


Data-oriented artificial intelligence can help reduce illusions and biases in generative AI systems, thereby improving the quality of their output.
Translated from The Paradigm Shift from Model-Centric to Data-Centric AI, author Rahul Pradhan has more than 16 years of experience and currently serves as the Vice President of Product and Strategy at Couchbase.
With the advancement of artificial intelligence (AI) such as transformer neural networks and generative adversarial networks (GAN), the technology field is undergoing a major transformation. Not only do these technologies hold enormous potential, they can also unlock innovation and creativity at scale. They can provide more accurate and efficient solutions and bring new business and development opportunities to various industries. The combination of transformer neural networks and GANs enables AI systems to better understand and generate human language, images and sounds, thereby promoting the development of natural language processing, computer vision and speech recognition and other fields. As these technologies become increasingly mature, we can expect more innovative applications and breakthroughs to emerge, bringing more to human society. As AI develops, data becomes critical. Data is the lifeblood that drives machine learning projects, turning concepts into practical insights. However, effectively leveraging data in AI projects is fraught with challenges, which hinders its adoption and realization of transformational value.
To enhance the development of AI, we are currently undergoing a paradigm shift from model-centric to data-centric AI transformation. The purpose of this shift is to reduce the hallucinations and biases that arise in generative adversarial network systems. By focusing on data-centric AI and bringing models closer to the data, we can improve the output of AI models and help enterprises realize their full potential. This shift will bring an important impetus to the development of AI.
Model-centered AI method
The traditional model-centered AI method is the main way of machine learning development. Its core idea is to improve the performance of the model through continuous iteration to generate the best model to handle the given data set. Researchers and engineers spend a lot of time fine-tuning the parameters, number of layers, and other architectural elements of the model. However, because building and fine-tuning models has been a complex and resource-intensive process in the past, requiring deep expertise to produce meaningful results, data has often been treated as a secondary factor. However, in recent years, with the advancement of machine learning technology and the enhancement of computing power, the importance of data has gradually been emphasized. Modern AI methods pay more attention to the quality and diversity of data, training models through larger data sets and more powerful computing power, thereby improving the performance and generalization capabilities of the model. This data-centric approach has become the current mainstream trend in the field of machine learning.
Transformation to data-centric AI
A data-centric approach improves the quality of data for model training, including cleaning, enhancing and ensuring that the data represents real-world scenarios.
As artificial intelligence (AI) models mature and expand in complexity, organizations need to focus on improving data quality and building closer alliances between models and data. In this evolving field, it is important to make a necessary and clear shift: bringing the model closer to the data, rather than transferring data to the model. This improves the quality of model output and reduces the illusions that often plague AI systems. A data-centric approach to AI is a cornerstone for organizations that want to deliver generative and predictive experiences based on the latest data.
Although data-centered AI is the direction of future development, model-centered AI still plays a key role in some scenarios. Model-centric AI is particularly important when data are limited or the goal is to explore model complexity and performance limits. It drives the frontiers of AI research and provides the possibility to solve problems where high-quality data is difficult to obtain. Therefore, model-centric AI is not just a supplement to data-driven AI, but an indispensable approach in the field of AI.
Reimagining AI with Data-Centric Thinking
By shifting to a data-centric approach to AI that ensures data quality and relevance, organizations can gain the following benefits:
Bridging reality by improving data quality
One of the typical benefits of a data-centric approach is the ability to deliver experiences that are tightly integrated with real-world scenarios. Unlike model-centric approaches where models often struggle with the fallacies of low-quality data, data-centric artificial intelligence (AI) seeks to bridge the gap between AI models and the dynamic reality they are trying to navigate.
Mitigating the Shadow of Illusions
AI hallucinations are primarily caused by defective data, characterized by the generation of incorrect or fictitious information. Moving to a data-centric approach can enhance the likelihood of reducing these errors. Training a model on a cleaner, more representative dataset produces more accurate and reliable output.
Unleashing the full potential of predictive and generative AI
With a solid foundation of high-quality data, organizations can unleash the full spectrum of AI’s predictive and generative capabilities. This shift makes AI more capable of interpreting existing data patterns while also generating new insights and experiences, fostering a culture of innovation and informed decision-making.
Leading the future of AI evolution with data
The transformation from model-centered to data-centered artificial intelligence (AI) methods represents a basic change in the way of thinking. This is putting data at the heart of the AI transformation journey. This shift is not just a technical tweak, but a conceptual recalibration that puts data at the heart of AI. As organizations embark on this path, they must cultivate a robust data infrastructure, develop data literacy, and create a culture that values data as the cornerstone of AI's promise.
Blending the best of both
Building powerful AI solutions requires a nuanced understanding of when to emphasize data and focus on model innovation. Balancing the benefits of model-centric and data-centric AI is critical to solving today's AI challenges so that organizations can get the most value from their AI projects. To help ensure that AI models are developed on the latest data and are accurate and reliable, organizations must embrace the transformation to data-centric AI.
The above is the detailed content of The artificial intelligence paradigm shifts from model-centric to data-centric. For more information, please follow other related articles on the PHP Chinese website!
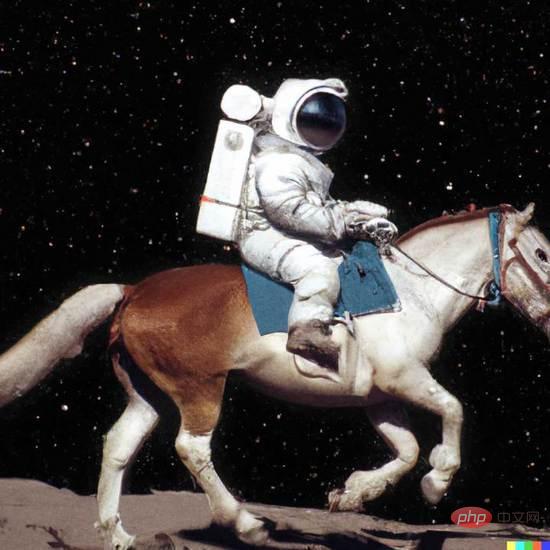
1 前言在发布DALL·E的15个月后,OpenAI在今年春天带了续作DALL·E 2,以其更加惊艳的效果和丰富的可玩性迅速占领了各大AI社区的头条。近年来,随着生成对抗网络(GAN)、变分自编码器(VAE)、扩散模型(Diffusion models)的出现,深度学习已向世人展现其强大的图像生成能力;加上GPT-3、BERT等NLP模型的成功,人类正逐步打破文本和图像的信息界限。在DALL·E 2中,只需输入简单的文本(prompt),它就可以生成多张1024*1024的高清图像。这些图像甚至
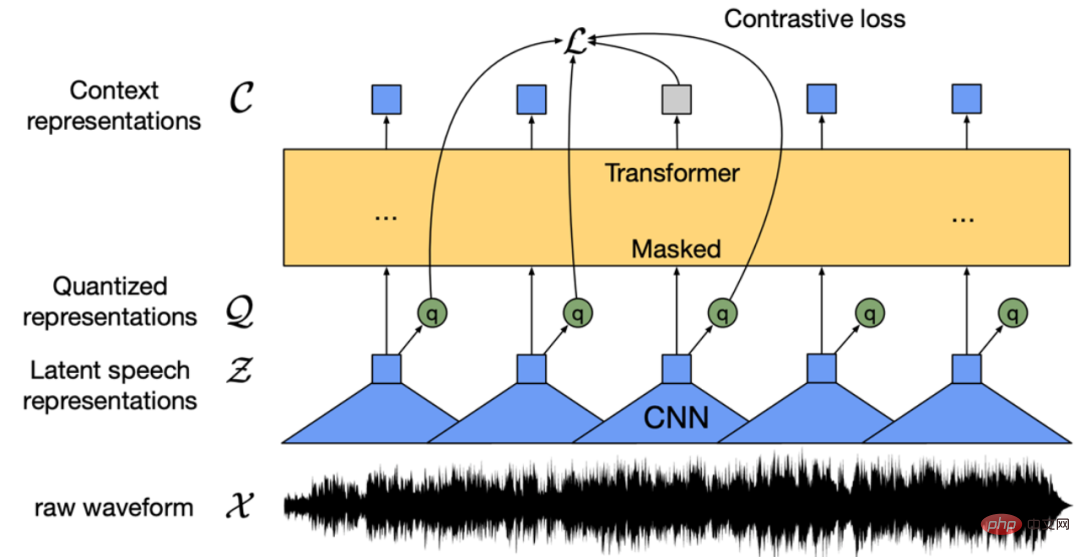
Wav2vec 2.0 [1],HuBERT [2] 和 WavLM [3] 等语音预训练模型,通过在多达上万小时的无标注语音数据(如 Libri-light )上的自监督学习,显著提升了自动语音识别(Automatic Speech Recognition, ASR),语音合成(Text-to-speech, TTS)和语音转换(Voice Conversation,VC)等语音下游任务的性能。然而这些模型都没有公开的中文版本,不便于应用在中文语音研究场景。 WenetSpeech [4] 是
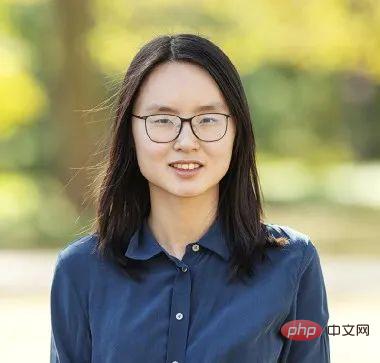
“Making large models smaller”这是很多语言模型研究人员的学术追求,针对大模型昂贵的环境和训练成本,陈丹琦在智源大会青源学术年会上做了题为“Making large models smaller”的特邀报告。报告中重点提及了基于记忆增强的TRIME算法和基于粗细粒度联合剪枝和逐层蒸馏的CofiPruning算法。前者能够在不改变模型结构的基础上兼顾语言模型困惑度和检索速度方面的优势;而后者可以在保证下游任务准确度的同时实现更快的处理速度,具有更小的模型结构。陈丹琦 普
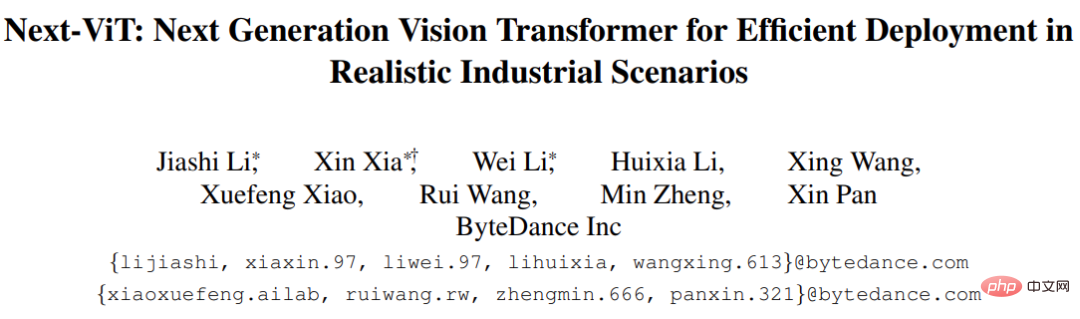
由于复杂的注意力机制和模型设计,大多数现有的视觉 Transformer(ViT)在现实的工业部署场景中不能像卷积神经网络(CNN)那样高效地执行。这就带来了一个问题:视觉神经网络能否像 CNN 一样快速推断并像 ViT 一样强大?近期一些工作试图设计 CNN-Transformer 混合架构来解决这个问题,但这些工作的整体性能远不能令人满意。基于此,来自字节跳动的研究者提出了一种能在现实工业场景中有效部署的下一代视觉 Transformer——Next-ViT。从延迟 / 准确性权衡的角度看,
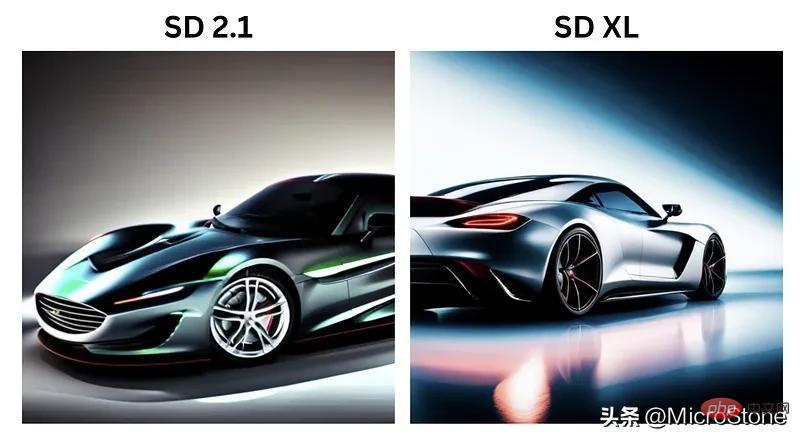
3月27号,Stability AI的创始人兼首席执行官Emad Mostaque在一条推文中宣布,Stable Diffusion XL 现已可用于公开测试。以下是一些事项:“XL”不是这个新的AI模型的官方名称。一旦发布稳定性AI公司的官方公告,名称将会更改。与先前版本相比,图像质量有所提高与先前版本相比,图像生成速度大大加快。示例图像让我们看看新旧AI模型在结果上的差异。Prompt: Luxury sports car with aerodynamic curves, shot in a
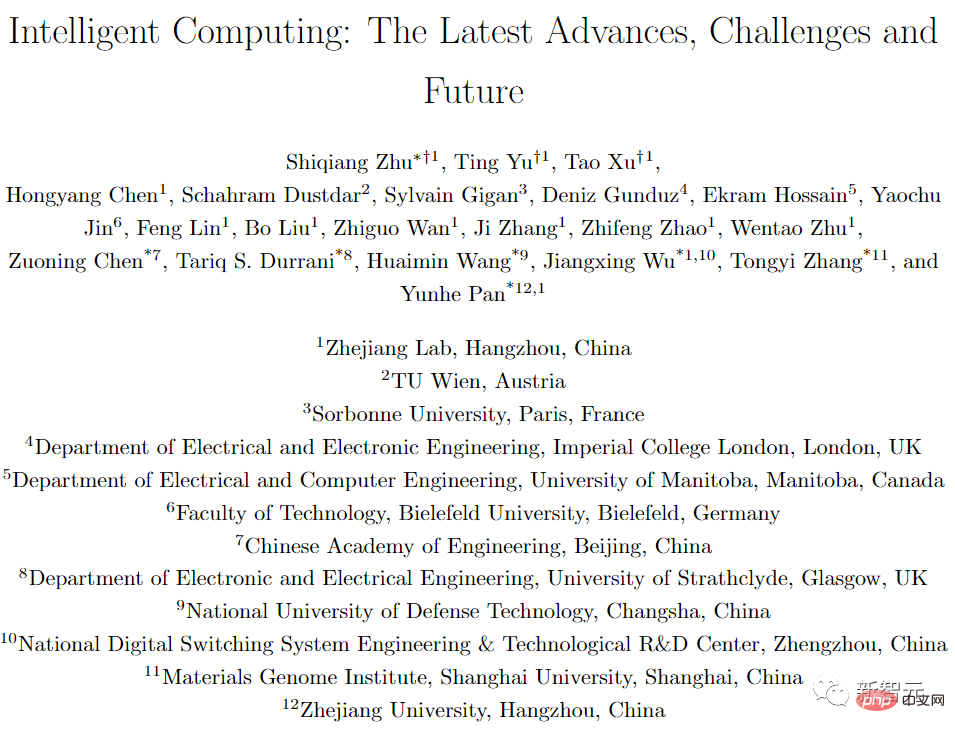
人工智能就是一个「拼财力」的行业,如果没有高性能计算设备,别说开发基础模型,就连微调模型都做不到。但如果只靠拼硬件,单靠当前计算性能的发展速度,迟早有一天无法满足日益膨胀的需求,所以还需要配套的软件来协调统筹计算能力,这时候就需要用到「智能计算」技术。最近,来自之江实验室、中国工程院、国防科技大学、浙江大学等多达十二个国内外研究机构共同发表了一篇论文,首次对智能计算领域进行了全面的调研,涵盖了理论基础、智能与计算的技术融合、重要应用、挑战和未来前景。论文链接:https://spj.scien
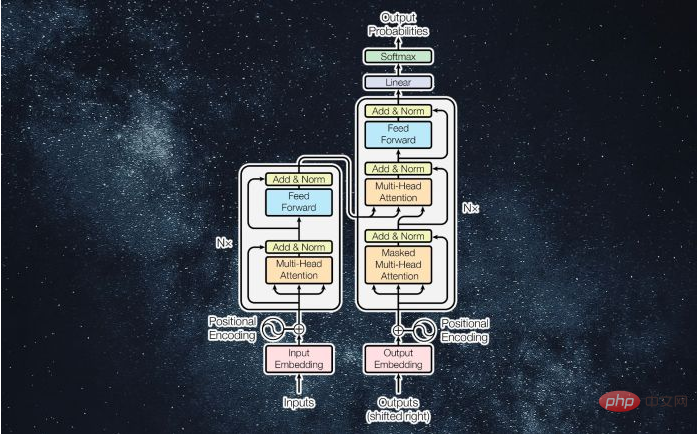
译者 | 李睿审校 | 孙淑娟近年来, Transformer 机器学习模型已经成为深度学习和深度神经网络技术进步的主要亮点之一。它主要用于自然语言处理中的高级应用。谷歌正在使用它来增强其搜索引擎结果。OpenAI 使用 Transformer 创建了著名的 GPT-2和 GPT-3模型。自从2017年首次亮相以来,Transformer 架构不断发展并扩展到多种不同的变体,从语言任务扩展到其他领域。它们已被用于时间序列预测。它们是 DeepMind 的蛋白质结构预测模型 AlphaFold
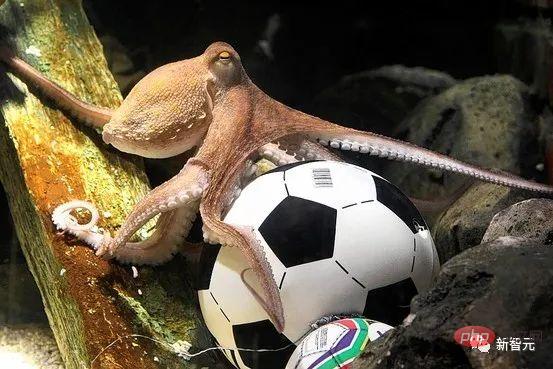
说起2010年南非世界杯的最大网红,一定非「章鱼保罗」莫属!这只位于德国海洋生物中心的神奇章鱼,不仅成功预测了德国队全部七场比赛的结果,还顺利地选出了最终的总冠军西班牙队。不幸的是,保罗已经永远地离开了我们,但它的「遗产」却在人们预测足球比赛结果的尝试中持续存在。在艾伦图灵研究所(The Alan Turing Institute),随着2022年卡塔尔世界杯的持续进行,三位研究员Nick Barlow、Jack Roberts和Ryan Chan决定用一种AI算法预测今年的冠军归属。预测模型图


Hot AI Tools

Undresser.AI Undress
AI-powered app for creating realistic nude photos

AI Clothes Remover
Online AI tool for removing clothes from photos.

Undress AI Tool
Undress images for free

Clothoff.io
AI clothes remover

AI Hentai Generator
Generate AI Hentai for free.

Hot Article

Hot Tools

Zend Studio 13.0.1
Powerful PHP integrated development environment
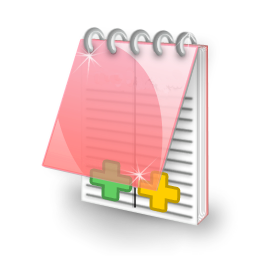
EditPlus Chinese cracked version
Small size, syntax highlighting, does not support code prompt function
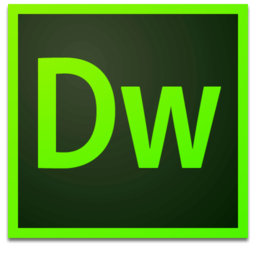
Dreamweaver Mac version
Visual web development tools

Atom editor mac version download
The most popular open source editor

mPDF
mPDF is a PHP library that can generate PDF files from UTF-8 encoded HTML. The original author, Ian Back, wrote mPDF to output PDF files "on the fly" from his website and handle different languages. It is slower than original scripts like HTML2FPDF and produces larger files when using Unicode fonts, but supports CSS styles etc. and has a lot of enhancements. Supports almost all languages, including RTL (Arabic and Hebrew) and CJK (Chinese, Japanese and Korean). Supports nested block-level elements (such as P, DIV),
