CycleGAN is an image conversion model based on deep learning. It can convert one type of image into another type of image by learning the mapping relationship between two fields. For example, it can convert an image of a horse into an image of a zebra, an image of a summer scene into an image of a winter scene, and so on. This image conversion technology has broad application prospects and can play an important role in fields such as computer vision, virtual reality, game development, and image enhancement. Through CycleGAN, we can achieve cross-domain image conversion and provide more flexible and diverse image processing solutions for various application scenarios.
The background of CycleGAN can be traced back to 2017, proposed by Zhu Junyan and others in the paper "Unpaired Image-to-Image Translation using Cycle-Consistent Adversarial Networks". In previous image conversion methods, pairs of image data are usually required for training. For example, if you want to convert a black-and-white image into a color image, you need a set of black-and-white images and corresponding color images. However, in practical applications, it is difficult to obtain such paired image data, which limits the application scope of traditional methods. Therefore, CycleGAN proposes an image conversion method that does not require paired image data and can convert between images in different fields, such as converting photos into works of art, converting dog images into wolf images, and so on. This method achieves unsupervised image transformation through a combination of adversarial networks and cycle consistency loss functions. Specifically, CycleGAN contains two generators and two discriminators, which are used to convert images from one domain to another and make authenticity judgments on the generated images. By optimizing the adversarial training between the generator and the discriminator, CycleGAN can learn the mapping relationship between the two fields, thereby achieving unsupervised image conversion. The innovation of this method is that it does not require paired image data as training samples, but uses a cycle consistency loss function to ensure the consistency between the generated image and the original image. In this way, CycleGAN has made great breakthroughs in the field of image conversion, bringing greater flexibility and feasibility to practical applications.
The function of CycleGAN is to achieve conversion between images in different fields. It implements image conversion from A to B and B to A through two generators and two discriminators. The generator learns image transformations through adversarial training, with the goal of minimizing the difference between the generated and real images. The discriminator distinguishes real and fake images by maximizing the difference between real images and generated images. Through this adversarial learning method, CycleGAN can achieve high-quality image conversion, allowing images in domain A to be converted into images in domain B, while maintaining the consistency and authenticity of the image. This method has wide applications in many fields, such as style transfer, image conversion, and image enhancement.
An important feature of CycleGAN is that it uses the cycle consistency loss function to ensure the consistency of image transformation. Specifically, for image conversion from A to B and image conversion from B to A, CycleGAN requires the generated image to be as close to the original image as possible after being converted back to the original domain to avoid inconsistent conversions. For example, convert an image of a horse into an image of a zebra, and then convert the image of a zebra back into an image of a horse. The final image should be consistent with the original image of a horse. Through the cycle consistency loss function, CycleGAN can improve the quality and consistency of image conversion, making the generated images more realistic and credible.
In addition to using the cycle consistency loss function, CycleGAN also uses conditional generative adversarial networks to achieve conditional image transformation. This means that the generator can receive condition information. For example, when converting a summer scenery into a winter scenery, the winter condition information can be passed to the generator to help it better learn the characteristics of the winter scenery. This approach allows the generator to more accurately generate images that meet the conditions.
In general, the emergence of CycleGAN solves the limitation of pairwise image data in traditional image conversion methods, making image conversion more flexible and practical. At present, CycleGAN has been widely used in image style conversion, image enhancement, virtual reality and other fields, and has achieved good results in the field of image generation.
The above is the detailed content of Image transformation model using deep learning: CycleGAN. For more information, please follow other related articles on the PHP Chinese website!
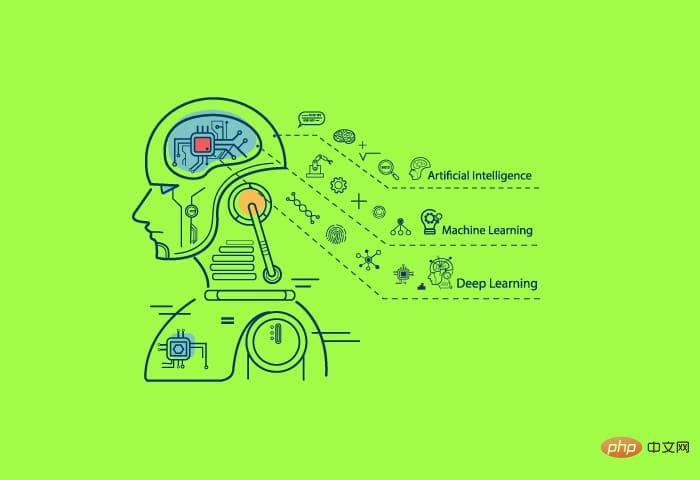
人工智能Artificial Intelligence(AI)、机器学习Machine Learning(ML)和深度学习Deep Learning(DL)通常可以互换使用。但是,它们并不完全相同。人工智能是最广泛的概念,它赋予机器模仿人类行为的能力。机器学习是将人工智能应用到系统或机器中,帮助其自我学习和不断改进。最后,深度学习使用复杂的算法和深度神经网络来重复训练特定的模型或模式。让我们看看每个术语的演变和历程,以更好地理解人工智能、机器学习和深度学习实际指的是什么。人工智能自过去 70 多
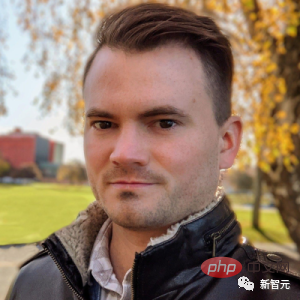
众所周知,在处理深度学习和神经网络任务时,最好使用GPU而不是CPU来处理,因为在神经网络方面,即使是一个比较低端的GPU,性能也会胜过CPU。深度学习是一个对计算有着大量需求的领域,从一定程度上来说,GPU的选择将从根本上决定深度学习的体验。但问题来了,如何选购合适的GPU也是件头疼烧脑的事。怎么避免踩雷,如何做出性价比高的选择?曾经拿到过斯坦福、UCL、CMU、NYU、UW 博士 offer、目前在华盛顿大学读博的知名评测博主Tim Dettmers就针对深度学习领域需要怎样的GPU,结合自
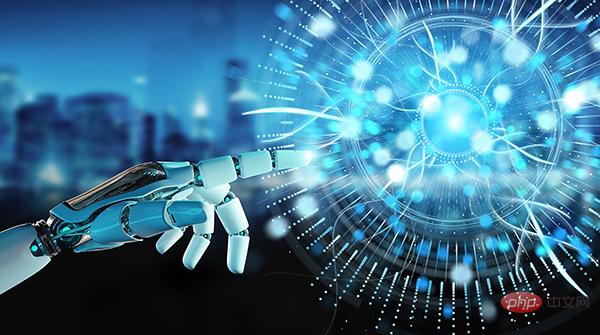
一. 背景介绍在字节跳动,基于深度学习的应用遍地开花,工程师关注模型效果的同时也需要关注线上服务一致性和性能,早期这通常需要算法专家和工程专家分工合作并紧密配合来完成,这种模式存在比较高的 diff 排查验证等成本。随着 PyTorch/TensorFlow 框架的流行,深度学习模型训练和在线推理完成了统一,开发者仅需要关注具体算法逻辑,调用框架的 Python API 完成训练验证过程即可,之后模型可以很方便的序列化导出,并由统一的高性能 C++ 引擎完成推理工作。提升了开发者训练到部署的体验
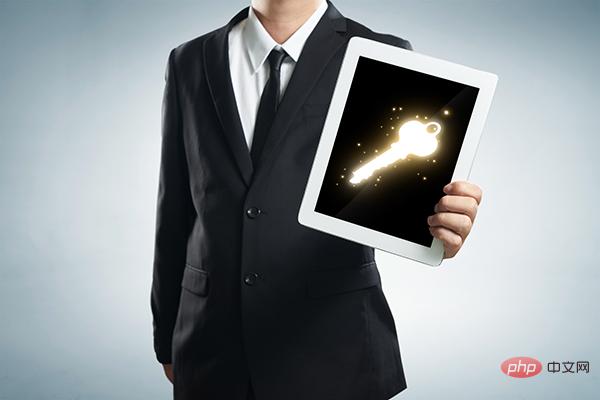
深度学习 (DL) 已成为计算机科学中最具影响力的领域之一,直接影响着当今人类生活和社会。与历史上所有其他技术创新一样,深度学习也被用于一些违法的行为。Deepfakes 就是这样一种深度学习应用,在过去的几年里已经进行了数百项研究,发明和优化各种使用 AI 的 Deepfake 检测,本文主要就是讨论如何对 Deepfake 进行检测。为了应对Deepfake,已经开发出了深度学习方法以及机器学习(非深度学习)方法来检测 。深度学习模型需要考虑大量参数,因此需要大量数据来训练此类模型。这正是
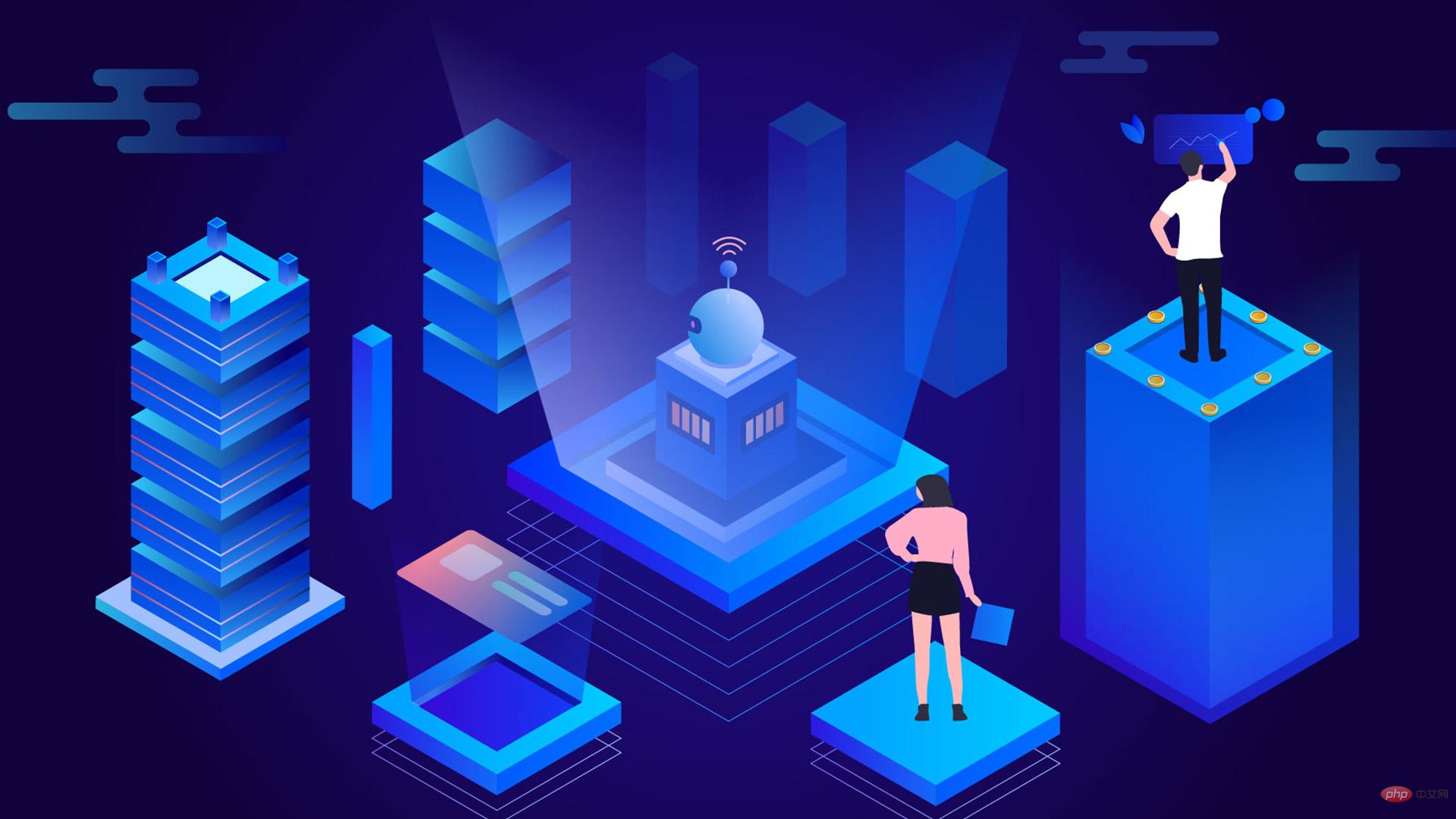
Part 01 概述 在实时音视频通信场景,麦克风采集用户语音的同时会采集大量环境噪声,传统降噪算法仅对平稳噪声(如电扇风声、白噪声、电路底噪等)有一定效果,对非平稳的瞬态噪声(如餐厅嘈杂噪声、地铁环境噪声、家庭厨房噪声等)降噪效果较差,严重影响用户的通话体验。针对泛家庭、办公等复杂场景中的上百种非平稳噪声问题,融合通信系统部生态赋能团队自主研发基于GRU模型的AI音频降噪技术,并通过算法和工程优化,将降噪模型尺寸从2.4MB压缩至82KB,运行内存降低约65%;计算复杂度从约186Mflop
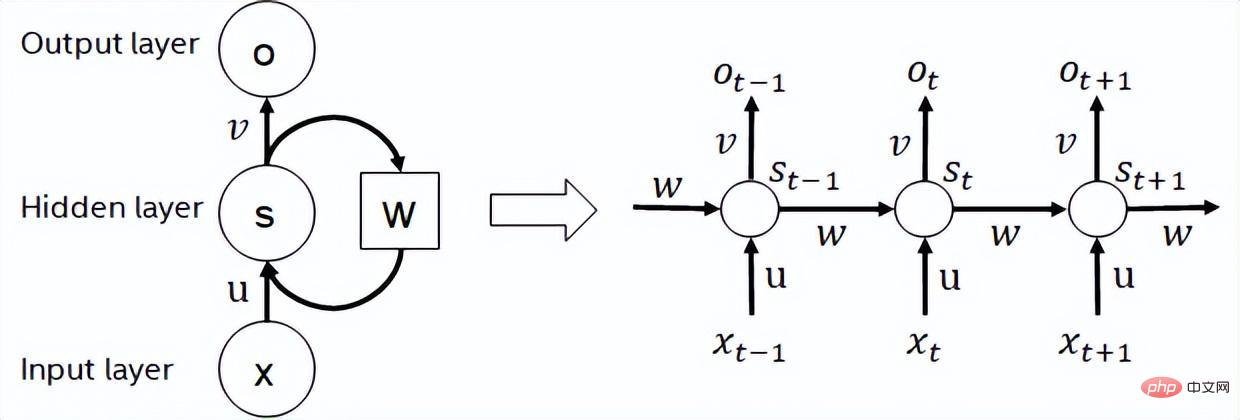
导读深度学习已在面向自然语言处理等领域的实际业务场景中广泛落地,对它的推理性能优化成为了部署环节中重要的一环。推理性能的提升:一方面,可以充分发挥部署硬件的能力,降低用户响应时间,同时节省成本;另一方面,可以在保持响应时间不变的前提下,使用结构更为复杂的深度学习模型,进而提升业务精度指标。本文针对地址标准化服务中的深度学习模型开展了推理性能优化工作。通过高性能算子、量化、编译优化等优化手段,在精度指标不降低的前提下,AI模型的模型端到端推理速度最高可获得了4.11倍的提升。1. 模型推理性能优化
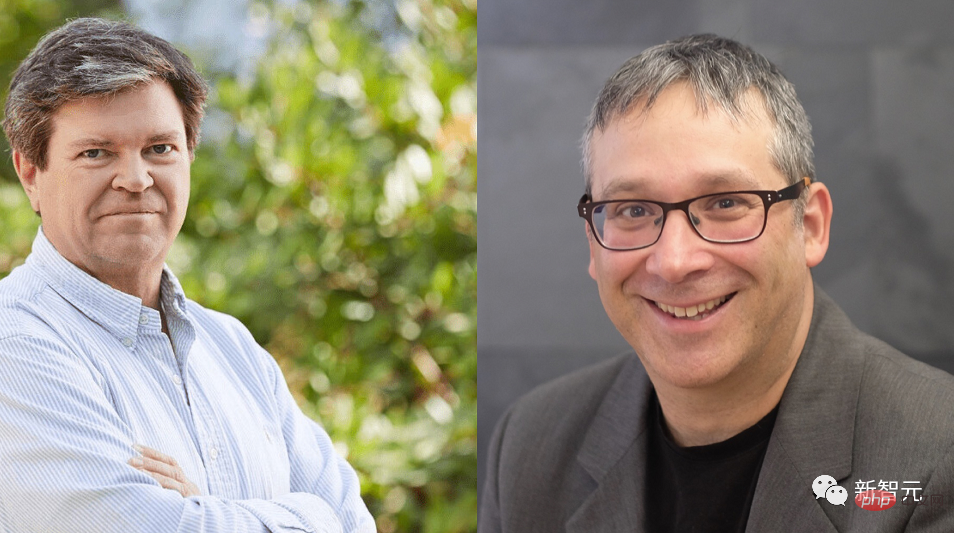
今天的主角,是一对AI界相爱相杀的老冤家:Yann LeCun和Gary Marcus在正式讲述这一次的「新仇」之前,我们先来回顾一下,两位大神的「旧恨」。LeCun与Marcus之争Facebook首席人工智能科学家和纽约大学教授,2018年图灵奖(Turing Award)得主杨立昆(Yann LeCun)在NOEMA杂志发表文章,回应此前Gary Marcus对AI与深度学习的评论。此前,Marcus在杂志Nautilus中发文,称深度学习已经「无法前进」Marcus此人,属于是看热闹的不
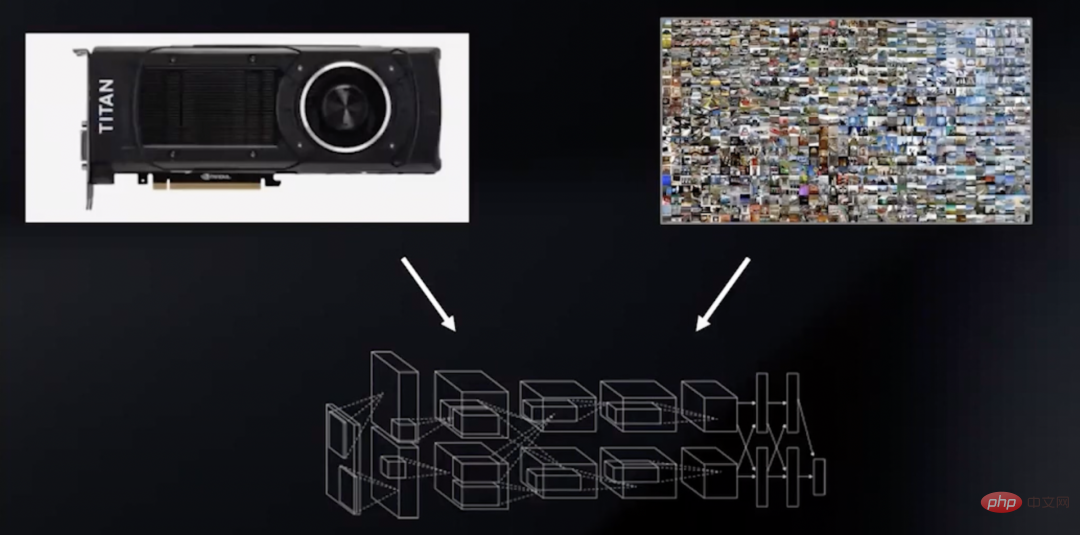
过去十年是深度学习的“黄金十年”,它彻底改变了人类的工作和娱乐方式,并且广泛应用到医疗、教育、产品设计等各行各业,而这一切离不开计算硬件的进步,特别是GPU的革新。 深度学习技术的成功实现取决于三大要素:第一是算法。20世纪80年代甚至更早就提出了大多数深度学习算法如深度神经网络、卷积神经网络、反向传播算法和随机梯度下降等。 第二是数据集。训练神经网络的数据集必须足够大,才能使神经网络的性能优于其他技术。直至21世纪初,诸如Pascal和ImageNet等大数据集才得以现世。 第三是硬件。只有


Hot AI Tools

Undresser.AI Undress
AI-powered app for creating realistic nude photos

AI Clothes Remover
Online AI tool for removing clothes from photos.

Undress AI Tool
Undress images for free

Clothoff.io
AI clothes remover

AI Hentai Generator
Generate AI Hentai for free.

Hot Article

Hot Tools
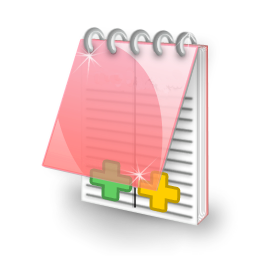
EditPlus Chinese cracked version
Small size, syntax highlighting, does not support code prompt function
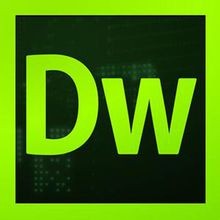
Dreamweaver CS6
Visual web development tools
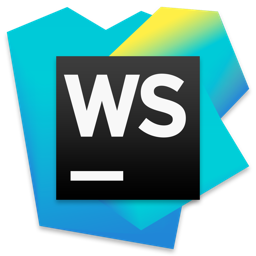
WebStorm Mac version
Useful JavaScript development tools
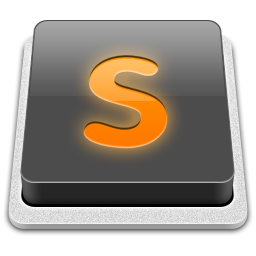
SublimeText3 Mac version
God-level code editing software (SublimeText3)

DVWA
Damn Vulnerable Web App (DVWA) is a PHP/MySQL web application that is very vulnerable. Its main goals are to be an aid for security professionals to test their skills and tools in a legal environment, to help web developers better understand the process of securing web applications, and to help teachers/students teach/learn in a classroom environment Web application security. The goal of DVWA is to practice some of the most common web vulnerabilities through a simple and straightforward interface, with varying degrees of difficulty. Please note that this software
