Deep image datasets are a very important data type in deep learning and computer vision tasks. It contains depth information for each pixel and can be used for a variety of applications such as scene reconstruction, object detection, and pose estimation. This article will introduce several commonly used depth image data sets, including their sources, characteristics and applications.
1.NYU Depth V2
NYU Depth V2 dataset contains depth images and RGB images of indoor scenes, with a total of 1449 scene samples. These scenes include various indoor environments such as bedrooms, living rooms, and kitchens. Each scene provides intrinsic and extrinsic parameter information of the camera, which can be used for tasks such as camera pose estimation and scene reconstruction. In addition, the data set also provides annotation information of objects in the scene, which can be used for tasks such as object detection and semantic segmentation.
2.Kinect Fusion
The Kinect Fusion dataset provides RGB-D images of multiple scenes and corresponding 3D models, suitable for Tasks such as scene reconstruction, 3D pose estimation and object detection. In addition, the data set also supports data formats from multiple depth sensors, including devices such as Microsoft Kinect, Asus Xtion Pro Live, and Primesense Carmine 1.08. This data provides researchers and developers with a rich resource for research and development in areas such as deep learning, computer vision, and robotics.
3.SUN RGB-D
SUN RGB-D contains RGB-D images and scene annotation information for indoor and outdoor scenes. The data set contains a total of 10,335 scene samples, of which 5,285 are indoor scenes and 5,050 are outdoor scenes. Each scene provides camera intrinsic and extrinsic parameter information, which can be used for tasks such as camera pose estimation and scene reconstruction. In addition, this data set also provides a variety of scene annotation information, including object categories, semantic segmentation and scene layout, etc., which can be used for tasks such as object detection, semantic segmentation and scene understanding.
4.ScanNet
ScanNet contains RGB-D images and scene annotation information of indoor scenes. The dataset contains a total of 1,513 scene samples, covering a variety of different indoor environments, including offices, shops, schools, etc. Each scene provides camera intrinsic and extrinsic parameter information, which can be used for tasks such as camera pose estimation and scene reconstruction. In addition, this data set also provides a variety of scene annotation information, including object categories, semantic segmentation and scene layout, etc., which can be used for tasks such as object detection, semantic segmentation and scene understanding.
5.3DMatch
3DMatch contains depth images and 3D point cloud data from multiple RGB-D sensors. The dataset contains a total of 1,525 scene samples, covering a variety of different indoor and outdoor environments. Each scene provides camera intrinsic and extrinsic parameter information, which can be used for tasks such as camera pose estimation and scene reconstruction. In addition, this data set also provides rich scene registration information, including point cloud registration and image registration, which can be used for tasks such as 3D reconstruction and scene matching.
In short, depth image datasets are an indispensable data type in the fields of deep learning and computer vision. They can be used for a variety of tasks, such as scene reconstruction, object detection, Pose estimation and semantic segmentation, etc. The data sets introduced above are all commonly used depth image data sets. Their sources are authentic and reliable, and their characteristics and applications are different. Appropriate data sets can be selected for training and evaluation according to the needs of specific tasks.
The above is the detailed content of An introduction to depth image datasets. For more information, please follow other related articles on the PHP Chinese website!
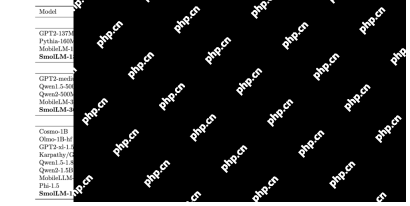
Harness the Power of On-Device AI: Building a Personal Chatbot CLI In the recent past, the concept of a personal AI assistant seemed like science fiction. Imagine Alex, a tech enthusiast, dreaming of a smart, local AI companion—one that doesn't rely
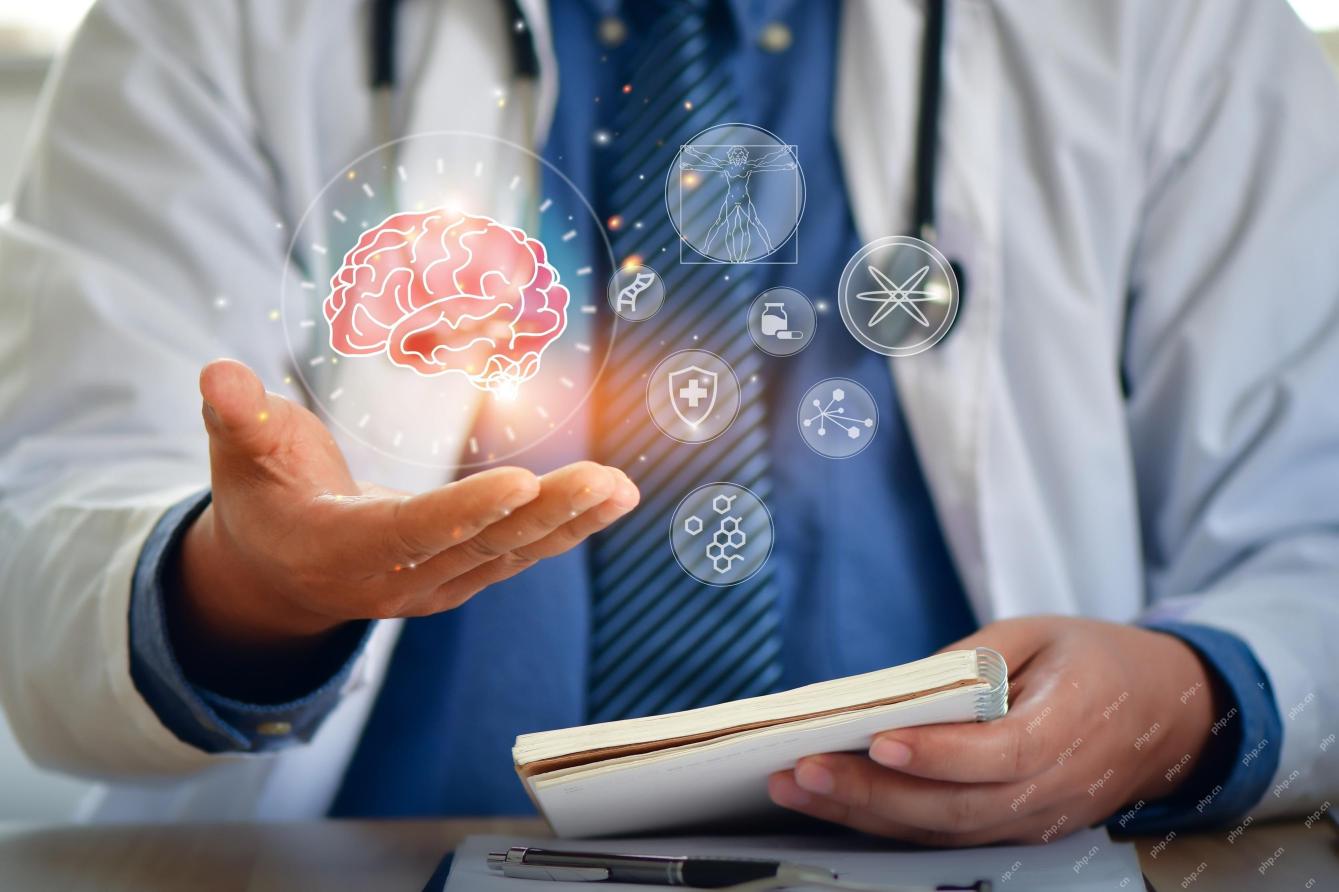
Their inaugural launch of AI4MH took place on April 15, 2025, and luminary Dr. Tom Insel, M.D., famed psychiatrist and neuroscientist, served as the kick-off speaker. Dr. Insel is renowned for his outstanding work in mental health research and techno
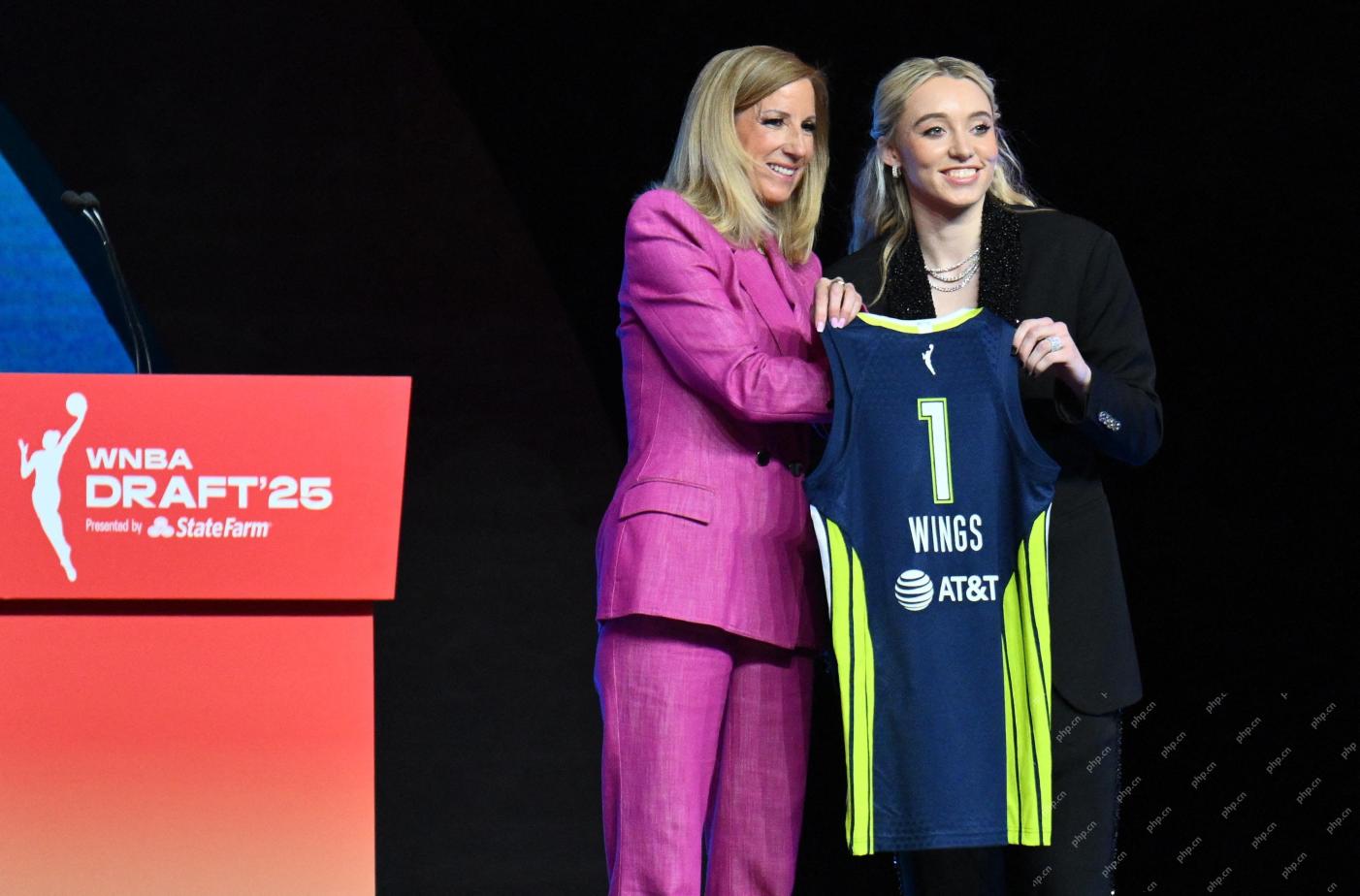
"We want to ensure that the WNBA remains a space where everyone, players, fans and corporate partners, feel safe, valued and empowered," Engelbert stated, addressing what has become one of women's sports' most damaging challenges. The anno
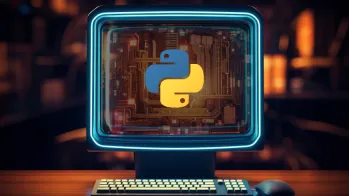
Introduction Python excels as a programming language, particularly in data science and generative AI. Efficient data manipulation (storage, management, and access) is crucial when dealing with large datasets. We've previously covered numbers and st
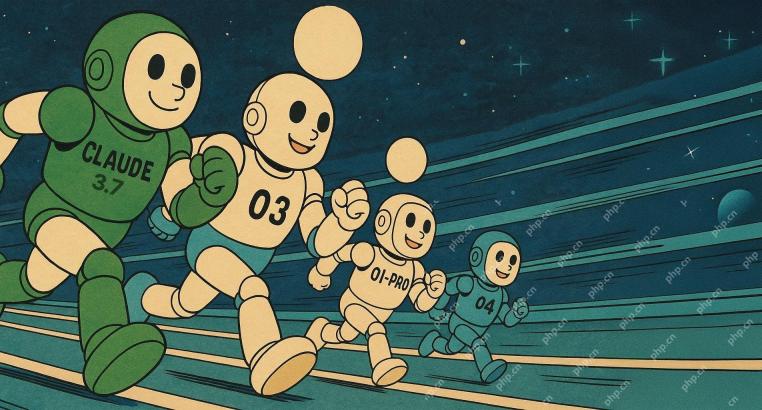
Before diving in, an important caveat: AI performance is non-deterministic and highly use-case specific. In simpler terms, Your Mileage May Vary. Don't take this (or any other) article as the final word—instead, test these models on your own scenario
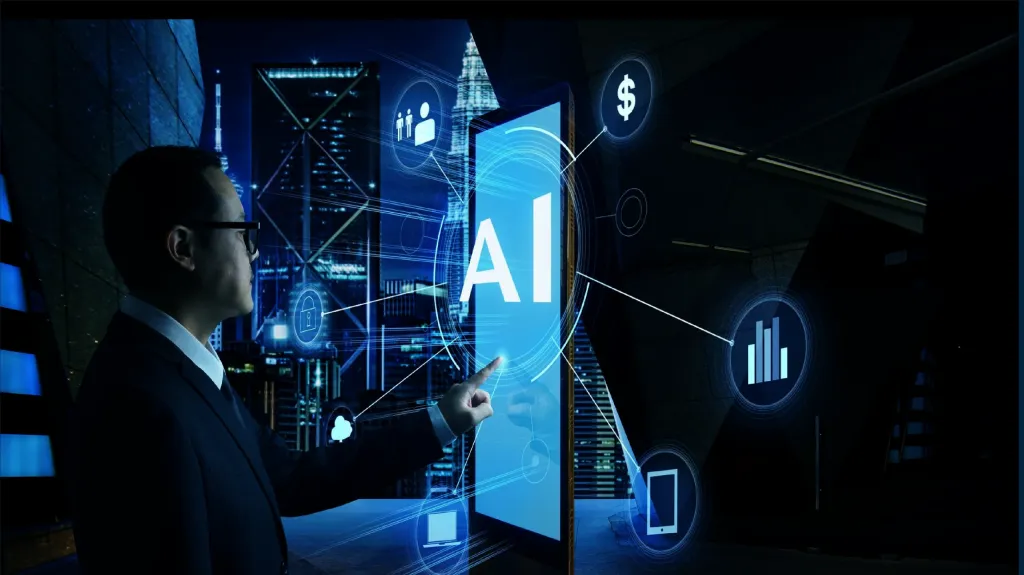
Building a Standout AI/ML Portfolio: A Guide for Beginners and Professionals Creating a compelling portfolio is crucial for securing roles in artificial intelligence (AI) and machine learning (ML). This guide provides advice for building a portfolio
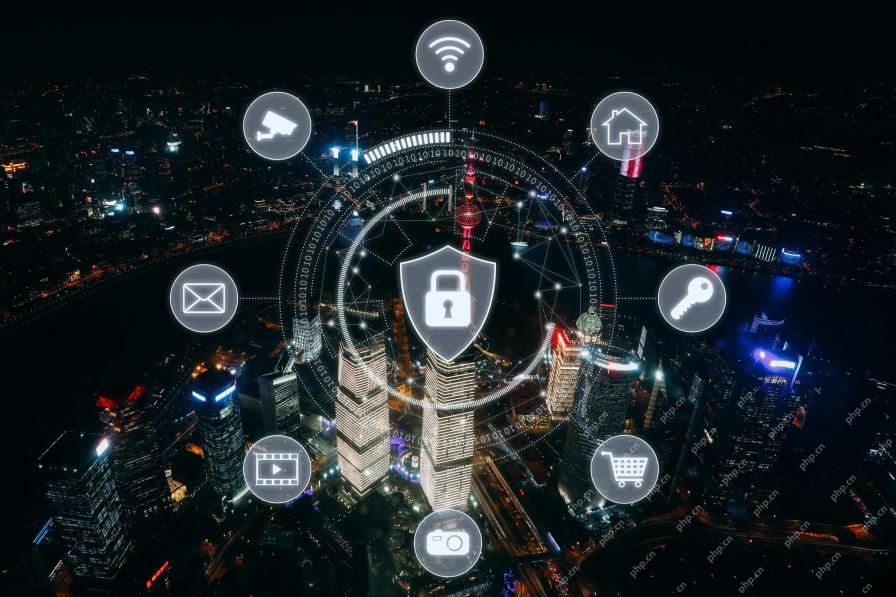
The result? Burnout, inefficiency, and a widening gap between detection and action. None of this should come as a shock to anyone who works in cybersecurity. The promise of agentic AI has emerged as a potential turning point, though. This new class
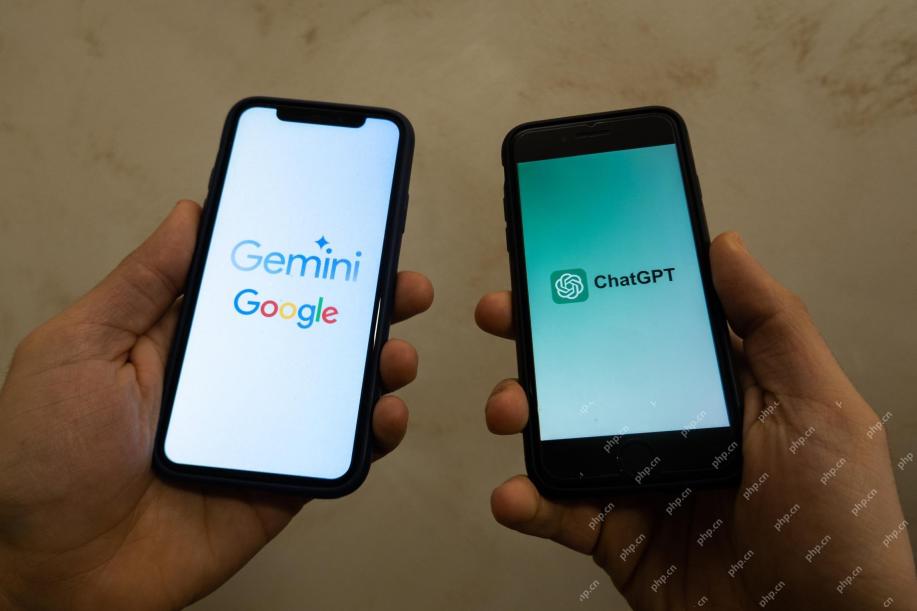
Immediate Impact versus Long-Term Partnership? Two weeks ago OpenAI stepped forward with a powerful short-term offer, granting U.S. and Canadian college students free access to ChatGPT Plus through the end of May 2025. This tool includes GPT‑4o, an a


Hot AI Tools

Undresser.AI Undress
AI-powered app for creating realistic nude photos

AI Clothes Remover
Online AI tool for removing clothes from photos.

Undress AI Tool
Undress images for free

Clothoff.io
AI clothes remover

AI Hentai Generator
Generate AI Hentai for free.

Hot Article

Hot Tools

Safe Exam Browser
Safe Exam Browser is a secure browser environment for taking online exams securely. This software turns any computer into a secure workstation. It controls access to any utility and prevents students from using unauthorized resources.
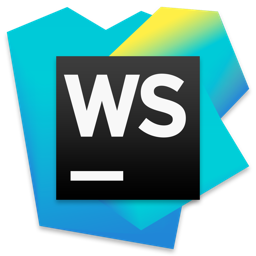
WebStorm Mac version
Useful JavaScript development tools

SAP NetWeaver Server Adapter for Eclipse
Integrate Eclipse with SAP NetWeaver application server.

MinGW - Minimalist GNU for Windows
This project is in the process of being migrated to osdn.net/projects/mingw, you can continue to follow us there. MinGW: A native Windows port of the GNU Compiler Collection (GCC), freely distributable import libraries and header files for building native Windows applications; includes extensions to the MSVC runtime to support C99 functionality. All MinGW software can run on 64-bit Windows platforms.

Atom editor mac version download
The most popular open source editor