The LLM-driven response engine is a response engine that uses large language models (LLM) as the core technology. LLM is a natural language processing technology based on deep learning. It learns the syntax, semantics and contextual information of natural language from massive text data through large-scale training, and generates natural and smooth text. The LLM-driven response engine can be applied to various scenarios. From a technical perspective, the LLM-driven response engine uses a pre-trained model to generate corresponding answers through the model's reasoning and generation capabilities by inputting questions or conversations. This technology is based on large amounts of training data and can generate high-quality, accurate answers. In terms of application scenarios, the LLM-driven response engine can be used in fields such as intelligent customer service, intelligent assistants, and intelligent question and answer systems. It can help users answer various questions and provide personalized service and support. In terms of development trends, with the development of big data and deep learning technology, LLM-driven response engines will continue to improve their language understanding and generation capabilities. In the future, it is expected to become
1. Technical Principles
1.1 Basic Principles of LLM
LLM is a natural language processing technology based on deep neural networks. Its basic principle is By training a neural network model to predict the probability distribution of the next word, the functions of text generation and understanding are achieved. Usually, LLM uses deep neural network structures such as Transformer to achieve this goal.
1.2 Technical implementation of response engine
The LLM-driven response engine mainly has two parts: input processing and output generation. Input processing is responsible for performing natural language processing operations such as word segmentation, part-of-speech tagging, and entity recognition on the natural language text input by the user to obtain structured information that represents the user's intention. Output generation uses LLM to generate smooth and natural text as answers based on this structured information.
2. Application scenarios
2.1 Chat robot
LLM-driven response engine is widely used in chat robots. Through training on large-scale dialogue data, the LLM model can learn the syntax, semantics and contextual information of natural language dialogue, thereby achieving smooth and natural dialogue responses.
2.2 Voice Assistant
The LLM-driven response engine can also be used in voice assistants. By converting speech into text, the response engine can recognize the user's intention and generate a corresponding answer, thereby making the voice assistant intelligent and natural.
2.3 Intelligent customer service
The LLM-driven response engine can also be used in intelligent customer service. By training large-scale customer service conversation data, the response engine can learn professional knowledge in different fields and intelligently answer user questions, improving customer satisfaction and service efficiency.
3. Development Trend
3.1 Continuous optimization of the model
With the continuous development of deep learning technology, the accuracy and efficiency of the LLM model are also constantly improving. In the future, the LLM-driven response engine will be more accurate and efficient, and can better adapt to the needs of different scenarios.
3.2 Multi-modal fusion
In the future, the LLM-driven response engine will pay more attention to multi-modal fusion. In addition to text input, it can also support multiple input methods such as images, voice, and video, and can generate corresponding answers based on different input methods.
3.3 Personalized customization
In the future, the LLM-driven response engine will pay more attention to personalized customization. Through the analysis of user historical conversation data, targeted answers can be achieved to improve user experience and satisfaction.
In short, the LLM-driven response engine is an intelligent natural language processing technology based on deep learning technology and has a wide range of application scenarios and development prospects.
The above is the detailed content of Disassembling the LLM-driven response engine. For more information, please follow other related articles on the PHP Chinese website!
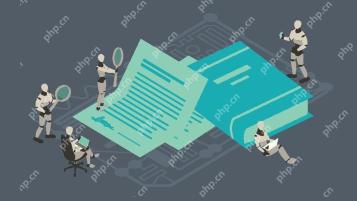
The legal tech revolution is gaining momentum, pushing legal professionals to actively embrace AI solutions. Passive resistance is no longer a viable option for those aiming to stay competitive. Why is Technology Adoption Crucial? Legal professional
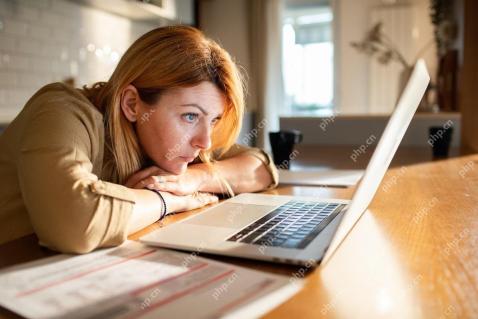
Many assume interactions with AI are anonymous, a stark contrast to human communication. However, AI actively profiles users during every chat. Every prompt, every word, is analyzed and categorized. Let's explore this critical aspect of the AI revo
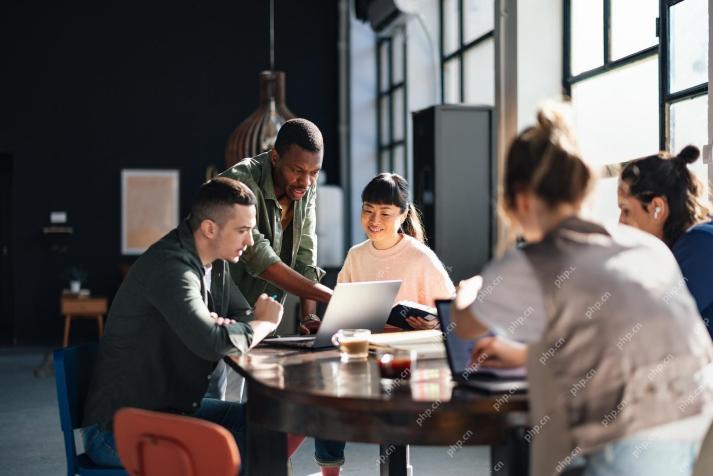
A successful artificial intelligence strategy cannot be separated from strong corporate culture support. As Peter Drucker said, business operations depend on people, and so does the success of artificial intelligence. For organizations that actively embrace artificial intelligence, building a corporate culture that adapts to AI is crucial, and it even determines the success or failure of AI strategies. West Monroe recently released a practical guide to building a thriving AI-friendly corporate culture, and here are some key points: 1. Clarify the success model of AI: First of all, we must have a clear vision of how AI can empower business. An ideal AI operation culture can achieve a natural integration of work processes between humans and AI systems. AI is good at certain tasks, while humans are good at creativity and judgment
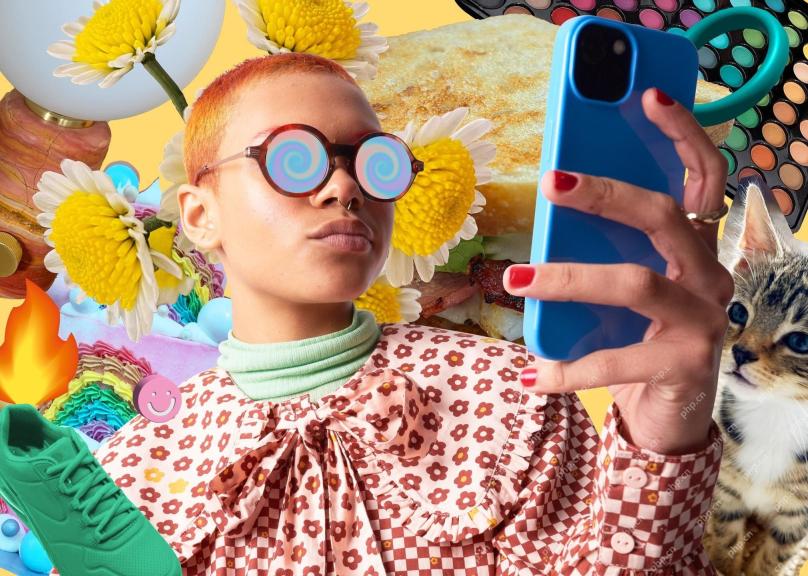
Meta upgrades AI assistant application, and the era of wearable AI is coming! The app, designed to compete with ChatGPT, offers standard AI features such as text, voice interaction, image generation and web search, but has now added geolocation capabilities for the first time. This means that Meta AI knows where you are and what you are viewing when answering your question. It uses your interests, location, profile and activity information to provide the latest situational information that was not possible before. The app also supports real-time translation, which completely changed the AI experience on Ray-Ban glasses and greatly improved its usefulness. The imposition of tariffs on foreign films is a naked exercise of power over the media and culture. If implemented, this will accelerate toward AI and virtual production
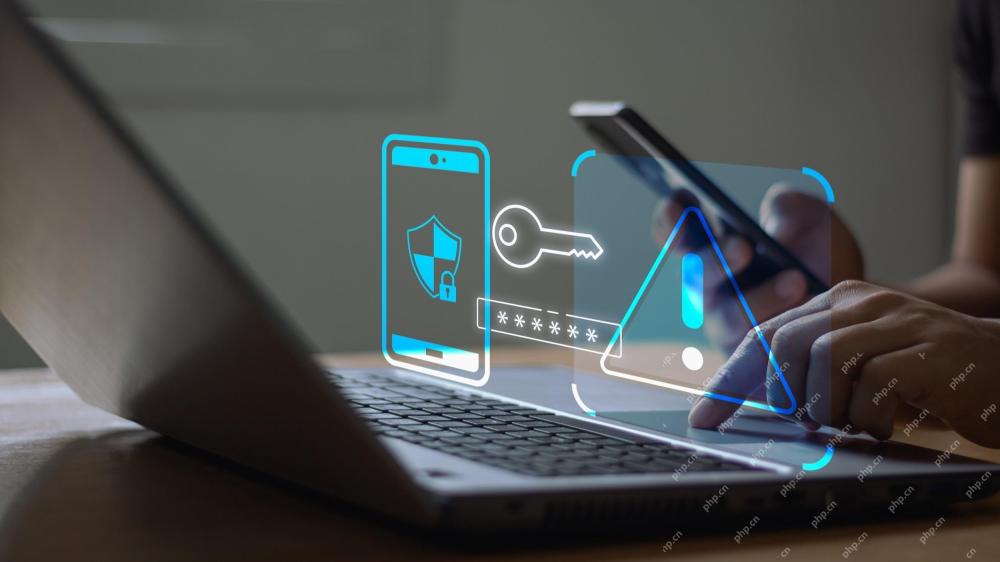
Artificial intelligence is revolutionizing the field of cybercrime, which forces us to learn new defensive skills. Cyber criminals are increasingly using powerful artificial intelligence technologies such as deep forgery and intelligent cyberattacks to fraud and destruction at an unprecedented scale. It is reported that 87% of global businesses have been targeted for AI cybercrime over the past year. So, how can we avoid becoming victims of this wave of smart crimes? Let’s explore how to identify risks and take protective measures at the individual and organizational level. How cybercriminals use artificial intelligence As technology advances, criminals are constantly looking for new ways to attack individuals, businesses and governments. The widespread use of artificial intelligence may be the latest aspect, but its potential harm is unprecedented. In particular, artificial intelligence
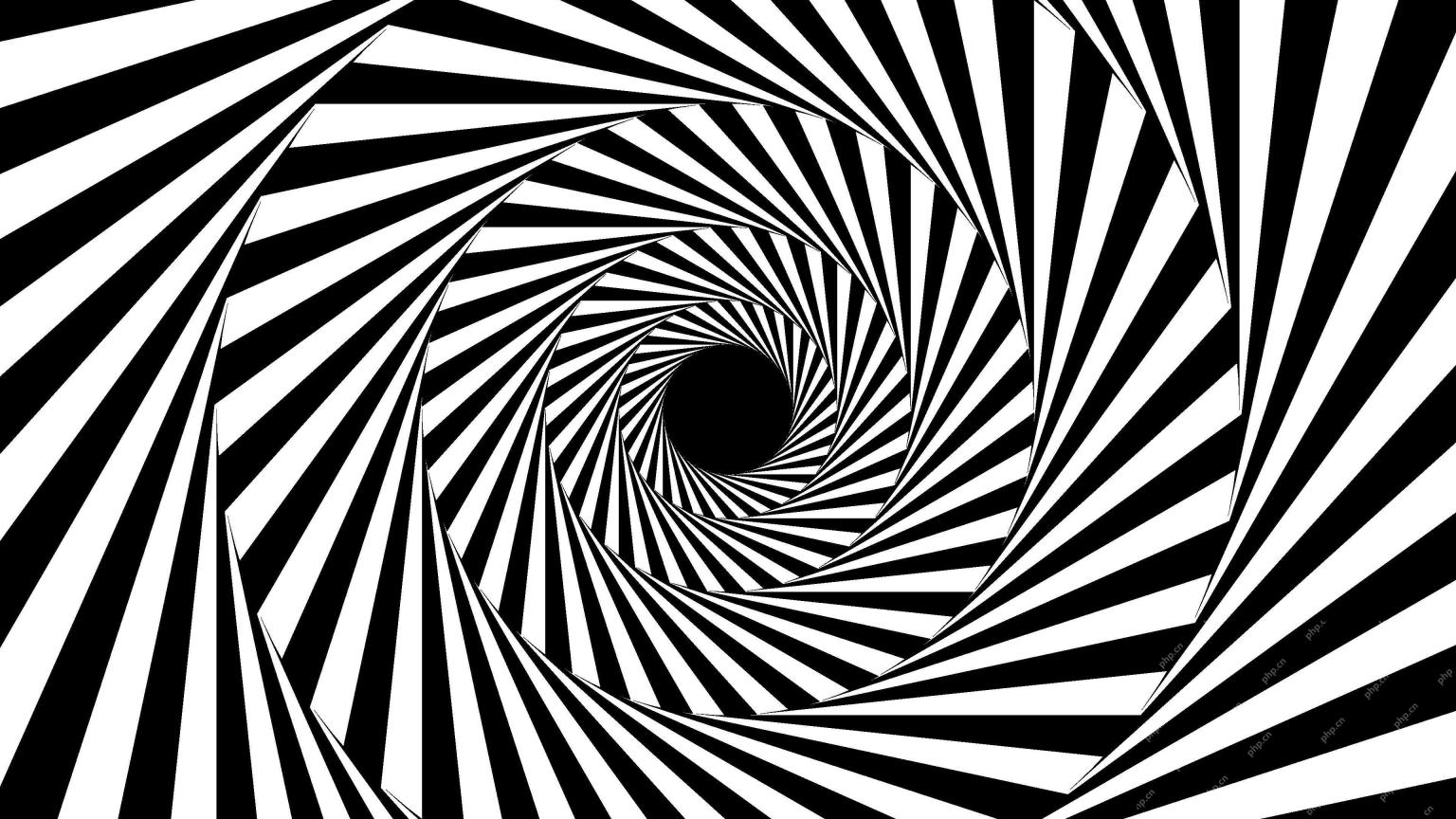
The intricate relationship between artificial intelligence (AI) and human intelligence (NI) is best understood as a feedback loop. Humans create AI, training it on data generated by human activity to enhance or replicate human capabilities. This AI
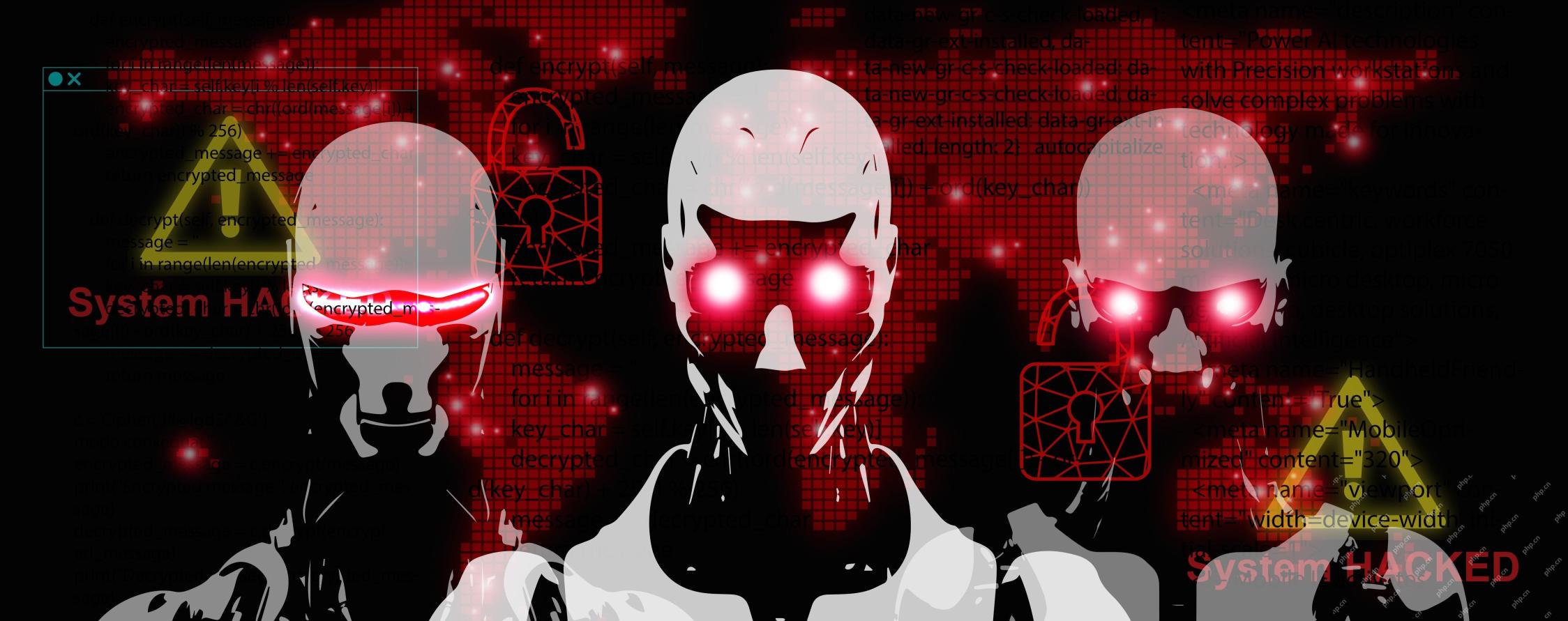
Anthropic's recent statement, highlighting the lack of understanding surrounding cutting-edge AI models, has sparked a heated debate among experts. Is this opacity a genuine technological crisis, or simply a temporary hurdle on the path to more soph
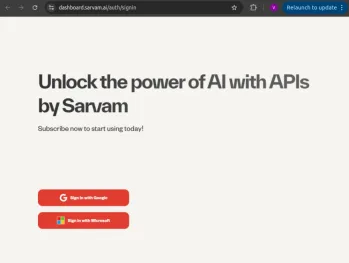
India is a diverse country with a rich tapestry of languages, making seamless communication across regions a persistent challenge. However, Sarvam’s Bulbul-V2 is helping to bridge this gap with its advanced text-to-speech (TTS) t


Hot AI Tools

Undresser.AI Undress
AI-powered app for creating realistic nude photos

AI Clothes Remover
Online AI tool for removing clothes from photos.

Undress AI Tool
Undress images for free

Clothoff.io
AI clothes remover

Video Face Swap
Swap faces in any video effortlessly with our completely free AI face swap tool!

Hot Article

Hot Tools

Safe Exam Browser
Safe Exam Browser is a secure browser environment for taking online exams securely. This software turns any computer into a secure workstation. It controls access to any utility and prevents students from using unauthorized resources.
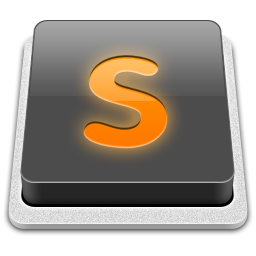
SublimeText3 Mac version
God-level code editing software (SublimeText3)

mPDF
mPDF is a PHP library that can generate PDF files from UTF-8 encoded HTML. The original author, Ian Back, wrote mPDF to output PDF files "on the fly" from his website and handle different languages. It is slower than original scripts like HTML2FPDF and produces larger files when using Unicode fonts, but supports CSS styles etc. and has a lot of enhancements. Supports almost all languages, including RTL (Arabic and Hebrew) and CJK (Chinese, Japanese and Korean). Supports nested block-level elements (such as P, DIV),

Notepad++7.3.1
Easy-to-use and free code editor
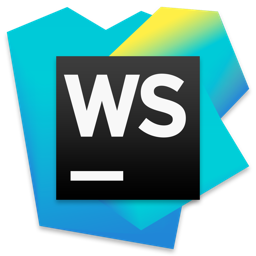
WebStorm Mac version
Useful JavaScript development tools
