In machine learning, matrix operations are an important mathematical tool used to process and transform data. By representing data in matrix form, you can perform various linear algebra operations such as matrix multiplication, addition, and inverse operations. These operations can be used for feature transformation, parameter optimization, and predictive calculations during model training and inference. Through matrix operations, we can effectively process large-scale data sets and take advantage of parallel computing to speed up the calculation process. In addition, matrix operations are closely related to fields such as feature extraction, dimensionality reduction, and image processing, providing a powerful mathematical foundation and tools for machine learning.
Matrix operations are widely used in machine learning. Several common application scenarios will be introduced in detail below.
1. Linear regression
Linear regression is a machine learning algorithm used to establish linear relationships between variables. It fits the difference between data points and a linear model by minimizing an objective function. Typically, the objective function uses mean square error to measure the degree of fit. In order to solve for regression coefficients and make predictions, matrix operations can be performed using methods such as normal equations or gradient descent.
2. Principal component analysis (PCA)
Principal component analysis is a commonly used dimensionality reduction technique for extracting data from high-dimensional data. Focus on extracting the most important features. PCA maps the original data to a new feature space so that the correlation between the new features is minimal. This mapping process involves eigenvalue decomposition of the data covariance matrix and selecting the eigenvector corresponding to the largest eigenvalue as the principal component. Through matrix operations, the PCA algorithm can be effectively implemented.
3. Neural Network
Neural network is a machine learning model that simulates the working way of neurons in the human brain. Neural networks usually consist of multiple layers of neurons, with each neuron connected to all neurons in the next layer. In neural networks, inputs and weights are represented as matrices, and information is transferred and transformed through a combination of matrix multiplication and nonlinear activation functions. Matrix operations play an important role in neural networks, used to calculate the input and output of each neuron, as well as update network parameters (weights and biases).
4. Image processing
Image processing is another important application field in machine learning. Images are usually represented as a matrix of pixel values. By performing matrix operations on image matrices, various image processing tasks can be achieved, such as image filtering, edge detection, image enhancement, etc. Common image processing techniques, such as convolutional neural networks, use convolution operations to extract and classify images.
5. Recommendation system
The recommendation system is a key technology that helps users discover personalized interests. Recommendation systems based on matrix operations are often used to analyze the interaction between users and items and predict items that the user may be interested in. By representing user ratings or behavior records as matrices, methods such as matrix factorization can be used to infer hidden features between users and items to provide personalized recommendations.
To sum up, matrix operations in machine learning play an important role in multiple application scenarios. They can effectively process high-dimensional data, extract features, optimize model parameters, and implement complex data transformation and prediction tasks. By using matrix operations, we can better understand and utilize data and build more accurate and effective machine learning models.
The above is the detailed content of Matrix calculation and practice in machine learning. For more information, please follow other related articles on the PHP Chinese website!
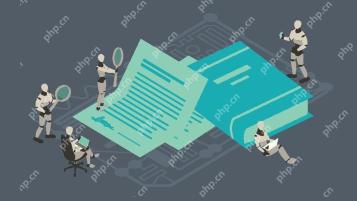
The legal tech revolution is gaining momentum, pushing legal professionals to actively embrace AI solutions. Passive resistance is no longer a viable option for those aiming to stay competitive. Why is Technology Adoption Crucial? Legal professional
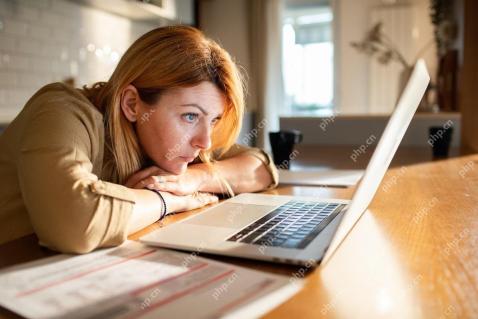
Many assume interactions with AI are anonymous, a stark contrast to human communication. However, AI actively profiles users during every chat. Every prompt, every word, is analyzed and categorized. Let's explore this critical aspect of the AI revo
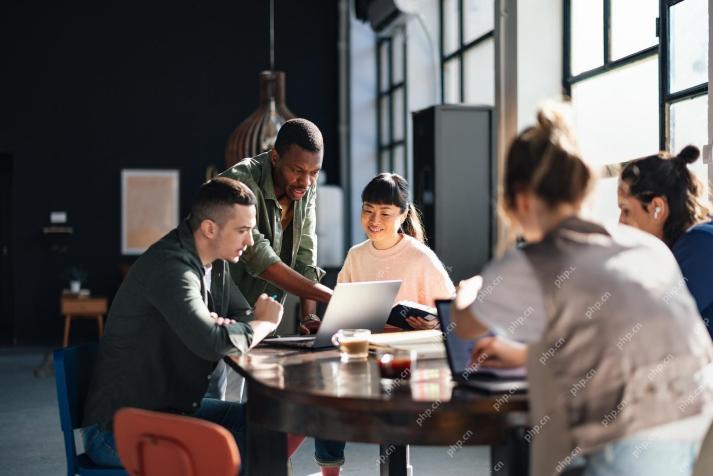
A successful artificial intelligence strategy cannot be separated from strong corporate culture support. As Peter Drucker said, business operations depend on people, and so does the success of artificial intelligence. For organizations that actively embrace artificial intelligence, building a corporate culture that adapts to AI is crucial, and it even determines the success or failure of AI strategies. West Monroe recently released a practical guide to building a thriving AI-friendly corporate culture, and here are some key points: 1. Clarify the success model of AI: First of all, we must have a clear vision of how AI can empower business. An ideal AI operation culture can achieve a natural integration of work processes between humans and AI systems. AI is good at certain tasks, while humans are good at creativity and judgment
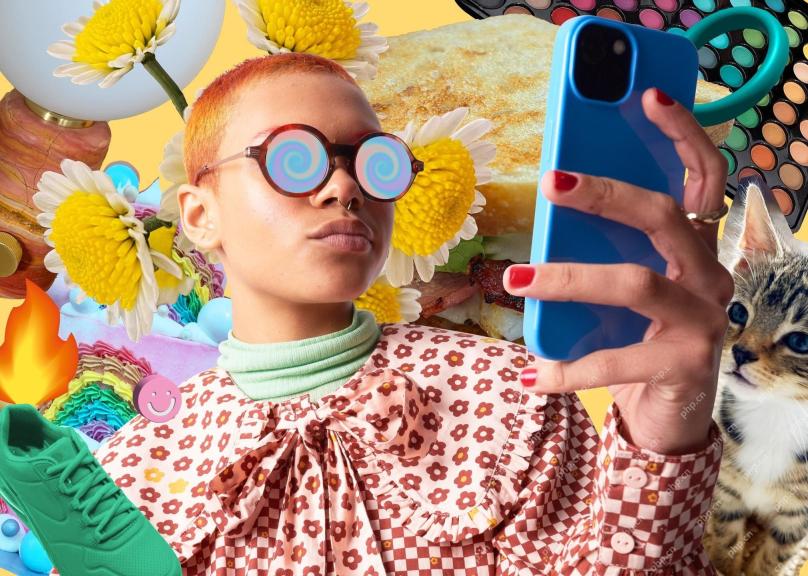
Meta upgrades AI assistant application, and the era of wearable AI is coming! The app, designed to compete with ChatGPT, offers standard AI features such as text, voice interaction, image generation and web search, but has now added geolocation capabilities for the first time. This means that Meta AI knows where you are and what you are viewing when answering your question. It uses your interests, location, profile and activity information to provide the latest situational information that was not possible before. The app also supports real-time translation, which completely changed the AI experience on Ray-Ban glasses and greatly improved its usefulness. The imposition of tariffs on foreign films is a naked exercise of power over the media and culture. If implemented, this will accelerate toward AI and virtual production
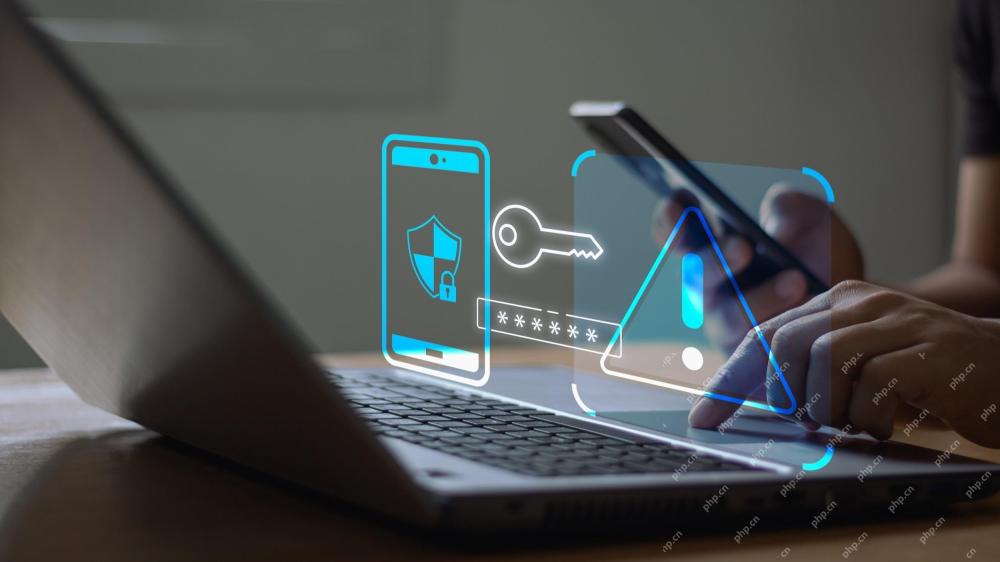
Artificial intelligence is revolutionizing the field of cybercrime, which forces us to learn new defensive skills. Cyber criminals are increasingly using powerful artificial intelligence technologies such as deep forgery and intelligent cyberattacks to fraud and destruction at an unprecedented scale. It is reported that 87% of global businesses have been targeted for AI cybercrime over the past year. So, how can we avoid becoming victims of this wave of smart crimes? Let’s explore how to identify risks and take protective measures at the individual and organizational level. How cybercriminals use artificial intelligence As technology advances, criminals are constantly looking for new ways to attack individuals, businesses and governments. The widespread use of artificial intelligence may be the latest aspect, but its potential harm is unprecedented. In particular, artificial intelligence
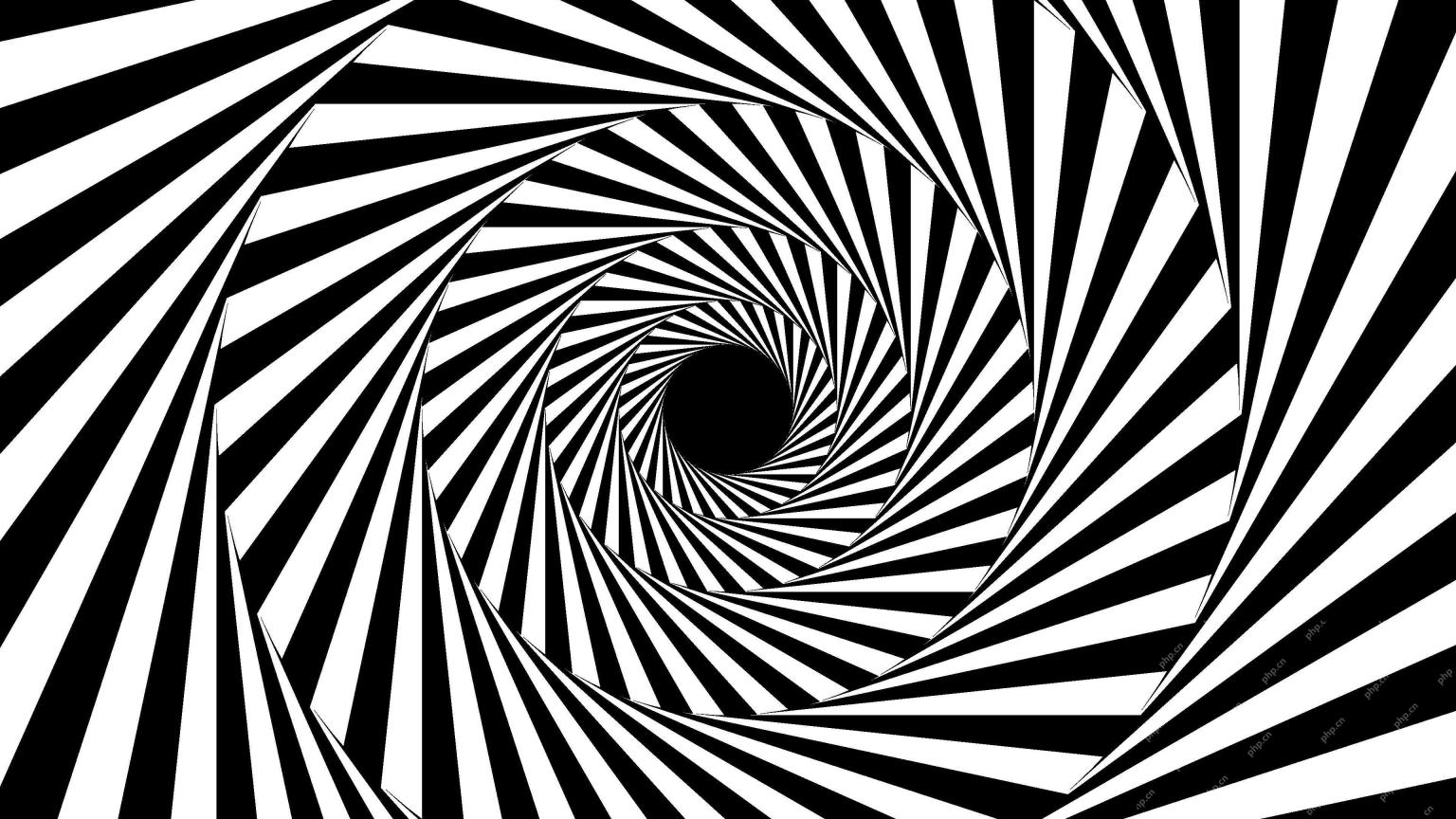
The intricate relationship between artificial intelligence (AI) and human intelligence (NI) is best understood as a feedback loop. Humans create AI, training it on data generated by human activity to enhance or replicate human capabilities. This AI
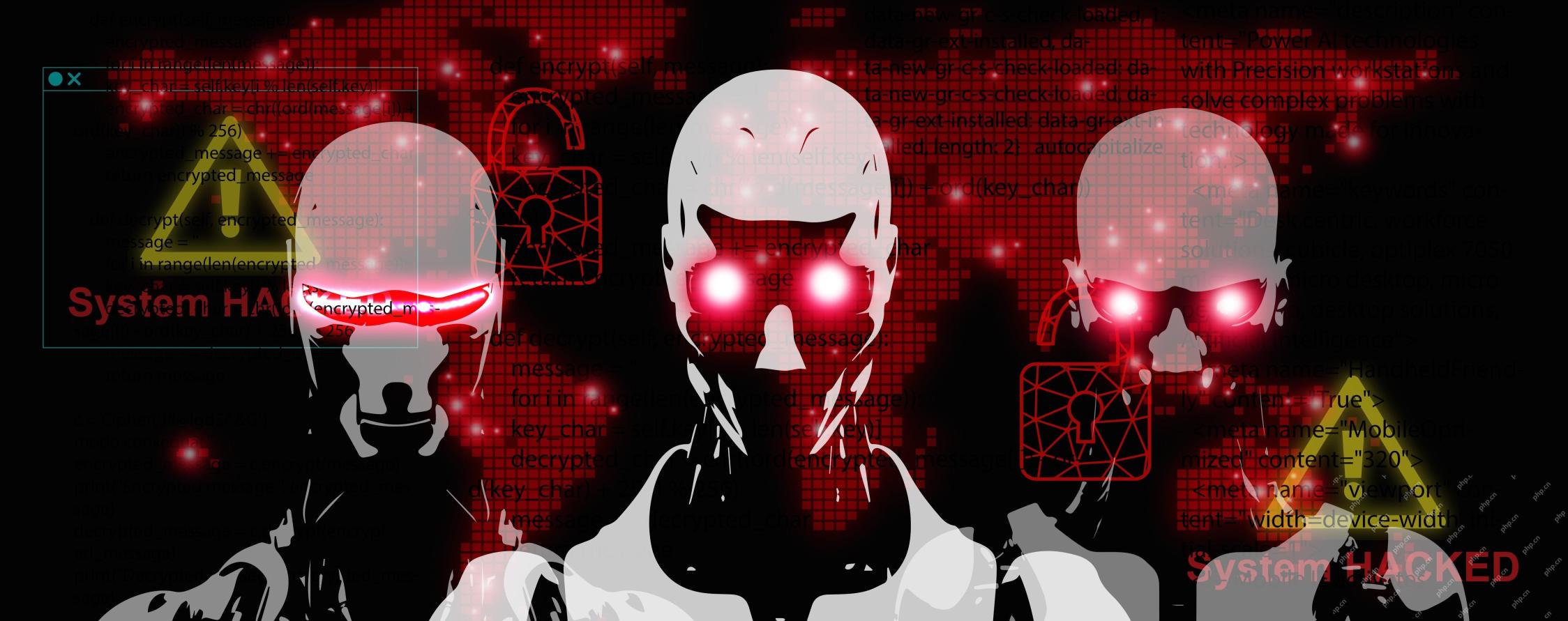
Anthropic's recent statement, highlighting the lack of understanding surrounding cutting-edge AI models, has sparked a heated debate among experts. Is this opacity a genuine technological crisis, or simply a temporary hurdle on the path to more soph
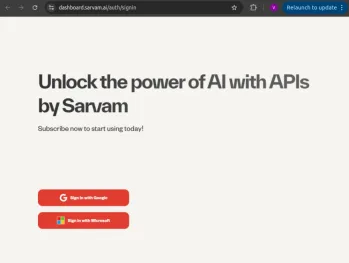
India is a diverse country with a rich tapestry of languages, making seamless communication across regions a persistent challenge. However, Sarvam’s Bulbul-V2 is helping to bridge this gap with its advanced text-to-speech (TTS) t


Hot AI Tools

Undresser.AI Undress
AI-powered app for creating realistic nude photos

AI Clothes Remover
Online AI tool for removing clothes from photos.

Undress AI Tool
Undress images for free

Clothoff.io
AI clothes remover

Video Face Swap
Swap faces in any video effortlessly with our completely free AI face swap tool!

Hot Article

Hot Tools

Safe Exam Browser
Safe Exam Browser is a secure browser environment for taking online exams securely. This software turns any computer into a secure workstation. It controls access to any utility and prevents students from using unauthorized resources.
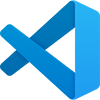
VSCode Windows 64-bit Download
A free and powerful IDE editor launched by Microsoft

SecLists
SecLists is the ultimate security tester's companion. It is a collection of various types of lists that are frequently used during security assessments, all in one place. SecLists helps make security testing more efficient and productive by conveniently providing all the lists a security tester might need. List types include usernames, passwords, URLs, fuzzing payloads, sensitive data patterns, web shells, and more. The tester can simply pull this repository onto a new test machine and he will have access to every type of list he needs.
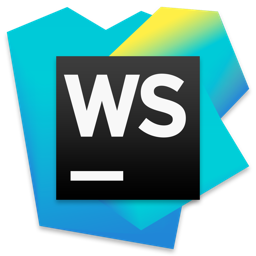
WebStorm Mac version
Useful JavaScript development tools

SAP NetWeaver Server Adapter for Eclipse
Integrate Eclipse with SAP NetWeaver application server.
