Local Binary Pattern (LBP) is a commonly used texture feature descriptor used to reveal the texture information of images. The LBP algorithm was first proposed by Ojala et al. in 1996, and has been continuously improved and developed in subsequent research.
The basic idea of the LBP algorithm is to compare the gray value of each pixel with its surrounding pixels and convert the comparison result into a binary number. In this way, each pixel can be represented as a local binary pattern. By counting the occurrence times of different local binary patterns in the image, the feature vector describing the texture information of the image can be obtained.
The specific implementation steps of the LBP algorithm are as follows:
Select a pixel in the image (called the central pixel) and several pixels around it (called neighbor pixels).
For each neighbor pixel, compare its gray value difference with the center pixel. If the gray value of the neighbor pixel is greater than the central pixel, set it to 1, otherwise set it to 0.
The binary values of neighboring pixels are concatenated to form the local binary pattern of the central pixel.
Traverse the entire image, perform the above operations on each pixel, and finally obtain an image composed of local binary patterns.
For the entire image, count the number of occurrences of different local binary patterns and form a feature vector.
The advantages of the LBP algorithm are that it is simple to calculate, does not require training, and can effectively describe image texture information. Therefore, it is widely used in image recognition, face recognition, pedestrian detection and other fields.
Local binary pattern image feature extraction steps
The local binary pattern algorithm can well describe the texture information of the image, so it is widely used for image feature extraction. The following are the general local binary pattern image feature extraction steps:
1. Image preprocessing: Convert the image into a grayscale image, and perform preprocessing operations such as histogram equalization to improve the contrast and Feature robustness.
2. Select the sampling point and sampling radius: In order to calculate the local binary pattern, you need to select the sampling point and sampling radius. The sampling point is the neighbor pixels around the central pixel, and the sampling radius is the distance from the central pixel to the sampling point.
3. Calculate the local binary pattern: For each pixel in the image, calculate its local binary pattern. Specifically, for each pixel, its gray value is compared with the gray value of the neighbor pixels around it. If the gray value of the neighbor pixel is greater than the gray value of the central pixel, then the weight of the neighbor pixel is is 1, otherwise it is 0. By combining the weights of all neighboring pixels into a binary number, the local binary pattern of the pixel is obtained.
4. Statistics of local binary patterns: For the entire image, count the number of occurrences of different local binary patterns and form a feature vector.
5. Feature vector normalization: Normalize the feature vectors to eliminate scale differences between different images.
6. Feature selection: Perform feature selection on the feature vector and select features that contribute more to the classification effect.
7. Feature classification: Use a classifier to classify feature vectors.
The local binary pattern image feature extraction algorithm is simple and effective, and can well describe the texture information of the image. Therefore, it has been widely used in image classification, face recognition, pedestrian detection and other fields.
How to use local binary pattern for face recognition
The local binary pattern algorithm has been widely used in the field of face recognition. The following are the general face recognition steps based on the LBP algorithm:
1. Data set preparation: Prepare a training set and a test set containing face images. Each image needs to be labeled with the face position and face label. .
2. Image preprocessing: Convert the image into a grayscale image, and perform preprocessing operations such as histogram equalization to improve the contrast of the image and the robustness of the features.
3. Face detection: Use a face detection algorithm (such as the Viola-Jones algorithm) to detect the face in the image, and crop and standardize the face part.
4. Feature extraction: For the cropped and standardized face images, use the LBP algorithm to extract features. The local binary pattern of each pixel is composed into a feature vector, and the feature vector is normalized.
5. Feature dimensionality reduction: Perform dimensionality reduction operations such as PCA or LDA on the feature vector to reduce the dimension of the feature vector and improve the separability of the feature.
6. Classifier training: Use the training set to train the classifier (such as SVM, KNN, etc.).
7. Test set classification: Use the trained classifier to classify the test set and obtain the prediction results.
8. Evaluate the model: Use accuracy, recall, F1 value and other indicators to evaluate the model, and tune the model parameters.
The above steps are the general face recognition process based on the LBP algorithm. In practical applications, it can be adjusted and optimized according to specific problems.
The above is the detailed content of In-depth analysis of local binary pattern LBP. For more information, please follow other related articles on the PHP Chinese website!
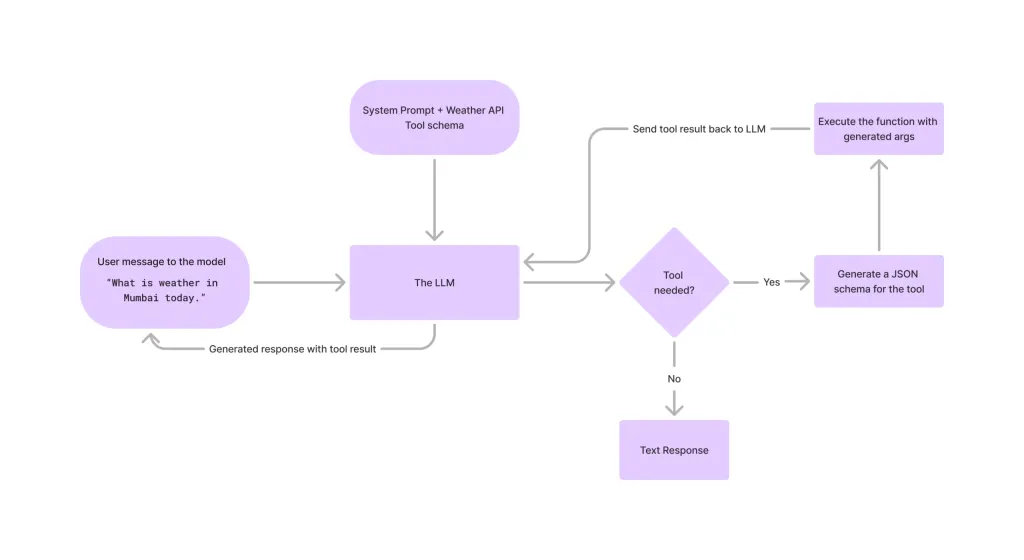
Large language models (LLMs) have surged in popularity, with the tool-calling feature dramatically expanding their capabilities beyond simple text generation. Now, LLMs can handle complex automation tasks such as dynamic UI creation and autonomous a
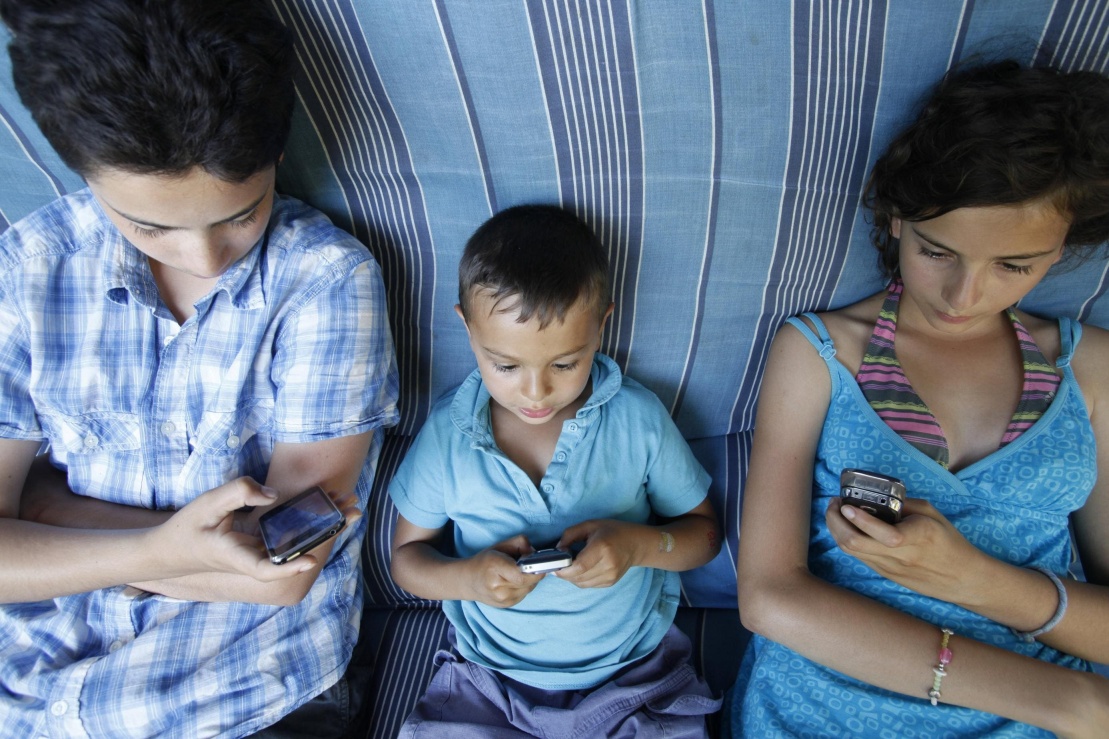
Can a video game ease anxiety, build focus, or support a child with ADHD? As healthcare challenges surge globally — especially among youth — innovators are turning to an unlikely tool: video games. Now one of the world’s largest entertainment indus
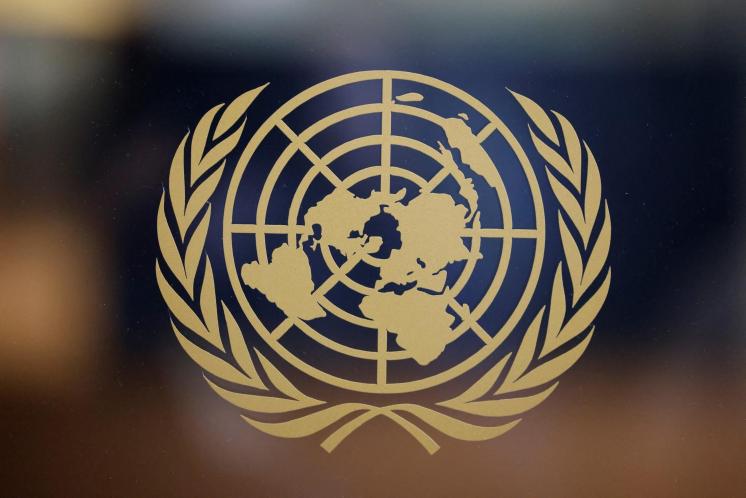
“History has shown that while technological progress drives economic growth, it does not on its own ensure equitable income distribution or promote inclusive human development,” writes Rebeca Grynspan, Secretary-General of UNCTAD, in the preamble.
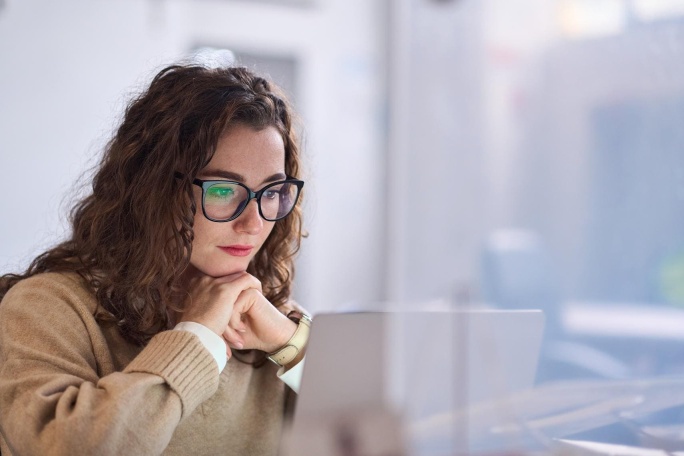
Easy-peasy, use generative AI as your negotiation tutor and sparring partner. Let’s talk about it. This analysis of an innovative AI breakthrough is part of my ongoing Forbes column coverage on the latest in AI, including identifying and explaining
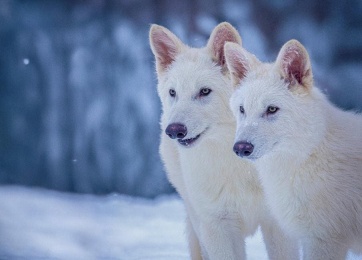
The TED2025 Conference, held in Vancouver, wrapped its 36th edition yesterday, April 11. It featured 80 speakers from more than 60 countries, including Sam Altman, Eric Schmidt, and Palmer Luckey. TED’s theme, “humanity reimagined,” was tailor made
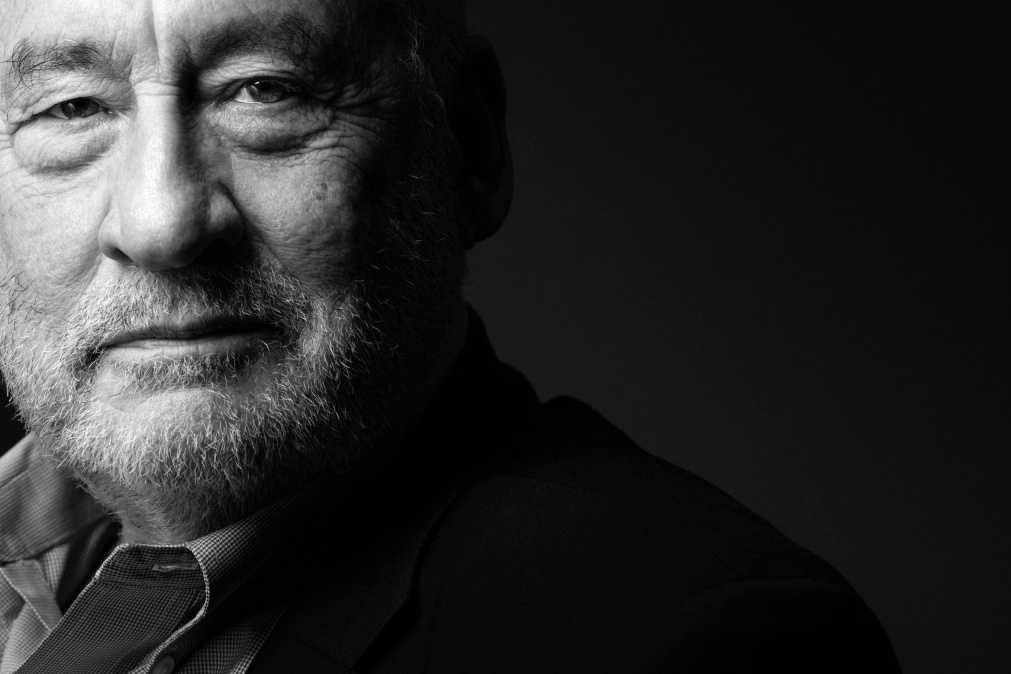
Joseph Stiglitz is renowned economist and recipient of the Nobel Prize in Economics in 2001. Stiglitz posits that AI can worsen existing inequalities and consolidated power in the hands of a few dominant corporations, ultimately undermining economic
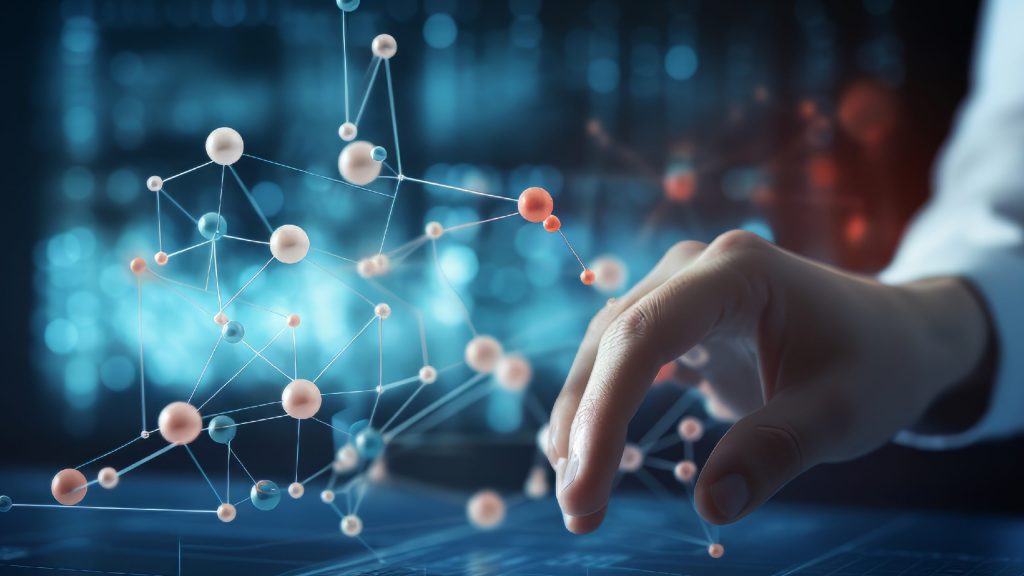
Graph Databases: Revolutionizing Data Management Through Relationships As data expands and its characteristics evolve across various fields, graph databases are emerging as transformative solutions for managing interconnected data. Unlike traditional
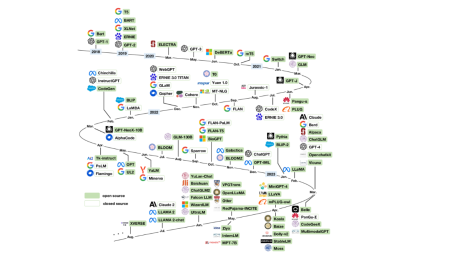
Large Language Model (LLM) Routing: Optimizing Performance Through Intelligent Task Distribution The rapidly evolving landscape of LLMs presents a diverse range of models, each with unique strengths and weaknesses. Some excel at creative content gen


Hot AI Tools

Undresser.AI Undress
AI-powered app for creating realistic nude photos

AI Clothes Remover
Online AI tool for removing clothes from photos.

Undress AI Tool
Undress images for free

Clothoff.io
AI clothes remover

AI Hentai Generator
Generate AI Hentai for free.

Hot Article

Hot Tools
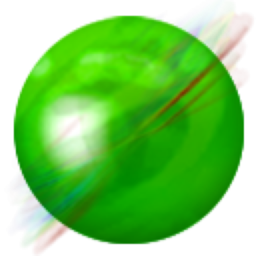
ZendStudio 13.5.1 Mac
Powerful PHP integrated development environment

SublimeText3 Linux new version
SublimeText3 Linux latest version
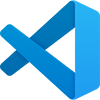
VSCode Windows 64-bit Download
A free and powerful IDE editor launched by Microsoft
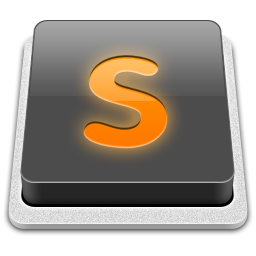
SublimeText3 Mac version
God-level code editing software (SublimeText3)
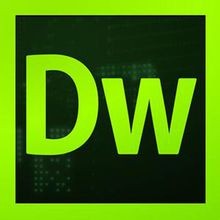
Dreamweaver CS6
Visual web development tools