Don't underestimate IT fundamentals when prioritizing AI
GenAI still plays an important role in many enterprises’ IT projects. According to a Harris Poll, two-thirds of business leaders say they have adopted GenAI tools within their companies. IDC predicts that enterprise investment in GenAI will more than double by 2024.
Although the IT field continues to evolve, security, cost control, identity management, etc. are still key areas that CIOs need to pay attention to. These basic aspects are still critical to the organization.
It’s easy to view these as competing priorities vying for CIO attention and budgets that pale in comparison to the board’s interest in the new, shiny opportunities promised by Gen AI, but when it comes to When it comes to successfully implementing these projects, it turns out that they depend on how well the IT organization implements basic elements such as connectivity, permissions and configuration management.
Dion Hinchcliffe, vice president and principal analyst at Constellation Research, pointed out that it is critical to master the basics of today's IT, including a flexible multi-cloud foundation, strong network security, effective data privacy and Intellectual Property Control. These elements are critical to realizing the potential of AI and building strong cross-application silos and open data foundations. They are like the necessary planks and nails that keep the ship moving.
He likened the basic knowledge of IT to be like "eating green vegetables", which is ordinary but essential. It is crucial to the long-term health and strength of the IT industry. This balanced diet analogy shows that a strong and modern IT infrastructure is the basis for AI and other advanced technologies to thrive.
Andy Mann, founder of independent research analyst Sagable, agrees, noting that the internal operations and basic functions of IT infrastructure play a key role in driving AI development, akin to "pickaxes and shovels." ". He believes that CIOs need to ensure that AI workloads are effectively managed and run, especially considering that CIOs need to handle the various shielding and processing tasks required by regular applications.
Data Due Diligence
GenAI, in particular, has special implications for data security, Mann said. How do you implement data loss prevention when you tell your AI to ingest all this data and reuse it? In fact, for security, compliance and efficiency reasons, CIOs will want to carefully Managing what data GenAI can access, for example, Retrieval Augmented Generation (RAG) is becoming a key technology that can make LLM useful when working with your own data - but you don't want to enter all the data, it's not just preparation The cost of a larger dataset than you need, which requires some expertise that is still uncommon and demands a high salary, also includes what you teach the model. Enter your entire Slack or Teams history, and you'll likely get a response like, "I'll do this tomorrow," which is perfectly fine for a human employee, but not exactly what you'd expect from a GenAI system.
Christian Buckley, director of Microsoft MVP and Rencore Partner Management, pointed out that AI tools such as Copilot may reveal deficiencies in enterprise information management, including the structure of enterprise data and metadata, information architecture, content cleansing, and some enterprises Inadequacies in rights management and data organization.
As data storage costs drop, many businesses are retaining unnecessary data or cleaning up data that is outdated or no longer useful after a migration or reorganization. "People don't go back and clean up the trash because there's no cost - except for your risk profile and your search performance going down," Buckley said. He warned that introducing GenAI capabilities without considering data hygiene will leave people disillusioned when they are not properly prepared to perform optimally.
The same issues came to light when Microsoft launched Delve, the previous Rapid integration that brought powerful search capabilities to SharePoint in 2010. He said: "When we started seeing search actually work in SharePoint, people would complain that it didn't work properly, but that's what it does, and what it does is expose your lack of management of the data. I heard people say, 'This violates all my permissions', no, it surfaces where you have holes in your data, you want to use AI in your data to have more powerful search capabilities, and are not sure how you enterprise these Data?"
Another problem is that GenAI tools and users cannot see the information that should be included because metadata tags and sensitivity labels are not applied correctly to the data.
Either way, poorly managed data can raise compliance and confidentiality issues, such as when external partners gain access to Gen AI tools that expose information that should only be available internally. After all, projects involving more external users will require a closer look at what information is being accessed and whether this external access is still appropriate, for example if you are a multinational company with employees in France withdrawing from HR under Australia’s superannuation or parental leave policies Resource robots get information there, even for internal use, which can cause confusion.
Buckley said: “If you don’t know what content you have, where it is, who can access it internally and externally, what is being shared, and how it is labeled, how can you say you are ready to govern? What about AI. From a governance perspective, you need to track what people are doing, where they are doing it, and how they are doing it, and that will continue to change."
Containers and Certificates
Privilege management relies on identity management, which is another area that requires continued attention. As the container ecosystem evolves and greater adoption of Kubernetes requires new skills, many CIOs are still scrambling to manage environments for containerized applications that are significantly different from virtualization - as enterprises decide how to respond to Broadcom's Significant changes to VMware's licensing approach, a transition likely to accelerate this year.
It also means more machine identities to manage, said Murali Palanisamy, chief solutions officer at IAM platform provider AppViewX. "Digital transformation as a whole is driving a significant increase in the use of connected devices, cloud services, cloud-native and containerized applications," he said. "All these additional computers, workloads and services require trusted identities, which expands the need for The need for computer identity management."
The Internet of Things, software supply chain security - especially mitigating this need through code signing - and using your data for GenAI are increasing the reliance on TLS certificates for secure access and use of private keys. Palanisamy added: "Whenever applications or machines communicate with each other, the vast majority of them use TLS certificates to establish trust, identify each other to the system, and securely authenticate and encrypt communications."
He believes that protecting the identities of these machines is critical and managing them can no longer be an ad hoc, manual process, especially with Google proposing to reduce the validity period of TLS certificates from 398 days to 90 days. This needs to be faster There are other regulatory changes to note as well: the SEC’s new cybersecurity rules starting in December 2023, the EU’s expanded Network and Information Systems Directive (NIS2), and an overall shift to make security standards risk-based rather than Like the Payment Card Industry Data Security Standard (PCI-DSS) updated to PCI 4.0.
Palanisamy added that managing computer identities also needs to be a core security focus area, relying on automation, automatic enrollment and deprovisioning to control access to private and sensitive data. “Machine identity plays a vital role when data needs to be protected in transit,” he said. “As AI projects evolve, managing computer identity is critical to ensure trust as well as secure authentication and encryption. "So that the right access to the right data can be managed and controlled, ensuring the security of sensitive and private data. It's easy to think that cloud projects are a lower priority than shiny new AI initiatives," but he said these projects are actually the foundation. sexual. He added: "Speed and agility are necessary for the success of AI projects, so security needs to be integrated into the underlying cloud infrastructure of AI projects from the beginning."
cost control
Finop and cost control of cloud services remain a priority, and with so much GenAI usage relying on cloud AI services and APIs, CIOs will want to consider budgeting and automation, especially for AI development and experimentation.
"If you have 100 people doing AI experiments and just one person forgets to deprovision their instance, you're going to get a bill," Mann said. Production workloads should also be monitored to see if you You can scale down to smaller instances, cheaper LLMs, or lower-level licensing using the same strategies and tools you use to manage the costs of other workloads. "Managing Copilot is more than just permissions management," he added. "People want to know if the licenses they paid for are being used."
Just as other cloud workloads need to be provided to enterprises based on licenses and API calls Just like the value to justify their cost, GenAI projects also need to evaluate whether they actually deliver the productivity gains and innovation they promise.
"I'm waiting for the first CIO to be fired for letting AI run too fast, for too long," Mann said. Here's the basic CIO discipline of interception and processing: What's in my portfolio? What's the value, what do I get for my money, and the utilization and quality of managing these workloads, this ITSM, ITIL style of discipline and portfolio management is going to make a comeback because you definitely need this level of discipline to cope with this new workload.
But in other areas, IT teams will be looking to increase budgets and spending.
Accelerated Hardware Updates
With Windows 10 set to expire in 2025, CIOs will plan to migrate to Windows 11 over the next 18 months to get promised security improvements by default , means investing in new PCs with newer generation CPUs that have the right instructions to support security features without compromising performance.
More and more of these devices will include Neural Processing Units (NPUs) or similar specialized hardware to speed up AI workloads on the device, whether it's editing video calls in real time or running Copilot in Windows 11, but quickly Hardware advancements may mean CIOs need to budget for shorter hardware refresh cycles in the future to stay current, and asset management of tracking which employees have the right PC hardware may be key to getting the promised productivity gains from AI.
Getting your data center ready may mean more investment than just the price you can find on a GPU. While most LLM will run in the cloud and be accessed via APIs, making GenAI tools useful to the business requires connecting them to your own data sources.
Evolve your network architecture to reduce latency and securely deliver better connectivity - whether you do this through 5G, Wi-Fi 6 and 7, or emerging satellite connectivity - whether to support hybrid and long-range critical to the job, but AI will further drive secure edge computing and networking needs.
Additionally, falling prices are driving the transition to all-flash object storage systems that offer database performance that will be beneficial for processing that requires fast throughput, said Steve Leeper, vice president of product marketing at data management software company Datadobi. and scalability of large data sets necessary for AI workloads, and will also cater to the insatiable data appetite and rapid access needs of AI-driven operations.
Overall, he added, CIOs need to consider the hardware infrastructure of the AI processing pipeline, starting with the amount and type of storage, interconnection networks and GPU farms for AI processing, as well as data processing: Identifying the right ones Data sets, quickly and accurately relocate data between points on the processing pipeline - meaning no silent data corruption - and ensure that AI processing results are also relocated to the appropriate location and storage class.
GenAI’s data sets won’t always be huge, Leeper said. “There will be data sets, large and small,” he said, some of which contain critical data that will need to be processed using local resources depending on the enterprise’s governance policies. Managing AI access to these data sets is very much on the CIO. familiar kind of traditional IT infrastructure management, so investing in these infrastructures as a priority this year will pay off for both.
“These problems can only be solved if we apply the discipline we have,” Mann added, “but they often go unaddressed because no one considers the longer-term implications, so No ownership,” but that may be changing. At the beginning of 2023, Gartner reported that only 15% of enterprises already had data storage management solutions to classify and optimize data, but the analyst firm expects this proportion to rise to at least 40% by 2027.
The above is the detailed content of Don't underestimate IT fundamentals when prioritizing AI. For more information, please follow other related articles on the PHP Chinese website!
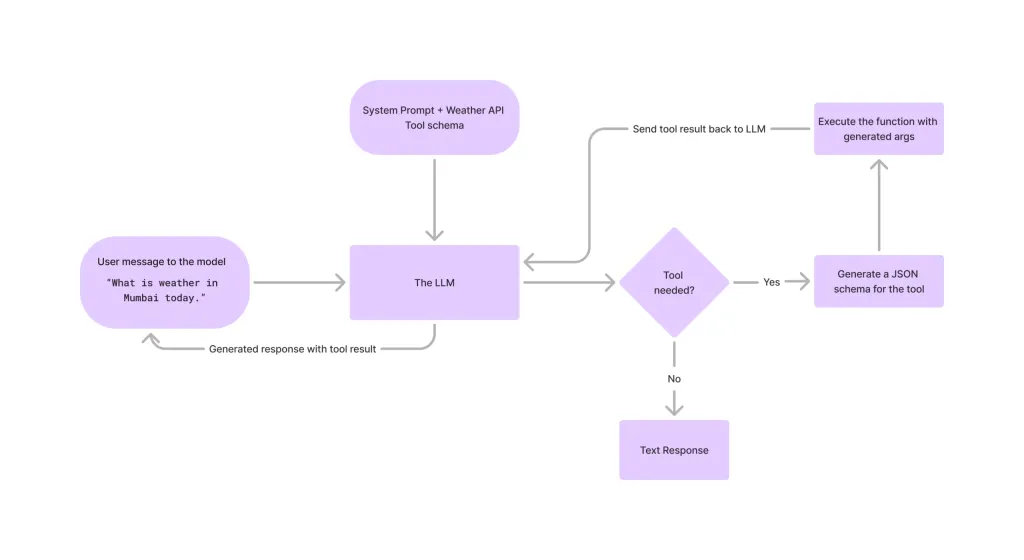
Large language models (LLMs) have surged in popularity, with the tool-calling feature dramatically expanding their capabilities beyond simple text generation. Now, LLMs can handle complex automation tasks such as dynamic UI creation and autonomous a
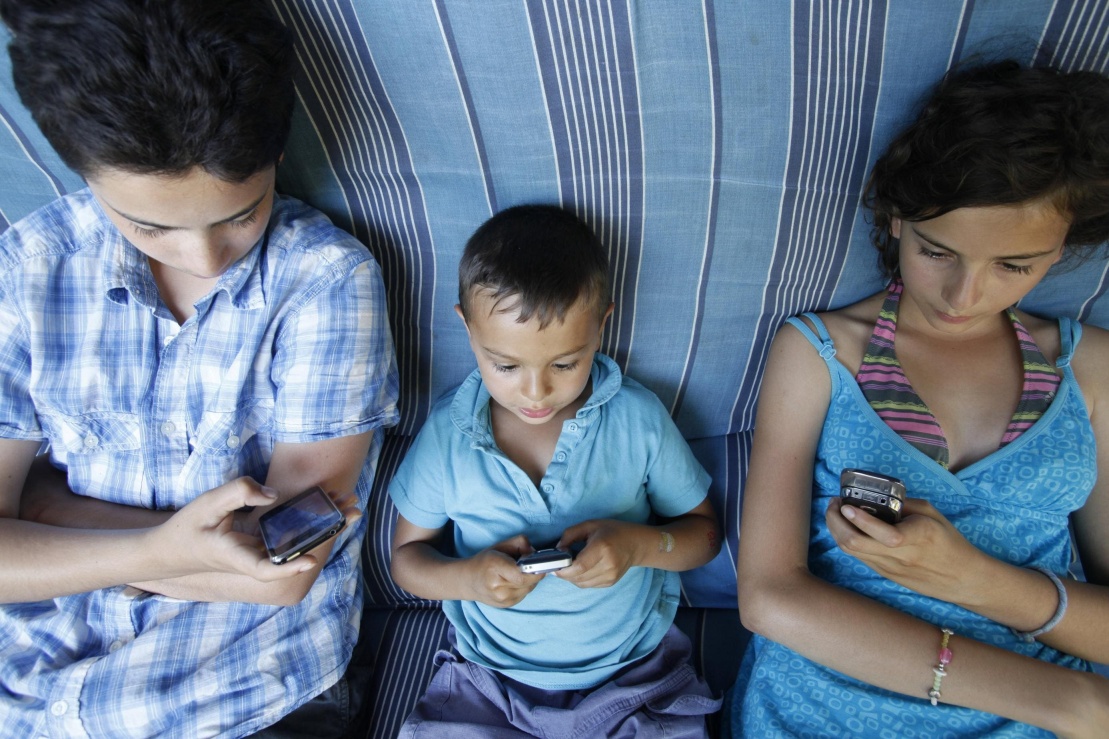
Can a video game ease anxiety, build focus, or support a child with ADHD? As healthcare challenges surge globally — especially among youth — innovators are turning to an unlikely tool: video games. Now one of the world’s largest entertainment indus
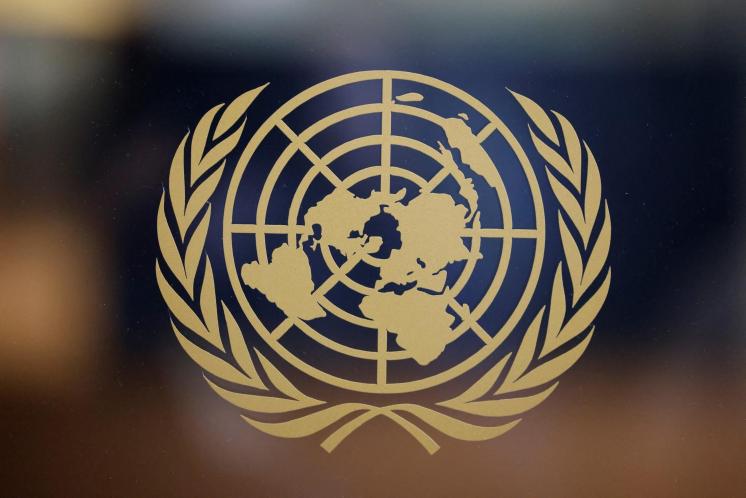
“History has shown that while technological progress drives economic growth, it does not on its own ensure equitable income distribution or promote inclusive human development,” writes Rebeca Grynspan, Secretary-General of UNCTAD, in the preamble.
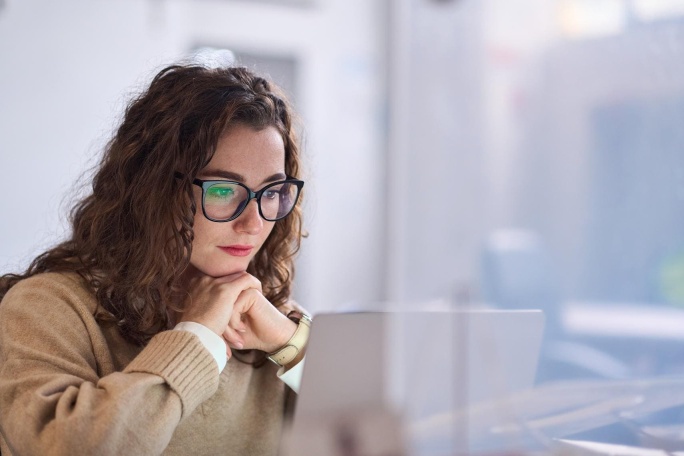
Easy-peasy, use generative AI as your negotiation tutor and sparring partner. Let’s talk about it. This analysis of an innovative AI breakthrough is part of my ongoing Forbes column coverage on the latest in AI, including identifying and explaining
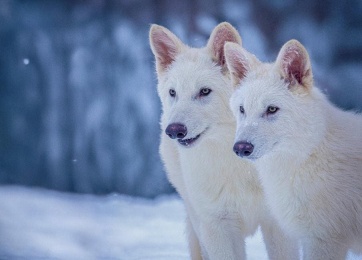
The TED2025 Conference, held in Vancouver, wrapped its 36th edition yesterday, April 11. It featured 80 speakers from more than 60 countries, including Sam Altman, Eric Schmidt, and Palmer Luckey. TED’s theme, “humanity reimagined,” was tailor made
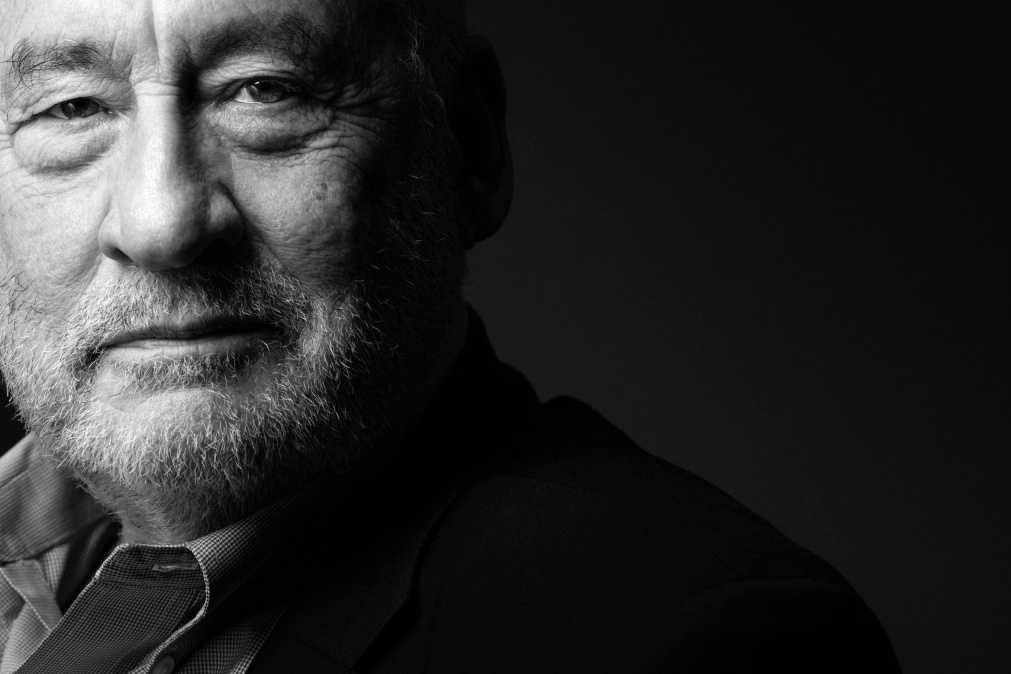
Joseph Stiglitz is renowned economist and recipient of the Nobel Prize in Economics in 2001. Stiglitz posits that AI can worsen existing inequalities and consolidated power in the hands of a few dominant corporations, ultimately undermining economic
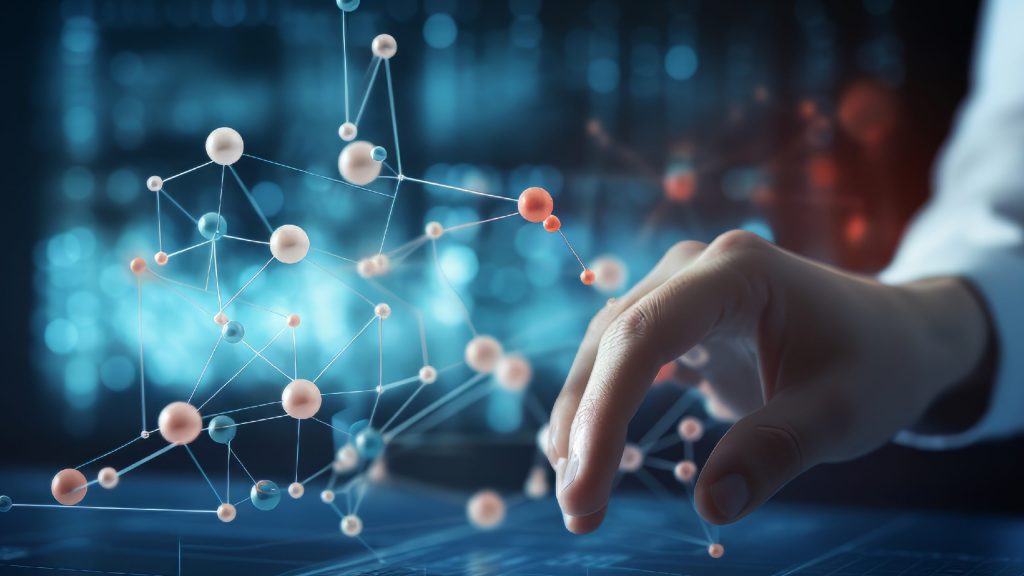
Graph Databases: Revolutionizing Data Management Through Relationships As data expands and its characteristics evolve across various fields, graph databases are emerging as transformative solutions for managing interconnected data. Unlike traditional
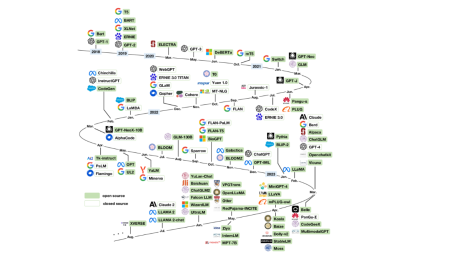
Large Language Model (LLM) Routing: Optimizing Performance Through Intelligent Task Distribution The rapidly evolving landscape of LLMs presents a diverse range of models, each with unique strengths and weaknesses. Some excel at creative content gen


Hot AI Tools

Undresser.AI Undress
AI-powered app for creating realistic nude photos

AI Clothes Remover
Online AI tool for removing clothes from photos.

Undress AI Tool
Undress images for free

Clothoff.io
AI clothes remover

AI Hentai Generator
Generate AI Hentai for free.

Hot Article

Hot Tools
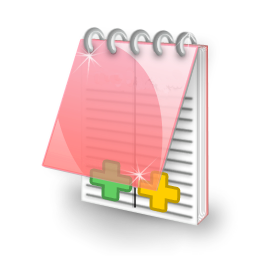
EditPlus Chinese cracked version
Small size, syntax highlighting, does not support code prompt function
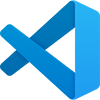
VSCode Windows 64-bit Download
A free and powerful IDE editor launched by Microsoft

MinGW - Minimalist GNU for Windows
This project is in the process of being migrated to osdn.net/projects/mingw, you can continue to follow us there. MinGW: A native Windows port of the GNU Compiler Collection (GCC), freely distributable import libraries and header files for building native Windows applications; includes extensions to the MSVC runtime to support C99 functionality. All MinGW software can run on 64-bit Windows platforms.

SublimeText3 Linux new version
SublimeText3 Linux latest version

DVWA
Damn Vulnerable Web App (DVWA) is a PHP/MySQL web application that is very vulnerable. Its main goals are to be an aid for security professionals to test their skills and tools in a legal environment, to help web developers better understand the process of securing web applications, and to help teachers/students teach/learn in a classroom environment Web application security. The goal of DVWA is to practice some of the most common web vulnerabilities through a simple and straightforward interface, with varying degrees of difficulty. Please note that this software