


Transfer learning applications and common technologies in large language model training
Large-scale language models refer to natural language processing models with more than 100 million parameters. Due to their sheer size and complexity, training such a model requires significant computing resources and data. Therefore, transfer learning has become an important method for training large language models. By utilizing existing models and data, the training process can be accelerated and performance can be improved. Transfer learning can transfer the parameters and knowledge of models trained on other tasks to the target task, thereby reducing data requirements and training time. This approach is widely used in both research and industry, laying the foundation for building more powerful language models.
Transfer learning is a method that uses an already trained model to adjust its parameters or some components when solving other tasks. In the field of natural language processing, transfer learning can improve the performance of other tasks by pre-training large language models, thereby reducing the time and amount of data required to train new tasks. This approach can help solve problems in specific tasks by leveraging the general language knowledge learned by the model on large-scale text data. Through transfer learning, we can transfer the knowledge of previously learned models to new tasks, thereby speeding up the training process of new tasks and often achieving better performance.
In transfer learning of large language models, there are several key issues to consider:
1. The choice of pre-training tasks is very Crucially, it needs to have enough complexity and diversity to fully utilize training data and computing resources, and to improve the performance of other tasks. Currently, the most common pre-training tasks include language models, masked language models, entity recognition, and text classification. These tasks can help the model learn the structure, grammar and semantics of language, thereby improving its performance in various natural language processing tasks. When selecting a pre-training task, it is necessary to comprehensively consider the availability of data and computing resources, as well as the relevance of the pre-training task to the target task. By rationally selecting pre-training tasks, the generalization ability of the model can be enhanced and the model's practical application can be improved.
When selecting a pre-training model, you need to consider the number of parameters, model complexity and training data. Currently popular ones include BERT, GPT, XLNet, etc.
3. Selection of fine-tuning strategy: Fine-tuning refers to using a small amount of task-specific data to adjust model parameters based on the pre-trained model to adapt to new tasks. The fine-tuning strategy should consider factors such as the size, quality, and diversity of the fine-tuning data, the selection of hyper-parameters such as the number of fine-tuning layers, learning rate, and regularization, and whether the parameters of some layers need to be frozen during the fine-tuning process.
In practice, the best transfer learning methods for large language models often include the following steps:
- Pre-training: Choose a Pre-training tasks and pre-trained models that are suitable for the current task, and use sufficient training data and computing resources for pre-training.
- Fine-tuning: Select appropriate fine-tuning strategies and hyper-parameters based on the characteristics and requirements of the new task, and use a small amount of task-specific data for fine-tuning.
- Performance evaluation and adjustment: Evaluate the performance of the model on new tasks, and adjust and improve the model according to actual needs.
It should be noted that in transfer learning, the quality and adaptability of the pre-trained model have a great impact on the final performance. Therefore, selecting appropriate pre-training tasks and models, and using sufficient training data and computing resources for pre-training are the keys to ensuring the effect of transfer learning. In addition, the selection of fine-tuning strategies and hyperparameters also need to be adjusted and optimized according to actual needs to achieve the best performance and efficiency.
For transfer learning of large language models, there are several common methods to choose from. Below is a detailed introduction to these methods to ensure that the information is true and correct.
1. Fine-tuning
Fine-tuning is the most common transfer learning method for large language models. In the fine-tuning process, the language model is first pre-trained using a large-scale data set (such as a general language model). Then, the weights of the pre-trained model are used as initial parameters for further training using small-scale data sets in specific fields. This allows the model to be adapted to a specific task while retaining the general knowledge pre-trained at scale.
2. Transfer learning based on feature extraction
This method involves using a pre-trained language model as a feature extractor. First, by passing the input data of the task to be solved to the pre-trained model, its hidden layer representation is obtained. These hidden layer representations can then be fed as features into new task-specific models, such as Support Vector Machines (SVMs) or Random Forests. This approach is especially suitable when the data set is small, because the pre-trained model can provide meaningful features.
3. Multi-task learning
Multi-task learning is a transfer learning method that shares knowledge by training multiple related tasks simultaneously. In large language models, datasets from multiple tasks can be combined and then used to train the model. The shared underlying language representation can help the model learn common language structures and semantic knowledge, thereby improving the model's performance on various tasks.
4. Combination of pre-training and task-specific architecture
This method combines the advantages of pre-training and task-specific architecture. First, a large-scale language model is used for pre-training to obtain a universal language representation. Then, a task-specific architecture is designed for the specific task, which can receive the output of the pre-trained model and perform further training and fine-tuning. This allows the model to be customized for specific tasks while retaining general knowledge.
5. Hierarchical method of transfer learning
Hierarchical transfer learning is a method that uses different levels of knowledge of the pre-trained model for Task-specific methods. Lower levels of knowledge typically contain more general and abstract information, while higher levels of knowledge are more specific and task-related. By performing fine-tuning or feature extraction at different levels of the model, the appropriate level of knowledge can be selected and utilized based on the needs of the task.
In general, through transfer learning, the general knowledge of large language models can be fully utilized and applied to various specific tasks, thereby improving the performance and generalization ability of the model. .
The above is the detailed content of Transfer learning applications and common technologies in large language model training. For more information, please follow other related articles on the PHP Chinese website!
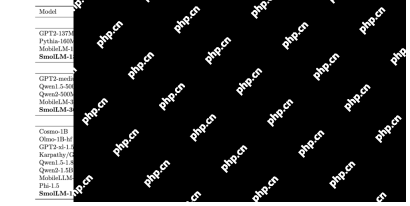
Harness the Power of On-Device AI: Building a Personal Chatbot CLI In the recent past, the concept of a personal AI assistant seemed like science fiction. Imagine Alex, a tech enthusiast, dreaming of a smart, local AI companion—one that doesn't rely
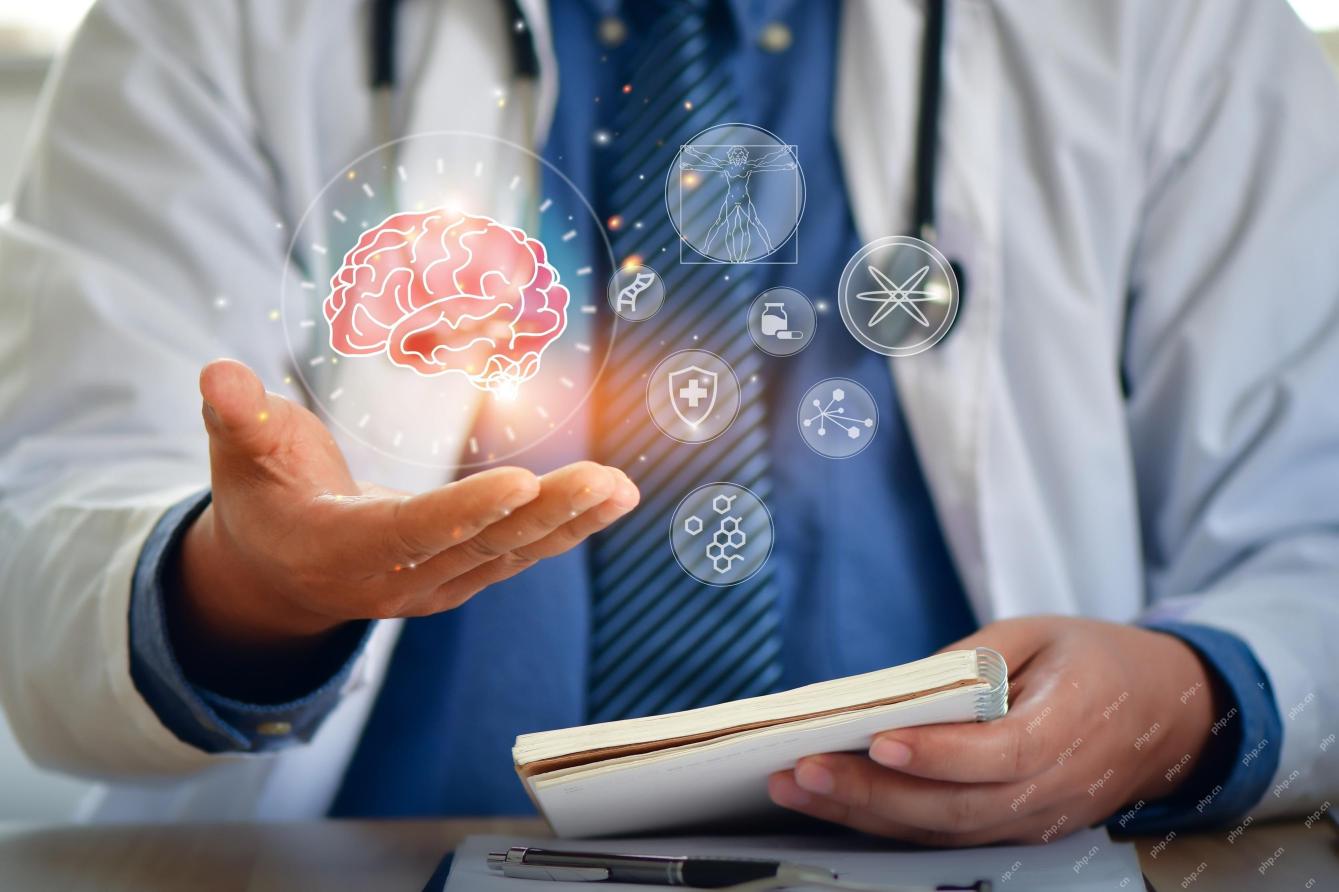
Their inaugural launch of AI4MH took place on April 15, 2025, and luminary Dr. Tom Insel, M.D., famed psychiatrist and neuroscientist, served as the kick-off speaker. Dr. Insel is renowned for his outstanding work in mental health research and techno
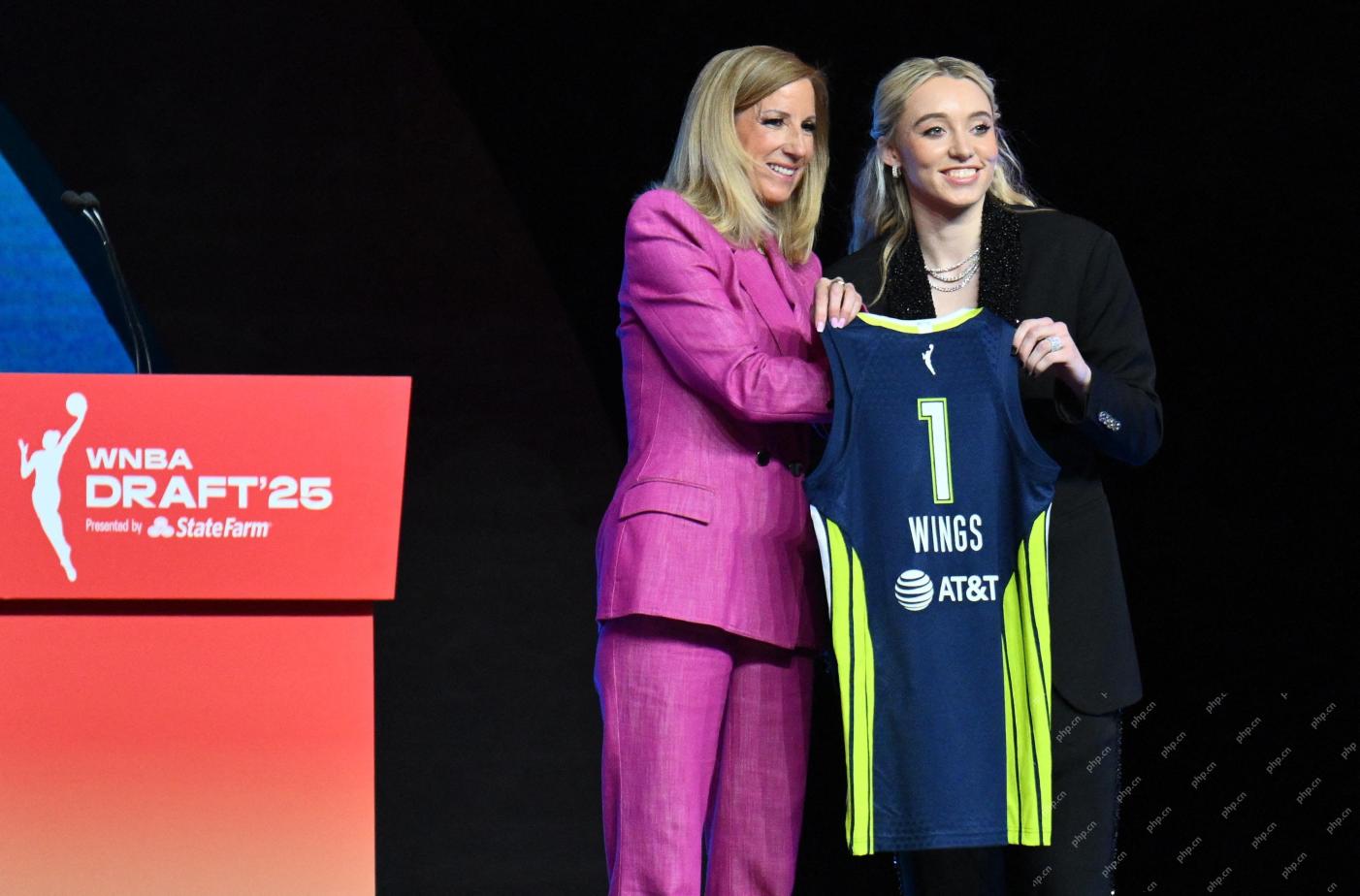
"We want to ensure that the WNBA remains a space where everyone, players, fans and corporate partners, feel safe, valued and empowered," Engelbert stated, addressing what has become one of women's sports' most damaging challenges. The anno
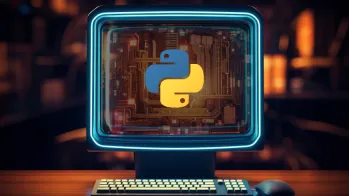
Introduction Python excels as a programming language, particularly in data science and generative AI. Efficient data manipulation (storage, management, and access) is crucial when dealing with large datasets. We've previously covered numbers and st
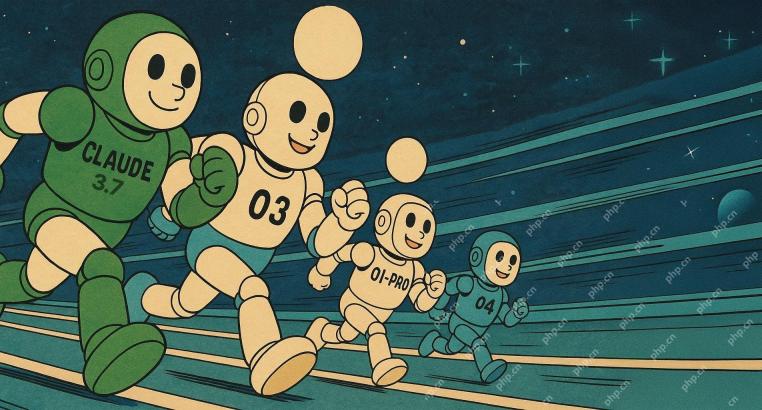
Before diving in, an important caveat: AI performance is non-deterministic and highly use-case specific. In simpler terms, Your Mileage May Vary. Don't take this (or any other) article as the final word—instead, test these models on your own scenario
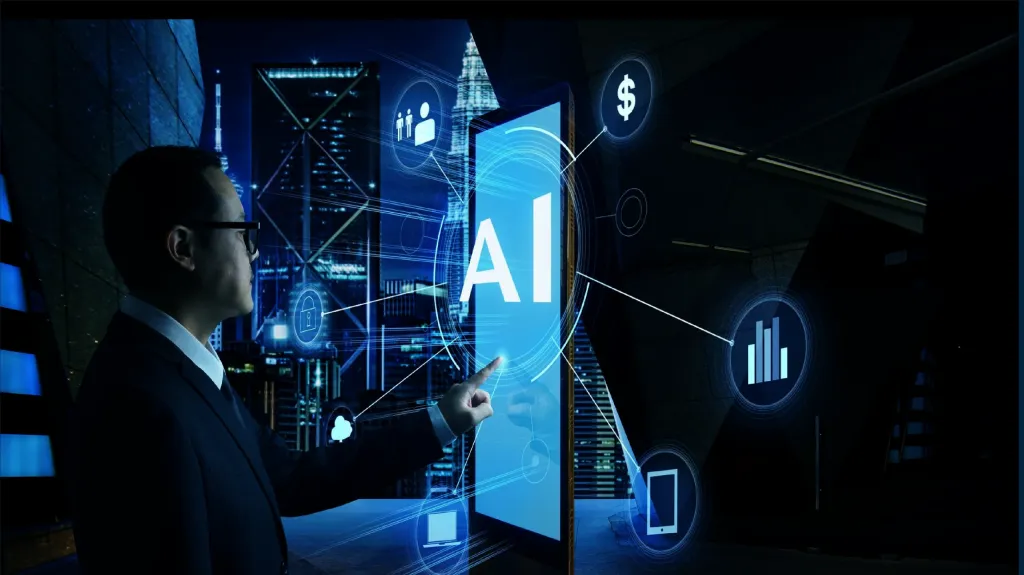
Building a Standout AI/ML Portfolio: A Guide for Beginners and Professionals Creating a compelling portfolio is crucial for securing roles in artificial intelligence (AI) and machine learning (ML). This guide provides advice for building a portfolio
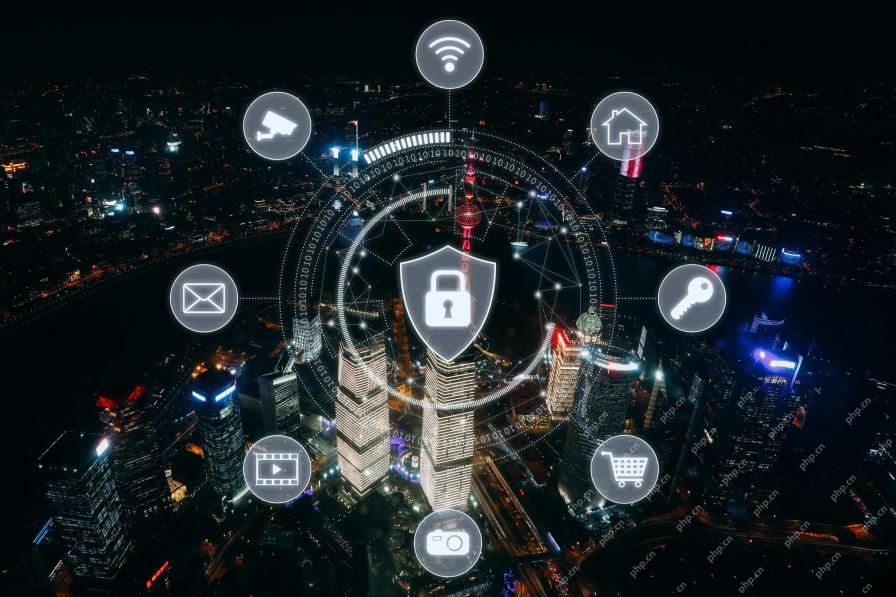
The result? Burnout, inefficiency, and a widening gap between detection and action. None of this should come as a shock to anyone who works in cybersecurity. The promise of agentic AI has emerged as a potential turning point, though. This new class
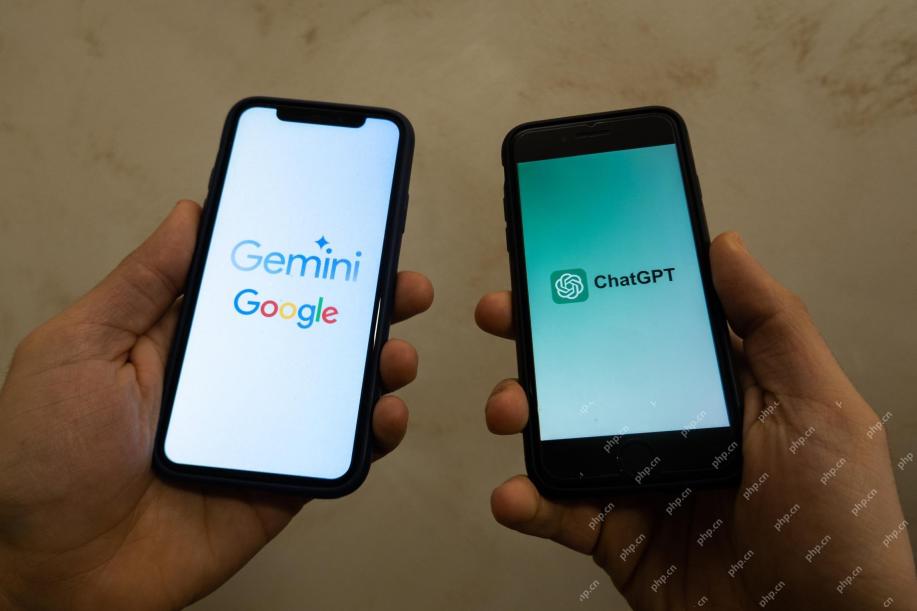
Immediate Impact versus Long-Term Partnership? Two weeks ago OpenAI stepped forward with a powerful short-term offer, granting U.S. and Canadian college students free access to ChatGPT Plus through the end of May 2025. This tool includes GPT‑4o, an a


Hot AI Tools

Undresser.AI Undress
AI-powered app for creating realistic nude photos

AI Clothes Remover
Online AI tool for removing clothes from photos.

Undress AI Tool
Undress images for free

Clothoff.io
AI clothes remover

AI Hentai Generator
Generate AI Hentai for free.

Hot Article

Hot Tools

Notepad++7.3.1
Easy-to-use and free code editor

SecLists
SecLists is the ultimate security tester's companion. It is a collection of various types of lists that are frequently used during security assessments, all in one place. SecLists helps make security testing more efficient and productive by conveniently providing all the lists a security tester might need. List types include usernames, passwords, URLs, fuzzing payloads, sensitive data patterns, web shells, and more. The tester can simply pull this repository onto a new test machine and he will have access to every type of list he needs.

PhpStorm Mac version
The latest (2018.2.1) professional PHP integrated development tool

Atom editor mac version download
The most popular open source editor
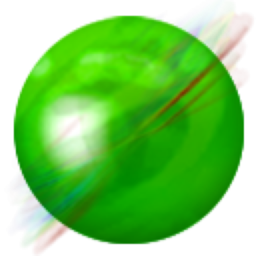
ZendStudio 13.5.1 Mac
Powerful PHP integrated development environment