This model uses the DiT framework like Sora.
As we all know, developing top-level T2I models requires a lot of resources, so it is basically impossible for individual researchers with limited resources. Affordability has also become a major obstacle to innovation in the AIGC (artificial intelligence content generation) community. At the same time, as time goes by, the AIGC community will be able to obtain continuously updated, higher-quality data sets and more advanced algorithms. So the key question is: how can we efficiently integrate these new elements into the existing model and make the model more powerful with limited resources? ? In order to explore this problem, a research team from research institutions such as Huawei’s Noah’s Ark Laboratory proposed a new training method: weak-to-strong training (weak- to-strong training). 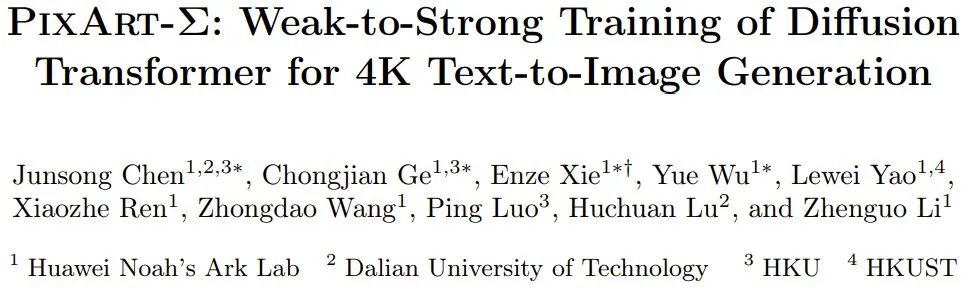
Paper title: PixArt-Σ: Weak-to-Strong Training of Diffusion Transformer for 4K Text-to-Image GenerationPaper address: https: //arxiv.org/pdf/2403.04692.pdfProject page: https://pixart-alpha.github.io/PixArt-sigma-project/ The team used the pre-trained base model of PixArt-α and integrated advanced elements to promote its continuous improvement, ultimately resulting in a more powerful model PixArt-Σ. Figure 1 shows some examples of generated results. 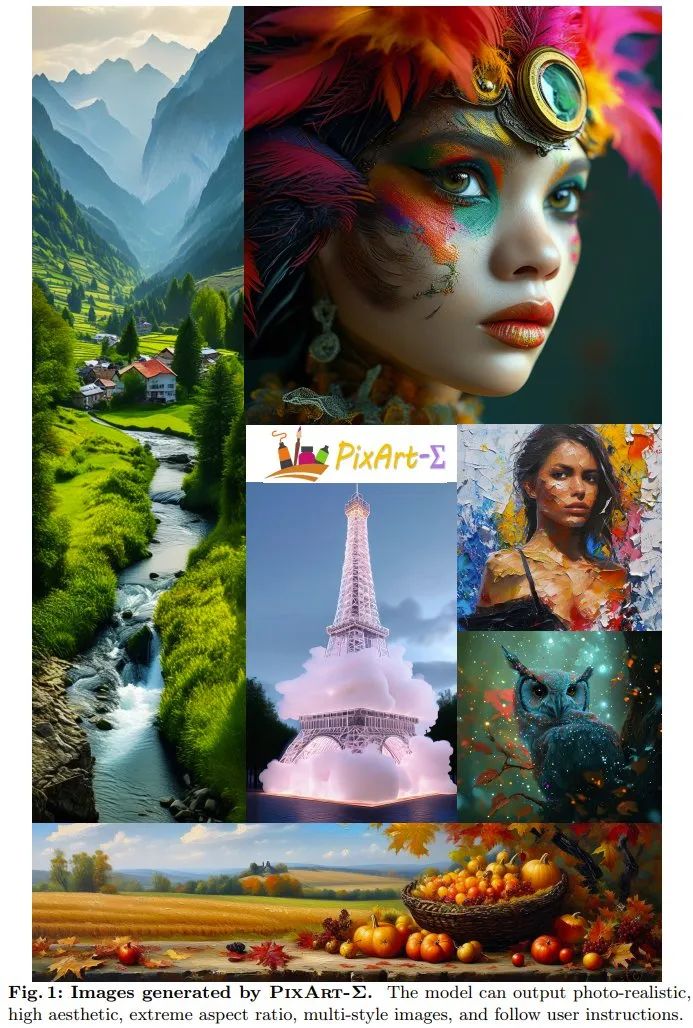
# Specifically, in order to achieve weak-to-strong training and create PixArt-Σ, the team adopted the following improvement measures. Higher quality training dataThe team collected a high-quality data Set Internal-Σ, which mainly focuses on two aspects: #(1) High-quality images: This dataset contains 33 million high-resolution images from the Internet, all Over 1K resolution, including 2.3 million images at approximately 4K resolution. The main features of these images are their high aesthetics and cover a wide range of artistic styles. (2) Dense and accurate description: In order to provide a more precise and detailed description for the above image, the team replaced the LLaVA used in PixArt-α with a A more powerful image descriptor Share-Captioner. Not only that, in order to improve the model’s ability to align text concepts and visual concepts, the team extended the token length of the text encoder (i.e. Flan-T5) to approximately 300 words . They observed that these improvements effectively eliminate the model's tendency to hallucinate, enabling higher quality text-image alignment. Table 1 below shows the statistics of different data sets. 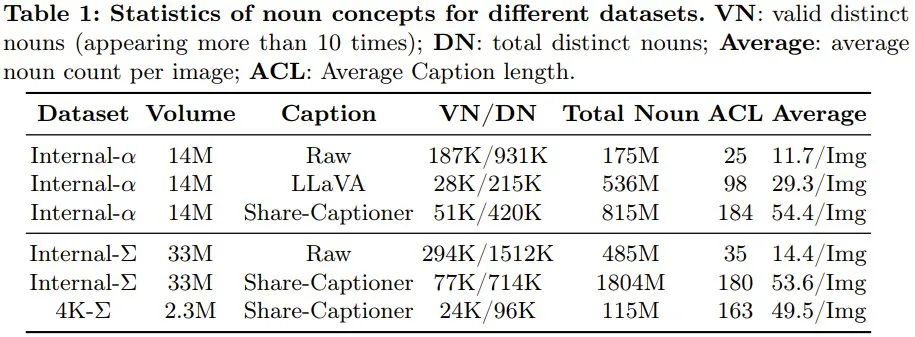
Efficient token compressionTo enhance PixArt-α, the team The generation resolution has been increased from 1K to 4K. In order to generate ultra-high resolution (such as 2K/4K) images, the number of tokens will increase significantly, which will lead to a significant increase in computing requirements. To solve this problem, they introduced a self-attention module specially tuned for the DiT framework, which uses key and value token compression. Specifically, they used grouped convolutions with stride 2 to perform local aggregation of keys and values, as shown in Figure 7 below. 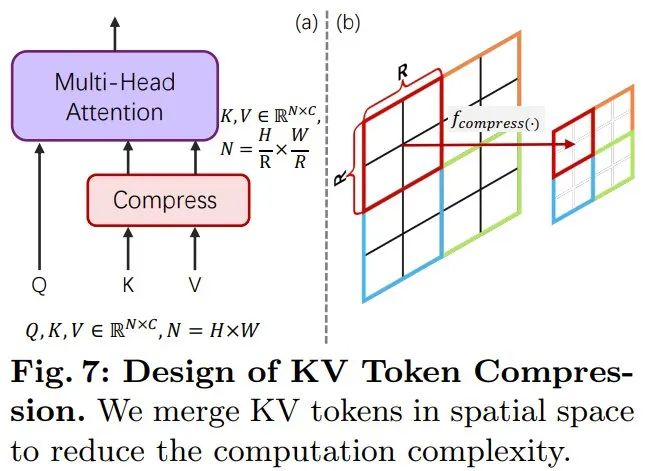
In addition, the team adopted a specially designed weight initialization scheme to achieve smooth adaptation from pre-trained models without using KV (key-value) compression. This design effectively reduces training and inference time for high-resolution image generation by approximately 34%. Weak to strong training strategyThe team proposed a variety of fine-tuning technology that can quickly and efficiently adjust weak models to strong models. These include: (1) Replacement uses a more powerful variational autoencoder (VAE): PixArt-α's VAE is replaced by SDXL's VAE. (2) From low resolution to high resolution expansion, in order to cope with the problem of performance degradation, they use the Position Embedding (PE) interpolation method. (3) Evolve from a model that does not use KV compression to a model that uses KV compression. #The experimental results verified the feasibility and effectiveness of the weak-to-strong training method. Through the above improvements, PixArt-Σ can generate high-quality 4K resolution images with the lowest possible training cost and as few model parameters as possible. Specifically, by starting with fine-tuning from an already pre-trained model, the team only used an additional 9% of the GPU time required by PixArt-α. A model capable of generating 1K high-resolution images. This performance is remarkable because it also uses new training data and a more powerful VAE. In addition, the parameter amount of PixArt-Σ is only 0.6B. In comparison, the parameter amount of SDXL and SD Cascade are 2.6B and 5.1B respectively. The beauty of the images generated by PixArt-Σ is comparable to that of current top-notch PixArt products, such as DALL・E 3 and MJV6. In addition, PixArt-Σ also demonstrates excellent capabilities for fine-grained alignment with text prompts.
Figure 2 shows the result of PixArt-Σ generating a 4K high-resolution image. It can be seen that the generated result follows complex and information-dense text instructions well. 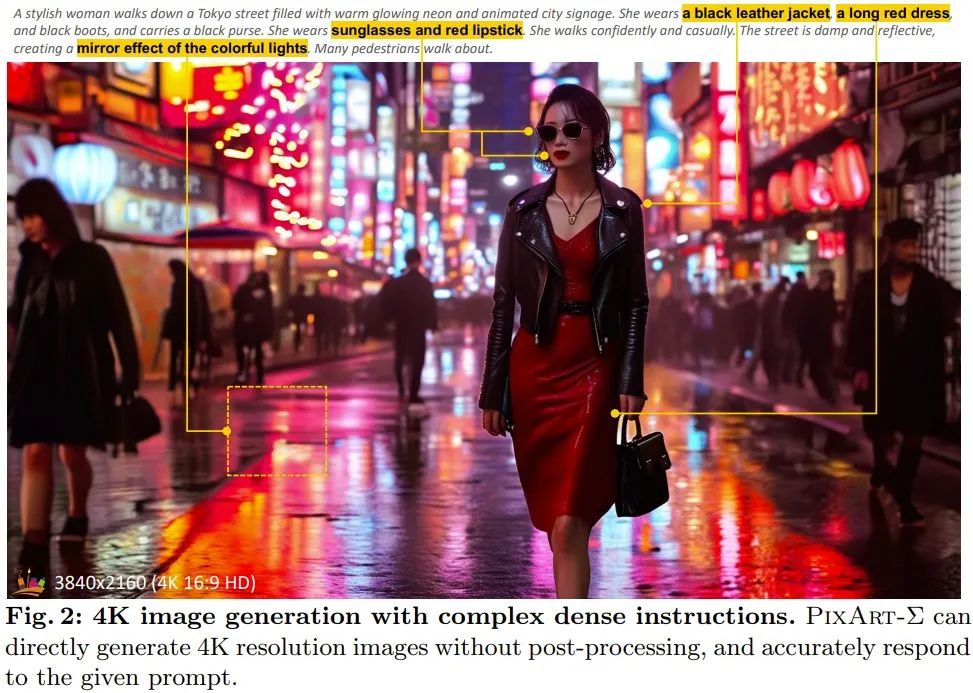
Training details: For the text encoder that performs conditional feature extraction, the team used a T5 encoder (i.e., Flan-T5-XXL) following the practices of Imagen and PixArt-α. The basic diffusion model is PixArt-α. Different from the practice of extracting a fixed 77 text tokens in most studies, the length of text tokens is increased from 120 in PixArt-α to 300 because the description information organized in Internal-Σ is more dense and can provide highly fine-grained details. . In addition, VAE uses a pre-trained frozen version of VAE from SDXL. Other implementation details are the same as PixArt-α.
The model is fine-tuned based on PixArt-α’s 256px pre-training checkpoint and uses positional embedding interpolation technology.
The final model (including 1K resolution) was trained on 32 V100 GPUs. They also used an additional 16 A100 GPUs to train 2K and 4K image generation models.
Evaluation indicators: In order to better demonstrate the aesthetics and semantic capabilities, the team collected 30,000 high-quality text-image pairs to compare the most powerful text-image pairs Models are benchmarked. PixArt-Σ is primarily evaluated here by human and AI preferences, as the FID metric may not appropriately reflect the generation quality.
Image quality assessment: The team qualitatively compared PixArt- Σ versus closed-source T2I products and open-source model generation quality. As shown in Figure 3, compared to the open source model SDXL and the team’s previous PixArt-α, the portraits generated by PixArt-Σ are more realistic and have better semantic analysis capabilities. PixArt-Σ follows user instructions better than SDXL.
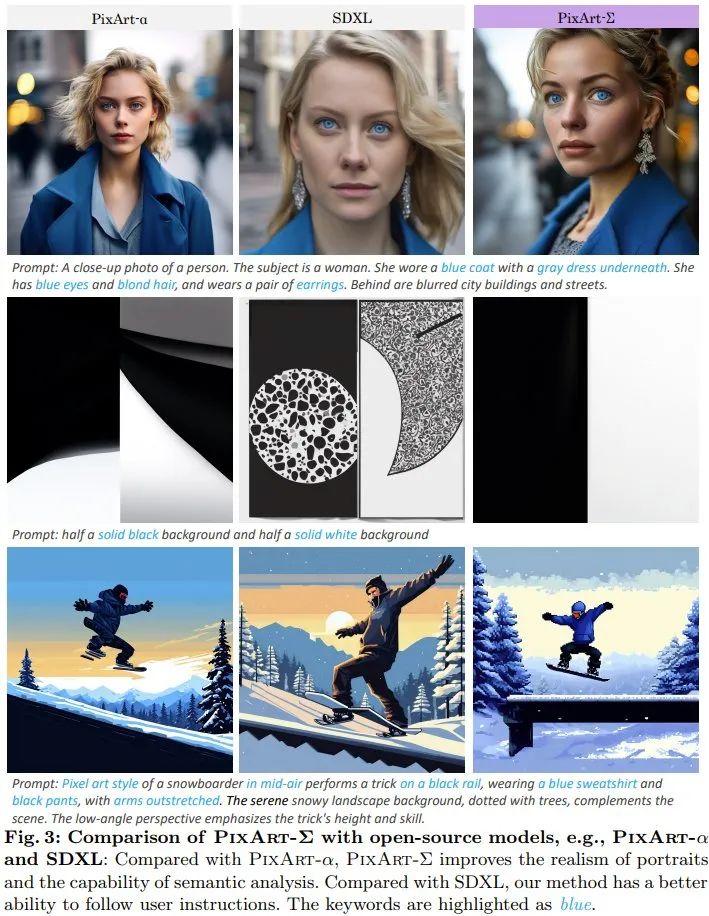
#PixArt-Σ not only outperforms open source models, but is also competitive with current closed source products, as shown in Figure 4.
Generate high-resolution images: The new method can directly generate 4K resolution images without any post-processing. In addition, PixArt-Σ can also accurately comply with complex and detailed long text provided by users. Therefore, users do not need to bother designing prompts to get satisfactory results. Human/AI (GPT-4V) preference study: The team also studied human and AI preferences for generated results. They collected the generation results of 6 open source models, including PixArt-α, PixArt-Σ, SD1.5, Stable Turbo, Stable XL, Stable Cascade and Playground-V2.0. They developed a website that collects human preference feedback by displaying prompts and corresponding images. #Human evaluators can rank images based on generation quality and how well they match the prompt. The results are shown in the blue bar graph of Figure 9. It can be seen that human evaluators prefer PixArt-Σ to the other 6 generators. Compared with previous Vincentian graph diffusion models, such as SDXL (2.6B parameters) and SD Cascade (5.1B parameters), PixArt-Σ can generate higher quality and more consistent with user prompts with much fewer parameters (0.6B) image.
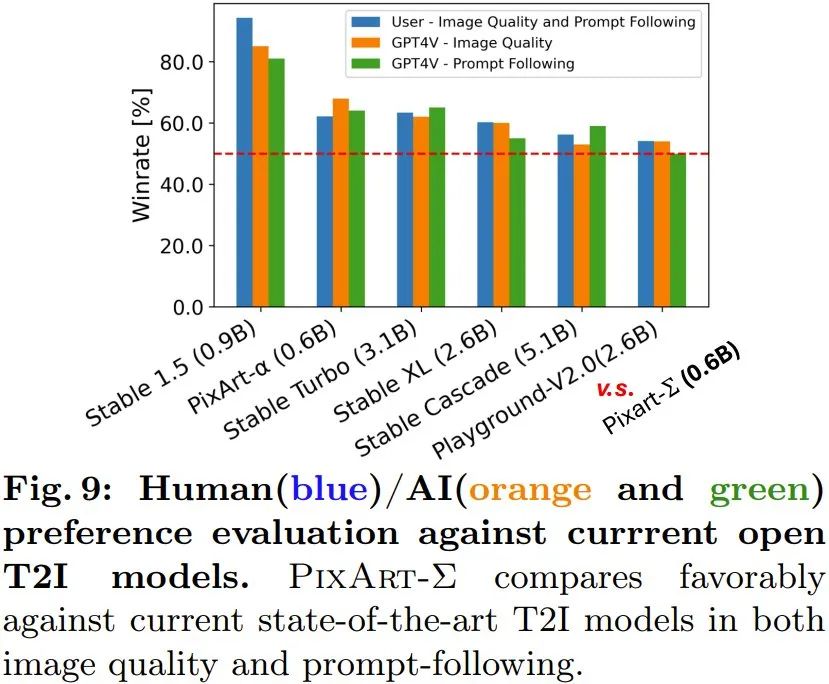
Additionally, the team used an advanced multi-modal model, GPT-4 Vision, to perform AI preference studies. What they do is feed GPT-4 Vision two images and let it vote based on image quality and image-text alignment. The results are shown in the orange and green bars in Figure 9, and it can be seen that the situation is basically consistent with human evaluation. The team also conducted ablation studies to verify the effectiveness of various improvement measures. For more details, please visit the original paper. Reference article: 1. https://www.shoufachen.com/Awesome-Diffusion-Transformers/The above is the detailed content of Based on DiT and supporting 4K image generation, Huawei Noah 0.6B Vincent graph model PixArt-Σ is here. For more information, please follow other related articles on the PHP Chinese website!