


High signal-to-noise ratio of fluorescence imaging is crucial for accurate visualization of biological phenomena, however, the noise issue remains one of the major challenges to imaging sensitivity.
The research team at Tsinghua University provides the spatial redundancy denoising Transformer (SRDTrans) to remove noise in fluorescence images in a self-supervised manner.
The team proposed a new sampling strategy to extract adjacent orthogonal training pairs based on spatial redundancy and eliminate the dependence on high imaging speed. In addition, they developed a lightweight spatiotemporal Transformer architecture capable of capturing distant dependencies and high-resolution features at low computational cost.
SRDTrans preserves high-frequency information without causing over-smoothing of structures or distortion of fluorescence traces. Furthermore, SRDTrans does not rely on specific imaging procedures and sample assumptions, making it suitable for expansion into various imaging modalities and biological applications.
The study was titled "Spatial redundancy transformer for self-supervised fluorescence image denoising" and was published in "Nature Computational Science" on December 11, 2023.
The rapid development of in vivo imaging technology allows researchers to observe biological structures and activities at the micron and even nanoscale. Fluorescence microscopy, as a popular imaging method, helps reveal new physiological and pathological mechanisms with its high spatiotemporal resolution and molecular specificity. The primary goal of fluorescence microscopy is to obtain clean, clear images that contain sufficient sample information to ensure the accuracy of downstream analysis and support confident conclusions.
However, due to the influence of various biophysical and biochemical factors, fluorescence imaging suffers from various limitations in practical operations. For example, the brightness, phototoxicity, and photobleaching of fluorophores can all have a negative impact on imaging results. In the case of photon limitation, the inherent photon shot noise can significantly reduce the signal-to-noise ratio (SNR) of the image, especially under low illumination and high-speed observation conditions. These factors make the quality and reliability of fluorescence imaging challenging and need to be overcome and optimized in practice.
A variety of methods have been proposed to remove noise in fluorescence images. Traditional denoising algorithms based on numerical filtering and mathematical optimization have unsatisfactory performance and limited applicability. In recent years, deep learning has shown remarkable achievements in the field of image denoising.
By iteratively training using ground truth (GT) datasets, deep neural networks are able to learn the mapping relationship between noisy images and their clean counterparts. The effectiveness of this supervision method mainly depends on the paired GT images.
Obtaining clean images with pixel-by-pixel registration is a huge challenge when observing the activity of living organisms because samples often undergo rapid dynamic changes. In order to alleviate this contradiction, some self-supervised methods have been proposed to achieve more applicable and practical denoising in fluorescence imaging.
In order to obtain better denoising performance, the ability to simultaneously extract global spatial information and long-range temporal correlation is crucial, and due to the locality of the convolution kernel, this is What neural networks (CNN) lack. In addition, the inherent spectral bias makes CNN tend to preferentially fit low-frequency features while ignoring high-frequency features, inevitably producing over-smooth denoising results.
The research team at Tsinghua University proposed the spatial redundancy denoising Transformer (SRDTrans) to solve these dilemmas.
Figure: SRDTrans principle and performance evaluation. (Source: paper)
On the one hand, the researchers proposed a spatially redundant sampling strategy to extract three-dimensional (3D) training from raw time-lapse data in two orthogonal directions right.
This scheme does not rely on the similarity between two adjacent frames, so SRDTrans is suitable for very fast activities and extremely low imaging speeds, which is consistent with what the team previously proposed DeepCAD exploiting temporal redundancy is complementary.
Since SRDTrans does not rely on any assumptions about contrast mechanisms, noise models, sample dynamics and imaging speed. Therefore, it can be easily extended to other biological samples and imaging modalities, such as membrane voltage imaging, single protein detection, light sheet microscopy, confocal microscopy, light field microscopy, and super-resolution microscopy.
On the other hand, the researchers designed a lightweight spatiotemporal transformation network to fully exploit long-range correlation. The optimized feature interaction mechanism enables the model to obtain high-resolution features with a small number of parameters. Compared with classic CNN, the proposed SRDTrans has stronger global perception and high-frequency maintenance capabilities, and is able to reveal fine-grained spatiotemporal patterns that were previously difficult to discern.
The team demonstrated the superior noise reduction performance of SRDTrans in two representative applications. The first is single-molecule localization microscopy (SMLM), where adjacent frames are random subsets of fluorophores.
Figure: Applying SRDTrans to experimental SMLM data. (Source: Paper)
The other is two-photon calcium imaging of large 3D neuronal populations at volumetric velocities as low as 0.3Hz. Extensive qualitative and quantitative results demonstrate that SRDTrans can serve as an essential denoising tool for fluorescence imaging to observe a variety of cellular and subcellular phenomena.
Figure: High-sensitivity calcium imaging of large neural volumes. (Source: paper)
SRDTrans also has some limitations, mainly in the basic assumption that adjacent pixels should have approximate structure. SRDTrans will fail if the spatial sampling rate is too low to provide sufficient redundancy. Another potential risk is the ability to generalize, as SRDTrans’ lightweight network architecture is better suited to specific tasks.
It is believed that training a specific model for specific data is the most reliable way to use deep learning for fluorescence image denoising. Therefore, new models should be trained to ensure optimal results when imaging parameters, modalities, and samples change.
As the development of fluorescent indicators moves toward faster kinetics, the imaging speed required to monitor biological dynamics at the millisecond level to record these rapid activities continues to grow. Obtaining sufficient sampling rates becomes increasingly challenging for denoising methods that rely on temporal redundancy. The team's perspective is to fill this gap by seeking to exploit spatial redundancy as an alternative to enable self-supervised denoising in more imaging applications.
Although the perfect case for spatially redundant sampling is a spatial sampling rate that is twice as high as diffraction-limited Nyquist sampling, thus ensuring that two adjacent pixels have nearly identical optical signals ; but in most cases, the endogenous similarity between the two spatially downsampled subsequences is sufficient to guide the training of the network.
However, this does not mean that the proposed spatial redundant sampling strategy can completely replace temporal redundant sampling, because ablation studies show that if equipped with the same network architecture, temporal redundancy Sampling enables better performance in high-speed imaging. The advantage of SRDTrans over DeepCAD at high imaging speeds is actually due to the Transformer architecture.
Generally speaking, spatial redundancy and temporal redundancy are two complementary sampling strategies that can achieve self-supervised training of fluorescence time-lapse imaging denoising networks. Which sampling strategy is used depends on which redundancy is greater in the data. It is worth noting that in many cases neither redundancy is sufficient to support current sampling strategies. The development of specific or more general self-supervised denoising methods will be of lasting value for fluorescence imaging.
Paper link: https://www.nature.com/articles/s43588-023-00568-2
The above is the detailed content of To remove noise in fluorescence images in a self-supervised manner, the Tsinghua team developed the spatial redundancy denoising Transformer method. For more information, please follow other related articles on the PHP Chinese website!
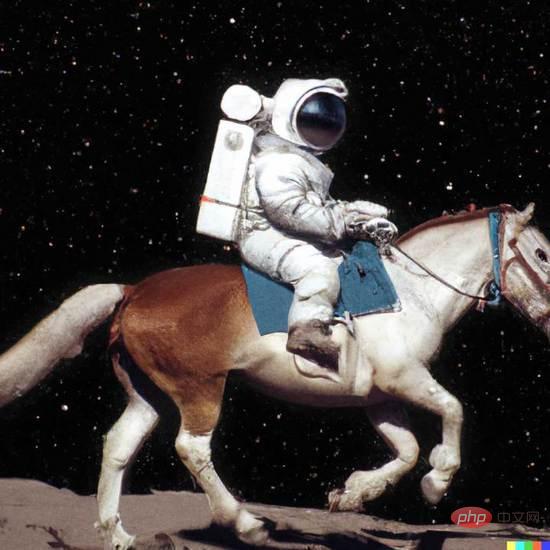
1 前言在发布DALL·E的15个月后,OpenAI在今年春天带了续作DALL·E 2,以其更加惊艳的效果和丰富的可玩性迅速占领了各大AI社区的头条。近年来,随着生成对抗网络(GAN)、变分自编码器(VAE)、扩散模型(Diffusion models)的出现,深度学习已向世人展现其强大的图像生成能力;加上GPT-3、BERT等NLP模型的成功,人类正逐步打破文本和图像的信息界限。在DALL·E 2中,只需输入简单的文本(prompt),它就可以生成多张1024*1024的高清图像。这些图像甚至
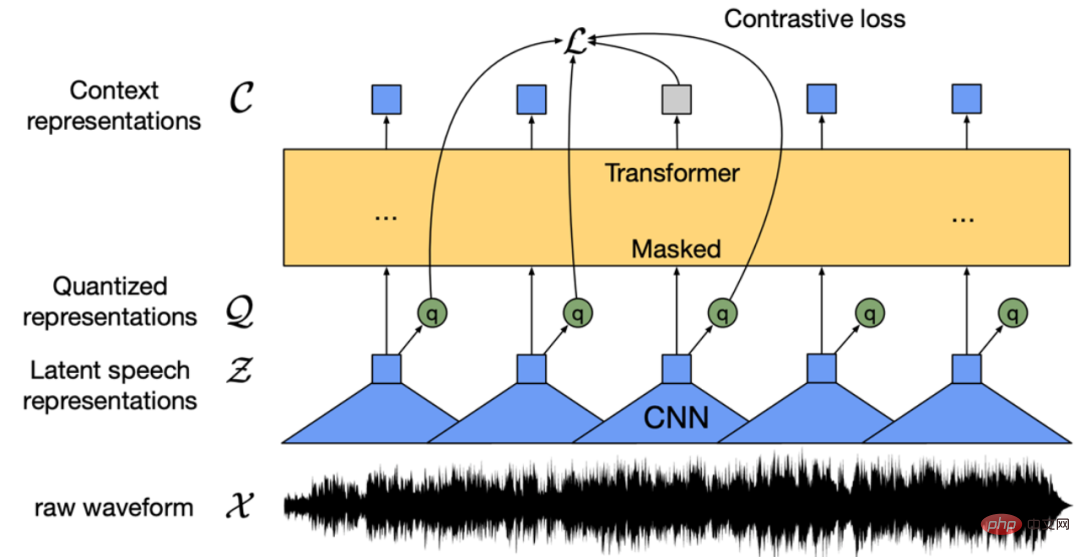
Wav2vec 2.0 [1],HuBERT [2] 和 WavLM [3] 等语音预训练模型,通过在多达上万小时的无标注语音数据(如 Libri-light )上的自监督学习,显著提升了自动语音识别(Automatic Speech Recognition, ASR),语音合成(Text-to-speech, TTS)和语音转换(Voice Conversation,VC)等语音下游任务的性能。然而这些模型都没有公开的中文版本,不便于应用在中文语音研究场景。 WenetSpeech [4] 是
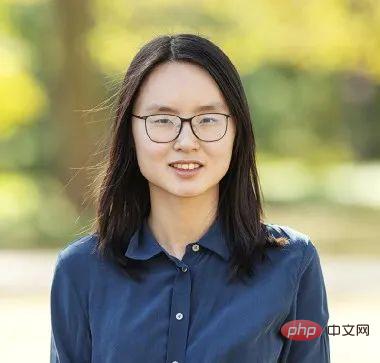
“Making large models smaller”这是很多语言模型研究人员的学术追求,针对大模型昂贵的环境和训练成本,陈丹琦在智源大会青源学术年会上做了题为“Making large models smaller”的特邀报告。报告中重点提及了基于记忆增强的TRIME算法和基于粗细粒度联合剪枝和逐层蒸馏的CofiPruning算法。前者能够在不改变模型结构的基础上兼顾语言模型困惑度和检索速度方面的优势;而后者可以在保证下游任务准确度的同时实现更快的处理速度,具有更小的模型结构。陈丹琦 普
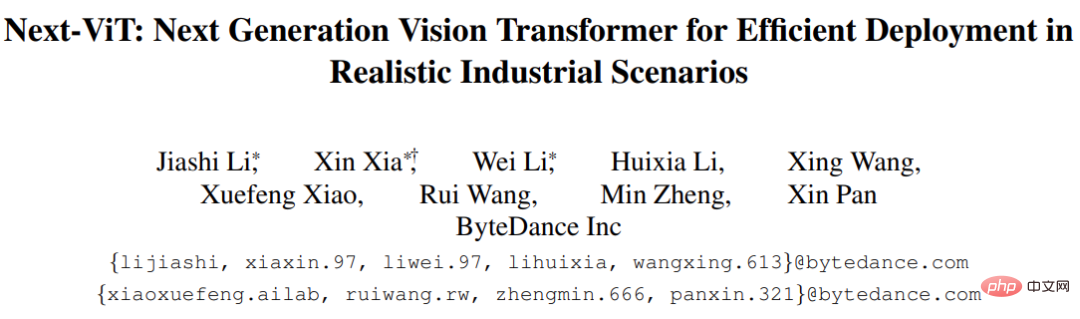
由于复杂的注意力机制和模型设计,大多数现有的视觉 Transformer(ViT)在现实的工业部署场景中不能像卷积神经网络(CNN)那样高效地执行。这就带来了一个问题:视觉神经网络能否像 CNN 一样快速推断并像 ViT 一样强大?近期一些工作试图设计 CNN-Transformer 混合架构来解决这个问题,但这些工作的整体性能远不能令人满意。基于此,来自字节跳动的研究者提出了一种能在现实工业场景中有效部署的下一代视觉 Transformer——Next-ViT。从延迟 / 准确性权衡的角度看,
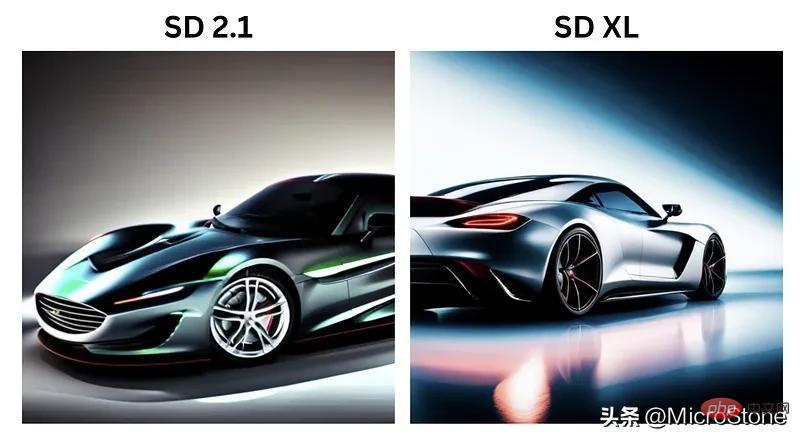
3月27号,Stability AI的创始人兼首席执行官Emad Mostaque在一条推文中宣布,Stable Diffusion XL 现已可用于公开测试。以下是一些事项:“XL”不是这个新的AI模型的官方名称。一旦发布稳定性AI公司的官方公告,名称将会更改。与先前版本相比,图像质量有所提高与先前版本相比,图像生成速度大大加快。示例图像让我们看看新旧AI模型在结果上的差异。Prompt: Luxury sports car with aerodynamic curves, shot in a
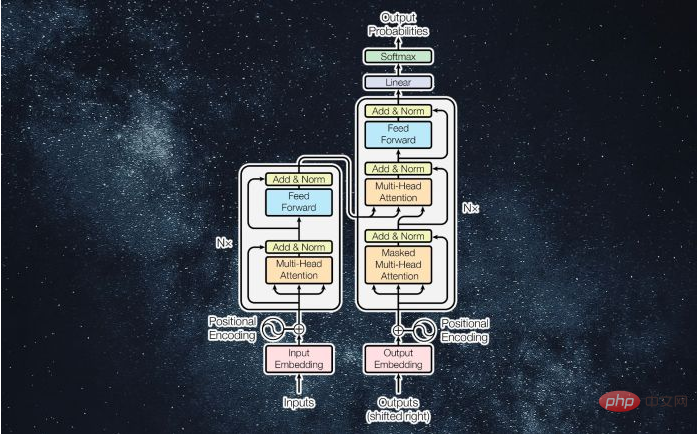
译者 | 李睿审校 | 孙淑娟近年来, Transformer 机器学习模型已经成为深度学习和深度神经网络技术进步的主要亮点之一。它主要用于自然语言处理中的高级应用。谷歌正在使用它来增强其搜索引擎结果。OpenAI 使用 Transformer 创建了著名的 GPT-2和 GPT-3模型。自从2017年首次亮相以来,Transformer 架构不断发展并扩展到多种不同的变体,从语言任务扩展到其他领域。它们已被用于时间序列预测。它们是 DeepMind 的蛋白质结构预测模型 AlphaFold
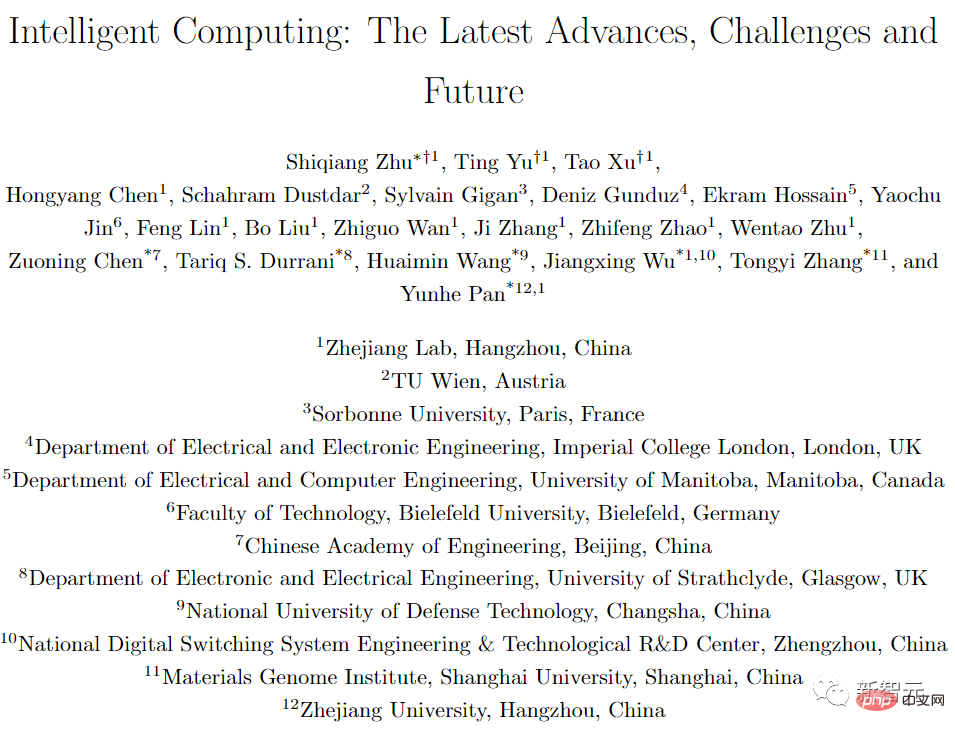
人工智能就是一个「拼财力」的行业,如果没有高性能计算设备,别说开发基础模型,就连微调模型都做不到。但如果只靠拼硬件,单靠当前计算性能的发展速度,迟早有一天无法满足日益膨胀的需求,所以还需要配套的软件来协调统筹计算能力,这时候就需要用到「智能计算」技术。最近,来自之江实验室、中国工程院、国防科技大学、浙江大学等多达十二个国内外研究机构共同发表了一篇论文,首次对智能计算领域进行了全面的调研,涵盖了理论基础、智能与计算的技术融合、重要应用、挑战和未来前景。论文链接:https://spj.scien
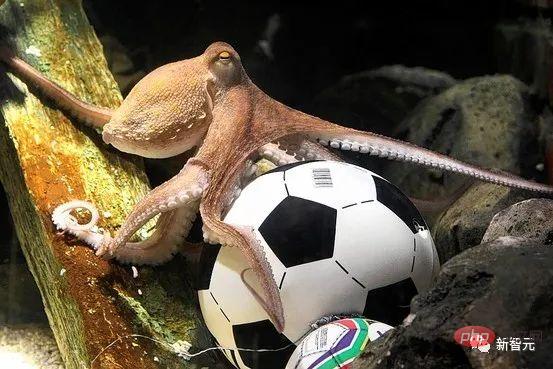
说起2010年南非世界杯的最大网红,一定非「章鱼保罗」莫属!这只位于德国海洋生物中心的神奇章鱼,不仅成功预测了德国队全部七场比赛的结果,还顺利地选出了最终的总冠军西班牙队。不幸的是,保罗已经永远地离开了我们,但它的「遗产」却在人们预测足球比赛结果的尝试中持续存在。在艾伦图灵研究所(The Alan Turing Institute),随着2022年卡塔尔世界杯的持续进行,三位研究员Nick Barlow、Jack Roberts和Ryan Chan决定用一种AI算法预测今年的冠军归属。预测模型图


Hot AI Tools

Undresser.AI Undress
AI-powered app for creating realistic nude photos

AI Clothes Remover
Online AI tool for removing clothes from photos.

Undress AI Tool
Undress images for free

Clothoff.io
AI clothes remover

AI Hentai Generator
Generate AI Hentai for free.

Hot Article

Hot Tools

SublimeText3 Linux new version
SublimeText3 Linux latest version

Atom editor mac version download
The most popular open source editor
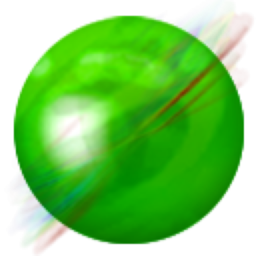
ZendStudio 13.5.1 Mac
Powerful PHP integrated development environment

Zend Studio 13.0.1
Powerful PHP integrated development environment
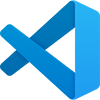
VSCode Windows 64-bit Download
A free and powerful IDE editor launched by Microsoft