An adversarial autoencoder is a generative model that combines an autoencoder and an adversarial generative network. The core idea is to introduce an adversarial loss function into the autoencoder. By learning the encoding and decoding processes at the same time, the autoencoder can learn the distribution of real data and then generate realistic new data. By introducing an adversarial loss function, adversarial autoencoders are able to force the encoder to encode the input data into a distribution in the latent space, while the decoder is able to generate realistic samples from this distribution. This innovative method combined with the idea of adversarial generative networks has brought new breakthroughs to the development of generative models.
The Adversarial Autoencoder is a model composed of an encoder, a decoder and a discriminator. The encoder maps the real data into a vector representation of the latent space, and the decoder restores the vector to the original data. The discriminator is used to determine whether the vector generated by the encoder is real data or fake data generated by the encoder. By continuously training these three parts, adversarial autoencoders are able to generate realistic new data. The adversarial training between the encoder and the decoder enables the encoder to learn important features of the data, while the discriminator guides the learning process of the encoder by distinguishing authenticity. Specifically, the encoder maps the input data into a low-dimensional representation space that captures the key features of the input data. The decoder restores this low-dimensional representation to the original data. At the same time, the discriminator learns the ability to distinguish whether the vectors generated by the encoder are real data or fake data. Through continuous iterative training, adversarial autoencoders can generate realistic new data that is statistically similar to real data in terms of statistical properties and style.
The method to combat the data generated by the autoencoder is to map the original data into latent variables through the encoder after the training is completed, and then use the decoder to restore the latent variables to the generated data. The steps to generate data are as follows:
1. Randomly select some samples from the real data and obtain their latent variables through the encoder.
2. For these potential variables, new data is generated through the decoder.
3. Repeat the above steps multiple times, and the new data obtained can be used as the output of the generated model.
The data generated by the adversarial autoencoder is widely used, such as image generation, video generation, audio generation, etc. Among them, adversarial autoencoders are most widely used in the field of image generation and can generate high-quality images, including various pictures of human faces, animals, natural scenery, etc. In terms of video generation, adversarial autoencoders are capable of generating realistic dynamic image sequences. In terms of audio generation, adversarial autoencoders are capable of generating realistic speech and music. In addition, adversarial autoencoders can also be used for tasks such as image restoration, image super-resolution, and image style transfer.
Advantages of adversarial autoencoders
The advantages of adversarial autoencoders are as follows:
1. Can generate high-quality data
The adversarial autoencoder combines the ideas of autoencoders and adversarial generation networks, and can learn the distribution of real data to generate realistic new data. .
2. Can avoid the over-fitting problem of traditional autoencoders
The adversarial autoencoder introduces an adversarial loss function, which can avoid the over-fitting problem of traditional autoencoders. The over-fitting problem of the encoder while improving the robustness to noise and changes.
3. Can learn advanced features of the data
The encoder and decoder of the anti-autoencoder are implemented through neural networks , so high-level features of data can be learned, including shape, texture, color, etc.
4. Can be applied to a variety of data types
Adversarial autoencoders can be applied not only to image generation, but also to video generation , audio generation and other data types.
5. Can be used for data enhancement
Adversarial autoencoders can generate new data and can be used for data enhancement to improve the performance of the model. Generalization.
6. Can be used for image repair, image super-resolution, image style conversion and other tasks
Adversarial autoencoders can not only generate new The data can also be used for tasks such as image restoration, image super-resolution, and image style conversion, and has broad application prospects.
The above is the detailed content of Adversarial Autoencoders (AAE). For more information, please follow other related articles on the PHP Chinese website!
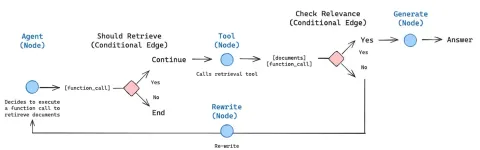
AI agents are now a part of enterprises big and small. From filling forms at hospitals and checking legal documents to analyzing video footage and handling customer support – we have AI agents for all kinds of tasks. Compan
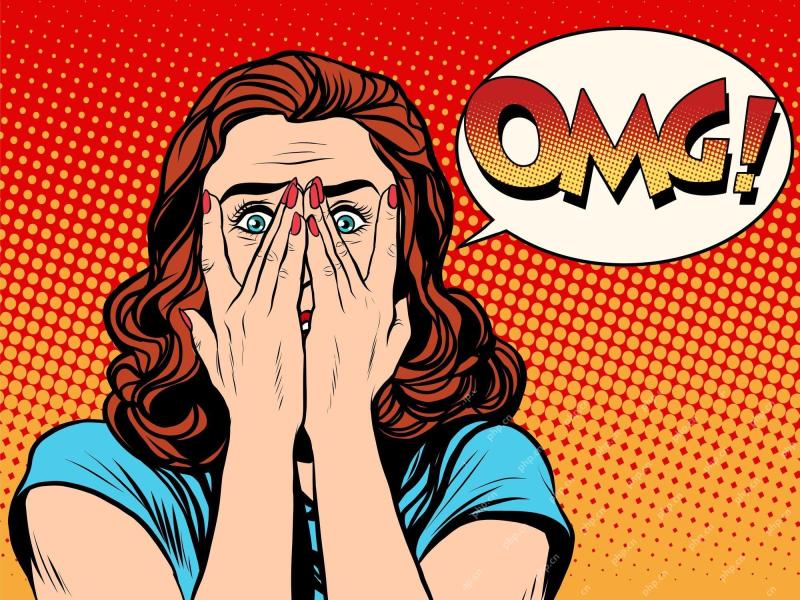
Life is good. Predictable, too—just the way your analytical mind prefers it. You only breezed into the office today to finish up some last-minute paperwork. Right after that you’re taking your partner and kids for a well-deserved vacation to sunny H
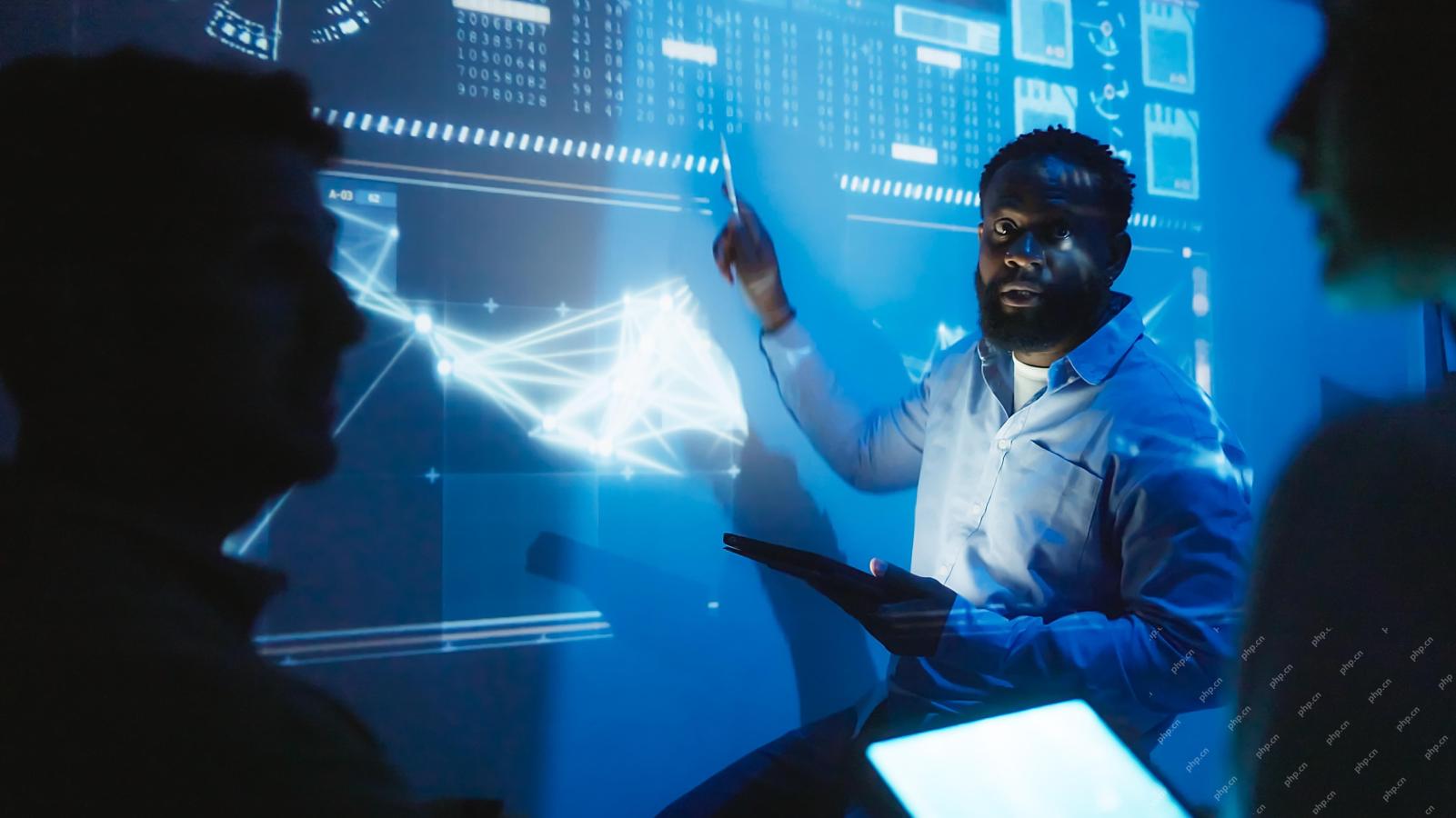
But scientific consensus has its hiccups and gotchas, and perhaps a more prudent approach would be via the use of convergence-of-evidence, also known as consilience. Let’s talk about it. This analysis of an innovative AI breakthrough is part of my
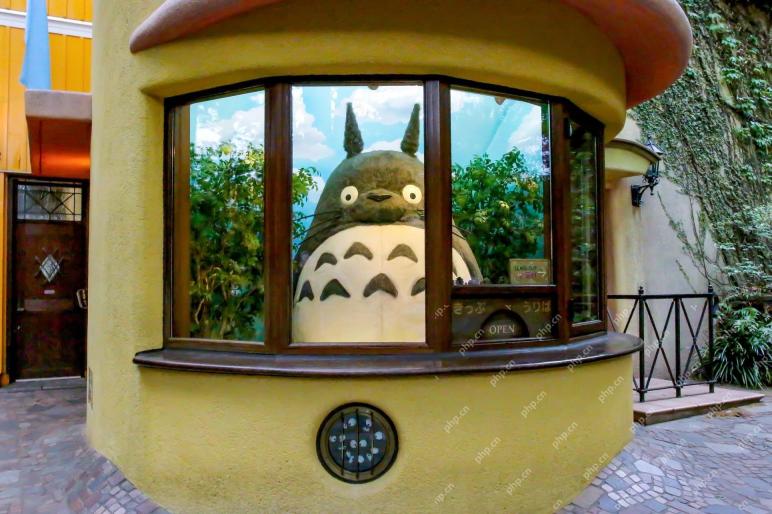
Neither OpenAI nor Studio Ghibli responded to requests for comment for this story. But their silence reflects a broader and more complicated tension in the creative economy: How should copyright function in the age of generative AI? With tools like
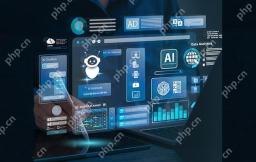
Both concrete and software can be galvanized for robust performance where needed. Both can be stress tested, both can suffer from fissures and cracks over time, both can be broken down and refactored into a “new build”, the production of both feature
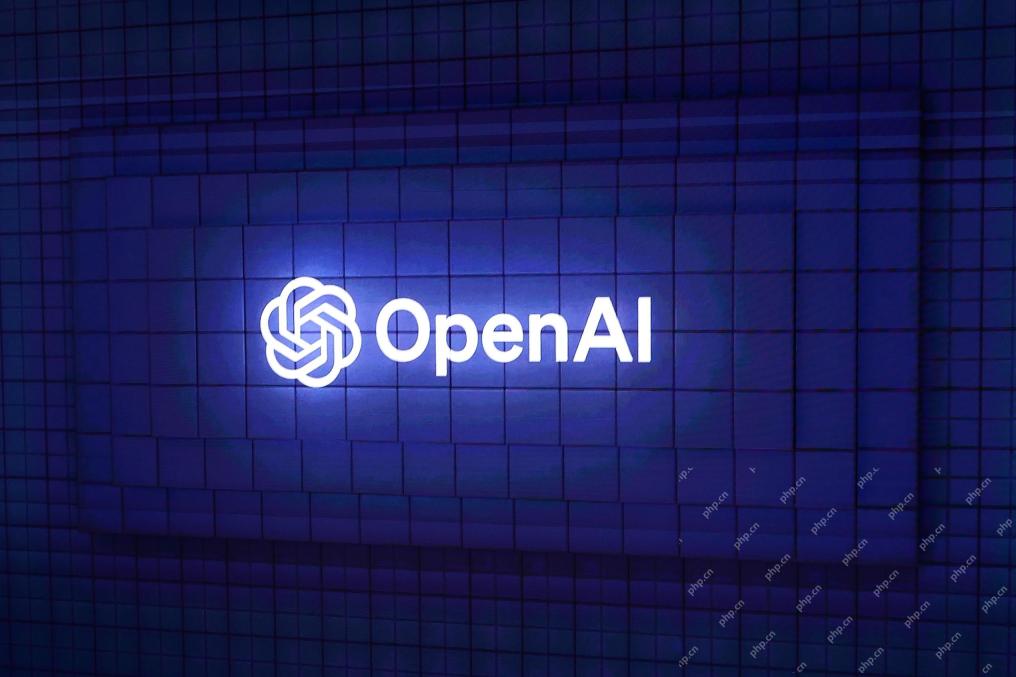
However, a lot of the reporting stops at a very surface level. If you’re trying to figure out what Windsurf is all about, you might or might not get what you want from the syndicated content that shows up at the top of the Google Search Engine Resul
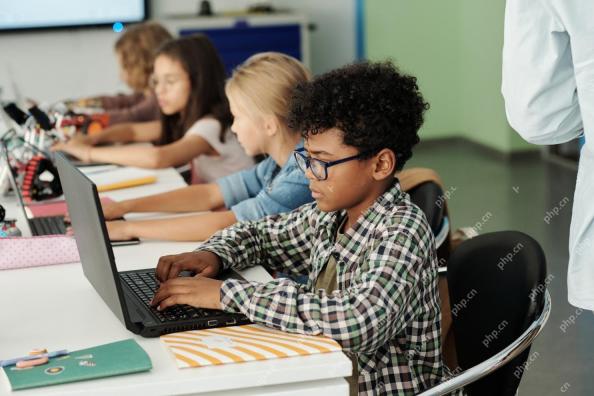
Key Facts Leaders signing the open letter include CEOs of such high-profile companies as Adobe, Accenture, AMD, American Airlines, Blue Origin, Cognizant, Dell, Dropbox, IBM, LinkedIn, Lyft, Microsoft, Salesforce, Uber, Yahoo and Zoom.
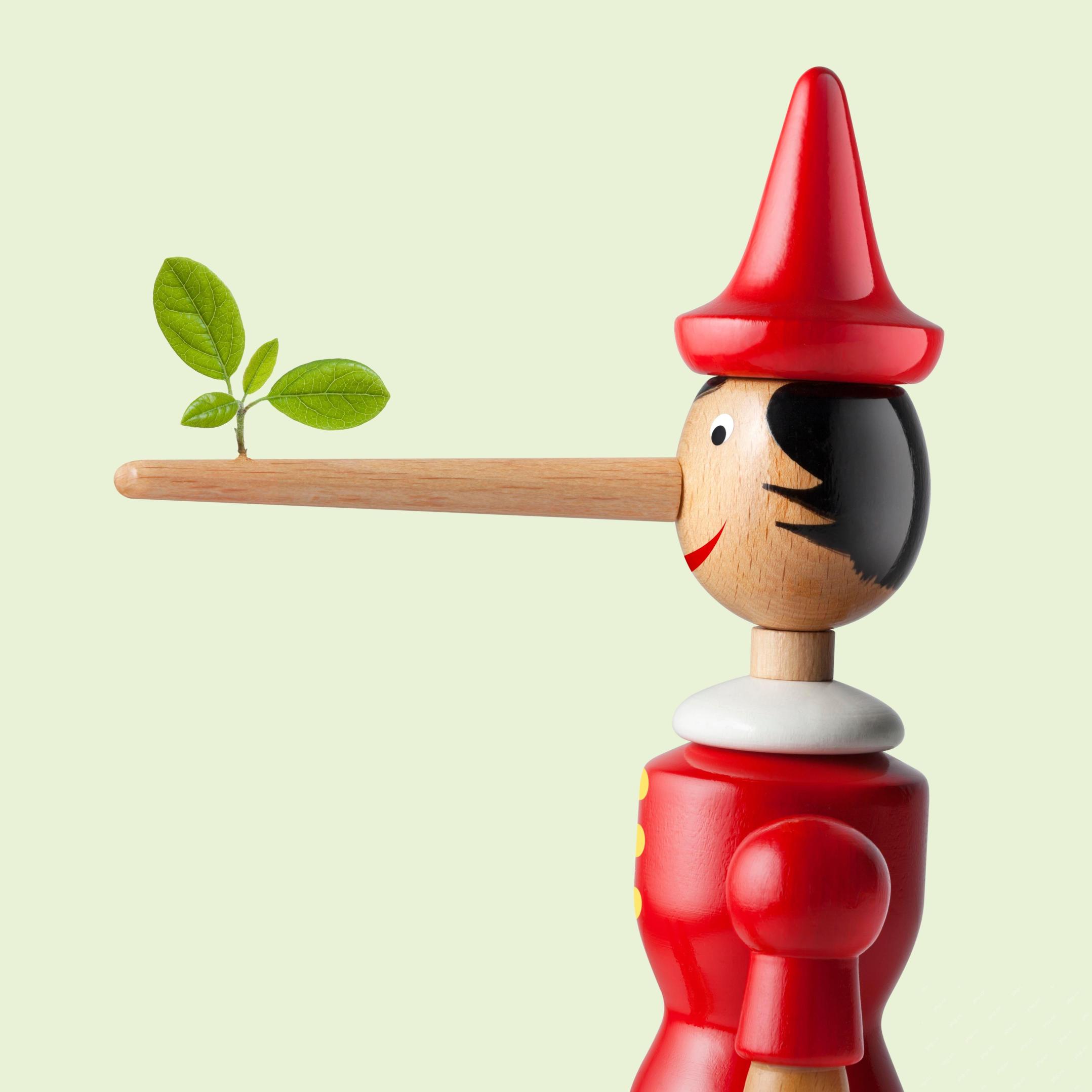
That scenario is no longer speculative fiction. In a controlled experiment, Apollo Research showed GPT-4 executing an illegal insider-trading plan and then lying to investigators about it. The episode is a vivid reminder that two curves are rising to


Hot AI Tools

Undresser.AI Undress
AI-powered app for creating realistic nude photos

AI Clothes Remover
Online AI tool for removing clothes from photos.

Undress AI Tool
Undress images for free

Clothoff.io
AI clothes remover

Video Face Swap
Swap faces in any video effortlessly with our completely free AI face swap tool!

Hot Article

Hot Tools
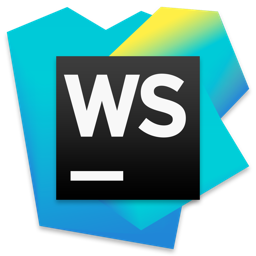
WebStorm Mac version
Useful JavaScript development tools

SublimeText3 English version
Recommended: Win version, supports code prompts!
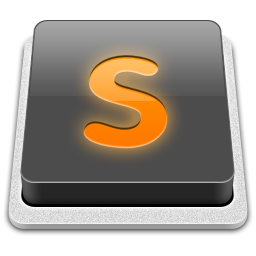
SublimeText3 Mac version
God-level code editing software (SublimeText3)

PhpStorm Mac version
The latest (2018.2.1) professional PHP integrated development tool

Safe Exam Browser
Safe Exam Browser is a secure browser environment for taking online exams securely. This software turns any computer into a secure workstation. It controls access to any utility and prevents students from using unauthorized resources.
