The progress of machine learning technology will definitely promote the development of handwriting recognition technology. This article will focus on handwriting recognition technologies and algorithms that currently perform well.
Capsule Networks (CapsNets)
Capsule networks are one of the latest and most advanced architectures in neural networks and are considered to be an important addition to existing Improvements in machine learning techniques.
Pooling layers in convolutional blocks are used to reduce data dimensionality and achieve spatial invariance for identifying and classifying objects in images. However, a drawback of pooling is that a large amount of spatial information about object rotation, position, scale, and other positional properties is lost in the process. Therefore, although the accuracy of image classification is high, the performance of locating the precise location of objects in the image is poor.
Capsule is a neuron module used to store information about the position, rotation, scale and other information of objects in high-dimensional vector space. Each dimension represents a special characteristic of the object.
The kernel that generates feature maps and extracts visual features works with dynamic routing by combining individual opinions from multiple groups called capsules. This results in equal variance between kernels and improves performance compared to CNNs.
The kernel that generates feature maps and extracts visual features works with dynamic routing by combining individual opinions from multiple groups (called capsules). This leads to equivalence between kernels and improved performance compared to CNNs.
Multi-dimensional Recurrent Neural Network (MDRNN)
RNN/LSTM (Long Short-Term Memory) processing sequential data is limited to processing one-dimensional data, such as Text, they cannot be extended directly to images.
Multidimensional Recurrent Neural Networks can replace a single recurrent connection in a standard Recurrent Neural Network with as many recurrent units as there are dimensions in the data.
During the forward pass, at each point in the data sequence, the hidden layer of the network receives external input and its own activations, which are one step backward from one dimension ongoing.
The main problem in recognition systems is to convert a two-dimensional image into a one-dimensional label sequence. This is done by passing the input data to a hierarchy of MDRNN layers. Selecting the height of the block gradually collapses the 2D image onto a 1D sequence, which can then be labeled by the output layer.
Multi-dimensional recurrent neural networks are designed to make language models robust to every combination of input dimensions, such as image rotation and shearing, ambiguity of strokes and local distortions of different handwriting styles properties and allow them to flexibly model multidimensional contexts.
Connectionist Temporal Classification(CTC)
This is an algorithm that handles tasks such as speech recognition and handwriting recognition, mapping the entire input data to output class/text.
Traditional recognition methods involve mapping images to corresponding text, however we do not know how patches of images are aligned with characters. CTC can be bypassed without knowing how specific parts of the speech audio or handwritten images align with specific characters.
The input to this algorithm is a vector representation of an image of handwritten text. There is no direct alignment between image pixel representation and character sequence. CTC aims to find this mapping by summing the probabilities of all possible alignments between them.
Models trained with CTC typically use recurrent neural networks to estimate the probability at each time step because recurrent neural networks take into account context in the input. It outputs the character score for each sequence element, represented by a matrix.
For decoding we can use:
Best path decoding: involves predicting the sentence by concatenating the most likely characters for each timestamp to form complete words, resulting in the best path. In the next training iteration, repeated characters and spaces are removed for better decoding of the text.
Beam Search Decoder: Suggests multiple output paths with the highest probability. Paths with smaller probabilities are discarded to keep the beam size constant. The results obtained through this method are more accurate and are often combined with language models to give meaningful results.
transformer model
The Transformer model adopts a different strategy and uses self-attention to remember the entire sequence. A non-cyclic handwriting method can be implemented using the transformer model.
The Transformer model combines the multi-head self-attention layer of the visual layer and the text layer to learn the language model-related dependencies of the character sequence to be decoded. The language knowledge is embedded in the model itself, so there is no need for any additional processing steps using a language model. It is also well suited for predicting outputs that are not part of the vocabulary.
This architecture has two parts:
Text transcriber, which outputs decoded characters by paying attention to each other on visual and language-related features.
Visual feature encoder, designed to extract relevant information from handwritten text images by focusing on various character positions and their contextual information.
Encoder-Decoder and Attention Network
Training handwriting recognition systems is always troubled by the scarcity of training data. To solve this problem, this method uses pre-trained feature vectors of text as a starting point. State-of-the-art models use attention mechanisms in conjunction with RNNs to focus on useful features for each timestamp.
The complete model architecture can be divided into four stages: normalize the input text image, encode the normalized input image into a 2D visual feature map, and use bidirectional LSTM for decoding To perform sequential modeling, the output vector of contextual information from the decoder is converted into words.
Scan, Attend and Read
This is a method for end-to-end handwriting recognition using attention mechanism. It scans the entire page at once. Therefore, it does not rely on splitting the entire word into characters or lines beforehand. This method uses a multidimensional LSTM (MDLSTM) architecture as a feature extractor similar to the above. The only difference is the last layer, where the extracted feature maps are folded vertically and a softmax activation function is applied to identify the corresponding text.
The attention model used here is a hybrid combination of content-based attention and location-based attention. The decoder LSTM module takes the previous state and attention maps and encoder features to generate the final output character and state vector for the next prediction.
Convolve, Attend and Spell
This is a sequence-to-sequence model for handwritten text recognition based on the attention mechanism. The architecture contains three main parts:
- An encoder consisting of a CNN and a bidirectional GRU
- Attention mechanism that focuses on relevant features
- The decoder formed by the one-way GRU is able to spell out the corresponding words character by character
Recurrent neural networks are most suitable for the temporal characteristics of the text. When paired with such a recurrent architecture, the attention mechanism plays a crucial role in focusing on the right features at each time step.
Handwritten text generation
Synthetic handwriting generation can generate realistic handwritten text, which can be used to enhance existing datasets.
Deep learning models require large amounts of data to train, and obtaining a large corpus of annotated handwritten images in different languages is a tedious task. We can solve this problem by using generative adversarial networks to generate training data.
ScrabbleGAN is a semi-supervised method for synthesizing handwritten text images. It relies on a generative model that can generate arbitrary-length word images using a fully convolutional network.
The above is the detailed content of Handwriting recognition technology and its algorithm classification. For more information, please follow other related articles on the PHP Chinese website!
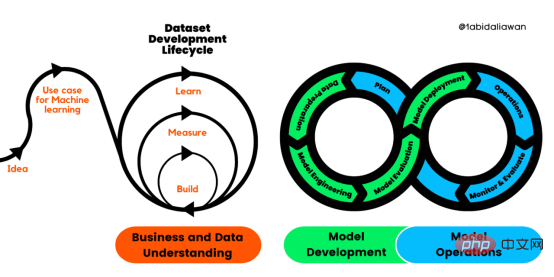
译者 | 布加迪审校 | 孙淑娟目前,没有用于构建和管理机器学习(ML)应用程序的标准实践。机器学习项目组织得不好,缺乏可重复性,而且从长远来看容易彻底失败。因此,我们需要一套流程来帮助自己在整个机器学习生命周期中保持质量、可持续性、稳健性和成本管理。图1. 机器学习开发生命周期流程使用质量保证方法开发机器学习应用程序的跨行业标准流程(CRISP-ML(Q))是CRISP-DM的升级版,以确保机器学习产品的质量。CRISP-ML(Q)有六个单独的阶段:1. 业务和数据理解2. 数据准备3. 模型
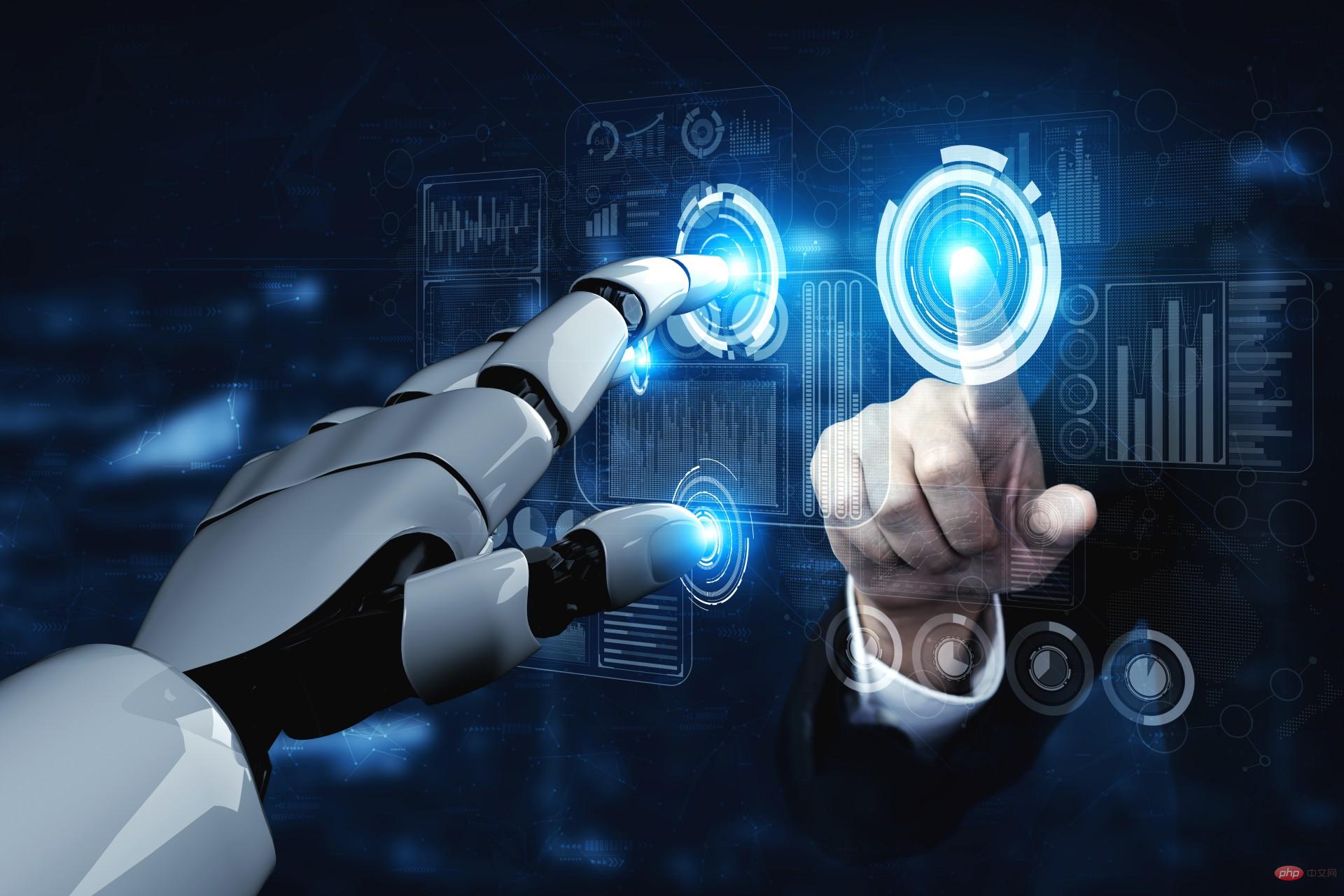
机器学习是一个不断发展的学科,一直在创造新的想法和技术。本文罗列了2023年机器学习的十大概念和技术。 本文罗列了2023年机器学习的十大概念和技术。2023年机器学习的十大概念和技术是一个教计算机从数据中学习的过程,无需明确的编程。机器学习是一个不断发展的学科,一直在创造新的想法和技术。为了保持领先,数据科学家应该关注其中一些网站,以跟上最新的发展。这将有助于了解机器学习中的技术如何在实践中使用,并为自己的业务或工作领域中的可能应用提供想法。2023年机器学习的十大概念和技术:1. 深度神经网
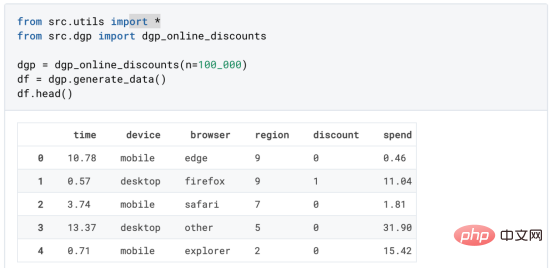
译者 | 朱先忠审校 | 孙淑娟在我之前的博客中,我们已经了解了如何使用因果树来评估政策的异质处理效应。如果你还没有阅读过,我建议你在阅读本文前先读一遍,因为我们在本文中认为你已经了解了此文中的部分与本文相关的内容。为什么是异质处理效应(HTE:heterogenous treatment effects)呢?首先,对异质处理效应的估计允许我们根据它们的预期结果(疾病、公司收入、客户满意度等)选择提供处理(药物、广告、产品等)的用户(患者、用户、客户等)。换句话说,估计HTE有助于我
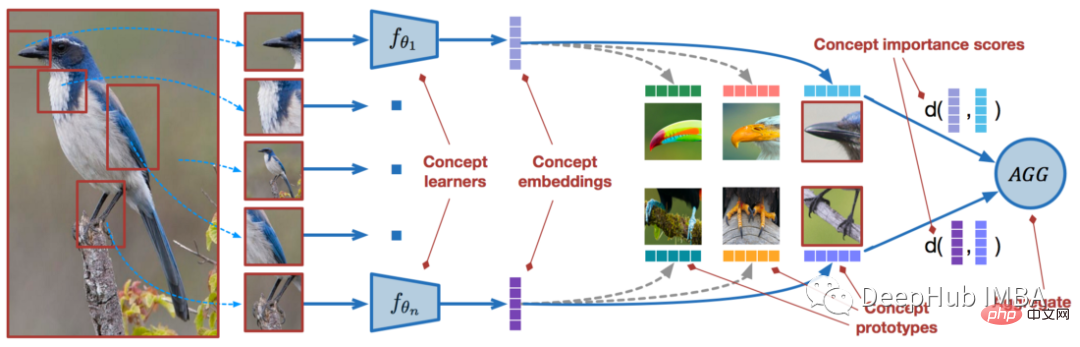
近年来,基于深度学习的模型在目标检测和图像识别等任务中表现出色。像ImageNet这样具有挑战性的图像分类数据集,包含1000种不同的对象分类,现在一些模型已经超过了人类水平上。但是这些模型依赖于监督训练流程,标记训练数据的可用性对它们有重大影响,并且模型能够检测到的类别也仅限于它们接受训练的类。由于在训练过程中没有足够的标记图像用于所有类,这些模型在现实环境中可能不太有用。并且我们希望的模型能够识别它在训练期间没有见到过的类,因为几乎不可能在所有潜在对象的图像上进行训练。我们将从几个样本中学习
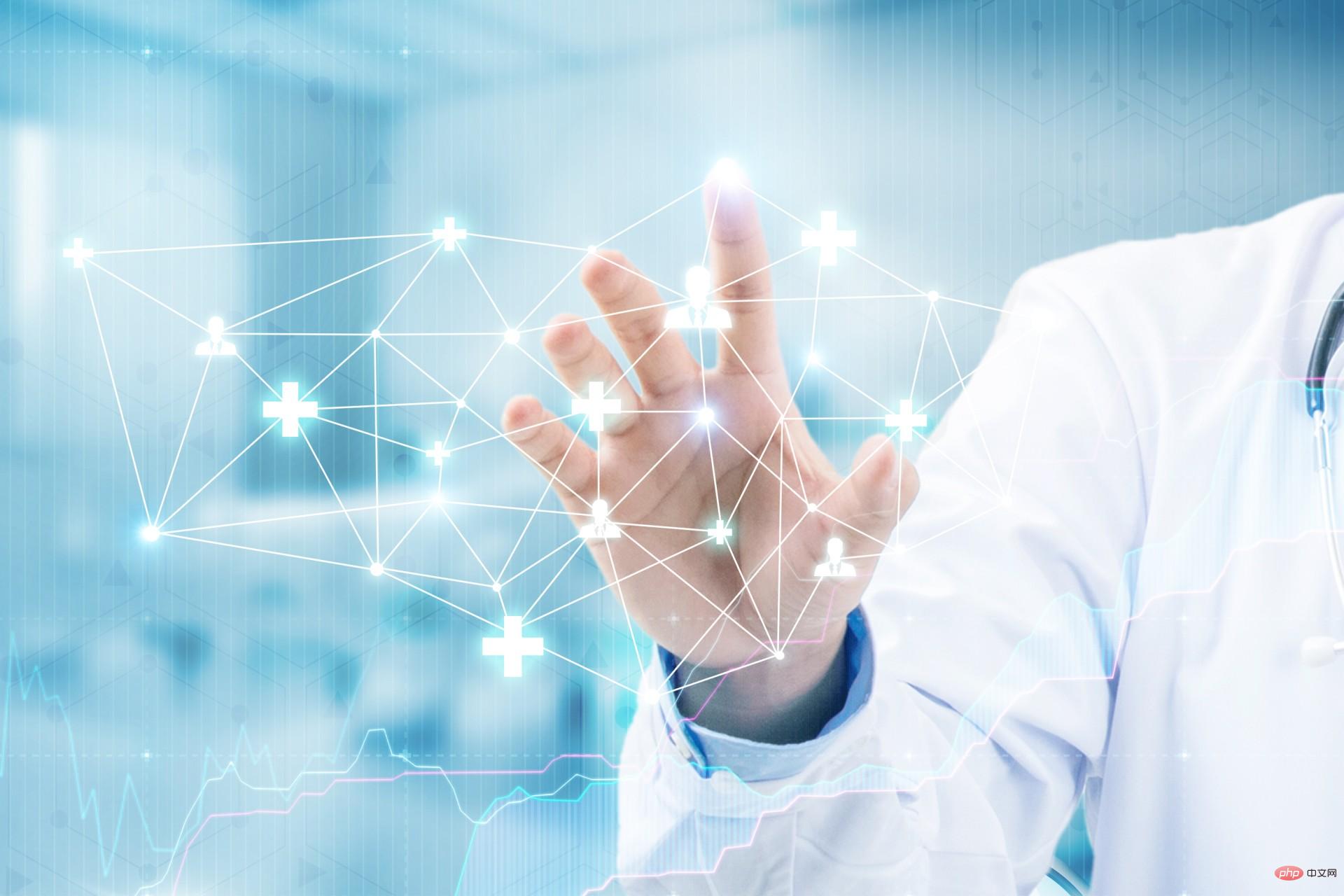
本文讨论使用LazyPredict来创建简单的ML模型。LazyPredict创建机器学习模型的特点是不需要大量的代码,同时在不修改参数的情况下进行多模型拟合,从而在众多模型中选出性能最佳的一个。 摘要本文讨论使用LazyPredict来创建简单的ML模型。LazyPredict创建机器学习模型的特点是不需要大量的代码,同时在不修改参数的情况下进行多模型拟合,从而在众多模型中选出性能最佳的一个。本文包括的内容如下:简介LazyPredict模块的安装在分类模型中实施LazyPredict
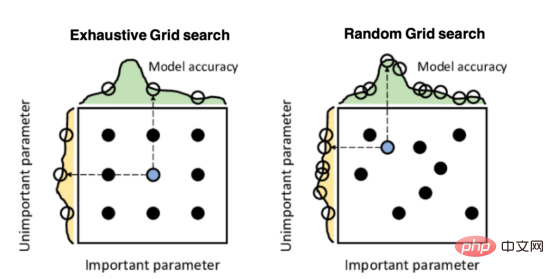
译者 | 朱先忠审校 | 孙淑娟引言模型超参数(或模型设置)的优化可能是训练机器学习算法中最重要的一步,因为它可以找到最小化模型损失函数的最佳参数。这一步对于构建不易过拟合的泛化模型也是必不可少的。优化模型超参数的最著名技术是穷举网格搜索和随机网格搜索。在第一种方法中,搜索空间被定义为跨越每个模型超参数的域的网格。通过在网格的每个点上训练模型来获得最优超参数。尽管网格搜索非常容易实现,但它在计算上变得昂贵,尤其是当要优化的变量数量很大时。另一方面,随机网格搜索是一种更快的优化方法,可以提供更好的
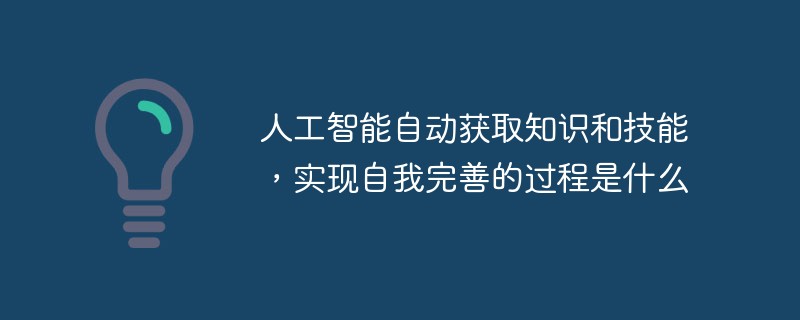
实现自我完善的过程是“机器学习”。机器学习是人工智能核心,是使计算机具有智能的根本途径;它使计算机能模拟人的学习行为,自动地通过学习来获取知识和技能,不断改善性能,实现自我完善。机器学习主要研究三方面问题:1、学习机理,人类获取知识、技能和抽象概念的天赋能力;2、学习方法,对生物学习机理进行简化的基础上,用计算的方法进行再现;3、学习系统,能够在一定程度上实现机器学习的系统。

本文将详细介绍用来提高机器学习效果的最常见的超参数优化方法。 译者 | 朱先忠审校 | 孙淑娟简介通常,在尝试改进机器学习模型时,人们首先想到的解决方案是添加更多的训练数据。额外的数据通常是有帮助(在某些情况下除外)的,但生成高质量的数据可能非常昂贵。通过使用现有数据获得最佳模型性能,超参数优化可以节省我们的时间和资源。顾名思义,超参数优化是为机器学习模型确定最佳超参数组合以满足优化函数(即,给定研究中的数据集,最大化模型的性能)的过程。换句话说,每个模型都会提供多个有关选项的调整“按钮


Hot AI Tools

Undresser.AI Undress
AI-powered app for creating realistic nude photos

AI Clothes Remover
Online AI tool for removing clothes from photos.

Undress AI Tool
Undress images for free

Clothoff.io
AI clothes remover

AI Hentai Generator
Generate AI Hentai for free.

Hot Article

Hot Tools
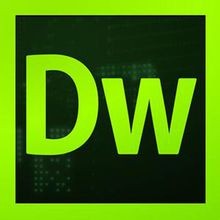
Dreamweaver CS6
Visual web development tools
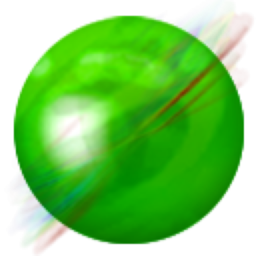
ZendStudio 13.5.1 Mac
Powerful PHP integrated development environment

Atom editor mac version download
The most popular open source editor
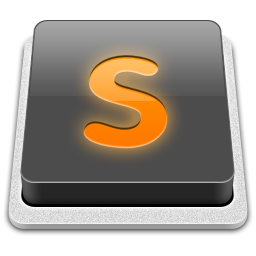
SublimeText3 Mac version
God-level code editing software (SublimeText3)

Safe Exam Browser
Safe Exam Browser is a secure browser environment for taking online exams securely. This software turns any computer into a secure workstation. It controls access to any utility and prevents students from using unauthorized resources.
