


Dilated convolution and dilated convolution are commonly used operations in convolutional neural networks. This article will introduce their differences and relationships in detail.
1. Dilated convolution
Dilated convolution, also known as dilated convolution or dilated convolution, is a convolutional neural network operations in. It is an extension based on the traditional convolution operation and increases the receptive field of the convolution kernel by inserting holes in the convolution kernel. This way, the network can better capture a wider range of features. Dilated convolution is widely used in the field of image processing and can improve the performance of the network without increasing the number of parameters and the amount of calculation. By expanding the receptive field of the convolution kernel, dilated convolution can better process the global information in the image, thereby improving the effect of feature extraction.
The main idea of dilated convolution is to introduce some intervals around the convolution kernel. These intervals allow the convolution kernel to move on the input feature map in a "jumping" manner, thereby Increase the size of the output feature map while keeping the size of the convolution kernel unchanged. Specifically, assuming that the input feature map is \sum_{m}\sum_{n}X_{(i m\times r),(j n\times r)}K_{m,n}
where r is the expansion rate, Represents the size of the hole in the convolution kernel, m and n are the row and column indexes in the convolution kernel. By changing the size of the expansion rate r, feature maps of different receptive fields can be obtained.
2. Atrous convolution
Atrous convolution is a convolution operation commonly used in convolutional neural networks. It is related to expansion The concepts of convolution are very similar, but their implementation is slightly different. The difference between dilated convolution and traditional convolution operation is that some holes are inserted in the convolution operation. These holes can make the convolution kernel "jump" move on the input feature map, thus reducing the size of the output feature map. Increase while keeping the size of the convolution kernel unchanged.
The main idea of dilated convolution is to insert some holes in the convolution kernel. These holes can make the convolution kernel "jump" move on the input feature map, thus making The size of the output feature map is increased while keeping the size of the convolution kernel unchanged. Specifically, assuming that the input feature map is \sum_{m}\sum_{n}X_{(i m\times r),(j n\times r)}K_{m,n}
where r is the void rate, Represents the size of the inserted hole, m and n are the row and column indexes in the convolution kernel. By changing the size of the hole rate r, feature maps of different receptive fields can be obtained.
3. The relationship between dilated convolution and dilated convolution
The concepts of dilated convolution and dilated convolution are very similar. An extension based on the traditional convolution operation. In fact, dilated convolution can be regarded as a special form of dilated convolution, because the hole rate d in dilated convolution is actually the hole rate r-1 in dilated convolution. Therefore, dilated convolution can be regarded as a special kind of dilated convolution, which expands the receptive field of the convolution kernel by inserting holes, and can also be implemented using dilated convolution.
In addition, both dilated convolution and dilated convolution can be used for a variety of tasks in convolutional neural networks, such as image classification, semantic segmentation, etc. They are both effective in different tasks. Can improve the performance of convolutional neural networks. However, since the hole rate d in dilated convolution is discrete, its receptive field is slightly less accurate than that of dilated convolution. Therefore, dilated convolutions may be more commonly used in tasks that require increased receptive fields.
In short, dilated convolution and dilated convolution are commonly used convolution operations in convolutional neural networks. They can be transformed into each other and can also be used in different tasks. Specifically, Which convolution operation needs to be decided based on specific task requirements.
The above is the detailed content of Compare the similarities, differences and relationships between dilated convolution and atrous convolution. For more information, please follow other related articles on the PHP Chinese website!
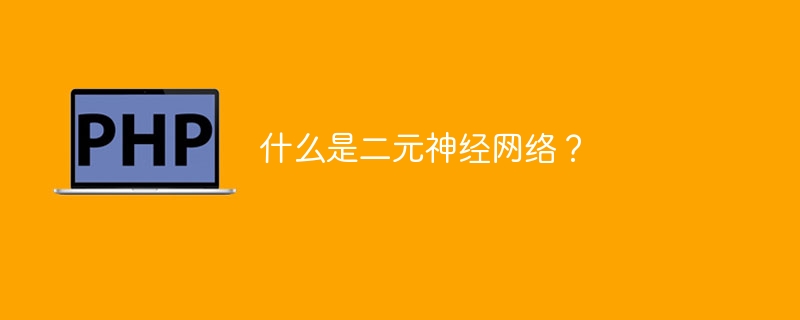
二元神经网络(BinaryNeuralNetworks,BNN)是一种神经网络,其神经元仅具有两个状态,即0或1。相对于传统的浮点数神经网络,BNN具有许多优点。首先,BNN可以利用二进制算术和逻辑运算,加快训练和推理速度。其次,BNN减少了内存和计算资源的需求,因为二进制数相对于浮点数来说需要更少的位数来表示。此外,BNN还具有提高模型的安全性和隐私性的潜力。由于BNN的权重和激活值仅为0或1,其模型参数更难以被攻击者分析和逆向工程。因此,BNN在一些对数据隐私和模型安全性有较高要求的应用中具
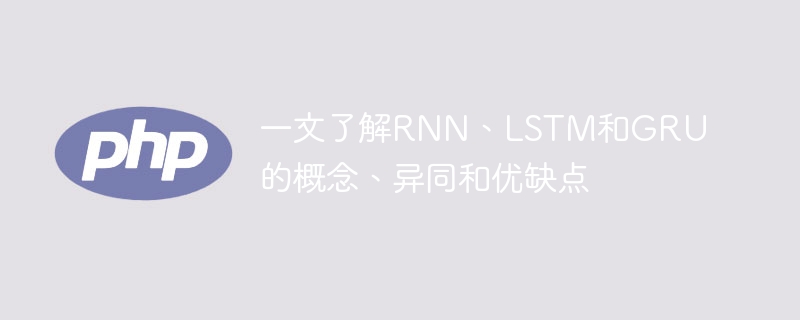
在时间序列数据中,观察之间存在依赖关系,因此它们不是相互独立的。然而,传统的神经网络将每个观察看作是独立的,这限制了模型对时间序列数据的建模能力。为了解决这个问题,循环神经网络(RNN)被引入,它引入了记忆的概念,通过在网络中建立数据点之间的依赖关系来捕捉时间序列数据的动态特性。通过循环连接,RNN可以将之前的信息传递到当前观察中,从而更好地预测未来的值。这使得RNN成为处理时间序列数据任务的强大工具。但是RNN是如何实现这种记忆的呢?RNN通过神经网络中的反馈回路实现记忆,这是RNN与传统神经
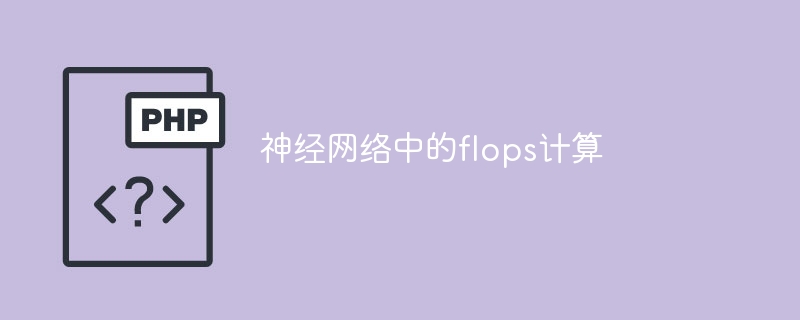
FLOPS是计算机性能评估的标准之一,用来衡量每秒的浮点运算次数。在神经网络中,FLOPS常用于评估模型的计算复杂度和计算资源的利用率。它是一个重要的指标,用来衡量计算机的计算能力和效率。神经网络是一种复杂的模型,由多层神经元组成,用于进行数据分类、回归和聚类等任务。训练和推断神经网络需要进行大量的矩阵乘法、卷积等计算操作,因此计算复杂度非常高。FLOPS(FloatingPointOperationsperSecond)可以用来衡量神经网络的计算复杂度,从而评估模型的计算资源使用效率。FLOP
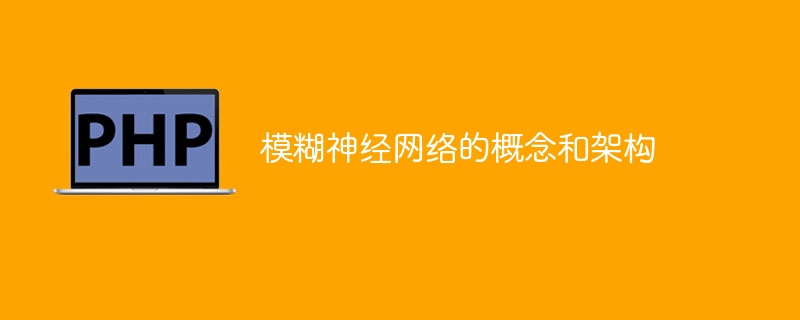
模糊神经网络是一种将模糊逻辑和神经网络结合的混合模型,用于解决传统神经网络难以处理的模糊或不确定性问题。它的设计受到人类认知中模糊性和不确定性的启发,因此被广泛应用于控制系统、模式识别、数据挖掘等领域。模糊神经网络的基本架构由模糊子系统和神经子系统组成。模糊子系统利用模糊逻辑对输入数据进行处理,将其转化为模糊集合,以表达输入数据的模糊性和不确定性。神经子系统则利用神经网络对模糊集合进行处理,用于分类、回归或聚类等任务。模糊子系统和神经子系统之间的相互作用使得模糊神经网络具备更强大的处理能力,能够
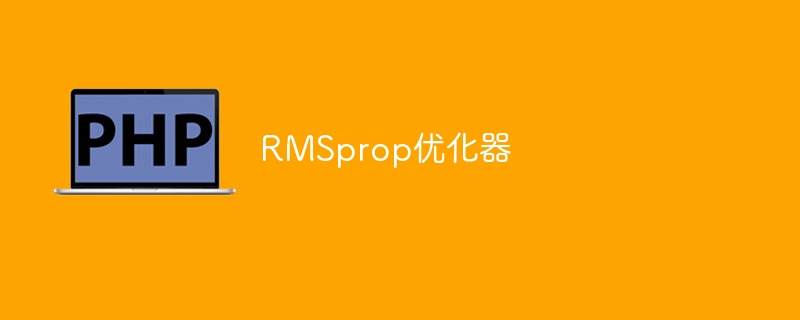
RMSprop是一种广泛使用的优化器,用于更新神经网络的权重。它是由GeoffreyHinton等人在2012年提出的,并且是Adam优化器的前身。RMSprop优化器的出现主要是为了解决SGD梯度下降算法中遇到的一些问题,例如梯度消失和梯度爆炸。通过使用RMSprop优化器,可以有效地调整学习速率,并且自适应地更新权重,从而提高深度学习模型的训练效果。RMSprop优化器的核心思想是对梯度进行加权平均,以使不同时间步的梯度对权重的更新产生不同的影响。具体而言,RMSprop会计算每个参数的平方
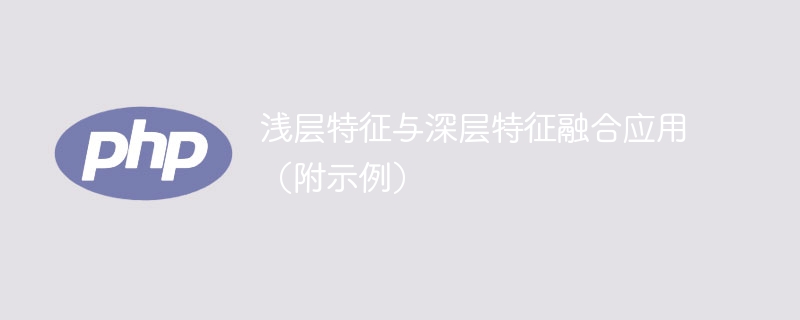
深度学习在计算机视觉领域取得了巨大成功,其中一项重要进展是使用深度卷积神经网络(CNN)进行图像分类。然而,深度CNN通常需要大量标记数据和计算资源。为了减少计算资源和标记数据的需求,研究人员开始研究如何融合浅层特征和深层特征以提高图像分类性能。这种融合方法可以利用浅层特征的高计算效率和深层特征的强表示能力。通过将两者结合,可以在保持较高分类准确性的同时降低计算成本和数据标记的要求。这种方法对于那些数据量较小或计算资源有限的应用场景尤为重要。通过深入研究浅层特征和深层特征的融合方法,我们可以进一
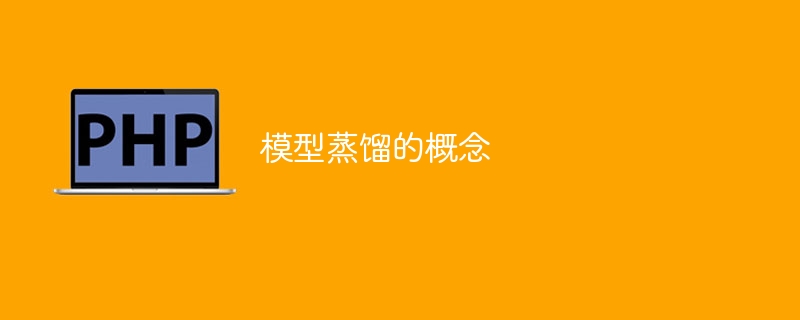
模型蒸馏是一种将大型复杂的神经网络模型(教师模型)的知识转移到小型简单的神经网络模型(学生模型)中的方法。通过这种方式,学生模型能够从教师模型中获得知识,并且在表现和泛化性能方面得到提升。通常情况下,大型神经网络模型(教师模型)在训练时需要消耗大量计算资源和时间。相比之下,小型神经网络模型(学生模型)具备更高的运行速度和更低的计算成本。为了提高学生模型的性能,同时保持较小的模型大小和计算成本,可以使用模型蒸馏技术将教师模型的知识转移给学生模型。这种转移过程可以通过将教师模型的输出概率分布作为学生
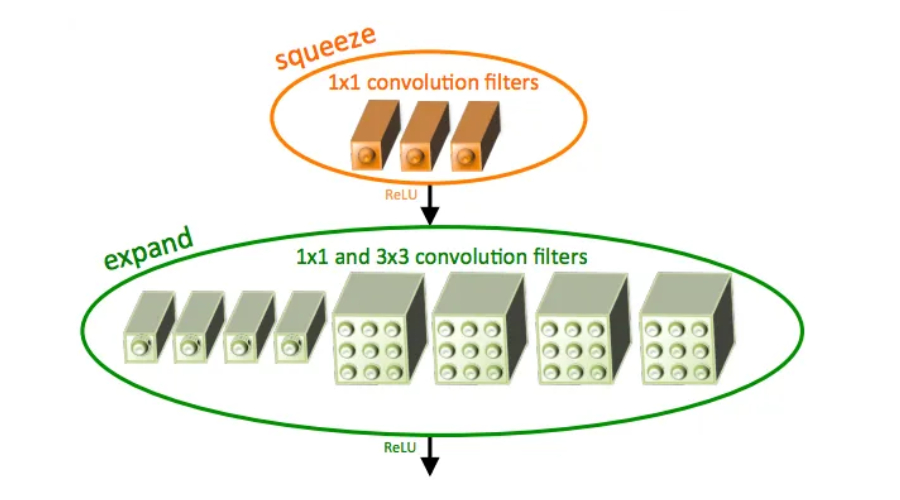
SqueezeNet是一种小巧而精确的算法,它在高精度和低复杂度之间达到了很好的平衡,因此非常适合资源有限的移动和嵌入式系统。2016年,DeepScale、加州大学伯克利分校和斯坦福大学的研究人员提出了一种紧凑高效的卷积神经网络(CNN)——SqueezeNet。近年来,研究人员对SqueezeNet进行了多次改进,其中包括SqueezeNetv1.1和SqueezeNetv2.0。这两个版本的改进不仅提高了准确性,还降低了计算成本。SqueezeNetv1.1在ImageNet数据集上的精度


Hot AI Tools

Undresser.AI Undress
AI-powered app for creating realistic nude photos

AI Clothes Remover
Online AI tool for removing clothes from photos.

Undress AI Tool
Undress images for free

Clothoff.io
AI clothes remover

AI Hentai Generator
Generate AI Hentai for free.

Hot Article

Hot Tools

PhpStorm Mac version
The latest (2018.2.1) professional PHP integrated development tool
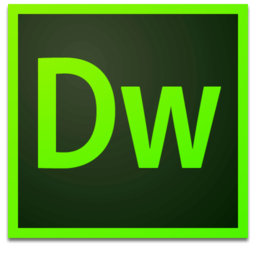
Dreamweaver Mac version
Visual web development tools

Notepad++7.3.1
Easy-to-use and free code editor

MinGW - Minimalist GNU for Windows
This project is in the process of being migrated to osdn.net/projects/mingw, you can continue to follow us there. MinGW: A native Windows port of the GNU Compiler Collection (GCC), freely distributable import libraries and header files for building native Windows applications; includes extensions to the MSVC runtime to support C99 functionality. All MinGW software can run on 64-bit Windows platforms.
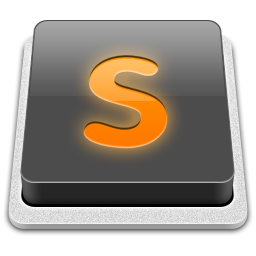
SublimeText3 Mac version
God-level code editing software (SublimeText3)
