Research on biases and self-correction methods of language models
The bias of language models is that when generating text, there may be a bias towards certain groups of people, themes or topics, resulting in the text being unbiased, neutral or discriminatory. This bias may arise from factors such as training data selection, training algorithm design, or model structure. To solve this problem, we need to focus on data diversity and ensure that training data includes a variety of backgrounds and perspectives. Additionally, we should review training algorithms and model structures to ensure their fairness and neutrality to improve the quality and inclusivity of generated text.
For example, there may be an excessive bias toward certain categories in the training data, causing the model to favor those categories when generating text. This bias may cause the model to perform poorly when dealing with other categories, affecting the performance of the model. In addition, the design of the model may contain some discriminatory assumptions or biases, such as stereotypes about certain groups of people. These biases can lead to unfair results when the model processes relevant data. Therefore, when applying models in fields such as natural language processing and social media analysis, these issues need to be evaluated and resolved to ensure the fairness and accuracy of the model.
Language models can self-correct biases in the following ways:
1. Data cleaning
Clean and balance the training data to avoid gender, racial, regional and other biases. Implemented using methods such as data preprocessing and enhancement.
2. Diverse Datasets
Use diverse, diverse data sets for training to avoid bias. This can be achieved by collecting broader data, cross-domain data, etc.
3. Regularization
During the training process, the model weight is restricted through the regularization method to avoid biasing towards certain specific models. enter. For example, you can use L1 or L2 regularization methods to limit the size of the model weights.
4. Balanced Sampling
In the training data, different categories of data are sampled in a balanced manner so that the model can better learn each category. Characteristics. For example, the data set can be balanced using oversampling, undersampling, etc.
5. Heuristic rules
Introduce heuristic rules to correct bias, for example, prohibiting the model from using some phrases that may lead to discrimination or vocabulary. For example, sensitive word filtering, sensitive word replacement, etc. can be used to avoid generating discriminatory text.
6. Supervised learning
Use the knowledge of human experts to perform supervised learning on the model. For example, let the experts conduct supervised learning on the text generated by the model. Evaluate and revise to improve model accuracy and fairness. For example, human review, manual correction, etc. can be used to review and correct text generated by the model.
7. Multi-task learning
During the training process, the language model is combined with other tasks for multi-task learning to improve the model generalization ability and fairness. For example, tasks such as sentiment analysis and text classification can be combined with language models for joint training.
8. Adversarial training
Through adversarial learning, the model can avoid bias when generating text. For example, an adversarial example generator can be used to perturb the text generated by the model to improve the robustness and fairness of the model.
9. Evaluation Metrics
When evaluating the performance of a language model, evaluate it using multiple fairness metrics to avoid evaluation bias. For example, the model can be evaluated using indicators such as fairness accuracy and fairness recall.
10. Feedback mechanism
Establish a user feedback mechanism to allow users to provide feedback on the text generated by the model to help the model self-correct biases. For example, a user feedback platform can be established to allow users to evaluate and provide feedback on the text generated by the model.
These methods can be used alone or in combination to achieve self-correction of language model biases.
The above is the detailed content of Research on biases and self-correction methods of language models. For more information, please follow other related articles on the PHP Chinese website!
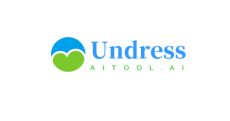
https://undressaitool.ai/ is Powerful mobile app with advanced AI features for adult content. Create AI-generated pornographic images or videos now!
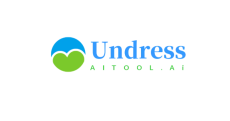
Tutorial on using undressAI to create pornographic pictures/videos: 1. Open the corresponding tool web link; 2. Click the tool button; 3. Upload the required content for production according to the page prompts; 4. Save and enjoy the results.
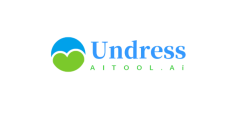
The official address of undress AI is:https://undressaitool.ai/;undressAI is Powerful mobile app with advanced AI features for adult content. Create AI-generated pornographic images or videos now!
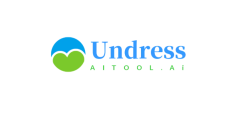
Tutorial on using undressAI to create pornographic pictures/videos: 1. Open the corresponding tool web link; 2. Click the tool button; 3. Upload the required content for production according to the page prompts; 4. Save and enjoy the results.
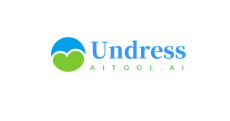
The official address of undress AI is:https://undressaitool.ai/;undressAI is Powerful mobile app with advanced AI features for adult content. Create AI-generated pornographic images or videos now!
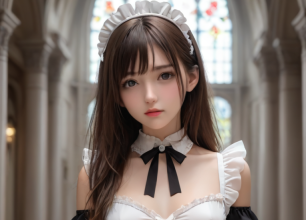
Tutorial on using undressAI to create pornographic pictures/videos: 1. Open the corresponding tool web link; 2. Click the tool button; 3. Upload the required content for production according to the page prompts; 4. Save and enjoy the results.
![[Ghibli-style images with AI] Introducing how to create free images with ChatGPT and copyright](https://img.php.cn/upload/article/001/242/473/174707263295098.jpg?x-oss-process=image/resize,p_40)
The latest model GPT-4o released by OpenAI not only can generate text, but also has image generation functions, which has attracted widespread attention. The most eye-catching feature is the generation of "Ghibli-style illustrations". Simply upload the photo to ChatGPT and give simple instructions to generate a dreamy image like a work in Studio Ghibli. This article will explain in detail the actual operation process, the effect experience, as well as the errors and copyright issues that need to be paid attention to. For details of the latest model "o3" released by OpenAI, please click here⬇️ Detailed explanation of OpenAI o3 (ChatGPT o3): Features, pricing system and o4-mini introduction Please click here for the English version of Ghibli-style article⬇️ Create Ji with ChatGPT
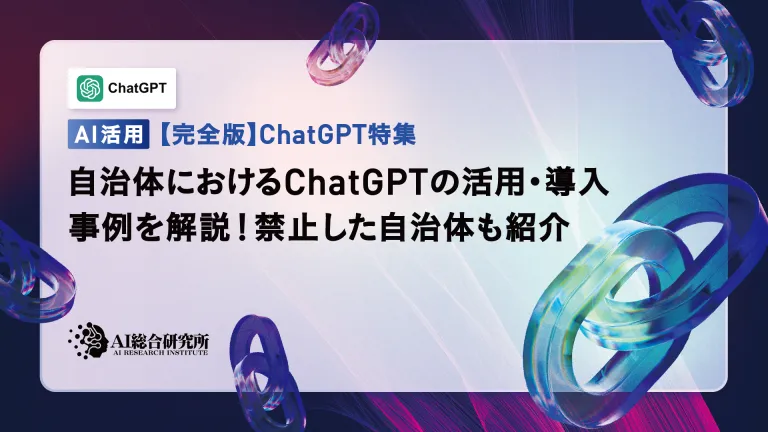
As a new communication method, the use and introduction of ChatGPT in local governments is attracting attention. While this trend is progressing in a wide range of areas, some local governments have declined to use ChatGPT. In this article, we will introduce examples of ChatGPT implementation in local governments. We will explore how we are achieving quality and efficiency improvements in local government services through a variety of reform examples, including supporting document creation and dialogue with citizens. Not only local government officials who aim to reduce staff workload and improve convenience for citizens, but also all interested in advanced use cases.


Hot AI Tools

Undresser.AI Undress
AI-powered app for creating realistic nude photos

AI Clothes Remover
Online AI tool for removing clothes from photos.

Undress AI Tool
Undress images for free

Clothoff.io
AI clothes remover

Video Face Swap
Swap faces in any video effortlessly with our completely free AI face swap tool!

Hot Article

Hot Tools
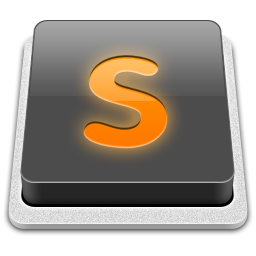
SublimeText3 Mac version
God-level code editing software (SublimeText3)
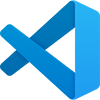
VSCode Windows 64-bit Download
A free and powerful IDE editor launched by Microsoft
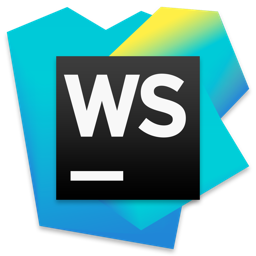
WebStorm Mac version
Useful JavaScript development tools

PhpStorm Mac version
The latest (2018.2.1) professional PHP integrated development tool
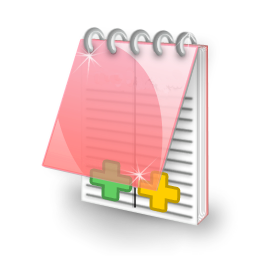
EditPlus Chinese cracked version
Small size, syntax highlighting, does not support code prompt function
