Deep residual networks are composed of multiple shallow networks
The Residual Network (ResNet) is a deep convolutional neural network (DCNN) that is unique in its ability to train and optimize very deep network structures. Its proposal has greatly promoted the development of the field of deep learning, and has been widely used in fields such as computer vision and natural language processing. ResNet solves the problem of gradient disappearance and gradient explosion by introducing residual connection, which allows the network to skip some layers during the learning process, thereby better conveying gradient information. This design makes the network easier to train, reduces the complexity and parameter amount of the network, and also improves the performance of the network. By using residual connections, ResNet is able to reach very deep network depths, even exceeding 1000 layers. This deep network structure has achieved remarkable results in tasks such as image classification, target detection, and semantic segmentation, becoming an important milestone in the field of deep learning.
The core idea of ResNet is to introduce a residual connection (Residual Connection) to directly add the input of the previous layer to the output of the next layer to build a "skip connection" path of. The advantage of this is that it makes it easier for the network to learn certain features or patterns, avoids the problem of difficulty in training deep networks, and reduces the phenomenon of gradient disappearance, thereby improving the network's convergence speed and generalization ability. This skip connection design allows information to be passed directly through the network, making it easier for the network to learn the residual, that is, the difference between the input and the output. By introducing such skip connections, ResNet can increase the depth of the network by adding additional layers without causing performance degradation. Therefore, ResNet has become one of the very important architectures in deep learning.
Compared with traditional convolutional neural networks, ResNet uses residual blocks to build each layer, not just simple feature maps. Each residual block consists of multiple convolutional layers and nonlinear activation functions, and there is also a residual connection. This design enables ResNet to implement very deep network structures, such as ResNet-50, ResNet-101 and ResNet-152, etc., with the number of layers reaching 50, 101 and 152 respectively. Through the introduction of residual blocks, ResNet solves the gradient disappearance and gradient explosion problems in deep networks, effectively improving the performance of the network and the convergence speed of training. Therefore, ResNet has become one of the most important and popular network structures in deep learning.
Another important feature of ResNet is its ability to behave like an ensemble of relatively shallow networks. Specifically, each ResNet residual block can be regarded as a new feature extraction method that can effectively capture features of different scales and abstractions and integrate them organically. In addition, the skip connections between these residual blocks can be regarded as a special set operation used to fuse the previous features with the later features, thereby enabling the network to better learn complex features and patterns. . This structure enables ResNet to perform deeper feature learning while avoiding the vanishing gradient problem and improving the performance and generalization ability of the model.
This combination method, which is similar to a relatively shallow network, gives ResNet strong interpretability and generalization performance. Since each residual block can be regarded as an independent feature extractor, by visualizing the output of each residual block, we can better understand the learning process and feature representation capabilities of the network. The introduction of skip connections can reduce the loss of feature information, thereby improving the generalization ability of the network.
In short, the introduction of ResNet has greatly promoted the development of the field of deep learning, and its success is largely attributed to the design of its unique residual connections and residual blocks. , allowing the network to achieve very deep structures and behave like a collection of relatively shallow networks. In this way, ResNet can better learn complex features and patterns, and can also improve the interpretability and generalization capabilities of the network, bringing great value to applications in fields such as computer vision and natural language processing. .
The above is the detailed content of Deep residual networks are composed of multiple shallow networks. For more information, please follow other related articles on the PHP Chinese website!
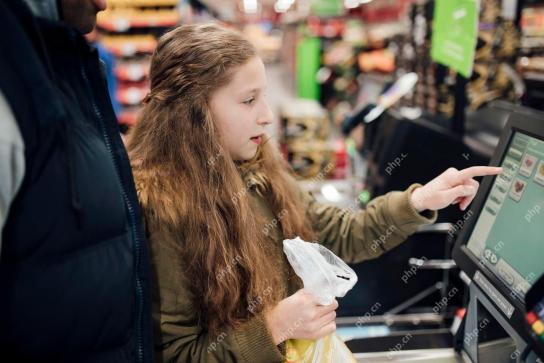
Revolutionizing the Checkout Experience Sam's Club's innovative "Just Go" system builds on its existing AI-powered "Scan & Go" technology, allowing members to scan purchases via the Sam's Club app during their shopping trip.
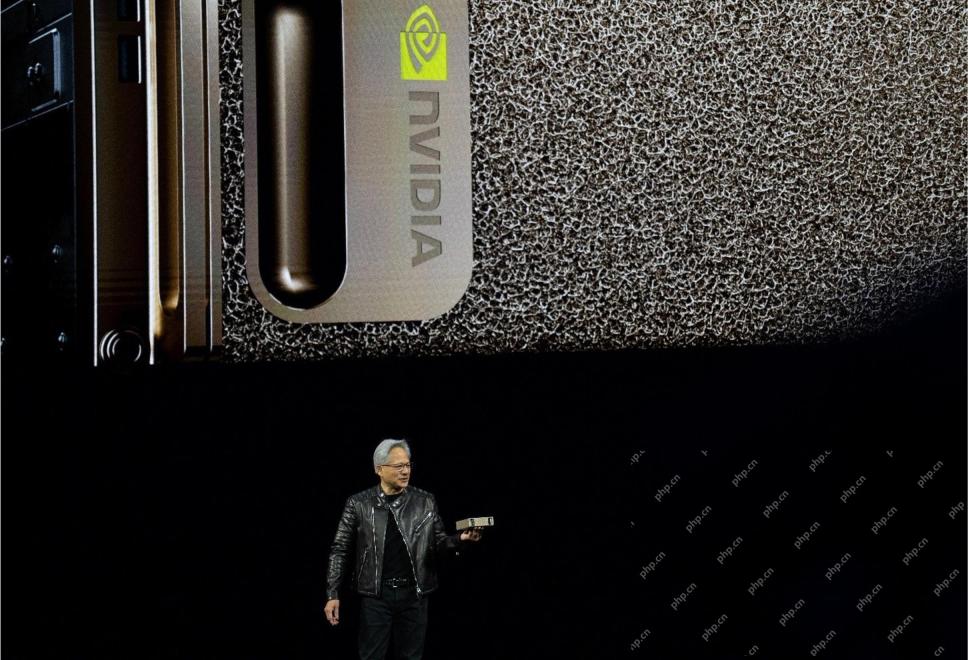
Nvidia's Enhanced Predictability and New Product Lineup at GTC 2025 Nvidia, a key player in AI infrastructure, is focusing on increased predictability for its clients. This involves consistent product delivery, meeting performance expectations, and
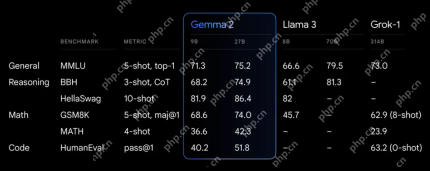
Google's Gemma 2: A Powerful, Efficient Language Model Google's Gemma family of language models, celebrated for efficiency and performance, has expanded with the arrival of Gemma 2. This latest release comprises two models: a 27-billion parameter ver
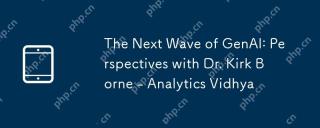
This Leading with Data episode features Dr. Kirk Borne, a leading data scientist, astrophysicist, and TEDx speaker. A renowned expert in big data, AI, and machine learning, Dr. Borne offers invaluable insights into the current state and future traje
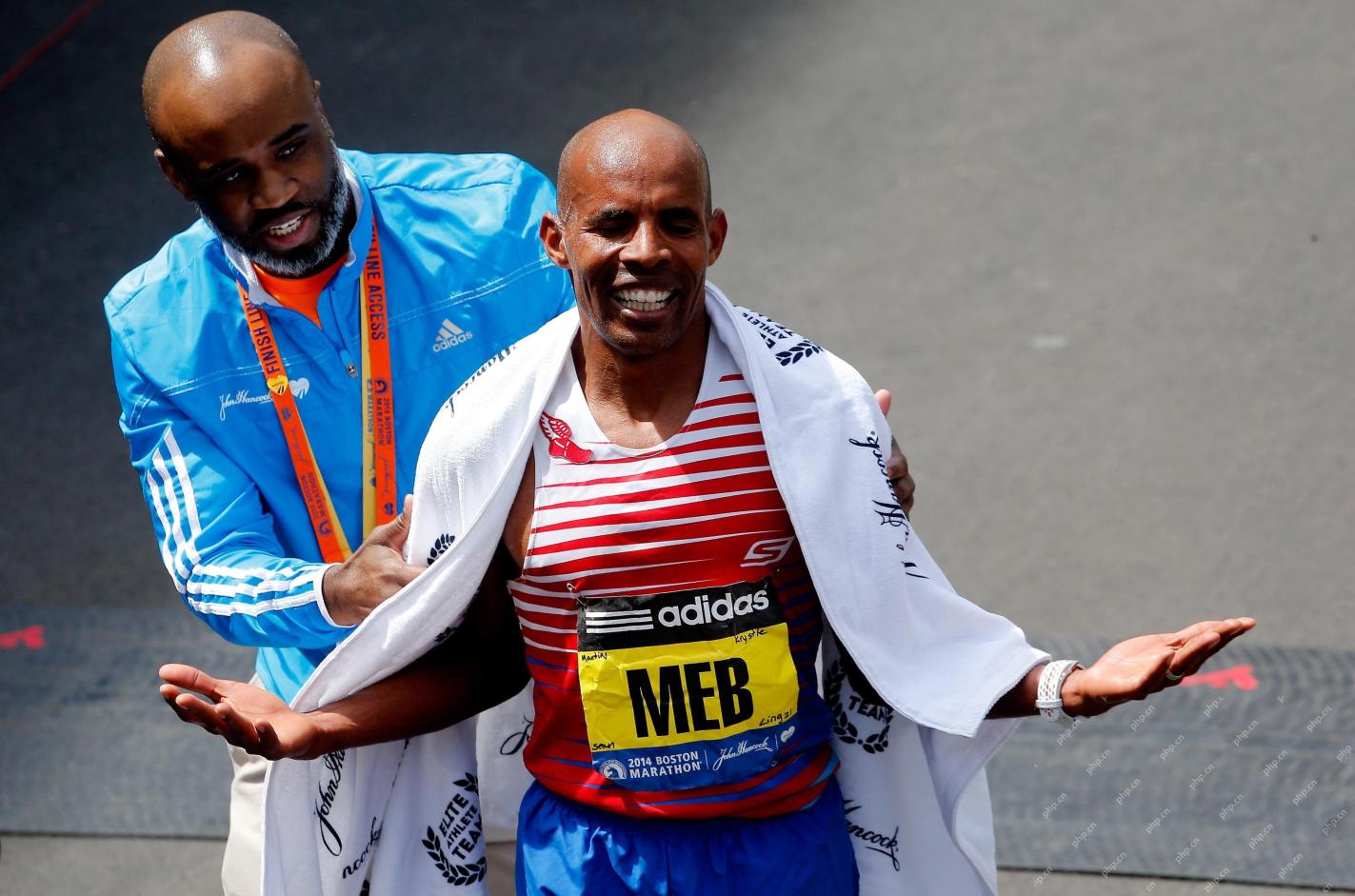
There were some very insightful perspectives in this speech—background information about engineering that showed us why artificial intelligence is so good at supporting people’s physical exercise. I will outline a core idea from each contributor’s perspective to demonstrate three design aspects that are an important part of our exploration of the application of artificial intelligence in sports. Edge devices and raw personal data This idea about artificial intelligence actually contains two components—one related to where we place large language models and the other is related to the differences between our human language and the language that our vital signs “express” when measured in real time. Alexander Amini knows a lot about running and tennis, but he still
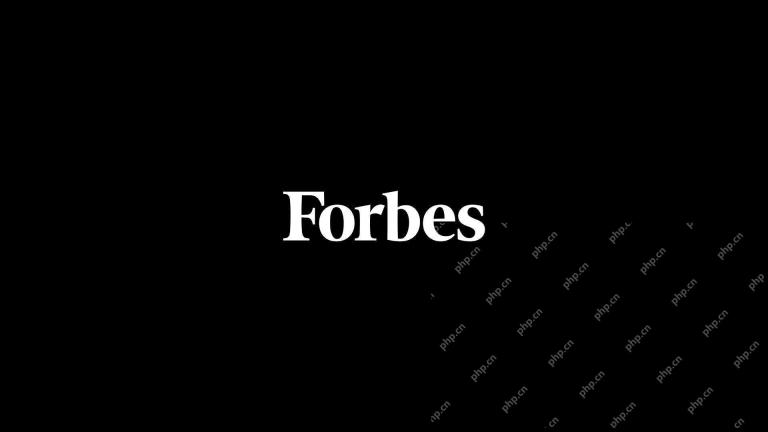
Caterpillar's Chief Information Officer and Senior Vice President of IT, Jamie Engstrom, leads a global team of over 2,200 IT professionals across 28 countries. With 26 years at Caterpillar, including four and a half years in her current role, Engst
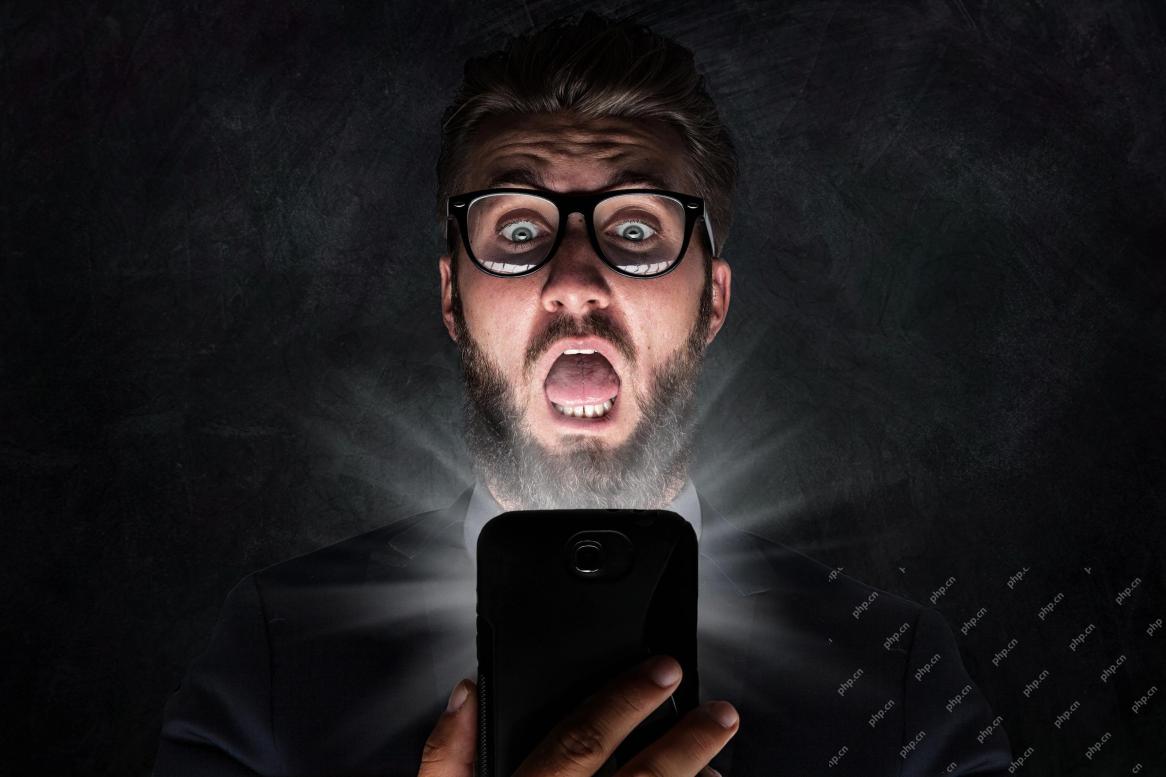
Google Photos' New Ultra HDR Tool: A Quick Guide Enhance your photos with Google Photos' new Ultra HDR tool, transforming standard images into vibrant, high-dynamic-range masterpieces. Ideal for social media, this tool boosts the impact of any photo,
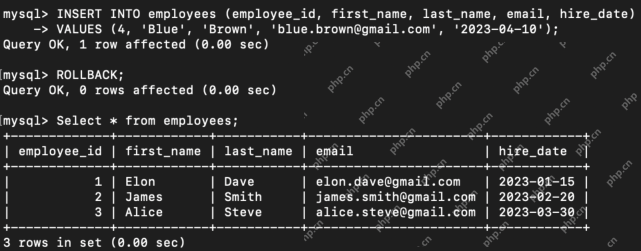
Introduction Transaction Control Language (TCL) commands are essential in SQL for managing changes made by Data Manipulation Language (DML) statements. These commands allow database administrators and users to control transaction processes, thereby


Hot AI Tools

Undresser.AI Undress
AI-powered app for creating realistic nude photos

AI Clothes Remover
Online AI tool for removing clothes from photos.

Undress AI Tool
Undress images for free

Clothoff.io
AI clothes remover

Video Face Swap
Swap faces in any video effortlessly with our completely free AI face swap tool!

Hot Article

Hot Tools

SublimeText3 English version
Recommended: Win version, supports code prompts!

mPDF
mPDF is a PHP library that can generate PDF files from UTF-8 encoded HTML. The original author, Ian Back, wrote mPDF to output PDF files "on the fly" from his website and handle different languages. It is slower than original scripts like HTML2FPDF and produces larger files when using Unicode fonts, but supports CSS styles etc. and has a lot of enhancements. Supports almost all languages, including RTL (Arabic and Hebrew) and CJK (Chinese, Japanese and Korean). Supports nested block-level elements (such as P, DIV),
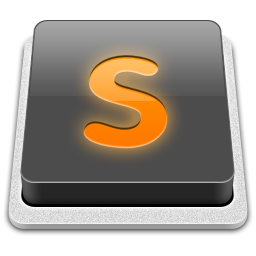
SublimeText3 Mac version
God-level code editing software (SublimeText3)

MinGW - Minimalist GNU for Windows
This project is in the process of being migrated to osdn.net/projects/mingw, you can continue to follow us there. MinGW: A native Windows port of the GNU Compiler Collection (GCC), freely distributable import libraries and header files for building native Windows applications; includes extensions to the MSVC runtime to support C99 functionality. All MinGW software can run on 64-bit Windows platforms.

Atom editor mac version download
The most popular open source editor