, and achieving an FID score of 1.58 on the ImageNet benchmark.
The latest versions of papers and codes are open source.
Background
Although diffusion models represented by DiT have achieved significant success in the field of image generation, researchers have found that diffusion models often It is difficult to efficiently learn the semantic relationships between parts of objects in images, and this limitation leads to low convergence efficiency of the training process.
Picture
For example, as shown in the picture above, DiT has learned at the 50kth training step Generate the dog's hair texture, and then learn to generate one of the dog's eyes and mouth at the 200k training step, but miss the other eye.
Even at the 300k training step, the relative position of the dog’s two ears generated by DiT is not very accurate.
This training and learning process reveals that the diffusion model fails to efficiently learn the semantic relationship between the various parts of the object in the image, but only learns the semantic information of each object independently.
The researchers speculate that the reason for this phenomenon is that the diffusion model learns the distribution of real image data by minimizing the prediction loss of each pixel. This process ignores the relationship between the various parts of the object in the image. The semantic relative relationship between them leads to the slow convergence speed of the model.
Method: Masked Diffusion Transformer
Inspired by the above observations, the researchers proposed the Masked Diffusion Transformer (MDT) to improve the training of diffusion models efficiency and build quality.
MDT proposes a mask modeling representation learning strategy designed for Diffusion Transformer to explicitly enhance Diffusion Transformer's learning ability of contextual semantic information and enhance the relationship between objects in the image Associative learning of semantic information.
Picture
As shown in the figure above, MDT introduces mask modeling while maintaining the diffusion training process Learning Strategies. By masking the noisy image token, MDT uses an asymmetric Diffusion Transformer (Asymmetric Diffusion Transformer) architecture to predict the masked image token from the noisy image token that has not been masked, thereby simultaneously achieving the mask modeling and diffusion training processes.
During the inference process, MDT still maintains the standard diffusion generation process. The design of MDT helps Diffusion Transformer have both the semantic information expression ability brought by mask modeling representation learning and the diffusion model's ability to generate image details.
Specifically, MDT maps images to latent space through VAE encoder and processes them in latent space to save computing costs.
During the training process, MDT first masks out some of the noise-added image tokens, and sends the remaining tokens to the Asymmetric Diffusion Transformer to predict all image tokens after denoising.
Asymmetric Diffusion Transformer Architecture
##Picture
Picture
##Picture
As shown in the figure above, MDTv2 further optimizes the learning process of diffusion and mask modeling by introducing a more efficient macro network structure designed for the Masked Diffusion process.
This includes integrating U-Net-style long-shortcut in the encoder and dense input-shortcut in the decoder.
Among them, dense input-shortcut will add noise to the masked token and send it to the decoder, retaining the noise information corresponding to the masked token, thus facilitating the training of the diffusion process. .
In addition, MDT has also introduced better training strategies including the faster Adan optimizer, time-step related loss weights, and expanded mask ratio to further accelerate Masked The training process of the Diffusion model.
Experimental results
ImageNet 256 benchmark generation quality comparison
Image
The above table compares the performance of MDT and DiT under different model sizes under the ImageNet 256 benchmark.
It is obvious that MDT achieves higher FID scores with less training cost at all model sizes.
The parameters and inference costs of MDT are basically the same as DiT, because as mentioned above, the standard diffusion process consistent with DiT is still maintained during the inference process of MDT.
For the largest XL model, MDTv2-XL/2 trained with 400k steps significantly outperforms DiT-XL/2 trained with 7000k steps, with a FID score improvement of 1.92. Under this setting, the results show that MDT has about 18 times faster training than DiT.
For small models, MDTv2-S/2 still achieves significantly better performance than DiT-S/2 with significantly fewer training steps. For example, with the same training of 400k steps, MDTv2 has an FID index of 39.50, which is significantly ahead of DiT's FID index of 68.40.
More importantly, this result also exceeds the performance of the larger model DiT-B/2 at 400k training steps (39.50 vs 43.47).
ImageNet 256 benchmark CFG generation quality comparison
Image
We are still here The above table compares the image generation performance of MDT and existing methods under classifier-free guidance.
MDT surpasses previous SOTA DiT and other methods with an FID score of 1.79. MDTv2 further improves performance, pushing the SOTA FID score for image generation to a new low of 1.58 with fewer training steps.
Similar to DiT, we did not observe saturation of the model’s FID scores during training as we continued training.
MDT refreshes SoTA on PaperWithCode’s leaderboard
Convergence speed comparison
Picture
The above picture compares the DiT-S/2 baseline, MDT-S/2 and MDTv2 on the 8×A100 GPU under the ImageNet 256 benchmark. - FID performance of S/2 under different training steps/training times.
Thanks to its better contextual learning capabilities, MDT surpasses DiT in both performance and generation speed. The training convergence speed of MDTv2 is more than 10 times higher than that of DiT.
MDT is about 3 times faster than DiT in terms of training steps and training time. MDTv2 further improves the training speed by approximately 5 times compared to MDT.
For example, MDTv2-S/2 shows better performance in just 13 hours (15k steps) than DiT-S/2 which takes about 100 hours (1500k steps) to train , which reveals that context representation learning is crucial for faster generative learning of diffusion models.
Summary & Discussion
MDT can utilize the characteristics of image objects by introducing a mask modeling representation learning scheme similar to MAE in the diffusion training process. Context information reconstructs the complete information of incomplete input images, thereby learning the correlation between semantic parts in the image, thereby improving the quality and learning speed of image generation.
Researchers believe that enhancing the semantic understanding of the physical world through visual representation learning can improve the simulation effect of the generative model on the physical world. This coincides with Sora's vision of building a physical world simulator through generative models. Hopefully this work will inspire more work on unifying representation learning and generative learning.
Reference:
https://arxiv.org/abs/2303.14389
The above is the detailed content of New work by Yan Shuicheng/Cheng Mingming! DiT training, the core component of Sora, is accelerated by 10 times, and Masked Diffusion Transformer V2 is open source. For more information, please follow other related articles on the PHP Chinese website!
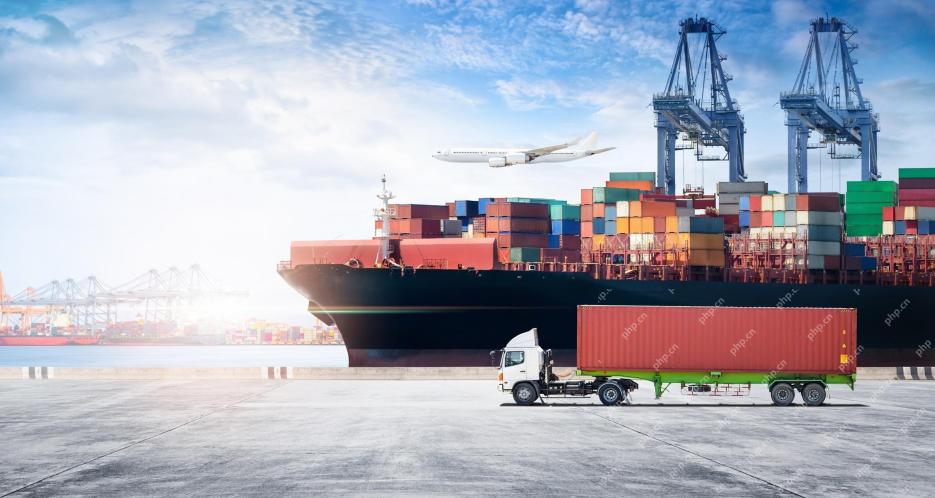
The term "AI-ready workforce" is frequently used, but what does it truly mean in the supply chain industry? According to Abe Eshkenazi, CEO of the Association for Supply Chain Management (ASCM), it signifies professionals capable of critic
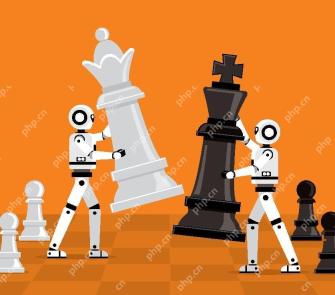
The decentralized AI revolution is quietly gaining momentum. This Friday in Austin, Texas, the Bittensor Endgame Summit marks a pivotal moment, transitioning decentralized AI (DeAI) from theory to practical application. Unlike the glitzy commercial
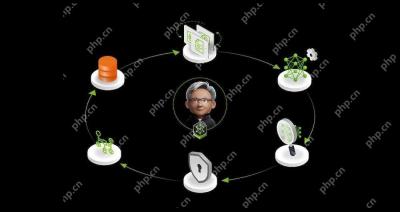
Enterprise AI faces data integration challenges The application of enterprise AI faces a major challenge: building systems that can maintain accuracy and practicality by continuously learning business data. NeMo microservices solve this problem by creating what Nvidia describes as "data flywheel", allowing AI systems to remain relevant through continuous exposure to enterprise information and user interaction. This newly launched toolkit contains five key microservices: NeMo Customizer handles fine-tuning of large language models with higher training throughput. NeMo Evaluator provides simplified evaluation of AI models for custom benchmarks. NeMo Guardrails implements security controls to maintain compliance and appropriateness
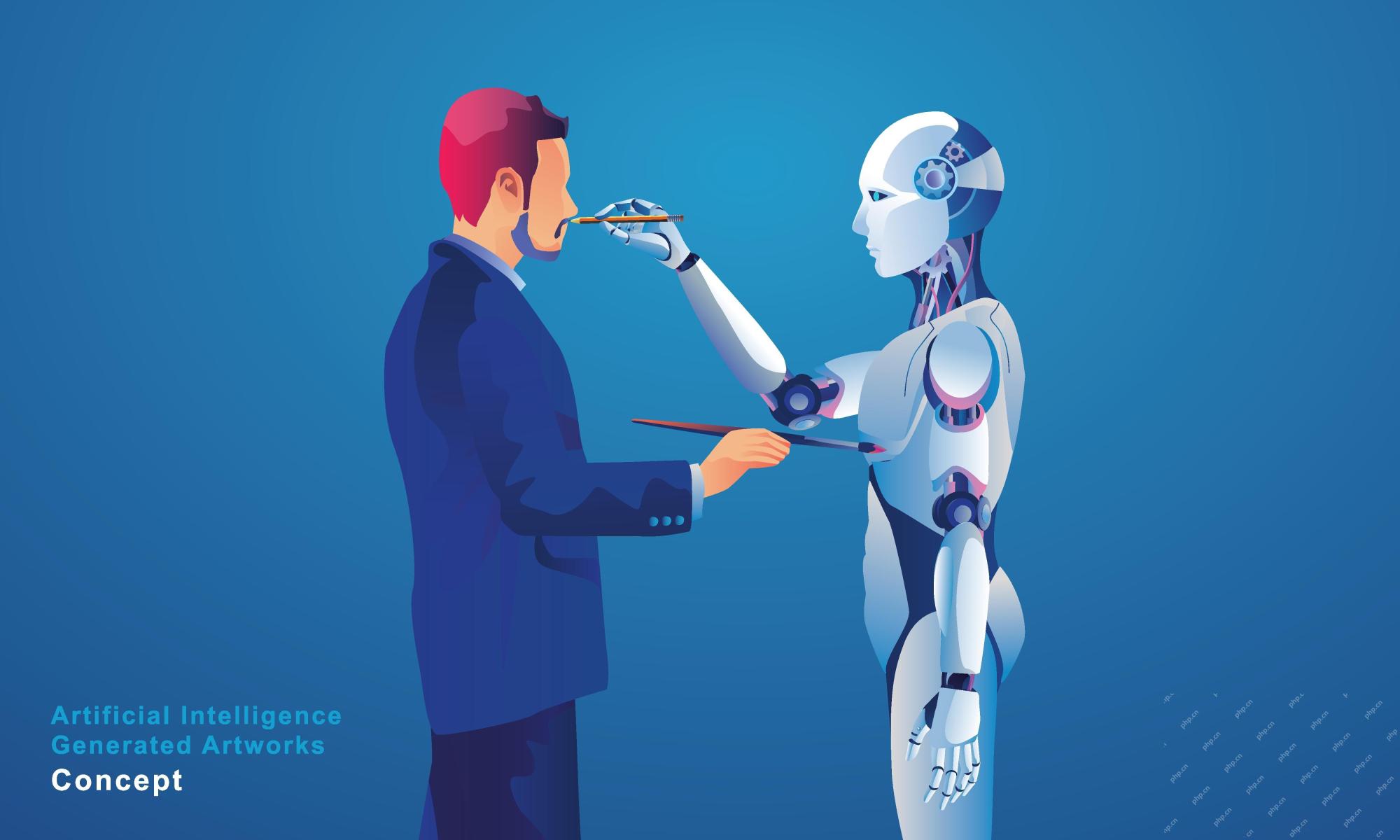
AI: The Future of Art and Design Artificial intelligence (AI) is changing the field of art and design in unprecedented ways, and its impact is no longer limited to amateurs, but more profoundly affecting professionals. Artwork and design schemes generated by AI are rapidly replacing traditional material images and designers in many transactional design activities such as advertising, social media image generation and web design. However, professional artists and designers also find the practical value of AI. They use AI as an auxiliary tool to explore new aesthetic possibilities, blend different styles, and create novel visual effects. AI helps artists and designers automate repetitive tasks, propose different design elements and provide creative input. AI supports style transfer, which is to apply a style of image
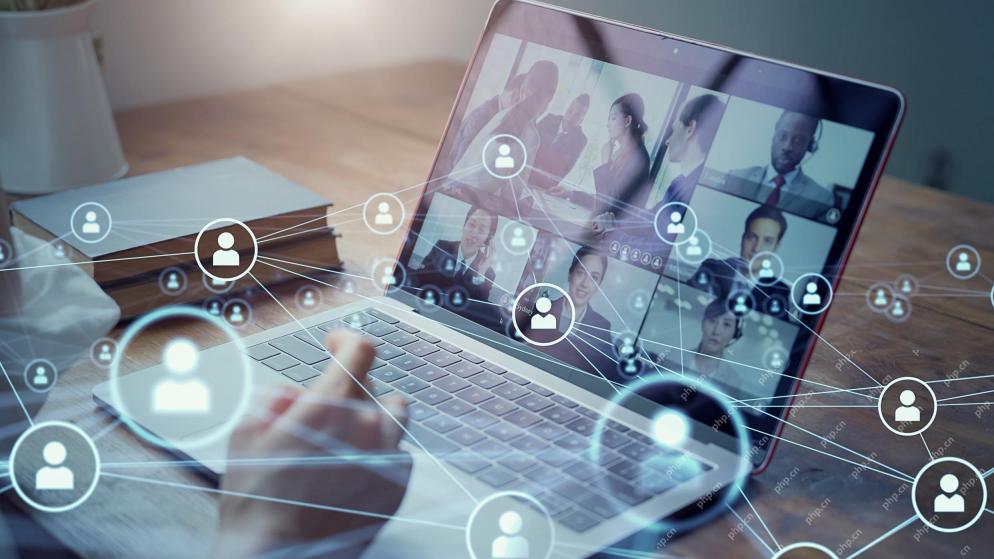
Zoom, initially known for its video conferencing platform, is leading a workplace revolution with its innovative use of agentic AI. A recent conversation with Zoom's CTO, XD Huang, revealed the company's ambitious vision. Defining Agentic AI Huang d
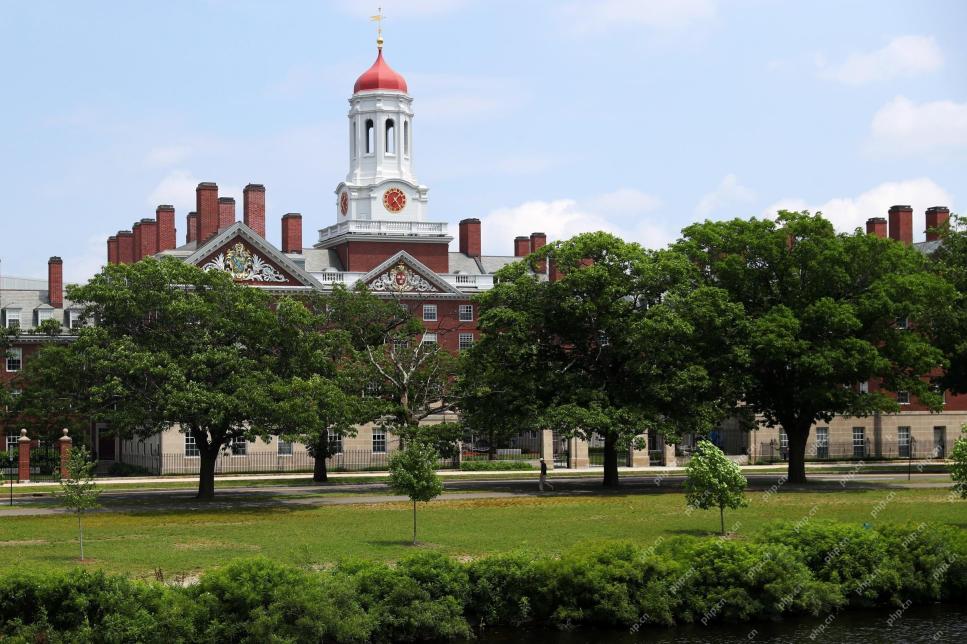
Will AI revolutionize education? This question is prompting serious reflection among educators and stakeholders. The integration of AI into education presents both opportunities and challenges. As Matthew Lynch of The Tech Edvocate notes, universit
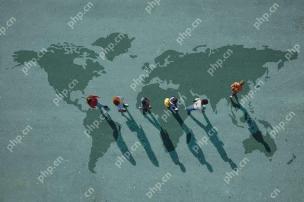
The development of scientific research and technology in the United States may face challenges, perhaps due to budget cuts. According to Nature, the number of American scientists applying for overseas jobs increased by 32% from January to March 2025 compared with the same period in 2024. A previous poll showed that 75% of the researchers surveyed were considering searching for jobs in Europe and Canada. Hundreds of NIH and NSF grants have been terminated in the past few months, with NIH’s new grants down by about $2.3 billion this year, a drop of nearly one-third. The leaked budget proposal shows that the Trump administration is considering sharply cutting budgets for scientific institutions, with a possible reduction of up to 50%. The turmoil in the field of basic research has also affected one of the major advantages of the United States: attracting overseas talents. 35
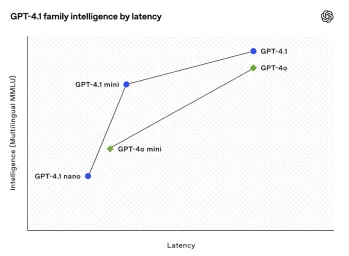
OpenAI unveils the powerful GPT-4.1 series: a family of three advanced language models designed for real-world applications. This significant leap forward offers faster response times, enhanced comprehension, and drastically reduced costs compared t


Hot AI Tools

Undresser.AI Undress
AI-powered app for creating realistic nude photos

AI Clothes Remover
Online AI tool for removing clothes from photos.

Undress AI Tool
Undress images for free

Clothoff.io
AI clothes remover

Video Face Swap
Swap faces in any video effortlessly with our completely free AI face swap tool!

Hot Article

Hot Tools

Safe Exam Browser
Safe Exam Browser is a secure browser environment for taking online exams securely. This software turns any computer into a secure workstation. It controls access to any utility and prevents students from using unauthorized resources.

Notepad++7.3.1
Easy-to-use and free code editor

SAP NetWeaver Server Adapter for Eclipse
Integrate Eclipse with SAP NetWeaver application server.

SublimeText3 Chinese version
Chinese version, very easy to use
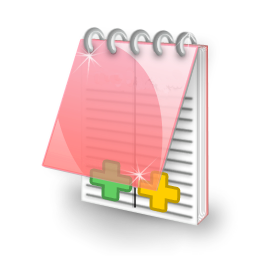
EditPlus Chinese cracked version
Small size, syntax highlighting, does not support code prompt function
