


What methods can be used for text classification of small-scale data sets?
Text classification methods suitable for ultra-small data sets mainly include traditional machine learning methods and deep learning methods. Traditional machine learning methods tend to perform better on small data sets because they can produce better models with limited data. In contrast, deep learning methods require more data for training to achieve good results. Traditional machine learning methods and deep learning methods will be briefly introduced below.
1. Traditional machine learning methods
In traditional machine learning methods, commonly used text classification algorithms include Naive Bayes and Support Vectors Machine (SVM), decision tree, etc. These algorithms are based on feature engineering methods, which convert text into feature vectors and then use machine learning algorithms for classification. Among them, the Naive Bayes algorithm is a classification algorithm based on Bayes' theorem. It assumes that all features are independent of each other, so classification can be performed by calculating the contribution of each feature to classification. The SVM algorithm is a classification and regression method that searches for an optimal hyperplane by mapping data into a high-dimensional space to separate different categories. The decision tree algorithm is a classification algorithm based on a tree structure. It establishes a tree model by continuously dividing the data set to achieve classification.
Traditional machine learning methods have the advantage of handling small data sets and lower computing resource requirements. In addition, they have relatively mature technology in feature engineering, which can improve model performance by selecting appropriate features. However, these methods also have some drawbacks. First, feature engineering requires a lot of manual involvement, and feature selection may have an impact on model performance. Second, these algorithms often cannot handle semantic information in text well because they can only handle numbers or discrete features and cannot handle natural language. Finally, these methods may face under-fitting or over-fitting issues when dealing with complex data sets. Therefore, to address these problems, it is necessary to consider using methods such as deep learning to overcome the limitations of traditional machine learning methods. Deep learning methods can automatically extract features, process semantic information in text, and have more powerful model fitting capabilities. However, deep learning methods also require more data and computing resources, as well as more complex model tuning processes. Therefore, when choosing a machine learning method, trade-offs need to be made based on the characteristics of the specific task and available resources.
For example, if we want to classify a set of news, we can use the naive Bayes algorithm in traditional machine learning methods. We can convert each news article into a feature vector and match it with predefined tags. For example, we can convert the title, text, release time and other information of the news into feature vectors, and then use the Naive Bayes algorithm to classify. This method can quickly classify news and does not require much data. However, this method may be affected by feature selection, which may affect the accuracy of classification if the selected features are not accurate enough.
2. Deep learning method
In the deep learning method, commonly used text classification algorithms include convolutional neural network (CNN), loop Neural network (RNN) and long short-term memory network (LSTM), etc. These algorithms are based on neural network methods, which can automatically learn the features in the input data and classify them. Among them, the CNN algorithm is a commonly used image processing algorithm, but it can also be used for text classification. It extracts features from the input data through convolution operations and pooling operations, and uses fully connected layers for classification. The RNN algorithm is an algorithm that can process sequence data. It can predict future states by memorizing past states, so it is suitable for processing text data. The LSTM algorithm is a variant of RNN that uses a gating mechanism to control the flow of information, thus solving the problems of gradient disappearance and gradient explosion in RNN.
The advantage of deep learning methods is that they can automatically learn features in input data and can handle complex semantic information. In addition, deep learning methods can speed up the training process by pre-training models and can use transfer learning techniques to solve the problem of small data sets. However, deep learning methods also have some disadvantages. First, deep learning methods require a large amount of data and computing resources to train a good model. Secondly, deep learning methods are highly black-box and difficult to explain the decision-making process of the model. Finally, deep learning methods often perform worse than traditional machine learning methods on small data sets.
For example, if we want to perform sentiment classification on a set of movie reviews, we can use the LSTM algorithm in the deep learning method. We can convert each review into a word vector and feed it into an LSTM model for classification. For example, we can use a pre-trained word vector model to convert each word into a word vector, and input the sequence of all word vectors into the LSTM model. This method can automatically learn features in the input data and can handle complex semantic information. However, since movie review datasets are usually small, we may need to use transfer learning techniques to improve the performance of the model.
In summary, traditional machine learning methods and deep learning methods have their own advantages and disadvantages. In the case of ultra-small data sets, traditional machine learning methods are more suitable for processing. When choosing an appropriate method, the choice needs to be based on the specific data set and task. If the data set is small, you can choose traditional machine learning methods and appropriate feature engineering; if the data set is large, you can choose deep learning methods and use pre-trained models and transfer learning techniques to improve the performance of the model. At the same time, when selecting a method, factors such as model interpretability, computational resources, and time cost also need to be considered.
The above is the detailed content of What methods can be used for text classification of small-scale data sets?. For more information, please follow other related articles on the PHP Chinese website!
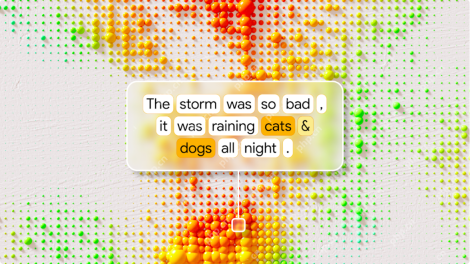
Exploring the Inner Workings of Language Models with Gemma Scope Understanding the complexities of AI language models is a significant challenge. Google's release of Gemma Scope, a comprehensive toolkit, offers researchers a powerful way to delve in
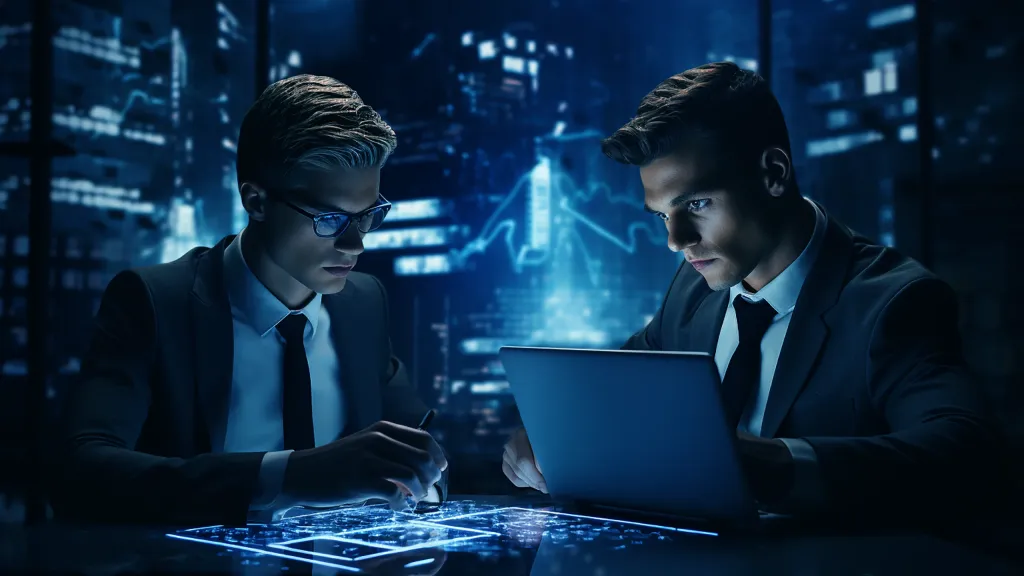
Unlocking Business Success: A Guide to Becoming a Business Intelligence Analyst Imagine transforming raw data into actionable insights that drive organizational growth. This is the power of a Business Intelligence (BI) Analyst – a crucial role in gu

SQL's ALTER TABLE Statement: Dynamically Adding Columns to Your Database In data management, SQL's adaptability is crucial. Need to adjust your database structure on the fly? The ALTER TABLE statement is your solution. This guide details adding colu
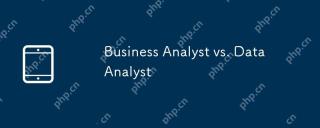
Introduction Imagine a bustling office where two professionals collaborate on a critical project. The business analyst focuses on the company's objectives, identifying areas for improvement, and ensuring strategic alignment with market trends. Simu
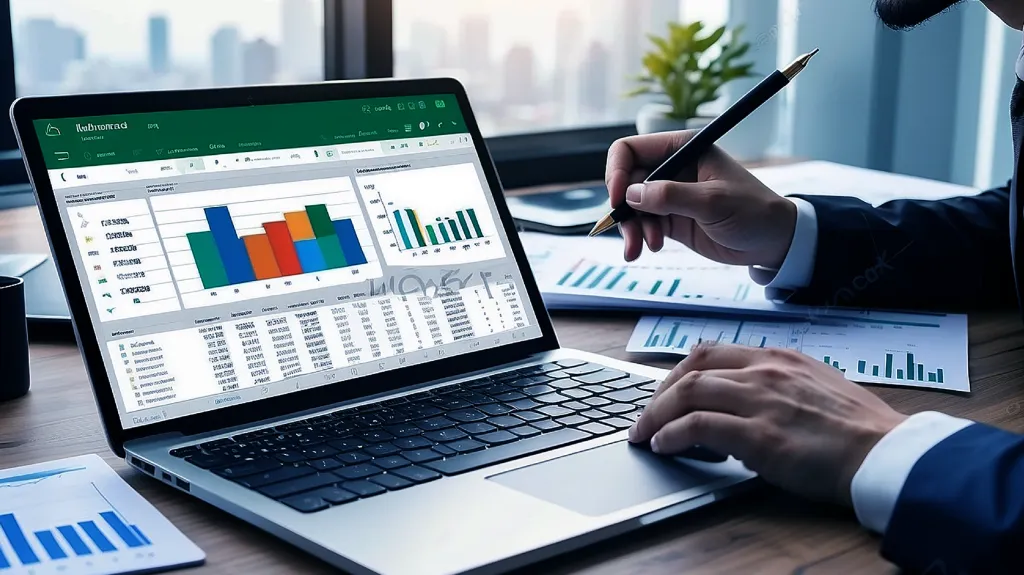
Excel data counting and analysis: detailed explanation of COUNT and COUNTA functions Accurate data counting and analysis are critical in Excel, especially when working with large data sets. Excel provides a variety of functions to achieve this, with the COUNT and COUNTA functions being key tools for counting the number of cells under different conditions. Although both functions are used to count cells, their design targets are targeted at different data types. Let's dig into the specific details of COUNT and COUNTA functions, highlight their unique features and differences, and learn how to apply them in data analysis. Overview of key points Understand COUNT and COU
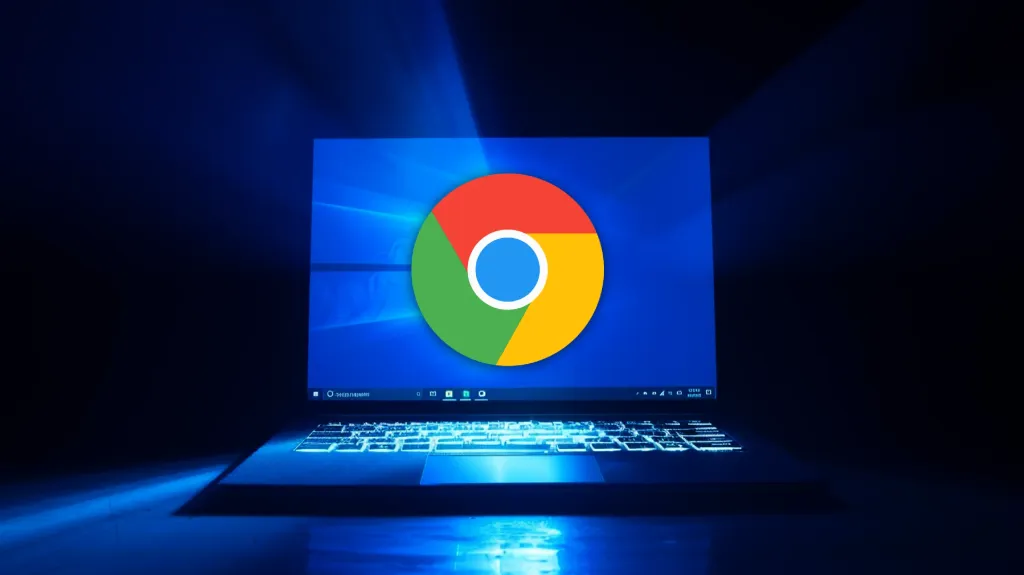
Google Chrome's AI Revolution: A Personalized and Efficient Browsing Experience Artificial Intelligence (AI) is rapidly transforming our daily lives, and Google Chrome is leading the charge in the web browsing arena. This article explores the exciti
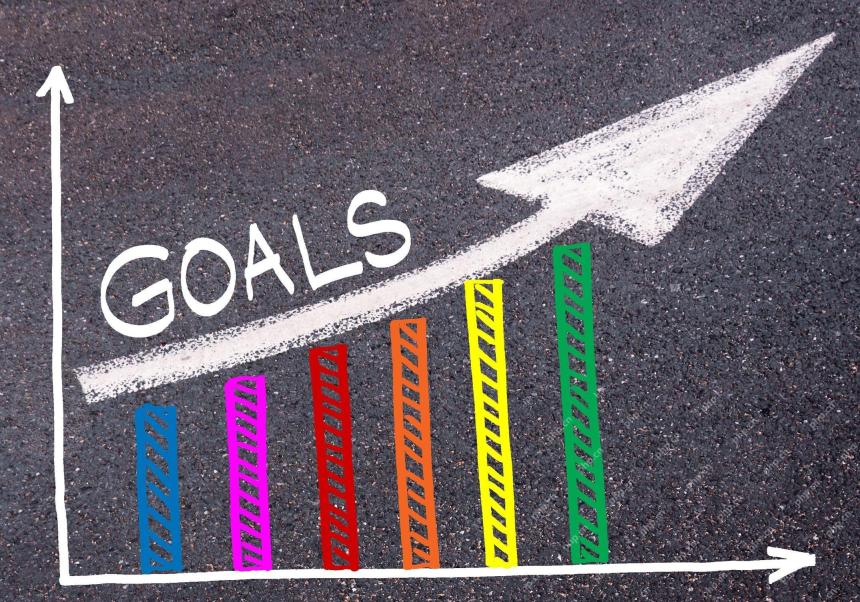
Reimagining Impact: The Quadruple Bottom Line For too long, the conversation has been dominated by a narrow view of AI’s impact, primarily focused on the bottom line of profit. However, a more holistic approach recognizes the interconnectedness of bu
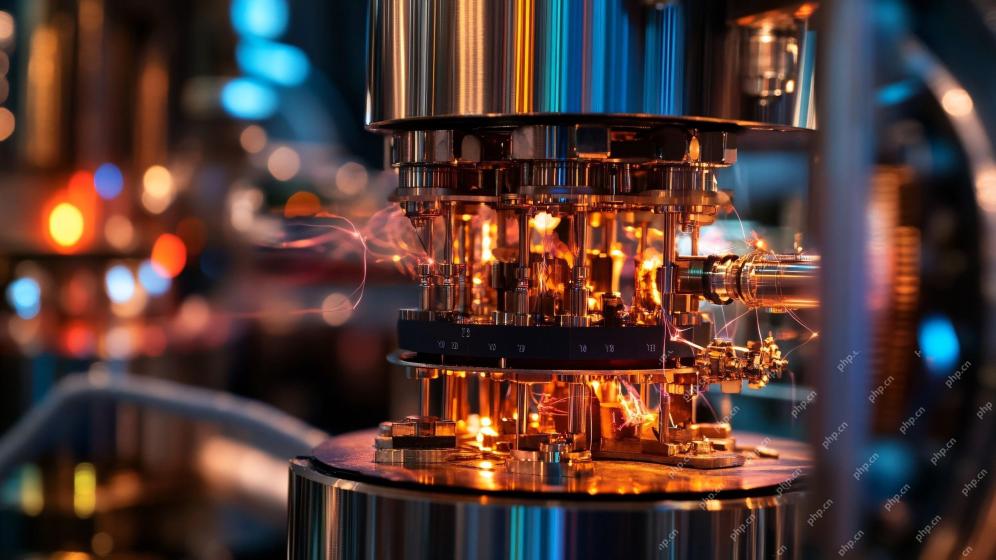
Things are moving steadily towards that point. The investment pouring into quantum service providers and startups shows that industry understands its significance. And a growing number of real-world use cases are emerging to demonstrate its value out


Hot AI Tools

Undresser.AI Undress
AI-powered app for creating realistic nude photos

AI Clothes Remover
Online AI tool for removing clothes from photos.

Undress AI Tool
Undress images for free

Clothoff.io
AI clothes remover

AI Hentai Generator
Generate AI Hentai for free.

Hot Article

Hot Tools

Safe Exam Browser
Safe Exam Browser is a secure browser environment for taking online exams securely. This software turns any computer into a secure workstation. It controls access to any utility and prevents students from using unauthorized resources.

Zend Studio 13.0.1
Powerful PHP integrated development environment

MantisBT
Mantis is an easy-to-deploy web-based defect tracking tool designed to aid in product defect tracking. It requires PHP, MySQL and a web server. Check out our demo and hosting services.
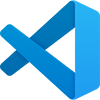
VSCode Windows 64-bit Download
A free and powerful IDE editor launched by Microsoft
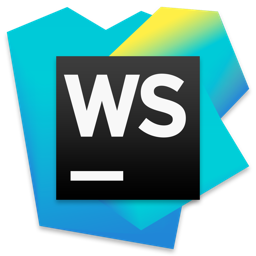
WebStorm Mac version
Useful JavaScript development tools