In this high-tech era, everyone must be familiar with generative artificial intelligence, or at least have heard of it. However, everyone always has concerns about the data generated by artificial intelligence, which has to involve data quality.
#In this modern era, everyone should be familiar with generative artificial intelligence, or at least have some understanding of it. However, there are still some concerns about the data generated by artificial intelligence, which has also led to discussions about data quality.
What is generative artificial intelligence?
Generative artificial intelligence is a type of artificial intelligence system whose main function is to generate new data, text, images, audio, etc., not just Analyze and process existing data. Generative artificial intelligence systems learn from large amounts of data and patterns to generate new content with certain logic and semantics, which is usually not seen in the training data.
Representative algorithms and models of generative artificial intelligence include:
- Generative Adversarial Network (GAN): GAN is a model composed of two neural networks, a generator network Responsible for generating new data samples, the discriminator network is responsible for evaluating the similarity between the generated samples and real data. Through adversarial training, the generator continuously improves the quality of generated data so that it approximates the real data distribution.
- Variational Autoencoder (VAE): VAE is a generative model that generates new data samples by learning the underlying distribution of the data. VAE combines the structure of the autoencoder and the idea of probabilistic generation model, which can generate data with certain variability.
- Autoregressive model: The autoregressive model gradually generates new data sequences by modeling sequence data. Typical autoregressive models include recurrent neural networks (RNN) and variants such as long short-term memory networks (LSTM) and gated recurrent units (GRU), as well as the latest transformer models (Transformer).
- Autoencoder (AE): An autoencoder is an unsupervised learning model that generates new data samples by learning a compressed representation of the data. Autoencoders can be generated by encoding input data into a low-dimensional representation and then decoding it into raw data samples.
Generative artificial intelligence is widely used in fields such as natural language generation, image generation, and music generation. It can be used to generate virtual artificial content, such as virtual character dialogue, artistic creation, video game environments, etc. It can also be used for content generation in augmented reality and virtual reality applications.
What is data quality?
Data quality refers to the attributes of data such as suitability, accuracy, completeness, consistency, timeliness and credibility during use. The quality of data directly affects the effectiveness of data analysis, mining and decision-making. Core aspects of data quality include data integrity, which ensures that the data is not missing or wrong; accuracy, which ensures that the data is correct and precise; consistency, which ensures that the data remains consistent across different systems; and timeliness, which ensures that the data is updated and Availability; Credibility, ensuring the data source is reliable and trustworthy. These aspects together constitute the basic standards of data quality, which are essential for ensuring data
- accuracy: Data accuracy refers to the degree to which the data is consistent with the real situation. Accurate data reflects the true state of the phenomenon or event of concern. Data accuracy is affected by data collection, input and processing.
- Integrity: Data integrity indicates whether the data contains all the required information, and whether the data is complete and not missing. Complete data can provide comprehensive information and avoid analysis bias caused by missing information.
- Consistency: Data consistency refers to whether the information in the data is consistent with each other without contradiction or conflict. Consistent data increases the credibility and reliability of the data.
- Timeliness: The timeliness of data indicates whether the data can be obtained and used in a timely manner when needed. Timely updated data can reflect the latest situation and contribute to the accuracy of decision-making and analysis.
- Credibility: The credibility of data indicates whether the source and quality of the data are credible, and whether the data has been verified and audited. Trustworthy data increases trust in data analysis and decision-making.
- Generality: The generality of the data indicates whether the data is universal and applicable, and whether it can meet the analysis and application of different scenarios and needs.
Data quality is an important indicator to measure the value and availability of data. High-quality data helps to improve the effectiveness and efficiency of data analysis and application, and is crucial to supporting data-driven decision-making and business processes. .
Can generative AI and data quality coexist?
Generative AI and data quality can coexist. In fact, data quality is critical to the performance and effectiveness of generative AI. . Generative AI models often require large amounts of high-quality data for training to produce accurate and smooth output. Poor data quality can result in unstable model training, inaccurate or biased output.
A variety of measures can be taken to ensure data quality, including but not limited to:
- Data cleaning: remove errors, anomalies or duplicates in the data to ensure data consistency and accuracy.
- Data annotation: Properly label and annotate the data to provide the supervision signals required for model training.
- Data balancing: Ensure that the number of samples in each category or distribution in the data set is balanced to avoid biasing the model against certain categories or situations.
- Data collection: Obtain high-quality data through diversified and representative data collection methods to ensure the model's generalization ability to different situations.
- Data privacy and security: Protect the privacy and security of user data and ensure that data processing and storage comply with relevant laws, regulations and privacy policies.
Although data quality is crucial to generative artificial intelligence, it is also important to note that generative artificial intelligence models can, to some extent, make up for the lack of data quality through large-scale data. . Therefore, even with limited data quality, it is still possible to improve the performance of generative AI by increasing the amount of data and using appropriate model architecture and training techniques. However, high-quality data is still one of the key factors to ensure model performance and effectiveness.
The above is the detailed content of Can generative AI and data quality coexist?. For more information, please follow other related articles on the PHP Chinese website!
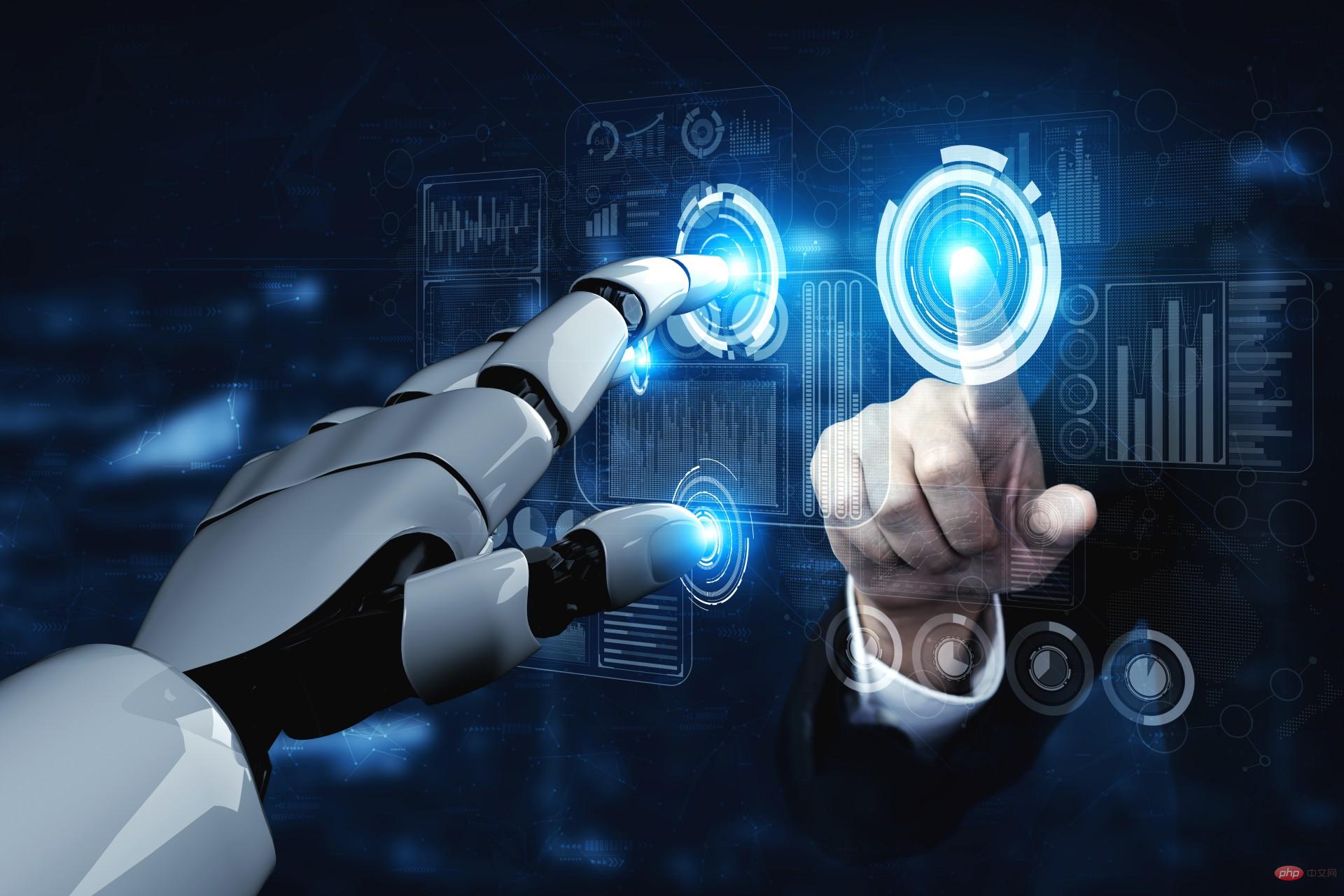
机器学习是一个不断发展的学科,一直在创造新的想法和技术。本文罗列了2023年机器学习的十大概念和技术。 本文罗列了2023年机器学习的十大概念和技术。2023年机器学习的十大概念和技术是一个教计算机从数据中学习的过程,无需明确的编程。机器学习是一个不断发展的学科,一直在创造新的想法和技术。为了保持领先,数据科学家应该关注其中一些网站,以跟上最新的发展。这将有助于了解机器学习中的技术如何在实践中使用,并为自己的业务或工作领域中的可能应用提供想法。2023年机器学习的十大概念和技术:1. 深度神经网

本文将详细介绍用来提高机器学习效果的最常见的超参数优化方法。 译者 | 朱先忠审校 | 孙淑娟简介通常,在尝试改进机器学习模型时,人们首先想到的解决方案是添加更多的训练数据。额外的数据通常是有帮助(在某些情况下除外)的,但生成高质量的数据可能非常昂贵。通过使用现有数据获得最佳模型性能,超参数优化可以节省我们的时间和资源。顾名思义,超参数优化是为机器学习模型确定最佳超参数组合以满足优化函数(即,给定研究中的数据集,最大化模型的性能)的过程。换句话说,每个模型都会提供多个有关选项的调整“按钮
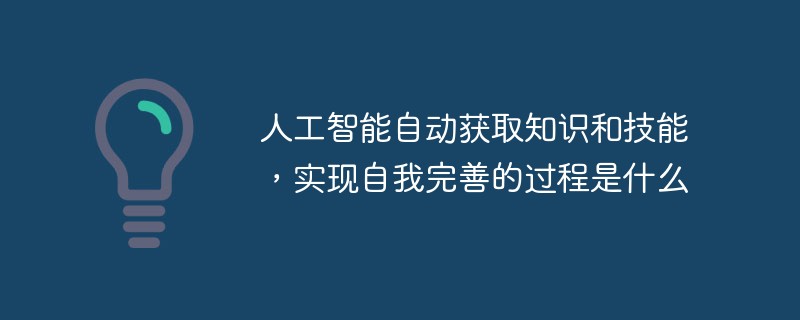
实现自我完善的过程是“机器学习”。机器学习是人工智能核心,是使计算机具有智能的根本途径;它使计算机能模拟人的学习行为,自动地通过学习来获取知识和技能,不断改善性能,实现自我完善。机器学习主要研究三方面问题:1、学习机理,人类获取知识、技能和抽象概念的天赋能力;2、学习方法,对生物学习机理进行简化的基础上,用计算的方法进行再现;3、学习系统,能够在一定程度上实现机器学习的系统。
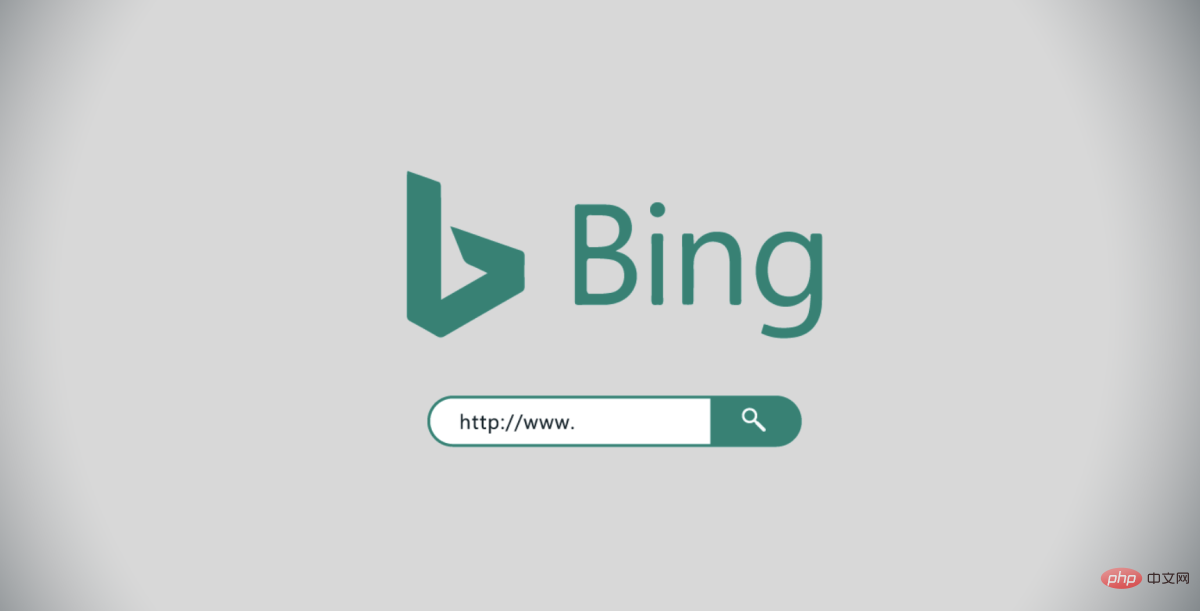
截至3月20日的数据显示,自微软2月7日推出其人工智能版本以来,必应搜索引擎的页面访问量增加了15.8%,而Alphabet旗下的谷歌搜索引擎则下降了近1%。 3月23日消息,外媒报道称,分析公司Similarweb的数据显示,在整合了OpenAI的技术后,微软旗下的必应在页面访问量方面实现了更多的增长。截至3月20日的数据显示,自微软2月7日推出其人工智能版本以来,必应搜索引擎的页面访问量增加了15.8%,而Alphabet旗下的谷歌搜索引擎则下降了近1%。这些数据是微软在与谷歌争夺生
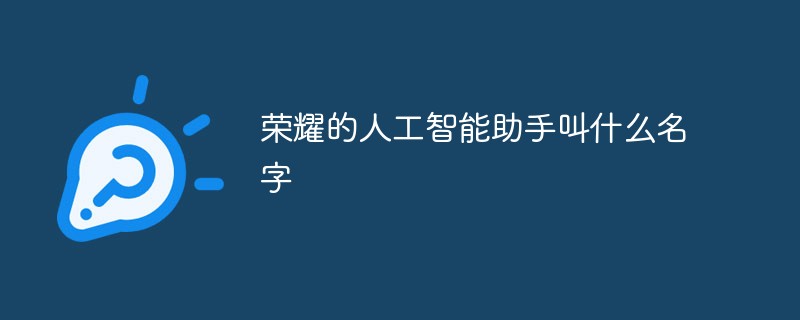
荣耀的人工智能助手叫“YOYO”,也即悠悠;YOYO除了能够实现语音操控等基本功能之外,还拥有智慧视觉、智慧识屏、情景智能、智慧搜索等功能,可以在系统设置页面中的智慧助手里进行相关的设置。
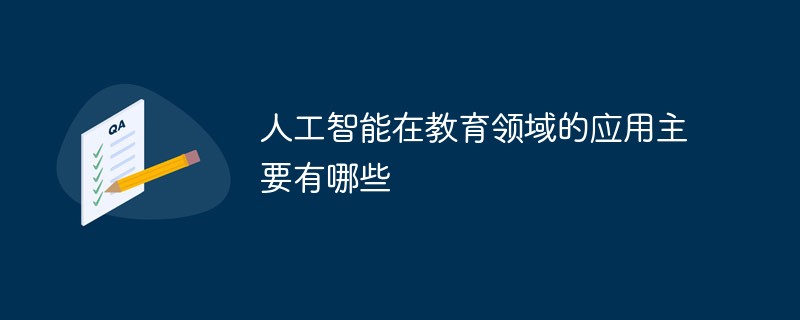
人工智能在教育领域的应用主要有个性化学习、虚拟导师、教育机器人和场景式教育。人工智能在教育领域的应用目前还处于早期探索阶段,但是潜力却是巨大的。
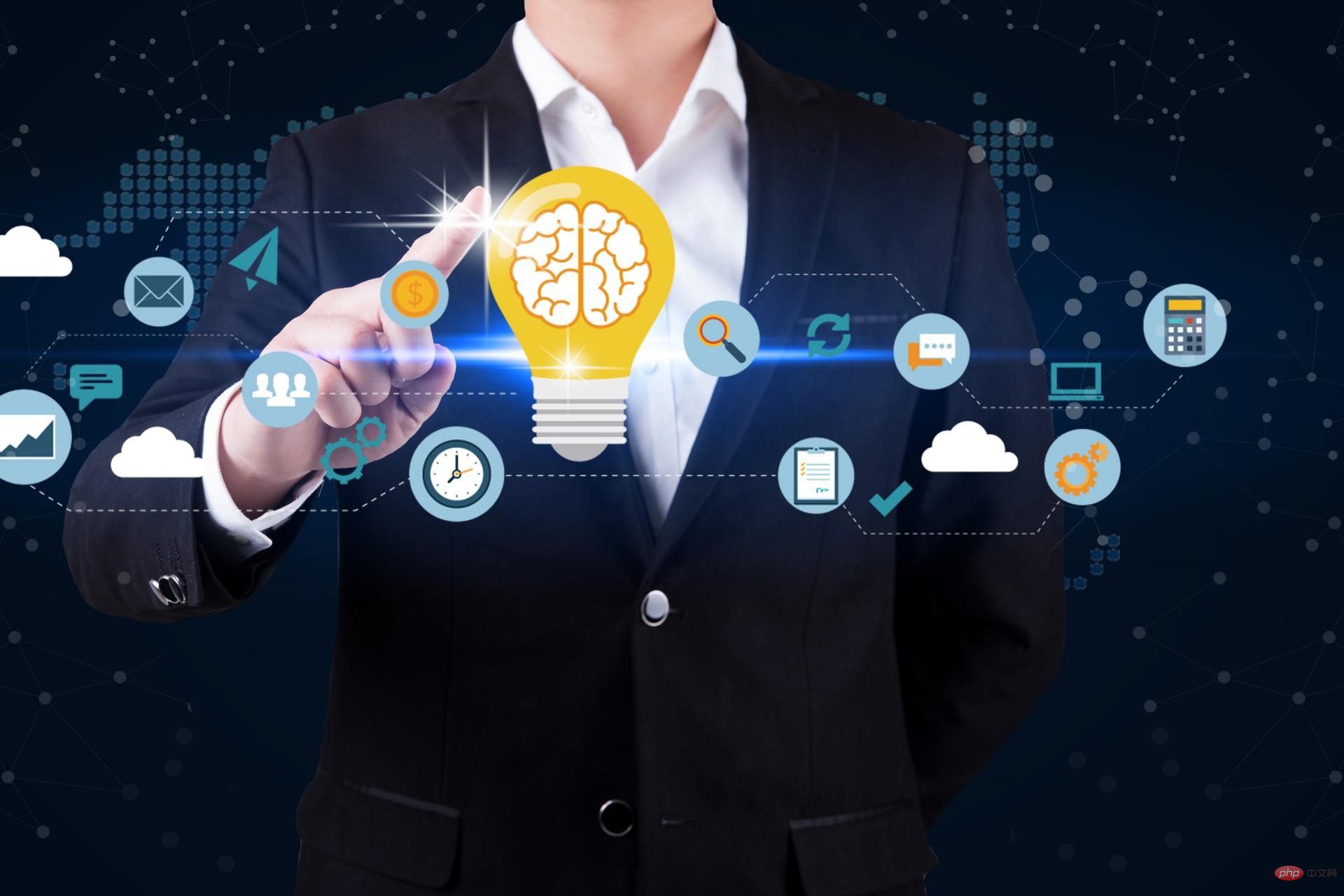
阅读论文可以说是我们的日常工作之一,论文的数量太多,我们如何快速阅读归纳呢?自从ChatGPT出现以后,有很多阅读论文的服务可以使用。其实使用ChatGPT API非常简单,我们只用30行python代码就可以在本地搭建一个自己的应用。 阅读论文可以说是我们的日常工作之一,论文的数量太多,我们如何快速阅读归纳呢?自从ChatGPT出现以后,有很多阅读论文的服务可以使用。其实使用ChatGPT API非常简单,我们只用30行python代码就可以在本地搭建一个自己的应用。使用 Python 和 C
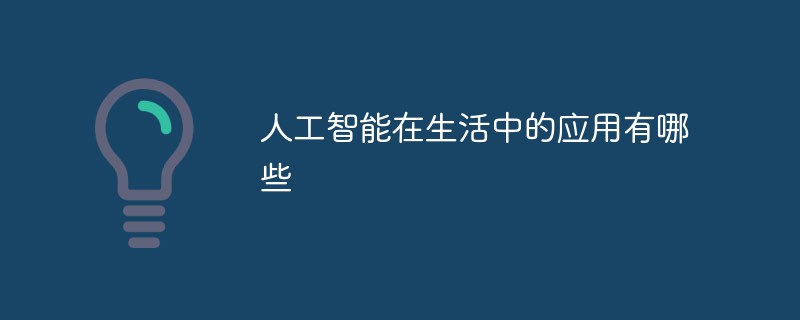
人工智能在生活中的应用有:1、虚拟个人助理,使用者可通过声控、文字输入的方式,来完成一些日常生活的小事;2、语音评测,利用云计算技术,将自动口语评测服务放在云端,并开放API接口供客户远程使用;3、无人汽车,主要依靠车内的以计算机系统为主的智能驾驶仪来实现无人驾驶的目标;4、天气预测,通过手机GPRS系统,定位到用户所处的位置,在利用算法,对覆盖全国的雷达图进行数据分析并预测。


Hot AI Tools

Undresser.AI Undress
AI-powered app for creating realistic nude photos

AI Clothes Remover
Online AI tool for removing clothes from photos.

Undress AI Tool
Undress images for free

Clothoff.io
AI clothes remover

AI Hentai Generator
Generate AI Hentai for free.

Hot Article

Hot Tools
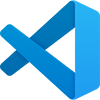
VSCode Windows 64-bit Download
A free and powerful IDE editor launched by Microsoft
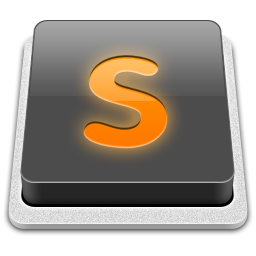
SublimeText3 Mac version
God-level code editing software (SublimeText3)

Zend Studio 13.0.1
Powerful PHP integrated development environment

mPDF
mPDF is a PHP library that can generate PDF files from UTF-8 encoded HTML. The original author, Ian Back, wrote mPDF to output PDF files "on the fly" from his website and handle different languages. It is slower than original scripts like HTML2FPDF and produces larger files when using Unicode fonts, but supports CSS styles etc. and has a lot of enhancements. Supports almost all languages, including RTL (Arabic and Hebrew) and CJK (Chinese, Japanese and Korean). Supports nested block-level elements (such as P, DIV),

SAP NetWeaver Server Adapter for Eclipse
Integrate Eclipse with SAP NetWeaver application server.
