


Python machine learning hyperparameter tuning: how to find the best model parameters
2. Why is hyperparameter tuning needed?
Different hyperparameter values may cause significant differences in model performance. For example, a learning rate that is too high may cause the model to oscillate or diverge during training, while a learning rate that is too low may cause the model to converge slowly. Therefore, it is necessary to find the optimal hyperparameter values through hyperparameter tuning to achieve the best performance of the model. 3. How to perform hyperparameter tuning?
Hyperparameter tuning is usually performed using methods such as grid search or random search. Grid search is a method of systematically searching for hyperparameter values. It sets the value of each hyperparameter to a set of predefined values, then trains and evaluates all possible combinations of hyperparameter values, and finally selects the performance Optimal hyperparameter values. Random search is a more flexible hyperparameter tuning method that searches for hyperparameter values through random sampling, then trains and evaluates these hyperparameter values, and finally selects the hyperparameter value with the best performance.
4. Tips for hyperparameter tuning
4.1 Using cross-validation
Cross-validation is a commonly used model evaluation method, which can help avoid overfitting and improve the generalization ability of the model. In hyperparameter tuning, the data set can be divided into multiple subsets, then different subsets are used to train and evaluate the model, and finally the results of all subsets are averaged to obtain the final performance evaluation result of the model.
4.2 Use early stopping
Early stopping is an effective technique to prevent overfitting. It can help the model automatically stop during the training process to avoid continuing training after the model reaches the best performance on the training set. The principle of early stopping is to stop training when the model's performance on the validation set no longer improves to prevent the model from overfitting on the training set.
4.3 Using Bayesian
OptimizationBayesian optimization is an optimization method based on Bayesian statistics, which can help find the best hyperparameter values in hyperparameter tuning. Bayesian optimization builds a probabilistic model of hyperparameter values and then continuously updates the model to find the best hyperparameter values.
4.4 Using automatic
Machine learningTools Automatic machine learning tools can help
automatethe entire process of hyperparameter tuning. It can automatically try different hyperparameter values and select the one with the best performance. Automatic machine learning tools can greatly simplify the process of hyperparameter tuning and improve the efficiency of hyperparameter tuning. 5. Example of hyperparameter tuning
# 导入必要的库 import numpy as np import pandas as pd from sklearn.model_selection import train_test_split from sklearn.svm import SVC # 加载数据集 data = pd.read_csv("data.csv") # 划分训练集和测试集 X = data.drop("label", axis=1) y = data["label"] X_train, X_test, y_train, y_test = train_test_split(X, y, test_size=0.2, random_state=0) # 定义超参数搜索空间 param_grid = { "C": [0.1, 1, 10, 100], "kernel": ["linear", "poly", "rbf", "sigmoid"] } # 创建网格搜索对象 grid_search = GridSearchCV(SVC(), param_grid, cv=5) # 执行网格搜索 grid_search.fit(X_train, y_train) # 选择最佳的超参数值 best_params = grid_search.best_params_ # 使用最佳的超参数值训练模型 model = SVC(**best_params) model.fit(X_train, y_train) # 评估模型的性能 score = model.score(X_test, y_test) print("模型的准确率为:", score)
This example demonstrates how to use the grid search method for hyperparameter tuning of a support vector machine (SVM) model. This example trains the model by setting a hyperparameter search space, then using a grid search object to search for hyperparameter values, and finally selecting the hyperparameter value with the best performance.
SummarizeHyperparameter tuning is a key step in optimizing model performance in machine learning. By adjusting the values of hyperparameters, you can find the best model parameters that take into account training accuracy and generalization ability. Hyperparameter tuning is usually performed using methods such as grid search or random search. In hyperparameter tuning, techniques such as cross-validation, early stopping, and Bayesian optimization can be used to improve the efficiency and accuracy of hyperparameter tuning.
The above is the detailed content of Python machine learning hyperparameter tuning: how to find the best model parameters. For more information, please follow other related articles on the PHP Chinese website!
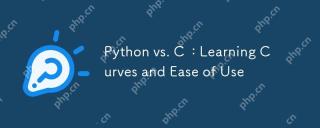
Python is easier to learn and use, while C is more powerful but complex. 1. Python syntax is concise and suitable for beginners. Dynamic typing and automatic memory management make it easy to use, but may cause runtime errors. 2.C provides low-level control and advanced features, suitable for high-performance applications, but has a high learning threshold and requires manual memory and type safety management.
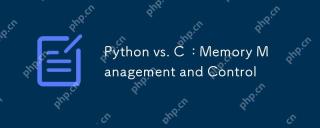
Python and C have significant differences in memory management and control. 1. Python uses automatic memory management, based on reference counting and garbage collection, simplifying the work of programmers. 2.C requires manual management of memory, providing more control but increasing complexity and error risk. Which language to choose should be based on project requirements and team technology stack.
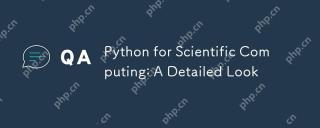
Python's applications in scientific computing include data analysis, machine learning, numerical simulation and visualization. 1.Numpy provides efficient multi-dimensional arrays and mathematical functions. 2. SciPy extends Numpy functionality and provides optimization and linear algebra tools. 3. Pandas is used for data processing and analysis. 4.Matplotlib is used to generate various graphs and visual results.
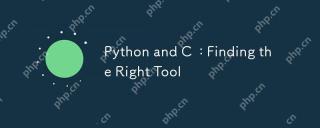
Whether to choose Python or C depends on project requirements: 1) Python is suitable for rapid development, data science, and scripting because of its concise syntax and rich libraries; 2) C is suitable for scenarios that require high performance and underlying control, such as system programming and game development, because of its compilation and manual memory management.
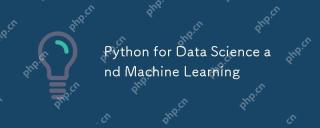
Python is widely used in data science and machine learning, mainly relying on its simplicity and a powerful library ecosystem. 1) Pandas is used for data processing and analysis, 2) Numpy provides efficient numerical calculations, and 3) Scikit-learn is used for machine learning model construction and optimization, these libraries make Python an ideal tool for data science and machine learning.
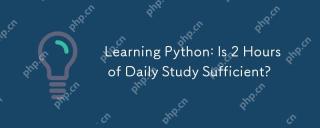
Is it enough to learn Python for two hours a day? It depends on your goals and learning methods. 1) Develop a clear learning plan, 2) Select appropriate learning resources and methods, 3) Practice and review and consolidate hands-on practice and review and consolidate, and you can gradually master the basic knowledge and advanced functions of Python during this period.
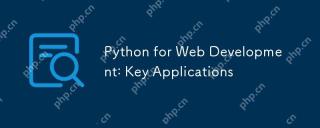
Key applications of Python in web development include the use of Django and Flask frameworks, API development, data analysis and visualization, machine learning and AI, and performance optimization. 1. Django and Flask framework: Django is suitable for rapid development of complex applications, and Flask is suitable for small or highly customized projects. 2. API development: Use Flask or DjangoRESTFramework to build RESTfulAPI. 3. Data analysis and visualization: Use Python to process data and display it through the web interface. 4. Machine Learning and AI: Python is used to build intelligent web applications. 5. Performance optimization: optimized through asynchronous programming, caching and code
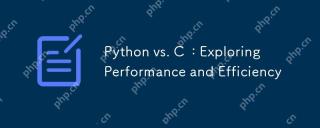
Python is better than C in development efficiency, but C is higher in execution performance. 1. Python's concise syntax and rich libraries improve development efficiency. 2.C's compilation-type characteristics and hardware control improve execution performance. When making a choice, you need to weigh the development speed and execution efficiency based on project needs.


Hot AI Tools

Undresser.AI Undress
AI-powered app for creating realistic nude photos

AI Clothes Remover
Online AI tool for removing clothes from photos.

Undress AI Tool
Undress images for free

Clothoff.io
AI clothes remover

Video Face Swap
Swap faces in any video effortlessly with our completely free AI face swap tool!

Hot Article

Hot Tools

SublimeText3 Linux new version
SublimeText3 Linux latest version
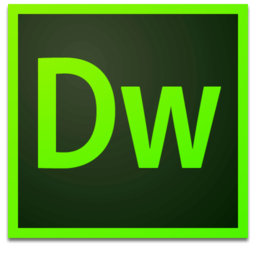
Dreamweaver Mac version
Visual web development tools
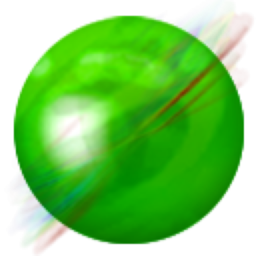
ZendStudio 13.5.1 Mac
Powerful PHP integrated development environment

SecLists
SecLists is the ultimate security tester's companion. It is a collection of various types of lists that are frequently used during security assessments, all in one place. SecLists helps make security testing more efficient and productive by conveniently providing all the lists a security tester might need. List types include usernames, passwords, URLs, fuzzing payloads, sensitive data patterns, web shells, and more. The tester can simply pull this repository onto a new test machine and he will have access to every type of list he needs.
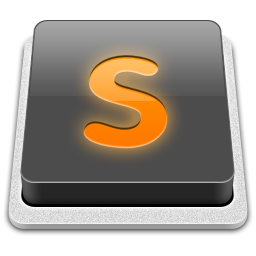
SublimeText3 Mac version
God-level code editing software (SublimeText3)