Written in front & the author’s personal understanding
I am very happy to be invited to participate in the Heart of Autonomous Driving event. We will share the resistance to online reconstruction of vectorized high-precision maps. Perturbation method ADMap. You can find our code at https://github.com/hht1996ok/ADMap. Thank you all for your attention and support.
In the field of autonomous driving, online high-definition map reconstruction is of great significance for planning and prediction tasks. Recent work has built many high-performance high-definition map reconstruction models to meet this need. However, the point order within the vectorized instance may be jittered or jagged due to prediction bias, which will affect subsequent tasks. Therefore, we propose the Anti-Disturbance Map reconstruction framework (ADMap). This article hopes to take into account model speed and overall accuracy, and not bother engineers when deploying. Therefore, three efficient and effective modules are proposed: Multi-Scale Perception Neck (MPN), Instance Interactive Attention (IIA), and Vector Direction Difference Loss (VDDL). By cascading to explore point order relationships between and within instances, our model better supervises the point order prediction process.
We verified the effectiveness of ADMap in nuScenes and Argoverse2 datasets. Experimental results show that ADMap exhibits the best performance in various benchmark tests. In the nuScenes benchmark, ADMap improves mAP by 4.2% and 5.5% compared to the baseline using only camera data and multi-modal data, respectively. ADMapv2 not only reduces inference latency, but also significantly improves baseline performance, with the highest mAP reaching 82.8%. In the Argoverse dataset, the mAP of ADMapv2 increased to 62.9% while the frame rate remained at 14.8FPS.
In summary, the ADMap we proposed has the following main contributions:
- Proposed an end-to-end ADMap and reconstructed a more stable vectorized high-precision map.
- MPN better captures multi-scale information without increasing reasoning resources. IIA completes effective interaction between instances and within instances, making point-level features more accurate. VDDL constrains points in more detail. The sequence reconstruction process is supervised on the geometric relationship of the point sequence.
- ADMap implements real-time reconstruction of vectorized high-precision maps and achieves the highest accuracy in the nuScenes benchmark and Argoverse2.
Method proposed
As shown in Figure 1, the prediction points in the example often inevitably have jitter or offset. This jitter will As a result, the reconstructed instance vector becomes unsmooth or jagged, seriously affecting the quality and practicality of online high-precision maps. We believe that the reason is that existing models do not fully consider the interaction between instances and within instances. Incomplete interaction between instance points and map topological information will lead to inaccurate predicted positions. In addition, only supervision such as L1 loss and cosine embedding loss cannot effectively use geometric relationships to constrain the prediction process of instance points. The network needs to use vector line segments between points to finely capture the direction information of the point sequence to more accurately constrain each point. prediction process.
In order to alleviate the above problems, we innovatively proposed the Anti-Disturbance Map reconstruction framework (ADMap) to achieve real-time and stable reconstruction of vectorized high-precision maps.
Method design
As shown in Figure 2, ADMap uses multi-scale perception neck (Multi-Scale Perception Neck, MPN), instance interactive attention (Instance Interactive Attention) , IIA) and Vector Direction Difference Loss (VDDL) to predict the point order topology more precisely. MPN, IIA and VDDL will be introduced respectively below.
Multi-Scale Perception Neck
In order to obtain more detailed BEV features, we introduce Multi-Scale Perception Neck (MPN ). MPN receives the fused BEV features as input. Through downsampling, the BEV features of each level are connected to an upsampling layer to restore the original size feature map. Finally, the feature maps at each level will be merged into multi-scale BEV features.
The dotted line in Figure 2 means that this step is only implemented during training, and the solid line means that this step will be implemented during both training and inference processes. During the training process, multi-scale BEV feature maps and BEV feature maps at each level are sent to the Transformer Decoder, which allows the network to predict instance information of the scene at different scales to capture more refined multi-scale features. During the inference process, MPN only retains multi-scale BEV features and does not output feature maps at each level. This ensures that the resource usage of the neck during inference remains unchanged.
Transformer Decoder
Transformer Decoder defines a set of instance-level queries and a set of point-level queries, and then shares the point-level queries to all instances. These hierarchical queries are defined as:
The decoder consists of several cascaded decoding layers that iteratively update the hierarchical query. In each decoding layer, hierarchical queries are input into the self-attention mechanism, which allows information to be exchanged between hierarchical queries. Deformable Attention is used to interact with hierarchical queries and multi-scale BEV features.
Instance Interactive Attention
In order to better obtain the characteristics of each instance in the decoding stage, we proposed Instance Interactive Attention (IIA), which consists of Instances self-attention and Points self-attention composition. Unlike MapTRv2 which extracts instance-level and point-level embeddings in parallel, IIA extracts query embeddings cascaded. Feature interactions between instance embeddings further help the network learn relationships between point-level embeddings.
As shown in Figure 3, the hierarchical embeddings output by Deformable cross-attention are input to Instances self-attention. After merging the point dimension and the channel dimension, the dimension transformation is. Subsequently, the hierarchical embedding is connected to the Embed Layer composed of multiple MLPs to obtain the instance query. The query is put into Multi-head self-attention to capture the topological relationship between instances and obtain the instance embedding. To incorporate instance-level information into point-level embeddings, we sum instance embeddings and hierarchical embeddings. The added features are input into Point self-attention, which interacts with the point features within each instance to further finely correlate the topological relationships between point sequences.
Vector Direction Difference Loss
The high-definition map contains vectorized static map elements, including lane lines, curbs, and crosswalks. ADMap proposes Vector Direction Difference Loss for these open shapes (lane lines, curbs) and closed shapes (crosswalks). We model the point sequence vector direction inside the instance, and the direction of the point can be supervised in more detail by the difference between the predicted vector direction and the true vector direction. In addition, points with large differences in real vector directions are considered to represent drastic changes in the topology of some scenes (more difficult to predict), and require more attention from the model. Therefore, points with larger true vector direction differences are given greater weight to ensure that the network can accurately predict this drastic change point.
Figure 4 shows the predicted point sequence { and the real point sequence { ) for the predicted vector line { and the initial modeling of true vector lines { . To ensure that opposite angles don't get the same loss, we calculate the cosine of the vector line angle difference θ':
where the function accumulates the vector lines The coordinate position represents the normalization operation. We use the vector angle difference of each point in the real instance to assign weights of different sizes to them. The weight is defined as follows:
# which represents the number of points in the instance, and the function represents the exponential function with the base e. Since the vector angle difference cannot be calculated between the first and last points, we set the weight of the first and last points to 1. When the vector angle difference in the ground truth becomes larger, we give that point a greater weight, which makes the network pay more attention to significantly changing map topology. The angle difference loss of each point in the point sequence is defined as:
We use θ to adjust the interval of the loss value to [0.0, 2.0]. By adding the cosines of the angle differences between adjacent vector lines at each point, this loss more comprehensively covers the geometric topology information of each point. Since the first and last two points have only one adjacent vector line, the loss of the first and last two points is the cosine of the single vector angle difference.
Experiment
For fair evaluation, we divide the map elements into three types: lane lines, road boundaries and crosswalks. The average accuracy (AP) is used to evaluate the quality of map construction, and the sum of the chamfer distances between the predicted point order and the true point order is used to determine whether the two match. The Chamfer distance threshold is set to [0.5, 1.0, 1.5], we calculate AP under these three thresholds respectively, and use the average as the final indicator.
Comparative Experiment
Table 1 reports the metrics of ADMap and state-of-the-art methods on the nuScenes dataset. Under the camera-only framework, ADMap's mAP increased by 5.5% compared to baseline (MapTR), and ADMapv2 increased by 1.4% compared to baseline (MapTRv2). ADMapv2 has a maximum mAP of 82.8%, achieving the best performance among current benchmarks. Some details will be announced in subsequent arxiv versions. In terms of speed, ADMap significantly improves model performance compared to its baseline at a slightly lower FPS. It is worth mentioning that ADMapv2 not only improves performance, but also improves model inference speed.
Table 2 reports the metrics of ADMap and state-of-the-art methods in Argoverse2. Under the camera-only framework, ADMap and ADMapv2 improved by 3.4% and 1.3% respectively compared to the baseline. Under the multi-modal framework, ADMap and ADMapv2 achieved the best performance, with mAP of 75.2% and 76.9% respectively. In terms of speed. ADMapv2 improved by 11.4ms compared to MapTRv2.
Ablation Experiment
In Table 3, we provide ablation experiments for each module of ADMap on the nuScenes benchmark.
#Table 4 shows the impact of inserting different attention mechanisms on the final performance. DSA stands for decoupled self-attention, and IIA stands for instance interactive attention. The results show that IIA improves mAP by 1.3% compared to DSA.
Table 5 reports the impact of adding backbone and neck layers on mAP after merging features. After adding backbone and neck layers based on SECOND, mAP increased by 1.2%. After adding MPN, the mAP of the model increased by 2.0% without increasing the inference time.
Table 6 reports the performance impact of adding VDDL to the nuScenes benchmark. It can be seen that when the weight is set to 1.0, mAP is the highest, reaching 53.3%.
#Table 7 reports the impact of the number of MPN downsampling layers on the final performance in the nuScenes benchmark. The more downsampling layers, the slower the model inference speed. Therefore, to balance speed and performance, we set the number of downsampling layers to 2.
In order to verify that ADMap effectively alleviates the point order disturbance problem, we proposed the average chamfer distance (ACE). We picked predicted instances whose sum of chamfer distances is less than 1.5 and calculated their average chamfer distance (ACE). The smaller the ACE is, the more accurate the instance point order prediction is. Table 8 proves that ADMap can effectively alleviate the problem of point cloud disturbance.
Visualization results
The following two pictures are the visualization results of the nuScenes data set and the Argoverse2 data set.
Summary
ADMap is an efficient and effective vectorized high-precision map reconstruction framework, which effectively alleviates the jitter or aliasing phenomenon that may occur in the point order of instance vectors due to prediction bias. Extensive experiments show that our proposed method achieves the best performance on both nuScenes and Argoverse2 benchmarks. We believe that ADMap will help advance research on vector high-precision map reconstruction tasks, thereby better promoting the development of autonomous driving and other fields.
The above is the detailed content of ADMap: A new idea for anti-interference online high-precision maps. For more information, please follow other related articles on the PHP Chinese website!
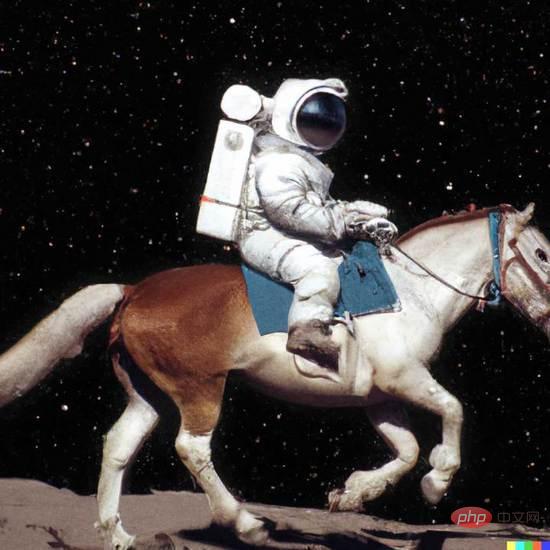
1 前言在发布DALL·E的15个月后,OpenAI在今年春天带了续作DALL·E 2,以其更加惊艳的效果和丰富的可玩性迅速占领了各大AI社区的头条。近年来,随着生成对抗网络(GAN)、变分自编码器(VAE)、扩散模型(Diffusion models)的出现,深度学习已向世人展现其强大的图像生成能力;加上GPT-3、BERT等NLP模型的成功,人类正逐步打破文本和图像的信息界限。在DALL·E 2中,只需输入简单的文本(prompt),它就可以生成多张1024*1024的高清图像。这些图像甚至
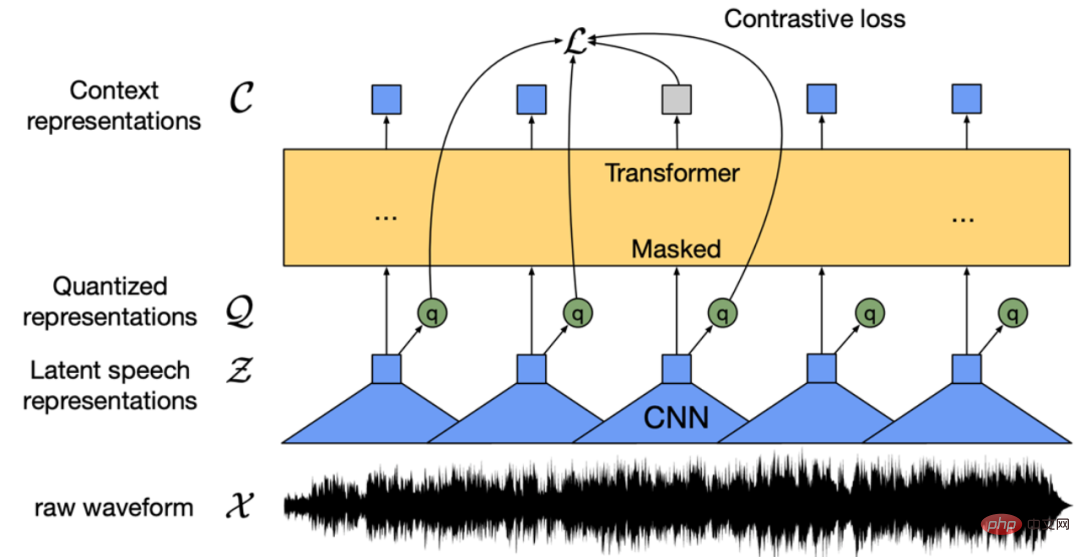
Wav2vec 2.0 [1],HuBERT [2] 和 WavLM [3] 等语音预训练模型,通过在多达上万小时的无标注语音数据(如 Libri-light )上的自监督学习,显著提升了自动语音识别(Automatic Speech Recognition, ASR),语音合成(Text-to-speech, TTS)和语音转换(Voice Conversation,VC)等语音下游任务的性能。然而这些模型都没有公开的中文版本,不便于应用在中文语音研究场景。 WenetSpeech [4] 是
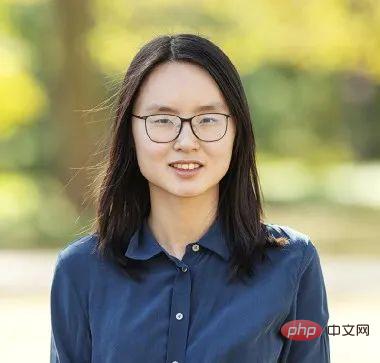
“Making large models smaller”这是很多语言模型研究人员的学术追求,针对大模型昂贵的环境和训练成本,陈丹琦在智源大会青源学术年会上做了题为“Making large models smaller”的特邀报告。报告中重点提及了基于记忆增强的TRIME算法和基于粗细粒度联合剪枝和逐层蒸馏的CofiPruning算法。前者能够在不改变模型结构的基础上兼顾语言模型困惑度和检索速度方面的优势;而后者可以在保证下游任务准确度的同时实现更快的处理速度,具有更小的模型结构。陈丹琦 普
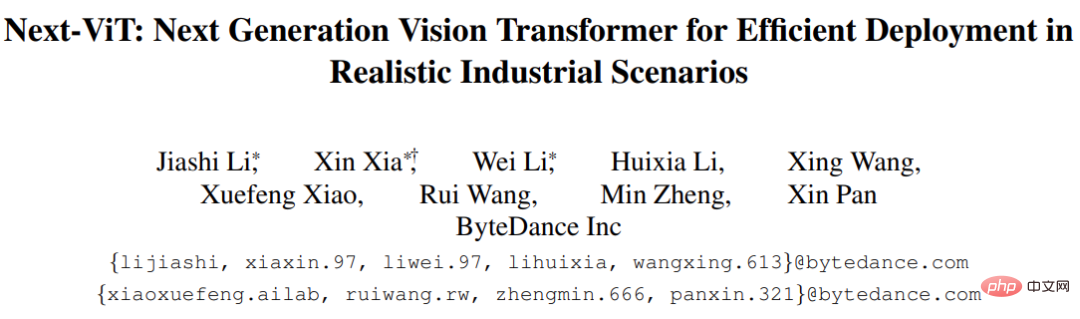
由于复杂的注意力机制和模型设计,大多数现有的视觉 Transformer(ViT)在现实的工业部署场景中不能像卷积神经网络(CNN)那样高效地执行。这就带来了一个问题:视觉神经网络能否像 CNN 一样快速推断并像 ViT 一样强大?近期一些工作试图设计 CNN-Transformer 混合架构来解决这个问题,但这些工作的整体性能远不能令人满意。基于此,来自字节跳动的研究者提出了一种能在现实工业场景中有效部署的下一代视觉 Transformer——Next-ViT。从延迟 / 准确性权衡的角度看,
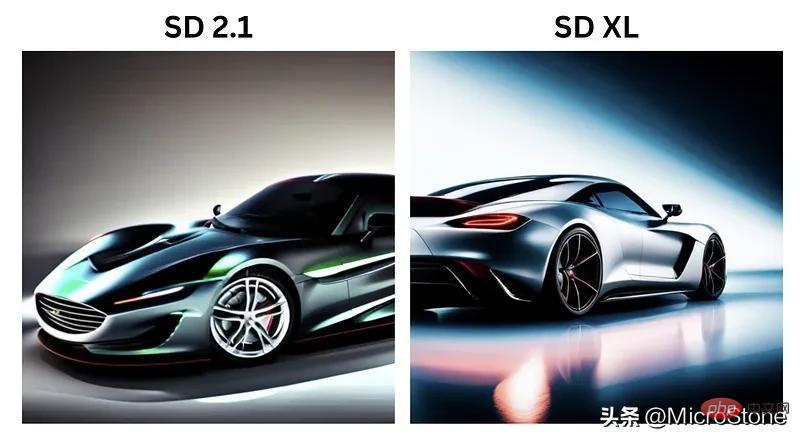
3月27号,Stability AI的创始人兼首席执行官Emad Mostaque在一条推文中宣布,Stable Diffusion XL 现已可用于公开测试。以下是一些事项:“XL”不是这个新的AI模型的官方名称。一旦发布稳定性AI公司的官方公告,名称将会更改。与先前版本相比,图像质量有所提高与先前版本相比,图像生成速度大大加快。示例图像让我们看看新旧AI模型在结果上的差异。Prompt: Luxury sports car with aerodynamic curves, shot in a
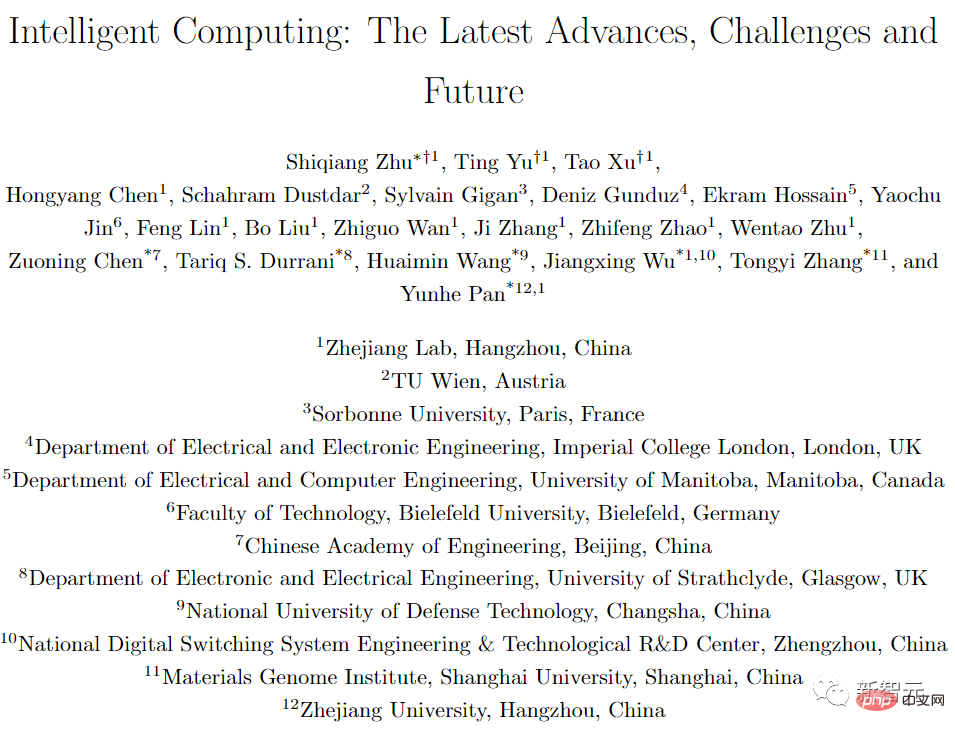
人工智能就是一个「拼财力」的行业,如果没有高性能计算设备,别说开发基础模型,就连微调模型都做不到。但如果只靠拼硬件,单靠当前计算性能的发展速度,迟早有一天无法满足日益膨胀的需求,所以还需要配套的软件来协调统筹计算能力,这时候就需要用到「智能计算」技术。最近,来自之江实验室、中国工程院、国防科技大学、浙江大学等多达十二个国内外研究机构共同发表了一篇论文,首次对智能计算领域进行了全面的调研,涵盖了理论基础、智能与计算的技术融合、重要应用、挑战和未来前景。论文链接:https://spj.scien
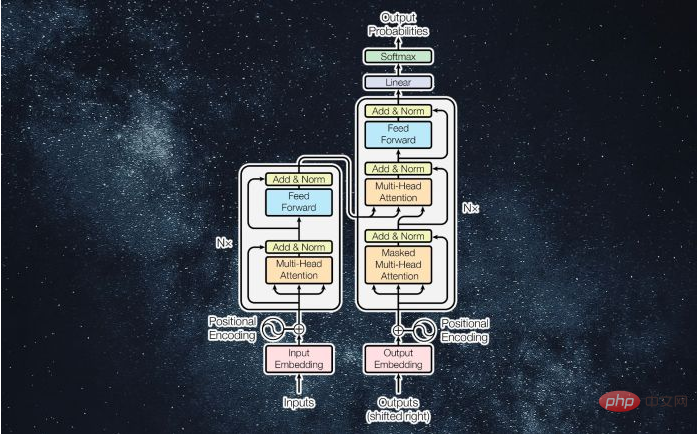
译者 | 李睿审校 | 孙淑娟近年来, Transformer 机器学习模型已经成为深度学习和深度神经网络技术进步的主要亮点之一。它主要用于自然语言处理中的高级应用。谷歌正在使用它来增强其搜索引擎结果。OpenAI 使用 Transformer 创建了著名的 GPT-2和 GPT-3模型。自从2017年首次亮相以来,Transformer 架构不断发展并扩展到多种不同的变体,从语言任务扩展到其他领域。它们已被用于时间序列预测。它们是 DeepMind 的蛋白质结构预测模型 AlphaFold
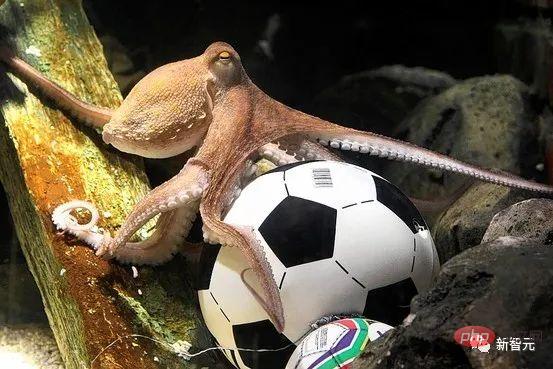
说起2010年南非世界杯的最大网红,一定非「章鱼保罗」莫属!这只位于德国海洋生物中心的神奇章鱼,不仅成功预测了德国队全部七场比赛的结果,还顺利地选出了最终的总冠军西班牙队。不幸的是,保罗已经永远地离开了我们,但它的「遗产」却在人们预测足球比赛结果的尝试中持续存在。在艾伦图灵研究所(The Alan Turing Institute),随着2022年卡塔尔世界杯的持续进行,三位研究员Nick Barlow、Jack Roberts和Ryan Chan决定用一种AI算法预测今年的冠军归属。预测模型图


Hot AI Tools

Undresser.AI Undress
AI-powered app for creating realistic nude photos

AI Clothes Remover
Online AI tool for removing clothes from photos.

Undress AI Tool
Undress images for free

Clothoff.io
AI clothes remover

AI Hentai Generator
Generate AI Hentai for free.

Hot Article

Hot Tools

SAP NetWeaver Server Adapter for Eclipse
Integrate Eclipse with SAP NetWeaver application server.

SublimeText3 Linux new version
SublimeText3 Linux latest version

MinGW - Minimalist GNU for Windows
This project is in the process of being migrated to osdn.net/projects/mingw, you can continue to follow us there. MinGW: A native Windows port of the GNU Compiler Collection (GCC), freely distributable import libraries and header files for building native Windows applications; includes extensions to the MSVC runtime to support C99 functionality. All MinGW software can run on 64-bit Windows platforms.
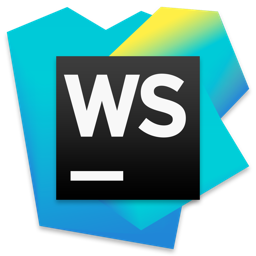
WebStorm Mac version
Useful JavaScript development tools
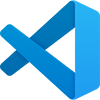
VSCode Windows 64-bit Download
A free and powerful IDE editor launched by Microsoft
