What are the classification methods to deal with imbalanced data sets?
In the field of machine learning, imbalanced data sets are a common problem, which refers to the large difference in the number of samples of different categories in the training data set. For example, in a binary classification problem, the number of positive samples is much smaller than the number of negative samples. This will cause the trained model to be more inclined to predict a larger number of categories and ignore a smaller number of categories, thus affecting the performance of the model. Therefore, imbalanced data sets need to be classified to improve model performance.
This article will use a specific example to illustrate how to classify imbalanced data sets. Suppose we have a binary classification problem where the number of positive samples is 100, the number of negative samples is 1000, and the dimension of the feature vector is 10. In order to deal with imbalanced data sets, the following steps can be taken: 1. Use undersampling or oversampling techniques to balance the data, such as the SMOTE algorithm. 2. Use appropriate evaluation indicators, such as accuracy, precision, recall, etc., to evaluate the performance of the model. 3. Adjust the threshold of the classifier to optimize the model’s performance on minority classes. 4. Use ensemble learning methods, such as random forests or gradient boosting trees, to improve the generalization performance of the model
1. Understand the data set: Analyze the data set and find the number of positive samples Much smaller than the number of negative samples.
2. Choose appropriate evaluation indicators: Due to the imbalance of the data set, we choose precision, recall and F1 value as evaluation indicators.
You can use the SMOTE algorithm to synthesize minority class samples and balance the data set. This can be implemented using the imblearn library.
from imblearn.over_sampling import SMOTE from sklearn.model_selection import train_test_split from sklearn.linear_model import LogisticRegression from sklearn.metrics import accuracy_score, recall_score, f1_score # 加载数据集并划分训练集和测试集 X_train, X_test, y_train, y_test = train_test_split(X, y, test_size=0.2, random_state=42) # 使用SMOTE算法进行数据重采样 smote = SMOTE(random_state=42) X_train_resampled, y_train_resampled = smote.fit_resample(X_train, y_train) # 训练逻辑回归模型 model = LogisticRegression(random_state=42) model.fit(X_train_resampled, y_train_resampled) # 在测试集上进行预测 y_pred = model.predict(X_test) # 计算评估指标 accuracy = accuracy_score(y_test, y_pred) recall = recall_score(y_test, y_pred) f1 = f1_score(y_test, y_pred) print("Accuracy: {:.2f}%, Recall: {:.2f}%, F1: {:.2f}%".format(accuracy*100, recall*100, f1*100))
4. Classification algorithm adjustment: When training the model, you can set category weights to balance the data set. For example, in the logistic regression algorithm, the class_weight parameter can be set to balance the number of samples in different categories.
# 训练逻辑回归模型并设置类别权重 model = LogisticRegression(random_state=42, class_weight="balanced") model.fit(X_train, y_train) # 在测试集上进行预测 y_pred = model.predict(X_test) # 计算评估指标 accuracy = accuracy_score(y_test, y_pred) recall = recall_score(y_test, y_pred) f1 = f1_score(y_test, y_pred) print("Accuracy: {:.2f}%, Recall: {:.2f}%, F1: {:.2f}%".format(accuracy*100, recall*100, f1*100))
5. Ensemble learning algorithm: We can use the random forest algorithm for ensemble learning. Specifically, it can be implemented using the sklearn library in Python:
from sklearn.ensemble import RandomForestClassifier # 训练随机森林模型 model = RandomForestClassifier(random_state=42) model.fit(X_train, y_train) # 在测试集上进行预测 y_pred = model.predict(X_test) # 计算评估指标 accuracy = accuracy_score(y_test, y_pred) recall = recall_score(y_test, y_pred) f1 = f1_score(y_test, y_pred) print("Accuracy: {:.2f}%, Recall: {:.2f}%, F1: {:.2f}%".format(accuracy*100, recall*100, f1*100))
In summary, methods for dealing with imbalanced data sets include data resampling, classification algorithm adjustment, and ensemble learning algorithms. The appropriate method needs to be selected based on the specific problem, and the model needs to be evaluated and adjusted to achieve better performance.
The above is the detailed content of What are the classification methods to deal with imbalanced data sets?. For more information, please follow other related articles on the PHP Chinese website!
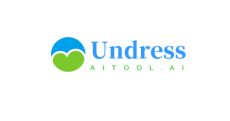
https://undressaitool.ai/ is Powerful mobile app with advanced AI features for adult content. Create AI-generated pornographic images or videos now!
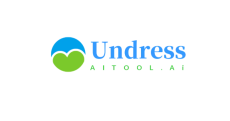
Tutorial on using undressAI to create pornographic pictures/videos: 1. Open the corresponding tool web link; 2. Click the tool button; 3. Upload the required content for production according to the page prompts; 4. Save and enjoy the results.
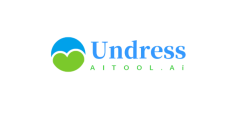
The official address of undress AI is:https://undressaitool.ai/;undressAI is Powerful mobile app with advanced AI features for adult content. Create AI-generated pornographic images or videos now!
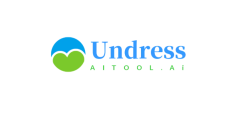
Tutorial on using undressAI to create pornographic pictures/videos: 1. Open the corresponding tool web link; 2. Click the tool button; 3. Upload the required content for production according to the page prompts; 4. Save and enjoy the results.
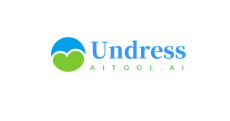
The official address of undress AI is:https://undressaitool.ai/;undressAI is Powerful mobile app with advanced AI features for adult content. Create AI-generated pornographic images or videos now!
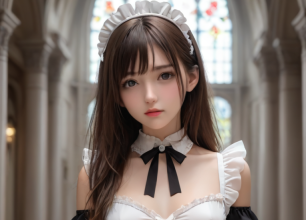
Tutorial on using undressAI to create pornographic pictures/videos: 1. Open the corresponding tool web link; 2. Click the tool button; 3. Upload the required content for production according to the page prompts; 4. Save and enjoy the results.
![[Ghibli-style images with AI] Introducing how to create free images with ChatGPT and copyright](https://img.php.cn/upload/article/001/242/473/174707263295098.jpg?x-oss-process=image/resize,p_40)
The latest model GPT-4o released by OpenAI not only can generate text, but also has image generation functions, which has attracted widespread attention. The most eye-catching feature is the generation of "Ghibli-style illustrations". Simply upload the photo to ChatGPT and give simple instructions to generate a dreamy image like a work in Studio Ghibli. This article will explain in detail the actual operation process, the effect experience, as well as the errors and copyright issues that need to be paid attention to. For details of the latest model "o3" released by OpenAI, please click here⬇️ Detailed explanation of OpenAI o3 (ChatGPT o3): Features, pricing system and o4-mini introduction Please click here for the English version of Ghibli-style article⬇️ Create Ji with ChatGPT
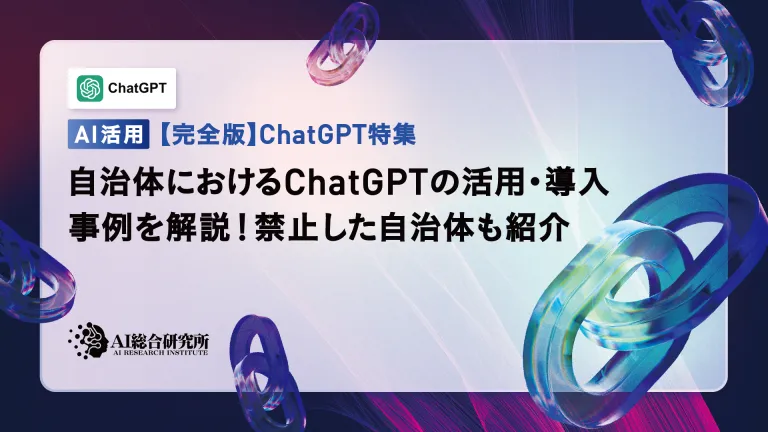
As a new communication method, the use and introduction of ChatGPT in local governments is attracting attention. While this trend is progressing in a wide range of areas, some local governments have declined to use ChatGPT. In this article, we will introduce examples of ChatGPT implementation in local governments. We will explore how we are achieving quality and efficiency improvements in local government services through a variety of reform examples, including supporting document creation and dialogue with citizens. Not only local government officials who aim to reduce staff workload and improve convenience for citizens, but also all interested in advanced use cases.


Hot AI Tools

Undresser.AI Undress
AI-powered app for creating realistic nude photos

AI Clothes Remover
Online AI tool for removing clothes from photos.

Undress AI Tool
Undress images for free

Clothoff.io
AI clothes remover

Video Face Swap
Swap faces in any video effortlessly with our completely free AI face swap tool!

Hot Article

Hot Tools
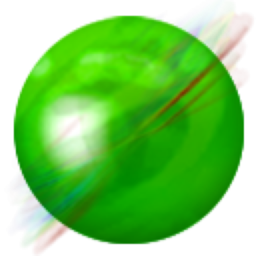
ZendStudio 13.5.1 Mac
Powerful PHP integrated development environment

SecLists
SecLists is the ultimate security tester's companion. It is a collection of various types of lists that are frequently used during security assessments, all in one place. SecLists helps make security testing more efficient and productive by conveniently providing all the lists a security tester might need. List types include usernames, passwords, URLs, fuzzing payloads, sensitive data patterns, web shells, and more. The tester can simply pull this repository onto a new test machine and he will have access to every type of list he needs.
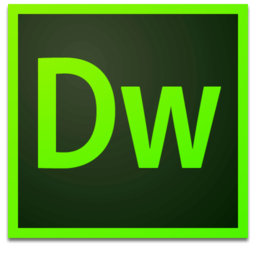
Dreamweaver Mac version
Visual web development tools
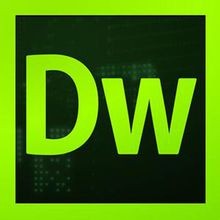
Dreamweaver CS6
Visual web development tools

SublimeText3 Chinese version
Chinese version, very easy to use
