The optimizer is an algorithm in neural networks that adjusts weights and biases to minimize the loss function and improve model accuracy. During training, the optimizer is mainly used to update parameters and guide the model to optimize in a better direction. Through methods such as gradient descent, the optimizer can automatically adjust weights and biases so that the model gradually approaches the optimal solution. This way, the network can learn better and improve prediction performance.
The optimizer updates model parameters based on the gradient of the loss function to minimize the loss function and improve model accuracy.
One of the functions of the optimizer is to improve the learning speed. It works by adjusting the learning rate based on the gradient of the loss function to better train the neural network. If the learning rate is too large, it will be difficult for the model to converge during the training process; if the learning rate is too small, the model training will be slow. Therefore, the optimizer can help us find a suitable learning rate to improve the training effect of the model.
Avoiding overfitting is an important task of the optimizer, which can be achieved through regularization methods (such as L1, L2 regularization). Overfitting refers to the phenomenon that a model performs well on training data but performs poorly on test data. By using regularization methods, the complexity of the model can be reduced, preventing the model from overfitting the training data, thereby improving the generalization ability of the model.
Common optimizer algorithms include gradient descent, stochastic gradient descent and Adam optimizer. They each have their own advantages and disadvantages in adjusting model parameters, and selection and adjustment need to be based on actual conditions.
The working principle of the optimizer in the neural network
The optimizer determines the direction of parameter adjustment by calculating the gradient of the loss function based on the current weights and biases to minimize the loss. The goal of the function. Based on the calculated gradients, the optimizer updates the weights and biases in the neural network. This update process can use different methods such as gradient descent, stochastic gradient descent, and Adam optimizer. These optimization algorithms update parameters based on the current gradient and learning rate, so that the loss function gradually decreases, thereby improving the performance of the neural network.
The optimizer automatically adjusts the learning rate based on the current learning progress to better train the neural network. If the learning rate is too large, the model will be difficult to converge; if the learning rate is too small, the model will train slowly.
Finally, the optimizer uses regularization methods to avoid overfitting, thereby improving the generalization ability of the model.
It should be noted that different optimizer algorithms have their own advantages and disadvantages in adjusting model parameters. When selecting and adjusting the optimizer, judgment should be made based on the actual situation. For example, the Adam optimizer has faster convergence speed and better generalization ability than the traditional gradient descent method. Therefore, when training a deep learning model, you can consider using the Adam optimizer to improve training efficiency and model performance.
The above is the detailed content of The Importance of Optimizers in Neural Networks. For more information, please follow other related articles on the PHP Chinese website!
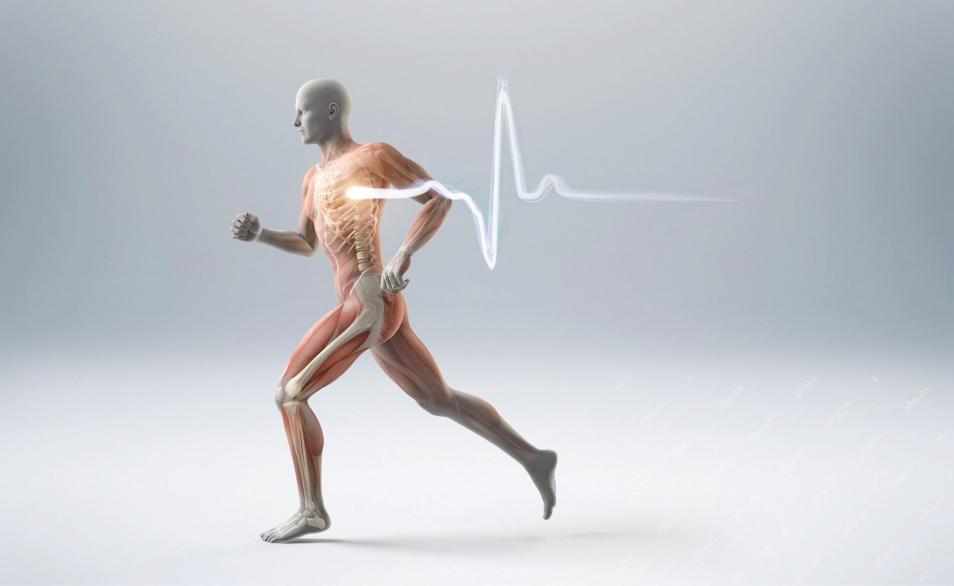
Scientists have extensively studied human and simpler neural networks (like those in C. elegans) to understand their functionality. However, a crucial question arises: how do we adapt our own neural networks to work effectively alongside novel AI s
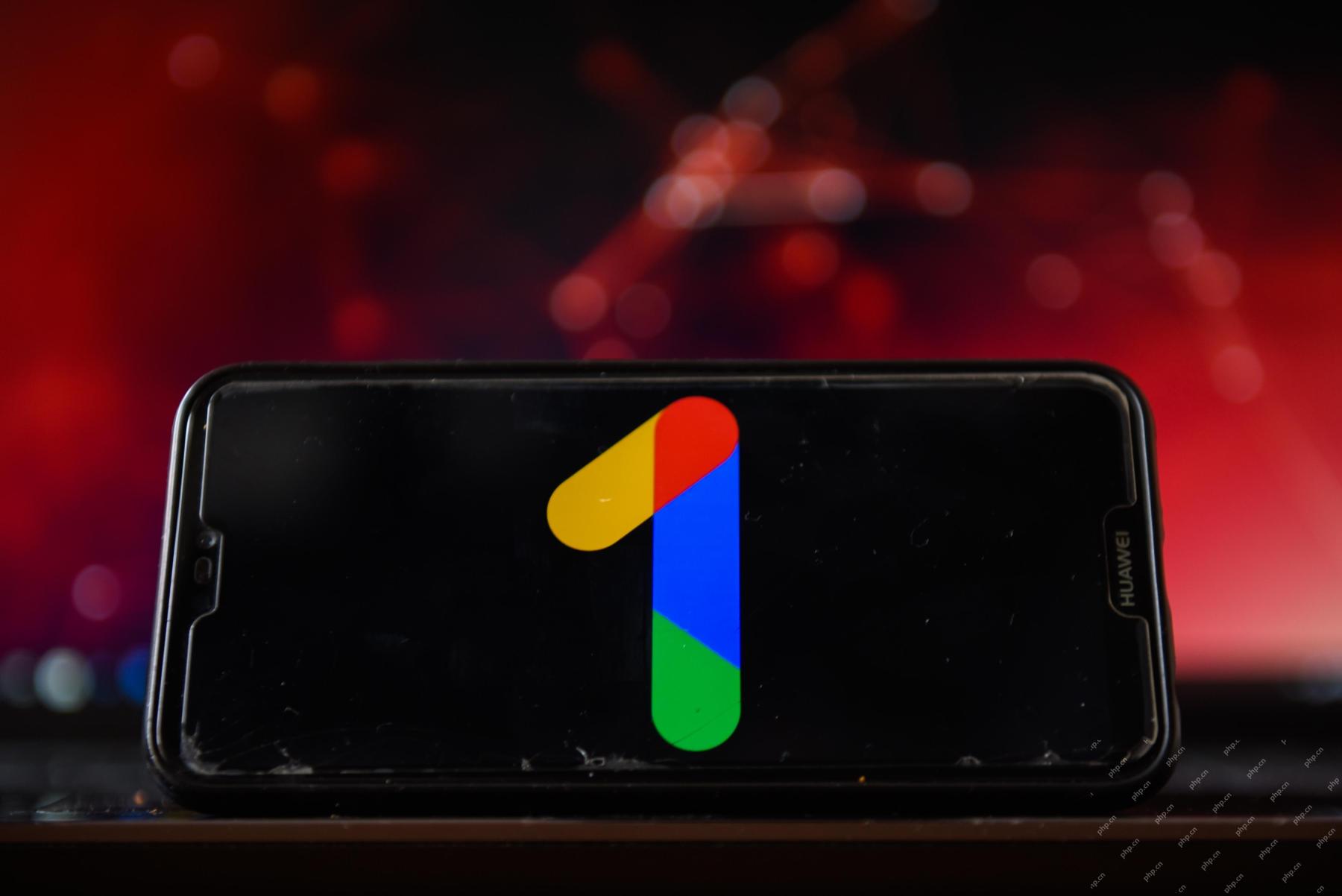
Google's Gemini Advanced: New Subscription Tiers on the Horizon Currently, accessing Gemini Advanced requires a $19.99/month Google One AI Premium plan. However, an Android Authority report hints at upcoming changes. Code within the latest Google P
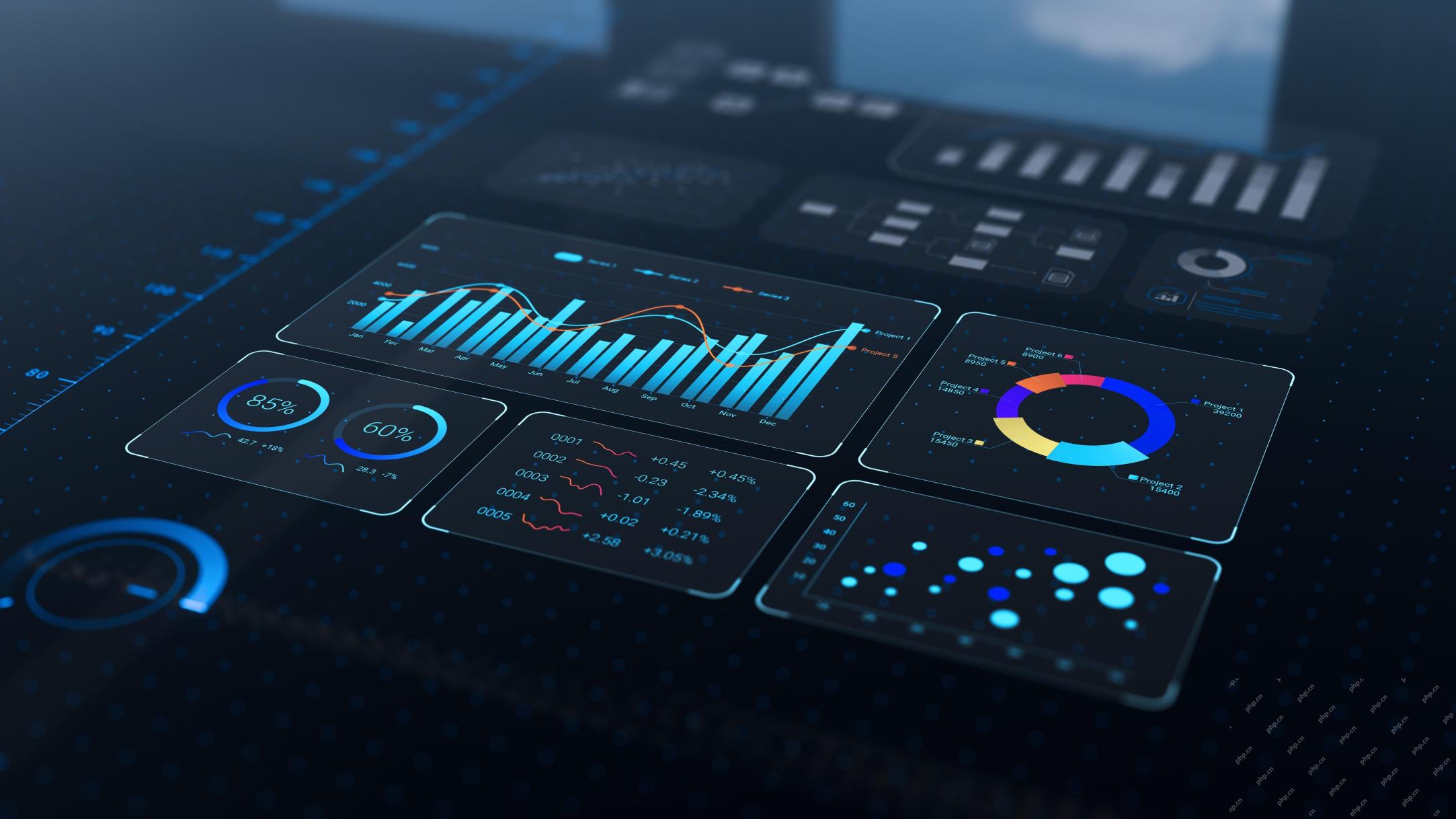
Despite the hype surrounding advanced AI capabilities, a significant challenge lurks within enterprise AI deployments: data processing bottlenecks. While CEOs celebrate AI advancements, engineers grapple with slow query times, overloaded pipelines, a

Handling documents is no longer just about opening files in your AI projects, it’s about transforming chaos into clarity. Docs such as PDFs, PowerPoints, and Word flood our workflows in every shape and size. Retrieving structured
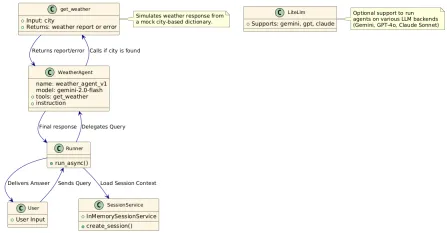
Harness the power of Google's Agent Development Kit (ADK) to create intelligent agents with real-world capabilities! This tutorial guides you through building conversational agents using ADK, supporting various language models like Gemini and GPT. W
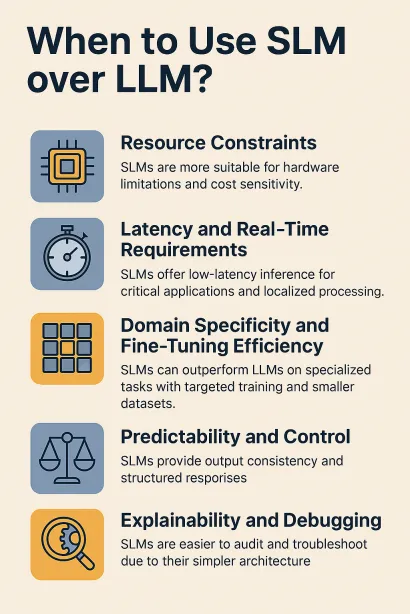
summary: Small Language Model (SLM) is designed for efficiency. They are better than the Large Language Model (LLM) in resource-deficient, real-time and privacy-sensitive environments. Best for focus-based tasks, especially where domain specificity, controllability, and interpretability are more important than general knowledge or creativity. SLMs are not a replacement for LLMs, but they are ideal when precision, speed and cost-effectiveness are critical. Technology helps us achieve more with fewer resources. It has always been a promoter, not a driver. From the steam engine era to the Internet bubble era, the power of technology lies in the extent to which it helps us solve problems. Artificial intelligence (AI) and more recently generative AI are no exception
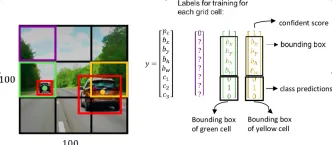
Harness the Power of Google Gemini for Computer Vision: A Comprehensive Guide Google Gemini, a leading AI chatbot, extends its capabilities beyond conversation to encompass powerful computer vision functionalities. This guide details how to utilize
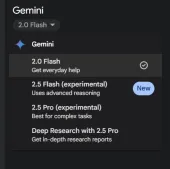
The AI landscape of 2025 is electrifying with the arrival of Google's Gemini 2.0 Flash and OpenAI's o4-mini. These cutting-edge models, launched weeks apart, boast comparable advanced features and impressive benchmark scores. This in-depth compariso


Hot AI Tools

Undresser.AI Undress
AI-powered app for creating realistic nude photos

AI Clothes Remover
Online AI tool for removing clothes from photos.

Undress AI Tool
Undress images for free

Clothoff.io
AI clothes remover

Video Face Swap
Swap faces in any video effortlessly with our completely free AI face swap tool!

Hot Article

Hot Tools
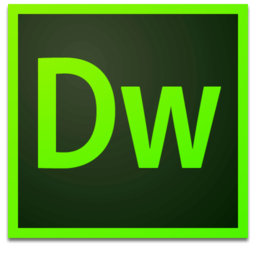
Dreamweaver Mac version
Visual web development tools

SecLists
SecLists is the ultimate security tester's companion. It is a collection of various types of lists that are frequently used during security assessments, all in one place. SecLists helps make security testing more efficient and productive by conveniently providing all the lists a security tester might need. List types include usernames, passwords, URLs, fuzzing payloads, sensitive data patterns, web shells, and more. The tester can simply pull this repository onto a new test machine and he will have access to every type of list he needs.

mPDF
mPDF is a PHP library that can generate PDF files from UTF-8 encoded HTML. The original author, Ian Back, wrote mPDF to output PDF files "on the fly" from his website and handle different languages. It is slower than original scripts like HTML2FPDF and produces larger files when using Unicode fonts, but supports CSS styles etc. and has a lot of enhancements. Supports almost all languages, including RTL (Arabic and Hebrew) and CJK (Chinese, Japanese and Korean). Supports nested block-level elements (such as P, DIV),

SublimeText3 English version
Recommended: Win version, supports code prompts!
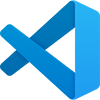
VSCode Windows 64-bit Download
A free and powerful IDE editor launched by Microsoft
