


Building a reliable caching system: Sharing of design and practical experience of Java caching mechanism
Introduction:
In most applications, data caching is an improvement A common approach to system performance. Caching reduces access to the underlying data source, significantly improving application response time. In Java, we can implement the caching mechanism in a variety of ways. This article will introduce some common caching design patterns and practical experiences, and provide specific code examples.
1. Cache design pattern:
- Memory-based cache
Memory-based cache is the most common cache design pattern. It stores data in memory for quick retrieval when the application needs it, typically using a HashMap or ConcurrentHashMap. Here is a simple memory-based cache example:
import java.util.HashMap; import java.util.Map; import java.util.concurrent.TimeUnit; public class InMemoryCache<T> { private final Map<String, CacheEntry<T>> cache; private final long expirationTime; private static class CacheEntry<T> { private final T value; private final long createTime; CacheEntry(T value) { this.value = value; this.createTime = System.currentTimeMillis(); } boolean isExpired(long expirationTime) { return System.currentTimeMillis() - createTime > expirationTime; } } public InMemoryCache(long expirationTime) { this.cache = new HashMap<>(); this.expirationTime = expirationTime; } public void put(String key, T value) { cache.put(key, new CacheEntry<>(value)); } public T get(String key) { CacheEntry<T> entry = cache.get(key); if (entry != null && !entry.isExpired(expirationTime)) { return entry.value; } else { cache.remove(key); return null; } } public static void main(String[] args) { InMemoryCache<String> cache = new InMemoryCache<>(TimeUnit.MINUTES.toMillis(30)); cache.put("key1", "value1"); String value = cache.get("key1"); System.out.println(value); } }
- Disk-based cache
Disk-based cache stores data in disk files so that it can be cached when the application needs it Read. This cache design pattern works well for larger data sets, but is slower to read than a memory-based cache. The following is a simple disk-based cache example:
import java.io.*; import java.util.HashMap; import java.util.Map; public class DiskCache<T> { private final Map<String, File> cache; public DiskCache() { this.cache = new HashMap<>(); } public void put(String key, T value) { try { File file = new File("cache/" + key + ".bin"); ObjectOutputStream outputStream = new ObjectOutputStream(new FileOutputStream(file)); outputStream.writeObject(value); outputStream.close(); cache.put(key, file); } catch (IOException e) { e.printStackTrace(); } } public T get(String key) { File file = cache.get(key); if (file != null && file.exists()) { try { ObjectInputStream inputStream = new ObjectInputStream(new FileInputStream(file)); T value = (T) inputStream.readObject(); inputStream.close(); return value; } catch (IOException | ClassNotFoundException e) { e.printStackTrace(); } } cache.remove(key); return null; } public static void main(String[] args) { DiskCache<String> cache = new DiskCache<>(); cache.put("key1", "value1"); String value = cache.get("key1"); System.out.println(value); } }
2. Caching practical experience:
- Selection of caching strategy
When selecting a caching strategy, you need to Consider the size of the cache, the lifecycle of the data, and the application's access patterns to the data. For frequently accessed and small-capacity data, you can choose memory-based caching; for larger-capacity data sets, you can use disk-based caching. - Cache cleaning and expiration processing
In order to prevent cached data from expiring, cache cleaning and expiration processing need to be performed regularly. You can set an expiration time based on the size and capacity of the cache, or use an elimination strategy (such as least recently used) for data cleaning. - Distributed processing of cache
In a distributed system, the consistency of cached data needs to be considered when multiple nodes share cached data. You can use a distributed cache system (such as Redis) to implement distributed processing of cache and ensure data consistency.
3. Conclusion:
By properly designing and using the caching mechanism, the performance and response speed of the application can be significantly improved. When building a reliable cache system, choose an appropriate cache strategy, perform cache cleaning and expiration regularly, and consider the consistency of distributed caches. This article provides specific code examples of memory- and disk-based caching design patterns, hoping to help readers build reliable caching systems.
References:
- Javatpoint. (2019). Java Cache. https://www.javatpoint.com/java-cache
- Baeldung. (2021) ). Spring Caching with Redis. https://www.baeldung.com/spring-data-redis-cache
The above is the detailed content of Building a stable and reliable caching system: Sharing experience in the design and implementation of Java caching mechanism. For more information, please follow other related articles on the PHP Chinese website!
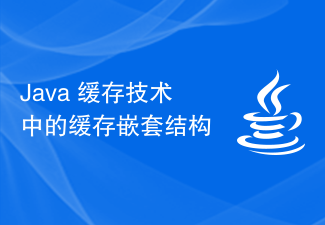
随着互联网的快速发展和用户数量的爆发式增长,如何提高网站或应用程序的性能成为了每个开发者都需要关注的问题。其中,缓存技术被广泛应用于提高系统的响应速度和减少数据库等后台操作的负担。Java缓存技术中的缓存嵌套结构可以更加有效地提升缓存的效率。缓存是存储数据的一种临时方式,通常存储在内存中,从而避免每次请求都需要访问数据库或其他数据源。简单地说,缓存是在内存
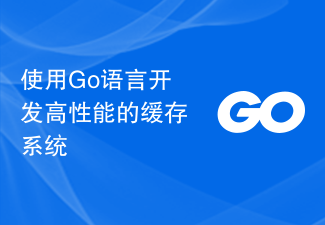
Go语言(又称Golang)一直以来以其高效的并发性能和优秀的性能而著称,因此非常适合用来开发高性能的缓存系统。本文将首先介绍为什么选择Go语言来开发缓存系统,然后将详细讨论如何利用Go语言的特性和优势来设计和实现高性能的缓存系统。为什么选择Go语言?Go语言具有以下特点,使其成为开发高性能缓存系统的理想选择:并发性能:Go语言内置的goroutine和ch
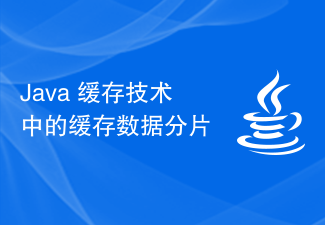
Java缓存技术中的缓存数据分片随着互联网的快速发展,大数据时代的到来,数据量的急剧增长给我们的数据存储和处理带来了巨大的挑战。为了解决这个问题,缓存技术应运而生。缓存是指将数据存储在更快的存储设备中,以便加速数据访问和读写操作。在Java缓存技术中,缓存数据分片是一种常见的技术手段。什么是缓存数据分片在高并发场景下,缓存往往成为了瓶颈。这时候,我们可以通
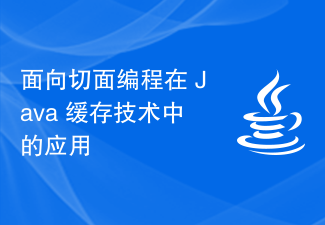
随着互联网应用的不断发展,对系统性能的要求变得越来越高,尤其是在数据缓存领域。Java缓存技术由于其高性能、高可用性和高扩展性等优势,已经成为了众多互联网应用的核心技术之一。然而,随着缓存规模的不断扩大和缓存逻辑的复杂化,难免会遇到一些问题,例如缓存数据的一致性和缓存命中率的提升等。面向切面编程(AOP)技术通过增强缓存逻辑的过程,能够有效地解决这些问
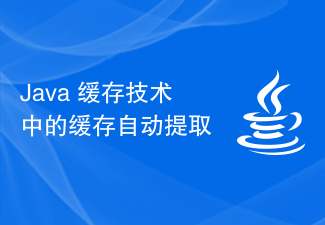
随着计算机技术的不断发展,数据的处理越来越成为了重要的课题。而在处理数据的过程中,缓存技术一直以来都是一个比较流行的解决方案。而其中的缓存自动提取技术更是为大量的应用提供了极大的便利。Java缓存技术中的缓存自动提取是一种根据缓存命中率自动决定缓存是否更新的技术。它通过对缓存命中率的监控和统计,对缓存库中的内容进行自动提取和更新。这项技术借助了Java
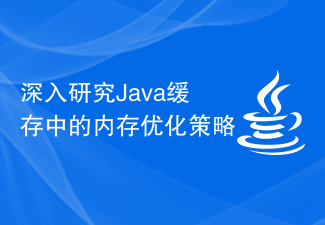
高效利用内存资源:探索Java缓存机制中的内存管理策略概述:在开发过程中,优化内存使用是提高应用程序性能的重要一环。Java作为一种高级编程语言,提供了灵活的内存管理机制,其中缓存是一种常用的技术手段。本文将介绍Java缓存机制的内存管理策略,并提供一些具体的代码示例。一、什么是缓存?缓存是一种将计算结果临时保存在内存中的技术。它通过预先将计算结果存储在内存
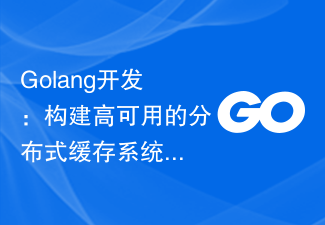
Golang开发:构建高可用的分布式缓存系统引言:随着互联网的不断发展,分布式缓存系统在大规模应用中扮演着重要的角色。在高并发环境下,传统的数据库存储方式往往无法满足应用的性能要求。因此,分布式缓存系统成为了实现高效、可扩展性的解决方案之一。本文将介绍如何使用Golang开发一个高可用的分布式缓存系统,并提供具体的代码示例,以供读者参考和学习。一、分布式缓存
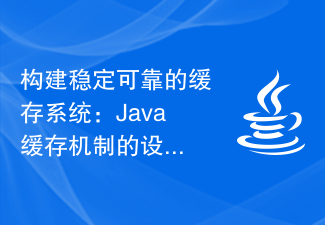
构建可靠的缓存系统:Java缓存机制的设计与实践经验分享引言:在大多数的应用程序中,数据缓存是提高系统性能的一种常见方法。通过缓存,可以减少对底层数据源的访问,从而显著缩短应用程序的响应时间。在Java中,我们可以采用多种方式实现缓存机制,本文将介绍一些常见的缓存设计模式和实践经验,并提供具体的代码示例。一、缓存设计模式:基于内存的缓存基于内存的缓存是最常见


Hot AI Tools

Undresser.AI Undress
AI-powered app for creating realistic nude photos

AI Clothes Remover
Online AI tool for removing clothes from photos.

Undress AI Tool
Undress images for free

Clothoff.io
AI clothes remover

AI Hentai Generator
Generate AI Hentai for free.

Hot Article

Hot Tools
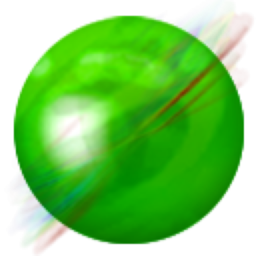
ZendStudio 13.5.1 Mac
Powerful PHP integrated development environment

MinGW - Minimalist GNU for Windows
This project is in the process of being migrated to osdn.net/projects/mingw, you can continue to follow us there. MinGW: A native Windows port of the GNU Compiler Collection (GCC), freely distributable import libraries and header files for building native Windows applications; includes extensions to the MSVC runtime to support C99 functionality. All MinGW software can run on 64-bit Windows platforms.

Notepad++7.3.1
Easy-to-use and free code editor
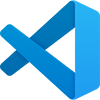
VSCode Windows 64-bit Download
A free and powerful IDE editor launched by Microsoft

SAP NetWeaver Server Adapter for Eclipse
Integrate Eclipse with SAP NetWeaver application server.