On January 22, the Yi series model family welcomed a new member: Yi Vision Language (Yi-VL) multi-modal language large model is officially open source to the world. It is reported that the Yi-VL model is developed based on the Yi language model, including two versions: Yi-VL-34B and Yi-VL-6B. Yi-VL model open source address:
- https://huggingface.co/01-ai
- https://www.modelscope.cn/organization/01ai
##With excellent image and text understanding and dialogue generation Ability, the Yi-VL model has achieved leading results on the English data set MMMU and the Chinese data set CMMMU, demonstrating its strong strength in complex interdisciplinary tasks.
MMMU (full name Massive Multi-discipline Multi-modal Understanding & Reasoning) data set contains 11,500 data from six core Problems in disciplines (Art & Design, Business, Science, Health & Medicine, Humanities & Social Sciences, and Technology & Engineering) involving highly heterogeneous image types and intertwined text-image information pose extremely high demands on the model's advanced perception and reasoning capabilities. Require. On this test set,
Yi-VL-34B surpassed a series of multi-modal large models with an accuracy of 41.6%, second only to GPT-4V (55.7%), showing strong cross- Ability to understand and apply subject knowledge. 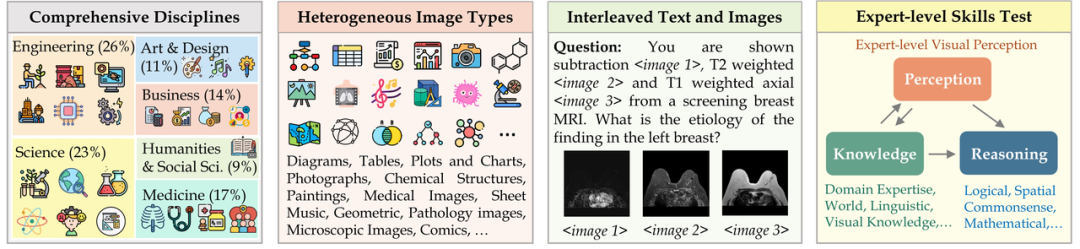
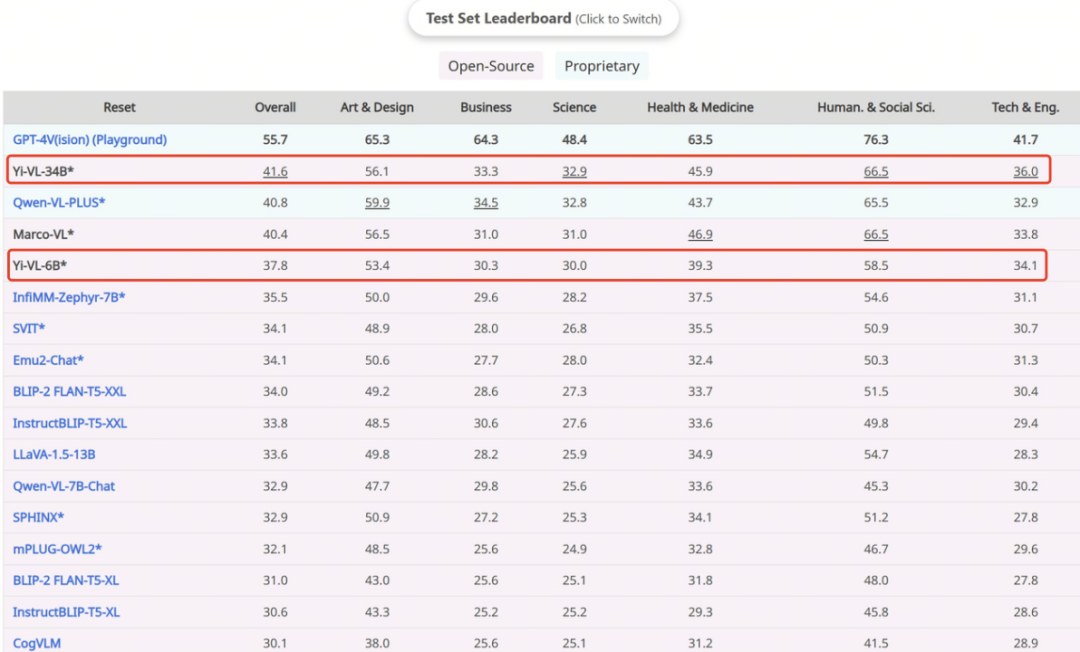
Source: https://mmmu-benchmark.github.ioOn the CMMMU data set created for the Chinese scene, the Yi-VL model shows the unique advantage of "understanding Chinese people better". CMMMU contains approximately 12,000 Chinese multimodal questions derived from university exams, quizzes, and textbooks. Among them,
GPT-4V has an accuracy of 43.7% on this test set, followed closely by Yi-VL-34B with an accuracy of 36.5%, ranking among the existing open source multi-modal models. Leading position. 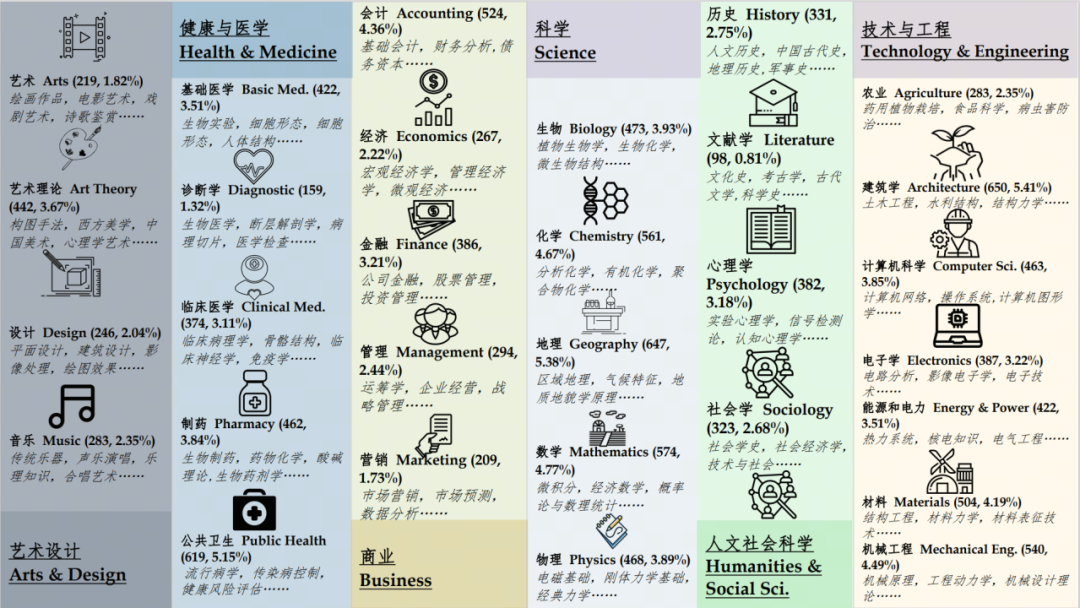
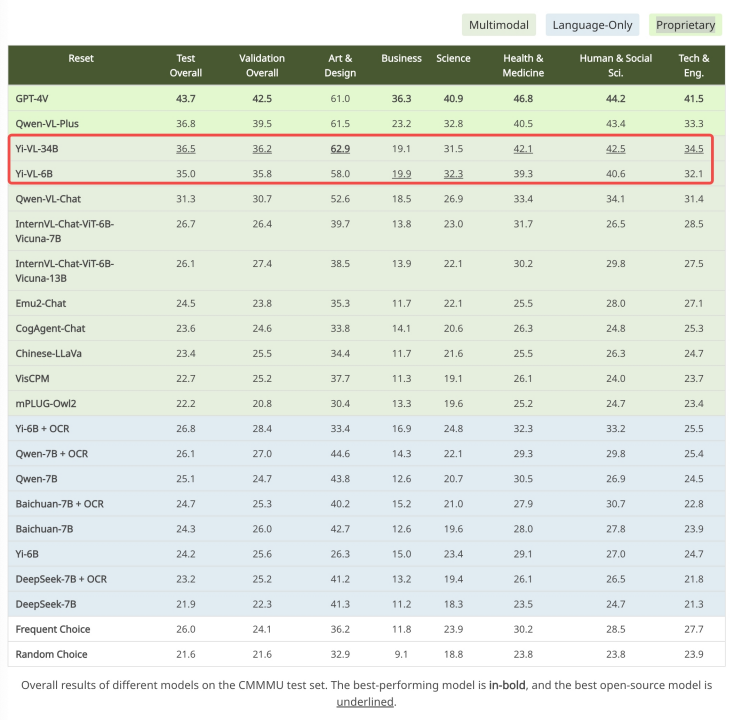
## Source: https://cmmmu-benchmark.github.io/
So, how does the Yi-VL model perform in diverse scenarios such as graphic and text dialogues?
Let’s look at two examples first: 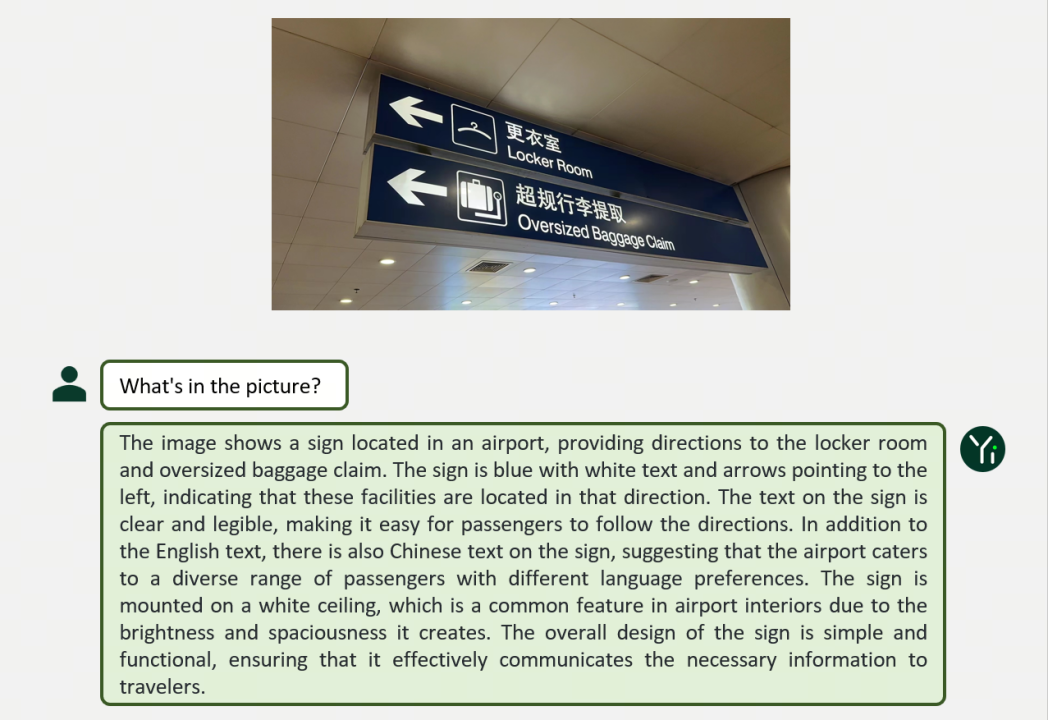
As you can see, Based on the powerful text understanding capabilities of the Yi language model, you can get a good multi-modal visual language model by simply aligning the pictures - this is also one of the core highlights of the Yi-VL model.
Yi-VL model architecture design and training method process overview. In terms of architecture design, the Yi-VL model is based on the open source LLaVA architecture and contains three Main module:
- Vision Transformer (ViT for short) is used for image encoding, using the open source OpenClip ViT-H/14 model to initialize trainable parameters. By learning to extract features from large-scale "image-text" pairs, the model has the ability to process and understand images.
- The Projection module brings the ability to spatially align image features with text features to the model. This module consists of a Multilayer Perceptron (MLP) containing layer normalizations. This design allows the model to more effectively fuse and process visual and text information, improving the accuracy of multi-modal understanding and generation.
- The introduction of Yi-34B-Chat and Yi-6B-Chat large-scale language models provides Yi-VL with powerful language understanding and generation capabilities. This part of the model uses advanced natural language processing technology to help Yi-VL deeply understand complex language structures and generate coherent and relevant text output.
In terms of training method, the training process of the Yi-VL model is divided into three carefully designed stages, aiming to Comprehensively improve the model’s visual and language processing capabilities.
- The first stage: Zero One Wish uses 100 million "image-text" paired data sets to train ViT and Projection modules. At this stage, the image resolution is set to 224x224 to enhance ViT’s knowledge acquisition capabilities in specific architectures while enabling efficient alignment with large language models.
- The second stage: Zero One Thing increases the image resolution of ViT to 448x448. This improvement makes the model better at recognizing complex visual details. This stage uses approximately 25 million image-text pairs.
- The third stage: Zero One Wish opens the parameters of the entire model for training, with the goal of improving the model's performance in multi-modal chat interaction. The training data covers a diverse range of data sources, with a total of approximately 1 million "image-text" pairs, ensuring the breadth and balance of the data.
The zero-yiwu technical team also verified that it can be trained with other multi-modal methods based on the powerful language understanding and generation capabilities of the Yi language model. Methods such as BLIP, Flamingo, EVA, etc. can quickly train multi-modal graphic and text models that can perform efficient image understanding and smooth graphic and text dialogue. The Yi series models can be used as base language models for multimodal models, providing a new option for the open source community. Currently, the Yi-VL model has been opened to the public on Hugging Face, ModelScope and other platforms. Users can experience the multiple functions of this model through the following link: Excellent performance in the scene. Welcome to explore the powerful functions of Yi-VL multi-modal language model and experience cutting-edge AI technology achievements. The above is the detailed content of Yi-VL large model is open source and ranks first in MMMU and CMMMU. For more information, please follow other related articles on the PHP Chinese website!