


The influence and role of hand features: a diffusion generative model perspective
The diffusion generative model is a generative model based on neural networks. Its main purpose is to learn a probability distribution to generate new data similar to the training data. In the field of computer vision, diffusion generative models are widely used in image generation and processing tasks. It has the following advantages: First, it is able to generate realistic images, making the generated images indistinguishable from real images. Secondly, it can be used for image repair, i.e. repairing corrupted images by generating missing image parts. In addition, diffusion generation models can also achieve super-resolution, which improves the clarity of images by generating high-resolution images. For hand features, the diffusion generation model can also generate realistic hand images and be used for tasks such as hand feature recognition. In summary, diffusion generation models have broad application prospects in the field of computer vision.
Hand features are an important field in human biometric technology. Human identity is mainly identified through features such as hand fingerprints, palm prints, palm veins and hand bones. The application of diffusion generation model in hand feature recognition is mainly reflected in two aspects: one is to generate realistic hand images, and generate real hand images through the model to improve the recognition accuracy; the other is to realize the recognition of hand features, Generative models are used for feature extraction and matching to achieve accurate recognition of hand features. These applications are expected to bring new breakthroughs to the development of hand biometric technology.
1. The diffusion generation model can be used to generate realistic hand images
Through the diffusion generation model, we can learn the hand features distribution and generate images similar to real hand images. This method can be used to generate more hand images, thereby expanding the hand image data set and improving the accuracy of hand feature recognition. Additionally, the generated hand images can be used to test the robustness and toughness of the hand feature recognition system.
2. Diffusion generation model can be used to realize the recognition of hand features
Hand feature recognition requires the establishment of a feature extraction model and classification device to extract features from hand images and identify individual identities. The diffusion generation model can be used to train the feature extraction model to improve the recognition accuracy of hand features. When training the feature extraction model, the diffusion generation model can extract important information in hand features by learning the distribution of hand images, thereby achieving more accurate feature extraction. In addition, the diffusion generation model can also be used to generate adversarial samples, thereby improving the robustness and resilience of the hand feature recognition system.
In short, the diffusion generation model has broad application prospects in hand feature recognition. It can be used to generate realistic hand images, expand hand image data sets, and improve the accuracy of hand feature recognition; at the same time, it can also be used to train feature extraction models, improve the accuracy of hand feature recognition, and generate Adversarial samples improve the robustness and toughness of hand feature recognition systems.
The above is the detailed content of The influence and role of hand features: a diffusion generative model perspective. For more information, please follow other related articles on the PHP Chinese website!
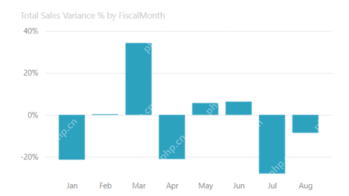
Harnessing the Power of Data Visualization with Microsoft Power BI Charts In today's data-driven world, effectively communicating complex information to non-technical audiences is crucial. Data visualization bridges this gap, transforming raw data i
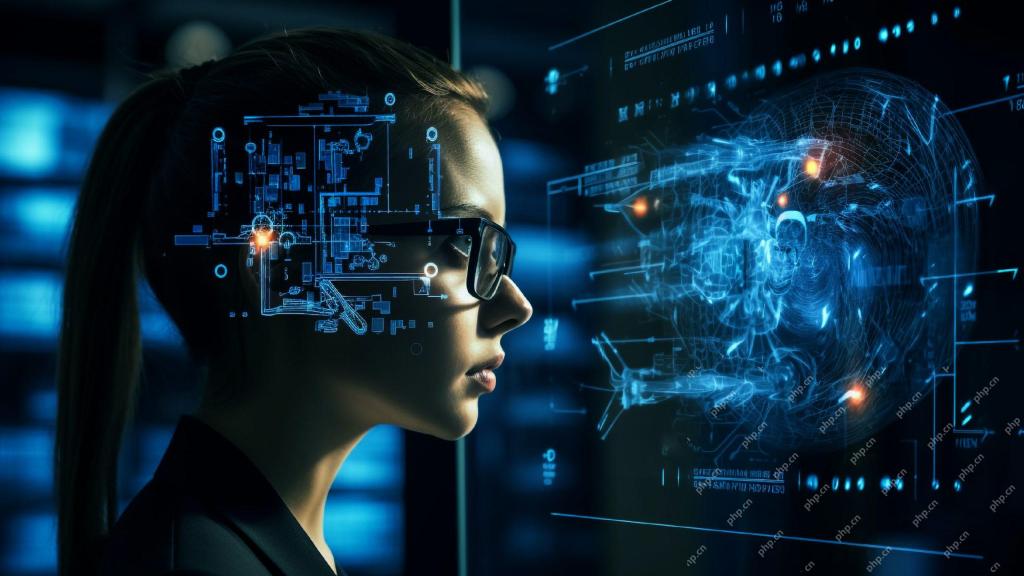
Expert Systems: A Deep Dive into AI's Decision-Making Power Imagine having access to expert advice on anything, from medical diagnoses to financial planning. That's the power of expert systems in artificial intelligence. These systems mimic the pro
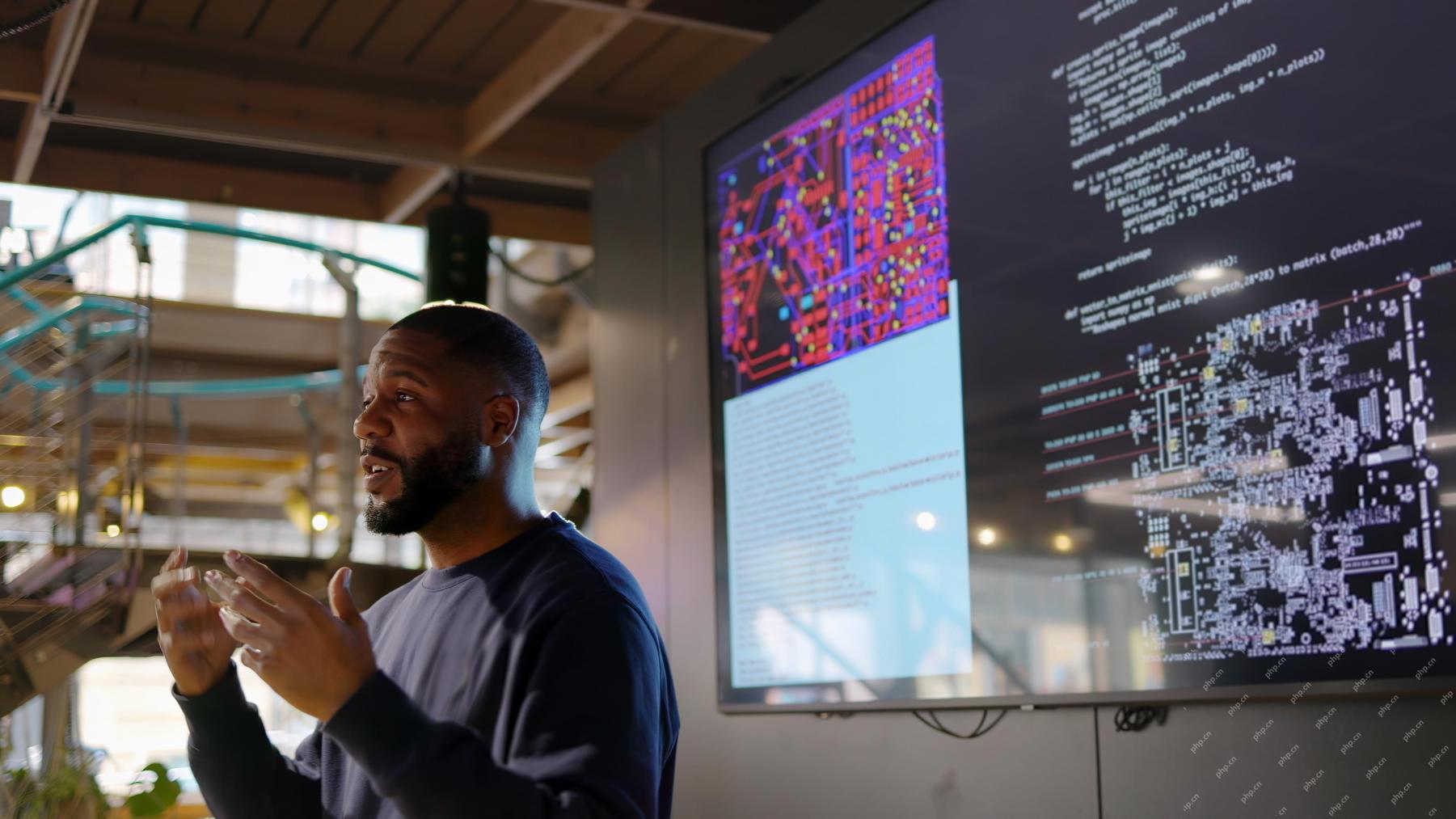
First of all, it’s apparent that this is happening quickly. Various companies are talking about the proportions of their code that are currently written by AI, and these are increasing at a rapid clip. There’s a lot of job displacement already around
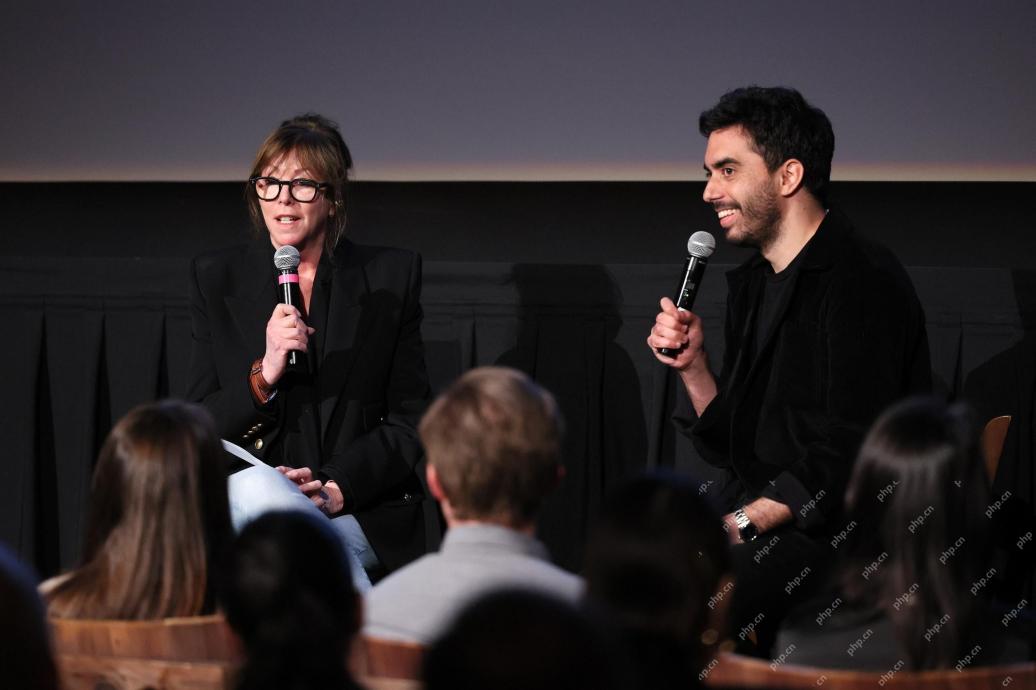
The film industry, alongside all creative sectors, from digital marketing to social media, stands at a technological crossroad. As artificial intelligence begins to reshape every aspect of visual storytelling and change the landscape of entertainment
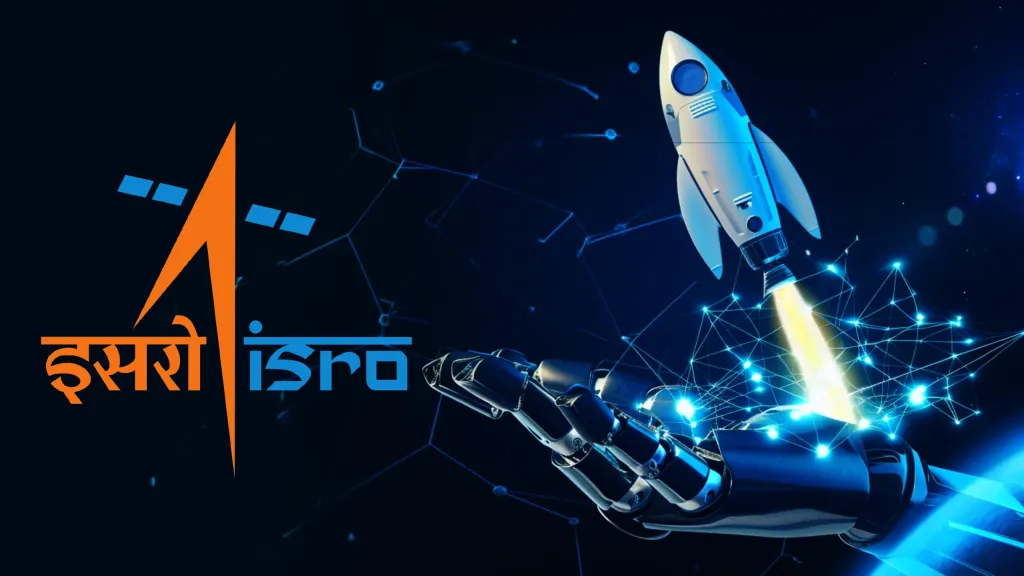
ISRO's Free AI/ML Online Course: A Gateway to Geospatial Technology Innovation The Indian Space Research Organisation (ISRO), through its Indian Institute of Remote Sensing (IIRS), is offering a fantastic opportunity for students and professionals to
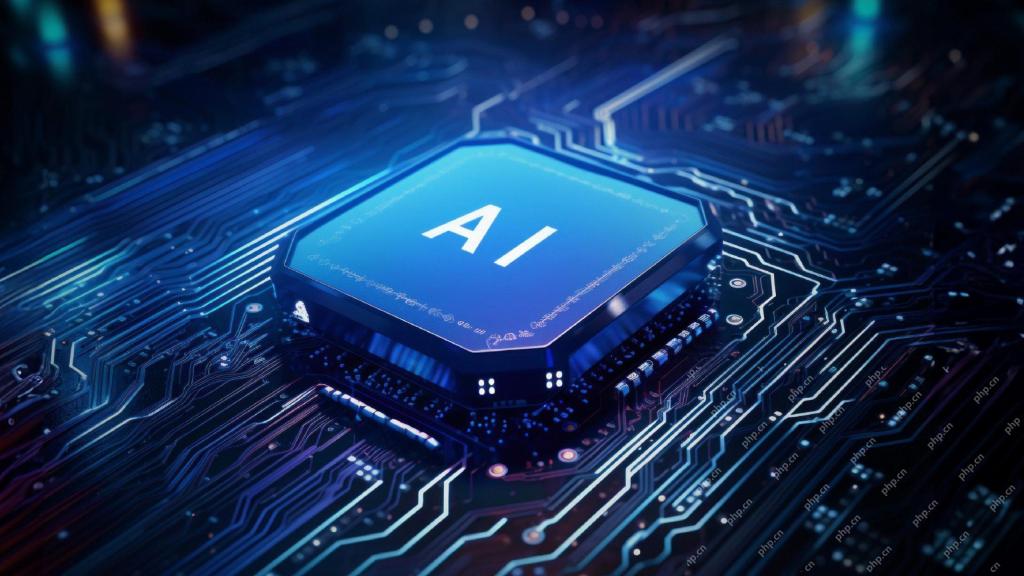
Local Search Algorithms: A Comprehensive Guide Planning a large-scale event requires efficient workload distribution. When traditional approaches fail, local search algorithms offer a powerful solution. This article explores hill climbing and simul
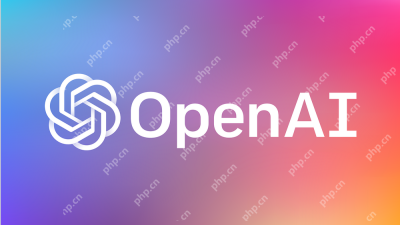
The release includes three distinct models, GPT-4.1, GPT-4.1 mini and GPT-4.1 nano, signaling a move toward task-specific optimizations within the large language model landscape. These models are not immediately replacing user-facing interfaces like
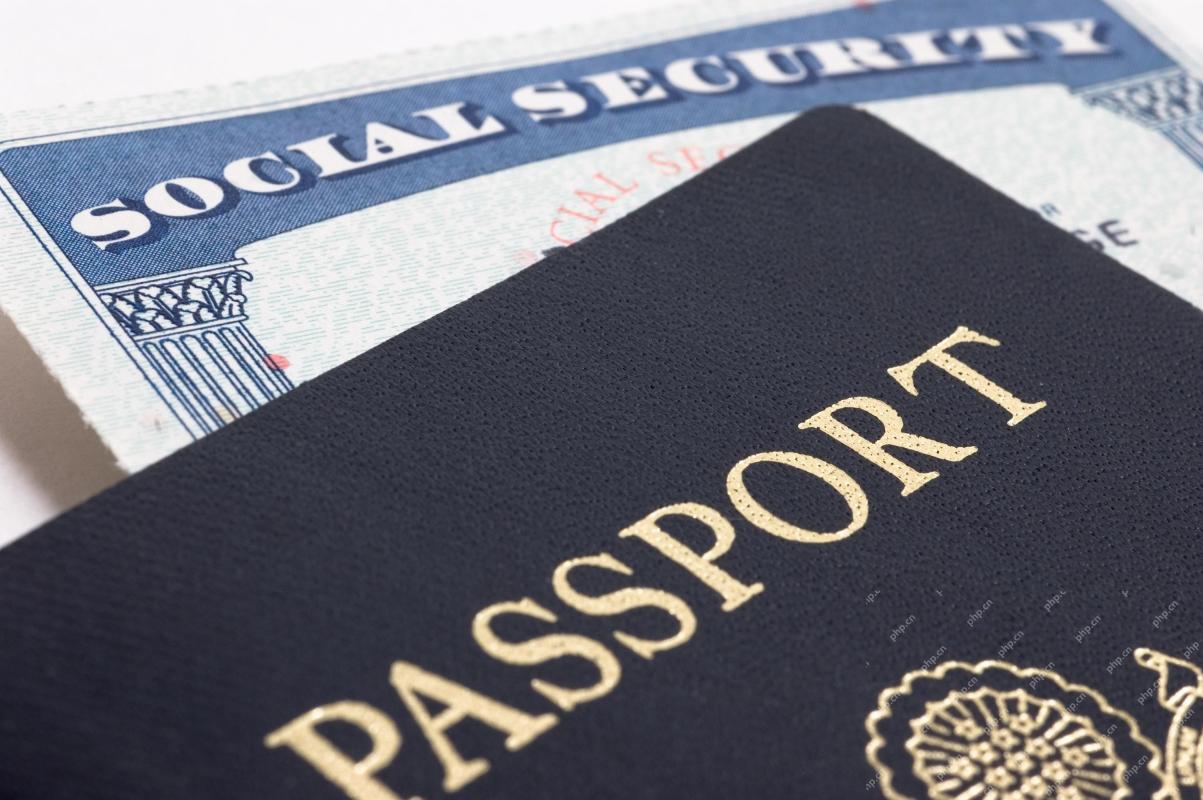
Chip giant Nvidia said on Monday it will start manufacturing AI supercomputers— machines that can process copious amounts of data and run complex algorithms— entirely within the U.S. for the first time. The announcement comes after President Trump si


Hot AI Tools

Undresser.AI Undress
AI-powered app for creating realistic nude photos

AI Clothes Remover
Online AI tool for removing clothes from photos.

Undress AI Tool
Undress images for free

Clothoff.io
AI clothes remover

AI Hentai Generator
Generate AI Hentai for free.

Hot Article

Hot Tools

mPDF
mPDF is a PHP library that can generate PDF files from UTF-8 encoded HTML. The original author, Ian Back, wrote mPDF to output PDF files "on the fly" from his website and handle different languages. It is slower than original scripts like HTML2FPDF and produces larger files when using Unicode fonts, but supports CSS styles etc. and has a lot of enhancements. Supports almost all languages, including RTL (Arabic and Hebrew) and CJK (Chinese, Japanese and Korean). Supports nested block-level elements (such as P, DIV),

MantisBT
Mantis is an easy-to-deploy web-based defect tracking tool designed to aid in product defect tracking. It requires PHP, MySQL and a web server. Check out our demo and hosting services.

Safe Exam Browser
Safe Exam Browser is a secure browser environment for taking online exams securely. This software turns any computer into a secure workstation. It controls access to any utility and prevents students from using unauthorized resources.
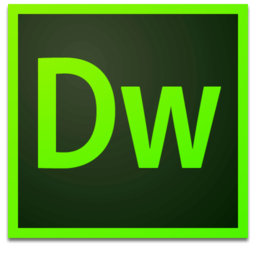
Dreamweaver Mac version
Visual web development tools
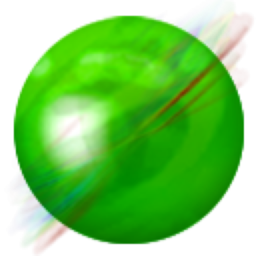
ZendStudio 13.5.1 Mac
Powerful PHP integrated development environment