


Paper Introduction
Human emotional behavior analysis has attracted much attention in human-computer interaction (HCI). This article is intended to introduce the paper we submitted to CVPR 2022 Affective Behavior Analysis in-the-wild (ABAW). To fully exploit emotional knowledge, we employ multi-modal features including spoken language, speech prosody, and facial expressions extracted from video clips in the Aff-Wild2 dataset. Based on these features, we propose a transformer-based multi-modal framework for action unit detection and expression recognition. This framework contributes to a more comprehensive understanding of human emotional behavior and provides new research directions in the field of human-computer interaction.
For the current frame image, we first encode it to extract static visual features. At the same time, we also use sliding windows to crop adjacent frames and extract three multi-modal features from image, audio and text sequences. Next, we introduce a transformer-based fusion module to fuse static visual features and dynamic multi-modal features. The cross-attention module in this fusion module helps focus the output integrated features on key parts that are helpful for downstream detection tasks. In order to further improve model performance, we adopted some data balancing techniques, data augmentation techniques and post-processing methods. In the official tests of ABAW3 Competition, our model ranked first on both EXPR and AU tracks. We demonstrate the effectiveness of our proposed method through extensive quantitative evaluation and ablation studies on the Aff-Wild2 dataset.
Paper link
https://arxiv.org/abs/2203.12367
The above is the detailed content of Facial Expression Analysis: Integrating Multimodal Information with Transformer. For more information, please follow other related articles on the PHP Chinese website!
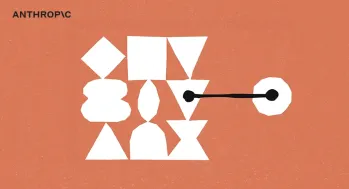
The Model Context Protocol (MCP): A Universal Connector for AI and Data We're all familiar with AI's role in daily coding. Replit, GitHub Copilot, Black Box AI, and Cursor IDE are just a few examples of how AI streamlines our workflows. But imagine
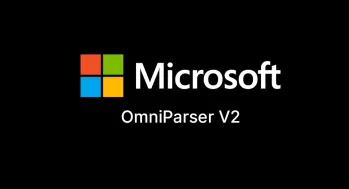
Microsoft's OmniParser V2 and OmniTool: Revolutionizing GUI Automation with AI Imagine AI that not only understands but also interacts with your Windows 11 interface like a seasoned professional. Microsoft's OmniParser V2 and OmniTool make this a re

Revolutionizing App Development: A Deep Dive into Replit Agent Tired of wrestling with complex development environments and obscure configuration files? Replit Agent aims to simplify the process of transforming ideas into functional apps. This AI-p
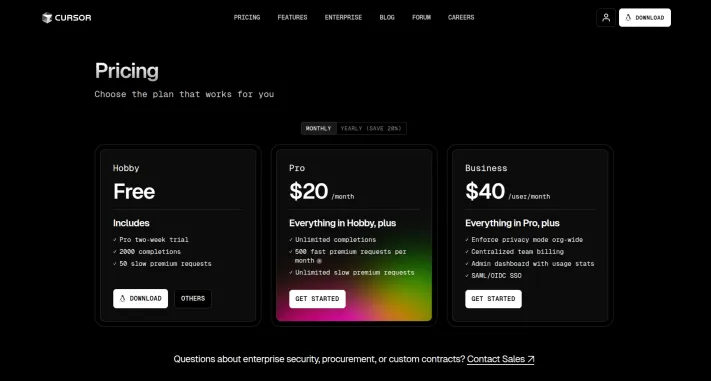
Vibe coding is reshaping the world of software development by letting us create applications using natural language instead of endless lines of code. Inspired by visionaries like Andrej Karpathy, this innovative approach lets dev
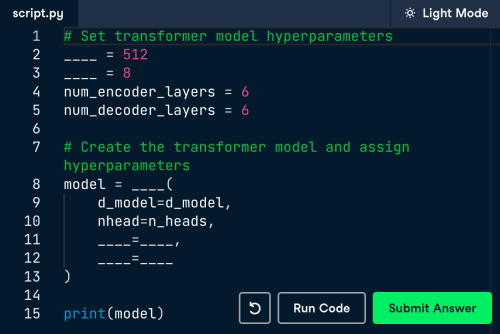
This blog post shares my experience testing Runway ML's new Act-One animation tool, covering both its web interface and Python API. While promising, my results were less impressive than expected. Want to explore Generative AI? Learn to use LLMs in P
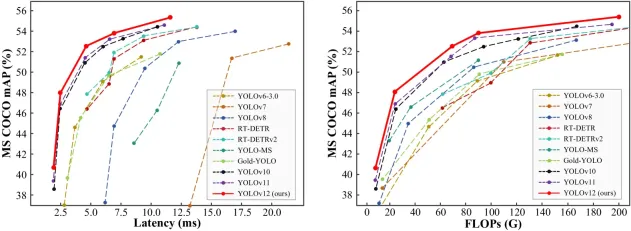
YOLO (You Only Look Once) has been a leading real-time object detection framework, with each iteration improving upon the previous versions. The latest version YOLO v12 introduces advancements that significantly enhance accuracy
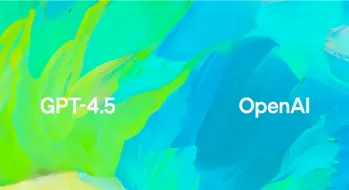
February 2025 has been yet another game-changing month for generative AI, bringing us some of the most anticipated model upgrades and groundbreaking new features. From xAI’s Grok 3 and Anthropic’s Claude 3.7 Sonnet, to OpenAI’s G
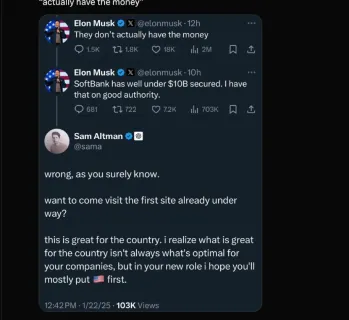
The $500 billion Stargate AI project, backed by tech giants like OpenAI, SoftBank, Oracle, and Nvidia, and supported by the U.S. government, aims to solidify American AI leadership. This ambitious undertaking promises a future shaped by AI advanceme


Hot AI Tools

Undresser.AI Undress
AI-powered app for creating realistic nude photos

AI Clothes Remover
Online AI tool for removing clothes from photos.

Undress AI Tool
Undress images for free

Clothoff.io
AI clothes remover

AI Hentai Generator
Generate AI Hentai for free.

Hot Article

Hot Tools

MantisBT
Mantis is an easy-to-deploy web-based defect tracking tool designed to aid in product defect tracking. It requires PHP, MySQL and a web server. Check out our demo and hosting services.

DVWA
Damn Vulnerable Web App (DVWA) is a PHP/MySQL web application that is very vulnerable. Its main goals are to be an aid for security professionals to test their skills and tools in a legal environment, to help web developers better understand the process of securing web applications, and to help teachers/students teach/learn in a classroom environment Web application security. The goal of DVWA is to practice some of the most common web vulnerabilities through a simple and straightforward interface, with varying degrees of difficulty. Please note that this software

SublimeText3 English version
Recommended: Win version, supports code prompts!

SAP NetWeaver Server Adapter for Eclipse
Integrate Eclipse with SAP NetWeaver application server.
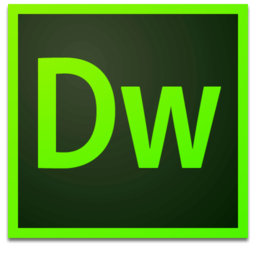
Dreamweaver Mac version
Visual web development tools
