1. Product positioning of TensorRT-LLM
TensorRT-LLM is a scalable inference solution developed by NVIDIA for large language models (LLM). It builds, compiles and executes calculation graphs based on the TensorRT deep learning compilation framework, and draws on the efficient Kernels implementation in FastTransformer. In addition, it utilizes NCCL for communication between devices. Developers can customize operators to meet specific needs based on technology development and demand differences, such as developing customized GEMM based on cutlass. TensorRT-LLM is NVIDIA's official inference solution, committed to providing high performance and continuously improving its practicality.
TensorRT-LLM is open source on GitHub and is divided into two branches: Release branch and Dev branch. The Release branch is updated once a month, while the Dev branch will update features from official or community sources more frequently to facilitate developers to experience and evaluate the latest features. The figure below shows the framework structure of TensorRT-LLM. Except for the green TensorRT compilation part and the kernels involving hardware information, other parts are open source.
TensorRT-LLM also provides an API similar to Pytorch to reduce developers’ learning costs, and provides many predefined models for User use.
Due to the large size of large language models, inference may not be completed on a single graphics card, so TensorRT-LLM provides two parallel mechanisms : Tensor Parallelism and Pipeline Parallelism to support multi-card or multi-machine reasoning. These mechanisms allow the model to be split into multiple parts and distributed across multiple graphics cards or machines for parallel computation to improve inference performance. Tensor Parallelism achieves parallel computing by distributing model parameters on different devices and calculating the output of different parts at the same time. Pipeline Parallelism divides the model into multiple stages, each stage is calculated in parallel on different devices, and the output is passed to the next stage, thereby achieving the overall
2. Important features of TensorRT-LLM
TensorRT-LLM is a powerful tool , with rich model support and low-precision inference capabilities. First of all, TensorRT-LLM supports mainstream large language models, including model adaptation completed by developers, such as Qwen (Qianwen), and has been included in official support. This means that users can easily extend or customize these predefined models and apply them to their own projects quickly and easily. Secondly, TensorRT-LLM uses the FP16/BF16 precision inference method by default. This low-precision reasoning can not only improve reasoning performance, but also use the industry's quantization methods to further optimize hardware throughput. By reducing the accuracy of the model, TensorRT-LLM can greatly improve the speed and efficiency of inference without sacrificing too much accuracy. In summary, TensorRT-LLM's rich model support and low-precision inference capabilities make it a very practical tool. Whether for developers or researchers, TensorRT-LLM can provide efficient inference solutions to help them achieve better performance in deep learning applications.
Another feature is the implementation of FMHA (fused multi-head attention) kernel. Since the most time-consuming part of Transformer is the calculation of self-attention, the official designed FMHA to optimize the calculation of self-attention, and provided different versions with accumulators of fp16 and fp32. In addition, in addition to the improvement in speed, the memory usage is also greatly reduced. We also provide a flash attention-based implementation that can extend sequence-length to arbitrary lengths.
The following is the detailed information of FMHA, where MQA is Multi Query Attention and GQA is Group Query Attention.
Another Kernel is MMHA (Masked Multi-Head Attention). FMHA is mainly used for calculations in the context phase, while MMHA mainly provides acceleration of attention in the generation phase and provides support for Volta and subsequent architectures. Compared with the implementation of FastTransformer, TensorRT-LLM is further optimized and the performance is improved by up to 2x.
#Another important feature is quantization technology, which achieves inference acceleration with lower precision. Commonly used quantization methods are mainly divided into PTQ (Post Training Quantization) and QAT (Quantization-aware Training). For TensorRT-LLM, the reasoning logic of these two quantization methods is the same. For LLM quantification technology, an important feature is the co-design of algorithm design and engineering implementation, that is, the characteristics of the hardware must be considered at the beginning of the design of the corresponding quantification method. Otherwise, the expected inference speed improvement may not be achieved.
The PTQ quantification steps in TensorRT are generally divided into the following steps. First, quantify the model, and then convert the weights and model into TensorRT-LLM. express. For some customized operations, users also need to write their own kernels. Commonly used PTQ quantification methods include INT8 weight-only, SmoothQuant, GPTQ and AWQ, which are typical co-design methods.
INT8 weight-only directly quantizes the weight to INT8, but the activation value remains as FP16. The advantage of this method is that model storage is reduced by 2x and the storage bandwidth for loading weights is halved, achieving the purpose of improving inference performance. This method is called W8A16 in the industry, that is, the weight is INT8 and the activation value is FP16/BF16 - stored with INT8 precision and calculated in FP16/BF16 format. This method is intuitive, does not change weights, is easy to implement, and has good generalization performance.
The second quantification method is SmoothQuant, which was jointly designed by NVIDIA and the community. It is observed that weights usually obey Gaussian distribution and are easy to quantize, but there are outliers in the activation values, and the utilization of quantization bits is not high.
SmoothQuant compresses the corresponding distribution by first smoothing the activation value, that is, dividing it by a scale. At the same time, in order to ensure equivalence, it is necessary to The weights are multiplied by the same scale. Afterwards, both weights and activations can be quantified. The corresponding storage and calculation precision can be INT8 or FP8, and INT8 or FP8 TensorCore can be used for calculation. In terms of implementation details, weights support Per-tensor and Per-channel quantization, and activation values support Per-tensor and Per-token quantification.
The third quantization method is GPTQ, a layer-by-layer quantization method achieved by minimizing the reconstruction loss. GPTQ is a weight-only method, and the calculation uses the FP16 data format. This method is used when quantizing large models. Since quantization itself is relatively expensive, the author designed some tricks to reduce the cost of quantization itself, such as Lazy batch-updates and quantizing the weights of all rows in the same order. GPTQ can also be used in conjunction with other methods such as grouping strategies. Moreover, TensorRT-LLM provides different implementation optimization performance for different situations. Specifically, when the batch size is small, cuda core is used to implement it; conversely, when the batch size is large, tensor core is used to implement it.
The fourth quantization method is AWQ. This method considers that not all weights are equally important, only 0.1%-1% of the weights (salient weights) contribute more to the model accuracy, and these weights depend on the activation value distribution rather than the weight distribution. The quantification process of this method is similar to SmoothQuant. The main difference is that scale is calculated based on the activation value distribution.
In addition to quantization, another way to improve TensorRT-LLM performance is to use multi-machine and multi-card inference. In some scenarios, large models are too large to be placed on a single GPU for inference, or they can be put down but the computing efficiency is affected, requiring multiple cards or multiple machines for inference.
TensorRT-LLM currently provides two parallel strategies, Tensor Parallelism and Pipeline Parallelism. TP splits the model vertically and places each part on different devices. This will introduce frequent data communication between devices and is generally used in scenarios with high interconnection between devices, such as NVLINK. Another segmentation method is horizontal segmentation. At this time, there is only one horizontal front, and the corresponding communication method is point-to-point communication, which is suitable for scenarios where the device communication bandwidth is weak.
The last feature to highlight is In-flight batching. Batching is a common practice to improve inference performance, but in LLM inference scenarios, the output length of each sample/request in a batch is unpredictable. If you follow the static batching method, the delay of a batch depends on the longest output in sample/request. Therefore, although the output of the shorter sample/request has ended, the computing resources have not been released, and its delay is the same as the delay of the longest output sample/request. The method of in-flight batching is to insert a new sample/request at the end of the sample/request. In this way, it not only reduces the delay of a single sample/request and avoids resource waste, but also improves the throughput of the entire system.
#3. TensorRT-LLM usage process
TensorRT-LLM is similar to TensorRT. First, you need to obtain a pre-trained model, then use the API provided by TensorRT-LLM to rewrite and reconstruct the model calculation graph, and then use TensorRT. Compile and optimize, and then save it as a serialized engine for inference deployment.
Taking Llama as an example, first install TensorRT-LLM, then download the pre-trained model, then use TensorRT-LLM to compile the model, and finally reasoning.
For debugging model inference, the debugging method of TensorRT-LLM is consistent with TensorRT. One of the optimizations provided thanks to the deep learning compiler, namely TensorRT, is layer fusion. Therefore, if you want to output the results of a certain layer, you need to mark the corresponding layer as the output layer to prevent it from being optimized by the compiler, and then compare and analyze it with the baseline. At the same time, every time a new output layer is marked, the TensorRT engine must be recompiled.
For custom layers, TensorRT-LLM provides many Pytorch-like operators to help users implement functions without having to write the kernel themselves. As shown in the example, the API provided by TensorRT-LLM is used to implement the logic of rms norm, and TensorRT will automatically generate the corresponding execution code on the GPU.
If the user has higher performance requirements or TensorRT-LLM does not provide building blocks to implement the corresponding functions, the user needs to customize the kernel at this time , and packaged as a plugin for use by TensorRT-LLM. The sample code is a sample code that implements SmoothQuant's customized GEMM and encapsulates it into a plugin for TensorRT-LLM to call.
##4. Inference performance of TensorRT-LLM
Details such as performance and configuration can be seen on the official website and will not be introduced in detail here. This product has been cooperating with many major domestic manufacturers since its establishment. Through feedback, in general, TensorRT-LLM is the best solution currently from a performance perspective. Since many factors such as technology iteration, optimization methods, and system optimization will affect performance and change very quickly, the performance data of TensorRT-LLM will not be introduced in detail here. If you are interested, you can go to the official website to learn the details. These performances are all reproducible.
Worth What I mention is that the performance of TensorRT-LLM has continued to improve compared with its previous version. As shown in the figure above, based on FP16, after using KVQuant, the usage of video memory is reduced while maintaining the same speed. Using INT8, you can see a significant improvement in throughput, and at the same time, the memory usage is further reduced. It can be seen that with the continuous evolution of TensorRT-LLM optimization technology, performance will continue to improve. This trend will continue.
##5. The future outlook of TensorRT-LLM
LLM is a scenario where reasoning is very expensive and cost-sensitive. We believe that in order to achieve the next hundred-fold acceleration effect, joint iteration of algorithms and hardware is required, and this goal can be achieved through co-design between software and hardware. The hardware provides lower-precision quantization, while the software perspective uses algorithms such as optimized quantization and network pruning to further improve performance.
# 6. Question and Answer Session
Q1: Does every calculation output need to be dequantized? What should I do if precision overflow occurs during quantization?
A1: Currently TensorRT-LLM provides two types of methods, namely FP8 and the INT4/INT8 quantization method just mentioned. Low-precision If INT8 is used as GEMM, the accumulator will use high-precision data types, such as fp16, or even fp32 to prevent overflow. Regarding inverse quantization, taking fp8 quantization as an example, when TensorRT-LLM optimizes the calculation graph, it may automatically move the inverse quantization node and merge it into other operations to achieve optimization purposes. However, the GPTQ and QAT introduced earlier are currently written in the kernel through hard coding, and there is no unified processing of quantization or dequantization nodes.
Q2: Are you currently doing inverse quantization specifically for specific models?
A2: The current quantification is indeed like this, providing support for different models. We have plans to make a cleaner API or to uniformly support model quantification through configuration items.
Q3: For best practices, should TensorRT-LLM be used directly or combined with Triton Inference Server? Are there any missing features if used together?
A3: Because some functions are not open source, if it is your own serving, you need to do adaptation work. If it is triton, it will be a complete solution.
Q4: There are several quantization methods for quantization calibration, and what is the acceleration ratio? How many points are there in the effects of these quantification schemes? The output length of each example in In-flight branching is unknown. How to do dynamic batching?
A4: You can talk privately about quantification performance. Regarding the effect, we only did basic verification to ensure that the implemented kernel is OK. We cannot guarantee that all quantification algorithms will work in actual business. As a result, there are still some uncontrollable factors, such as the data set used for quantification and its impact. Regarding in-flight batching, it refers to detecting and judging whether the output of a certain sample/request has ended during runtime. If so, and then insert other arriving requests, TensorRT-LLM will not and cannot predict the length of the predicted output.
Q5: Will the C interface and python interface of In-flight branching be consistent? The installation cost of TensorRT-LLM is high. Are there any improvement plans in the future? Will TensorRT-LLM have a different development perspective from VLLM?
A5: We will try our best to provide a consistent interface between c runtime and python runtime, which is already under planning. Previously, the team focused on improving performance and improving functions, and will continue to improve on ease of use in the future. It is not easy to compare directly with vllm here, but NVIDIA will continue to increase investment in TensorRT-LLM development, community and customer support to provide the industry with the best LLM inference solution.
The above is the detailed content of Uncovering the NVIDIA large model inference framework: TensorRT-LLM. For more information, please follow other related articles on the PHP Chinese website!
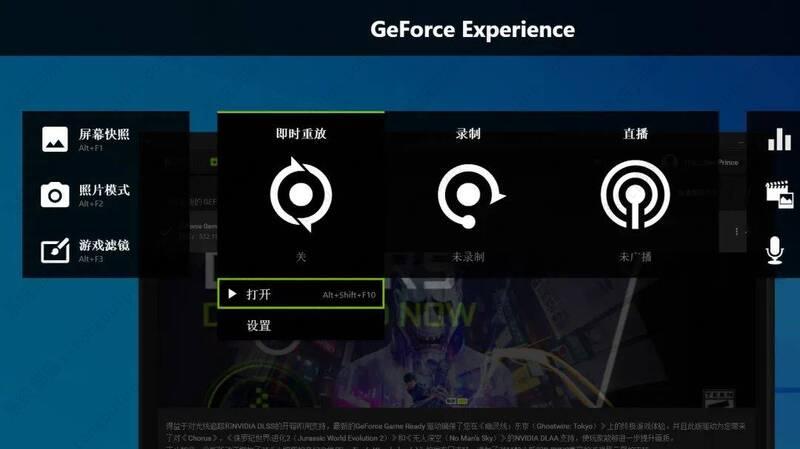
NVIDIA显卡是有自带的录屏功能的,用户们可以直接的利用快捷键录制桌面或者是游戏画面,不过也有用户们反应快捷键使用不了,那么这是怎么回事?下面就让本站来为用户们来仔细的介绍一下n卡录屏快捷键没反应问题解析吧。 n卡录屏快捷键没反应问题解析 方法一、自动录制 1、自动录制即时重放模式,玩家可以将其视为自动录制模式,首先打开NVIDIAGeForceExperience。 2、Alt+Z键呼出软件菜单之后,点击即时重放下方的打开按钮即可开始录制,或通过Alt+Shift+F10快捷键开
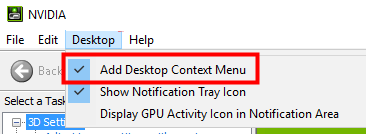
Win11右键没有nvidia控制面板解决方法?很多的用户们在使用电脑的时候都会经常需要打开nvidia控制面板,但是也有不少的用户们发现自己找不到nvidia控制面板,那么这要怎么办?下面就让本站来为用户们来仔细的介绍一下Win11右键没有nvidia控制面板的解决方法吧。Win11右键没有nvidia控制面板的解决方法1、确保它没有被隐藏按键盘上的Windows+R以打开一个新的运行框并输入control。在右上角的查看方式下:选择大图标。打开NVIDIA控制面板,将鼠标悬停在桌面选项上查看
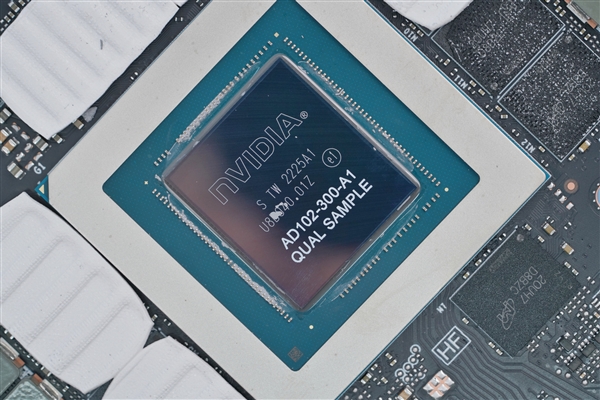
11月16日,NVIDIA正在积极研发专为中国大陆及港澳地区设计的新版本显卡RTX4090D,以应对当地的生产和销售禁令。这款特别版显卡将带来一系列独特的特性和设计调整,以适应当地市场的特殊需求和规定。该显卡以中国龙年2024年为寓意,因此在名称中加入了“D”,代表“Dragon”据业内消息透露,这款RTX4090D将采用一个与原版RTX4090不同的GPU核心,编号为AD102-250。这一编号与RTX4090上的AD102-300/301相比,在数字上显得更低,预示着可能的性能降级。根据NV
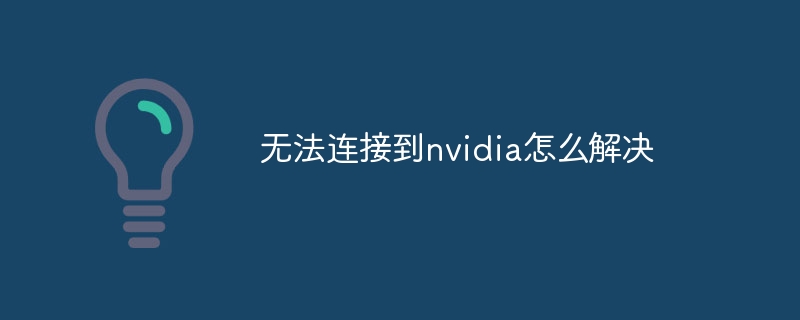
无法连接到nvidia的解决办法:1、检查网络连接;2、检查防火墙设置;3、检查代理设置;4、使用其他网络连接;5、检查NVIDIA服务器状态;6、更新驱动程序;7、重新启动NVIDIA的网络服务。详细介绍:1、检查网络连接,确保计算机正常连接到互联网,可以尝试重新启动路由器或调整网络设置,以确保可以连接到NVIDIA服务;2、检查防火墙设置,防火墙可能会阻止计算机等等。
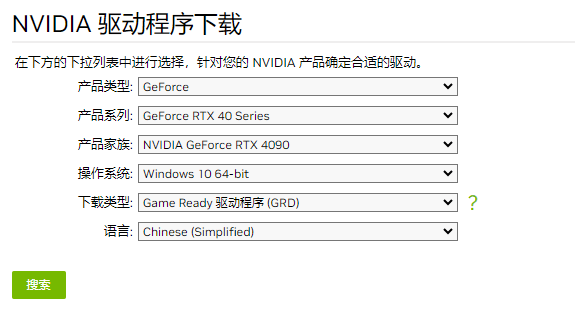
NVIDIA是目前使用人数最多的显卡厂商,很多用户都会首选给自己的电脑安装NVIDIA显卡。但是在使用过程中不免会遇到一些问题,比如NVIDIA驱动程序安装失败,这该如何解决?导致这种情况的原因有很多,下面就来看看具体的解决办法。 步骤一:下载最新的显卡驱动 您需要前往NVIDIA官网下载适用于您的显卡的最新驱动程序。进入驱动程序页面后,选择您的产品类型、产品系列、产品家族、操作系统、下载类型和语言。点击搜索后,网站将自动查询适合您的驱动程序版本。 以搭载GeForceRTX4090的
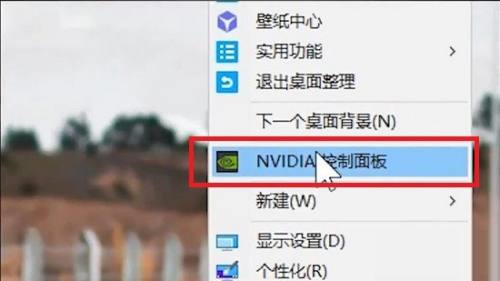
小伙伴们知道nvidia控制面板首选图形处理器在哪吗?今天小编就来讲解nvidia控制面板首选图形处理器的位置介绍,感兴趣的快跟小编一起来看看吧,希望能够帮助到大家。1、我们需要右键桌面空白处,打开“nvidia控制面板”(如图所示)。2、然后进入左边“3D设置”下的“管理3D设置”(如图所示)。3、进入后,在右边就能找到“首选图形处理器”了(如图所示)。
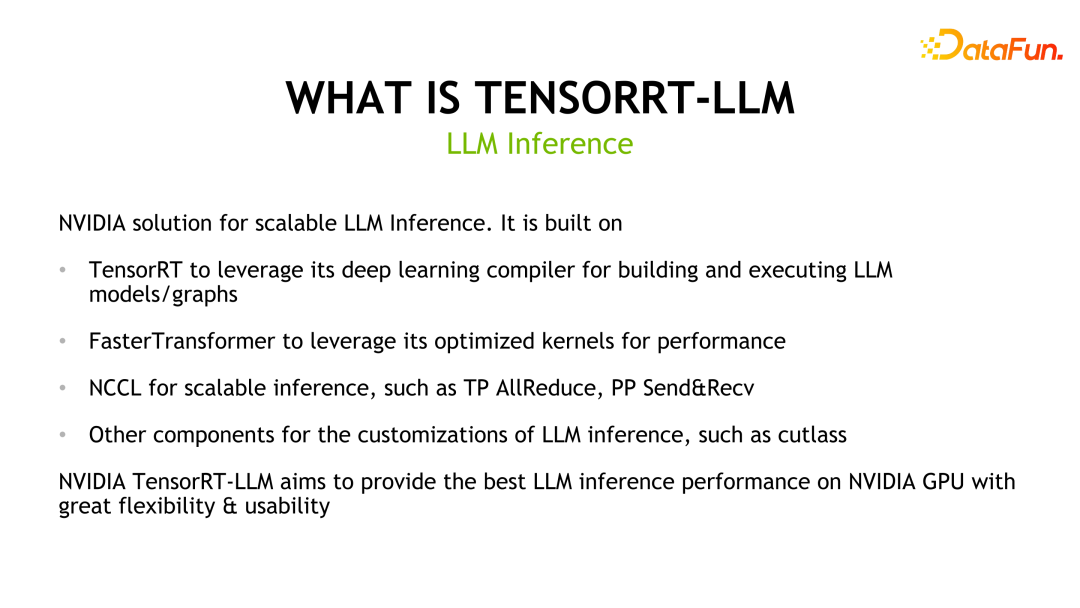
一、TensorRT-LLM的产品定位TensorRT-LLM是NVIDIA为大型语言模型(LLM)开发的可扩展推理方案。它基于TensorRT深度学习编译框架构建、编译和执行计算图,并借鉴了FastTransformer中高效的Kernels实现。此外,它还利用NCCL实现设备间的通信。开发者可以根据技术发展和需求差异,定制算子以满足特定需求,例如基于cutlass开发定制的GEMM。TensorRT-LLM是NVIDIA官方推理方案,致力于提供高性能并不断完善其实用性。TensorRT-LL
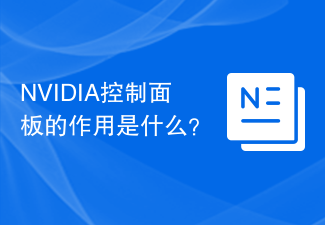
NVIDIA控制面板是干嘛的随着计算机科技发展的日新月异,显卡的重要性变得越来越大。而NVIDIA作为全球著名的显卡制造商之一,其控制面板更是备受瞩目。那么,NVIDIA控制面板究竟是干什么的呢?本文将为大家详细介绍NVIDIA控制面板的功能和用途。首先,我们来了解NVIDIA控制面板的概念与定义。NVIDIA控制面板是一种用于管理和配置显卡相关设置的软件。


Hot AI Tools

Undresser.AI Undress
AI-powered app for creating realistic nude photos

AI Clothes Remover
Online AI tool for removing clothes from photos.

Undress AI Tool
Undress images for free

Clothoff.io
AI clothes remover

AI Hentai Generator
Generate AI Hentai for free.

Hot Article

Hot Tools

SAP NetWeaver Server Adapter for Eclipse
Integrate Eclipse with SAP NetWeaver application server.

PhpStorm Mac version
The latest (2018.2.1) professional PHP integrated development tool

SublimeText3 Chinese version
Chinese version, very easy to use

MantisBT
Mantis is an easy-to-deploy web-based defect tracking tool designed to aid in product defect tracking. It requires PHP, MySQL and a web server. Check out our demo and hosting services.
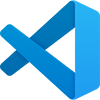
VSCode Windows 64-bit Download
A free and powerful IDE editor launched by Microsoft