


Image segmentation is an important task in the field of computer vision. Its goal is to divide an image into several non-overlapping regions, and the pixels in each region have similar characteristics. Image segmentation plays an important role in many applications such as medical image analysis, autonomous driving, and drone monitoring. By segmenting the image into regions, we can better understand and process each part of the image, providing a more accurate and effective basis for subsequent analysis and processing.
In image segmentation, annotation refers to manually labeling each pixel to the category or region to which it belongs. Accurate annotations are crucial for training machine learning models because they form the basis for the model to learn image features. Annotation consistency refers to the consistency of the results obtained when multiple annotators annotate the same image. To ensure the accuracy and consistency of annotation, multiple annotators are usually required to annotate the same image in practical applications. This multi-person annotation method can provide a more reliable data basis for model training.
The impact of the consistency of annotations on the model can be discussed from the following aspects:
Data quality is a key factor, and annotations The consistency directly affects the quality of data. If there are large differences between different annotators, the quality of the labeled data will be reduced, which in turn affects the model's ability to learn accurate features from it. Therefore, there needs to be as much consistency as possible between annotators to improve the quality of the data.
The consistency of annotations has an important impact on the effectiveness of the training model. Low consistency can lead to overfitting or underfitting. To improve generalization, annotators should be consistent.
3. Model performance: The consistency of annotations will also directly affect the performance of the model. If the agreement between annotators is higher, the performance of the trained model will be better. On the other hand, if the agreement between annotators is low, the performance of the model will decrease accordingly.
4. Data volume: Consistency of annotations also affects the amount of data required. If the agreement between annotators is high, less data can be used to train the model. On the contrary, if the agreement between annotators is low, then more data need to be used to train the model to improve the performance of the model.
In order to improve the consistency of annotations, the following methods can be adopted:
1. Training annotators: Annotators should undergo specialized Training to learn how to annotate images correctly. Training can include theoretical knowledge and practical operations.
2. Define accurate standards: Annotators should follow accurate annotation standards, and these standards should be clear and unambiguous. For example, characteristics such as pixel color or texture that each category represents should be defined.
3. Use multiple annotators: Use multiple annotators to annotate the same image, and then use some statistical methods to fuse these annotation results. This can reduce differences between annotators and improve annotation consistency.
4. Automated annotation: Use automated methods to annotate images, such as segmentation using deep learning models. Although automated methods also have errors, the accuracy and consistency of annotations can be improved through subsequent manual verification.
You can also obtain annotated image data sets through NetEase crowdsourcing data service.
In short, the consistency of annotations has an important impact on the performance of image segmentation models. The higher the agreement between annotators, the better the model’s generalization ability and performance. In order to improve the consistency of annotation, methods such as training annotators, defining accurate standards, using multiple annotators and automating annotation can be adopted. These methods can help improve data quality, training effectiveness, model performance, and reduce the amount of data required.
The above is the detailed content of What effect does the annotation consistency of the model have on image segmentation?. For more information, please follow other related articles on the PHP Chinese website!
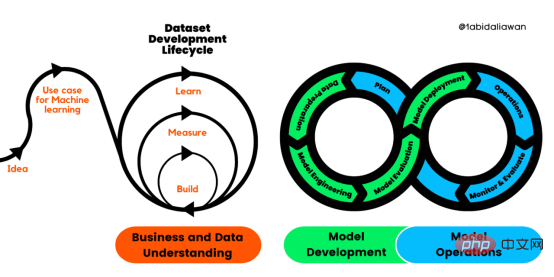
译者 | 布加迪审校 | 孙淑娟目前,没有用于构建和管理机器学习(ML)应用程序的标准实践。机器学习项目组织得不好,缺乏可重复性,而且从长远来看容易彻底失败。因此,我们需要一套流程来帮助自己在整个机器学习生命周期中保持质量、可持续性、稳健性和成本管理。图1. 机器学习开发生命周期流程使用质量保证方法开发机器学习应用程序的跨行业标准流程(CRISP-ML(Q))是CRISP-DM的升级版,以确保机器学习产品的质量。CRISP-ML(Q)有六个单独的阶段:1. 业务和数据理解2. 数据准备3. 模型
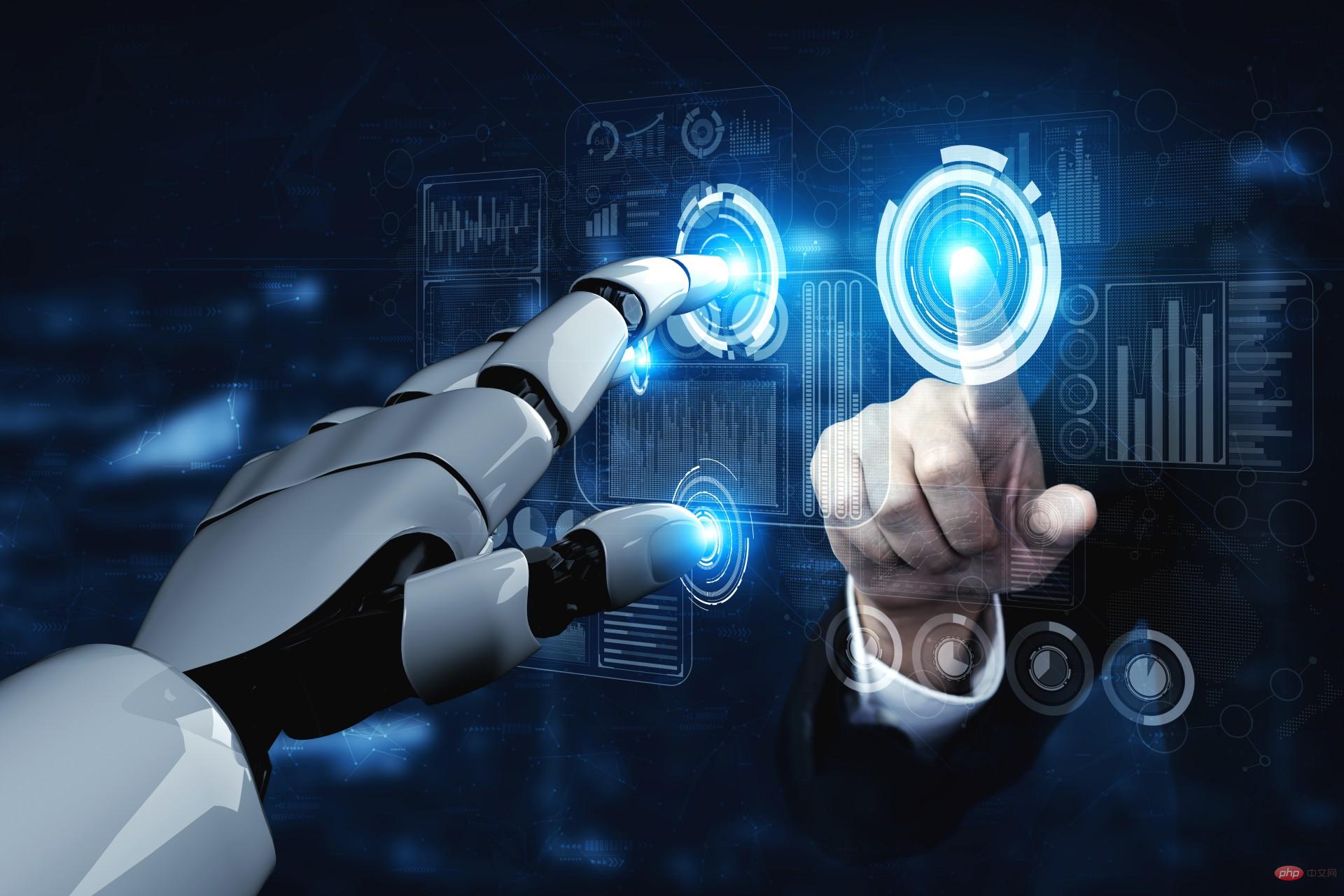
机器学习是一个不断发展的学科,一直在创造新的想法和技术。本文罗列了2023年机器学习的十大概念和技术。 本文罗列了2023年机器学习的十大概念和技术。2023年机器学习的十大概念和技术是一个教计算机从数据中学习的过程,无需明确的编程。机器学习是一个不断发展的学科,一直在创造新的想法和技术。为了保持领先,数据科学家应该关注其中一些网站,以跟上最新的发展。这将有助于了解机器学习中的技术如何在实践中使用,并为自己的业务或工作领域中的可能应用提供想法。2023年机器学习的十大概念和技术:1. 深度神经网
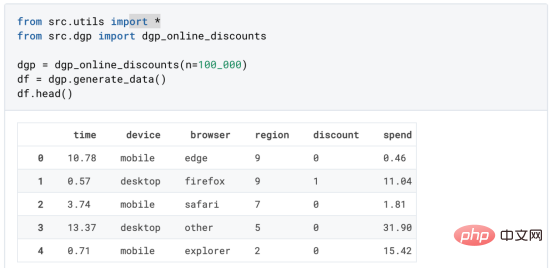
译者 | 朱先忠审校 | 孙淑娟在我之前的博客中,我们已经了解了如何使用因果树来评估政策的异质处理效应。如果你还没有阅读过,我建议你在阅读本文前先读一遍,因为我们在本文中认为你已经了解了此文中的部分与本文相关的内容。为什么是异质处理效应(HTE:heterogenous treatment effects)呢?首先,对异质处理效应的估计允许我们根据它们的预期结果(疾病、公司收入、客户满意度等)选择提供处理(药物、广告、产品等)的用户(患者、用户、客户等)。换句话说,估计HTE有助于我
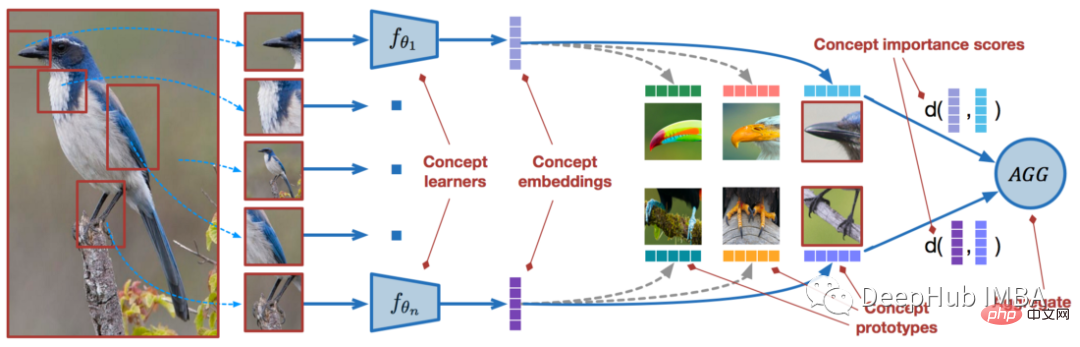
近年来,基于深度学习的模型在目标检测和图像识别等任务中表现出色。像ImageNet这样具有挑战性的图像分类数据集,包含1000种不同的对象分类,现在一些模型已经超过了人类水平上。但是这些模型依赖于监督训练流程,标记训练数据的可用性对它们有重大影响,并且模型能够检测到的类别也仅限于它们接受训练的类。由于在训练过程中没有足够的标记图像用于所有类,这些模型在现实环境中可能不太有用。并且我们希望的模型能够识别它在训练期间没有见到过的类,因为几乎不可能在所有潜在对象的图像上进行训练。我们将从几个样本中学习
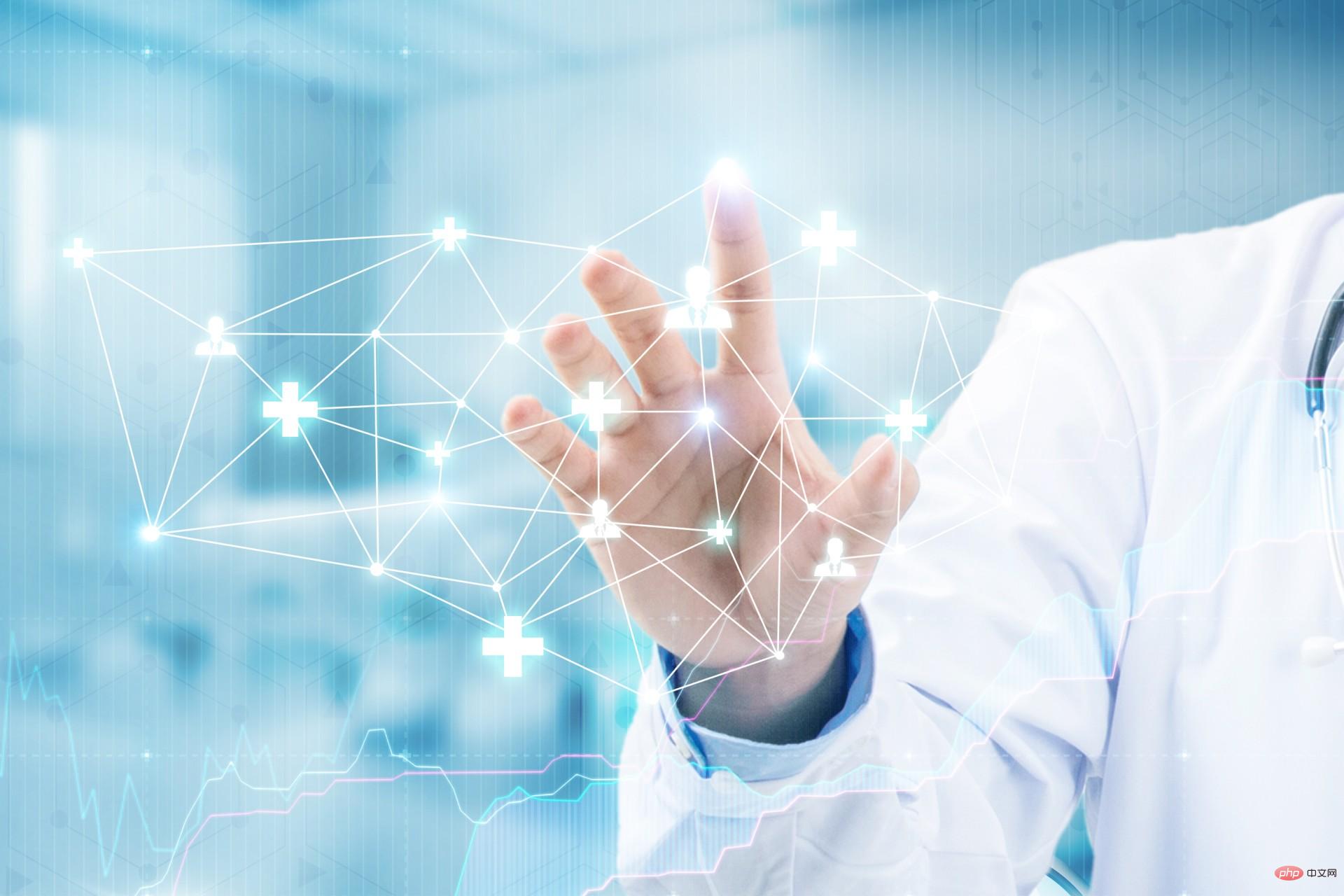
本文讨论使用LazyPredict来创建简单的ML模型。LazyPredict创建机器学习模型的特点是不需要大量的代码,同时在不修改参数的情况下进行多模型拟合,从而在众多模型中选出性能最佳的一个。 摘要本文讨论使用LazyPredict来创建简单的ML模型。LazyPredict创建机器学习模型的特点是不需要大量的代码,同时在不修改参数的情况下进行多模型拟合,从而在众多模型中选出性能最佳的一个。本文包括的内容如下:简介LazyPredict模块的安装在分类模型中实施LazyPredict
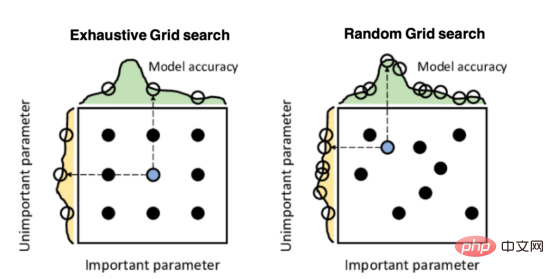
译者 | 朱先忠审校 | 孙淑娟引言模型超参数(或模型设置)的优化可能是训练机器学习算法中最重要的一步,因为它可以找到最小化模型损失函数的最佳参数。这一步对于构建不易过拟合的泛化模型也是必不可少的。优化模型超参数的最著名技术是穷举网格搜索和随机网格搜索。在第一种方法中,搜索空间被定义为跨越每个模型超参数的域的网格。通过在网格的每个点上训练模型来获得最优超参数。尽管网格搜索非常容易实现,但它在计算上变得昂贵,尤其是当要优化的变量数量很大时。另一方面,随机网格搜索是一种更快的优化方法,可以提供更好的

本文将详细介绍用来提高机器学习效果的最常见的超参数优化方法。 译者 | 朱先忠审校 | 孙淑娟简介通常,在尝试改进机器学习模型时,人们首先想到的解决方案是添加更多的训练数据。额外的数据通常是有帮助(在某些情况下除外)的,但生成高质量的数据可能非常昂贵。通过使用现有数据获得最佳模型性能,超参数优化可以节省我们的时间和资源。顾名思义,超参数优化是为机器学习模型确定最佳超参数组合以满足优化函数(即,给定研究中的数据集,最大化模型的性能)的过程。换句话说,每个模型都会提供多个有关选项的调整“按钮
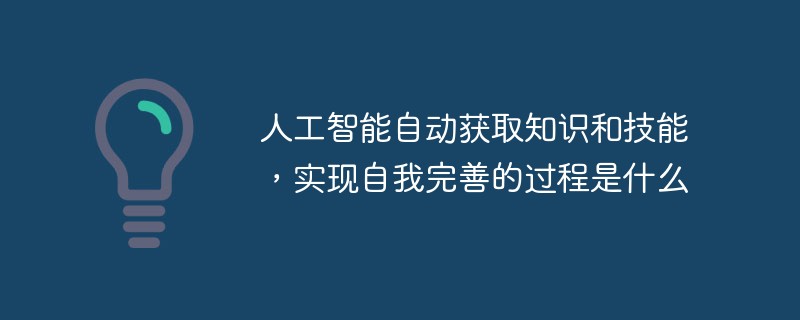
实现自我完善的过程是“机器学习”。机器学习是人工智能核心,是使计算机具有智能的根本途径;它使计算机能模拟人的学习行为,自动地通过学习来获取知识和技能,不断改善性能,实现自我完善。机器学习主要研究三方面问题:1、学习机理,人类获取知识、技能和抽象概念的天赋能力;2、学习方法,对生物学习机理进行简化的基础上,用计算的方法进行再现;3、学习系统,能够在一定程度上实现机器学习的系统。


Hot AI Tools

Undresser.AI Undress
AI-powered app for creating realistic nude photos

AI Clothes Remover
Online AI tool for removing clothes from photos.

Undress AI Tool
Undress images for free

Clothoff.io
AI clothes remover

AI Hentai Generator
Generate AI Hentai for free.

Hot Article

Hot Tools

SublimeText3 English version
Recommended: Win version, supports code prompts!

SAP NetWeaver Server Adapter for Eclipse
Integrate Eclipse with SAP NetWeaver application server.
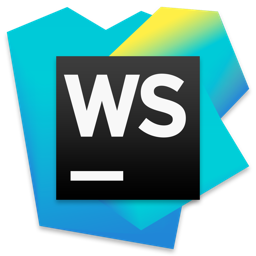
WebStorm Mac version
Useful JavaScript development tools

SublimeText3 Linux new version
SublimeText3 Linux latest version

MinGW - Minimalist GNU for Windows
This project is in the process of being migrated to osdn.net/projects/mingw, you can continue to follow us there. MinGW: A native Windows port of the GNU Compiler Collection (GCC), freely distributable import libraries and header files for building native Windows applications; includes extensions to the MSVC runtime to support C99 functionality. All MinGW software can run on 64-bit Windows platforms.
