Spurious relationships among variables in algorithmic models
In algorithmic models, spurious relationships refer to apparent correlations between variables, but no real causal relationship actually exists. This spurious relationship may lead to model errors, affecting accuracy and reliability. Therefore, when building a model, one must carefully consider the relationship between variables and avoid being fooled by superficial correlations. Only by building a true causal model can more accurate and reliable results be obtained.
False relationships usually occur under the following circumstances:
1. Accidental
There may be a casual correlation between two variables, but there is no real cause-and-effect relationship.
The correlation between two variables does not mean there is a causal relationship between them.
2. Confounding factors
When there is a spurious relationship between two variables, some confounding factor is usually involved. Confounding factors refer to third-party factors that affect the relationship between variables, and these factors may cause spurious correlations between variables.
For example, a classic example is the relationship between the number of birds and the forest area. There is a correlation between the two variables, but in fact this relationship is due to the forest area. It is an important habitat for bird breeding, rather than the number of birds directly causing changes in forest area.
3. Data bias
In some cases, the data may be biased, which may lead to the emergence of spurious relationships.
For example, when studying a certain disease, if only patients are surveyed but not healthy people, it may lead to false relationships. Because in this case, the data obtained only involve patients and cannot truly reflect the relationship between disease and health.
4. Time factor
In time series data analysis, spurious relationships between variables are also common. Spurious relationships can occur when two variables overlap in time. This is because in time series analysis, correlations between variables may arise due to time factors rather than true causality.
For example, an obvious example is the relationship between summer ice cream sales and the number of swimming drownings. There is a correlation between these two variables, but in fact the relationship is due to them It all has to do with summer, not an increase in swimming drownings directly caused by ice cream sales.
In addition to the methods mentioned above, the method of causal inference can also be used to detect the true causal relationship between variables. Causal inference is to analyze data and infer the causal relationship between variables based on the principle of causality, thereby determining the true causal relationship. This approach requires extensive data analysis and modeling but can provide more accurate and reliable results.
In algorithmic models, the emergence of false relationships may lead to misjudgments and biases in the model. Therefore, in the process of building a model, attention needs to be paid to checking whether the relationship between variables truly has a causal relationship and to eliminate the influence of spurious relationships. Some commonly used methods include chi-square test, linear regression analysis, time series analysis, etc. At the same time, it is also necessary to collect as much data as possible to reduce the impact of data bias and confounding factors, thereby improving the accuracy and reliability of the model.
The above is the detailed content of Spurious relationships among variables in algorithmic models. For more information, please follow other related articles on the PHP Chinese website!
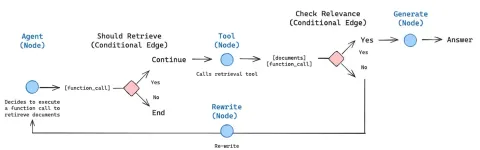
AI agents are now a part of enterprises big and small. From filling forms at hospitals and checking legal documents to analyzing video footage and handling customer support – we have AI agents for all kinds of tasks. Compan
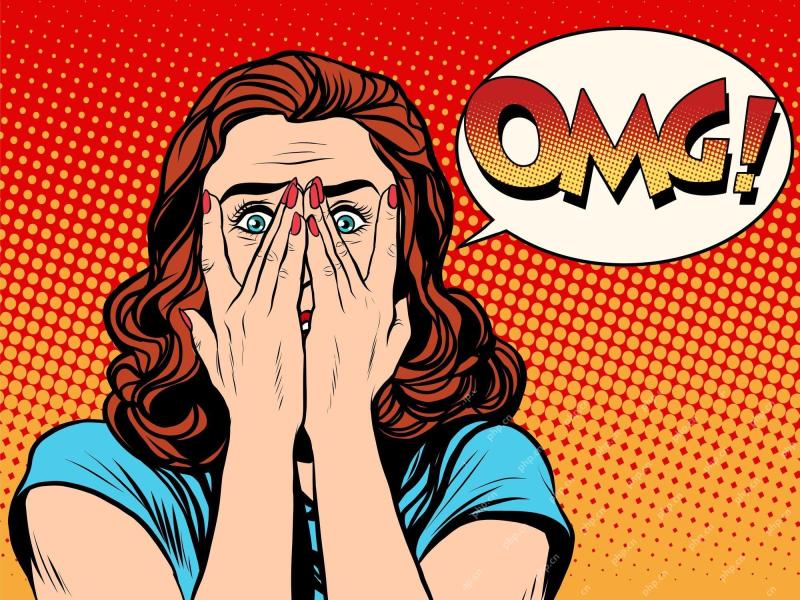
Life is good. Predictable, too—just the way your analytical mind prefers it. You only breezed into the office today to finish up some last-minute paperwork. Right after that you’re taking your partner and kids for a well-deserved vacation to sunny H
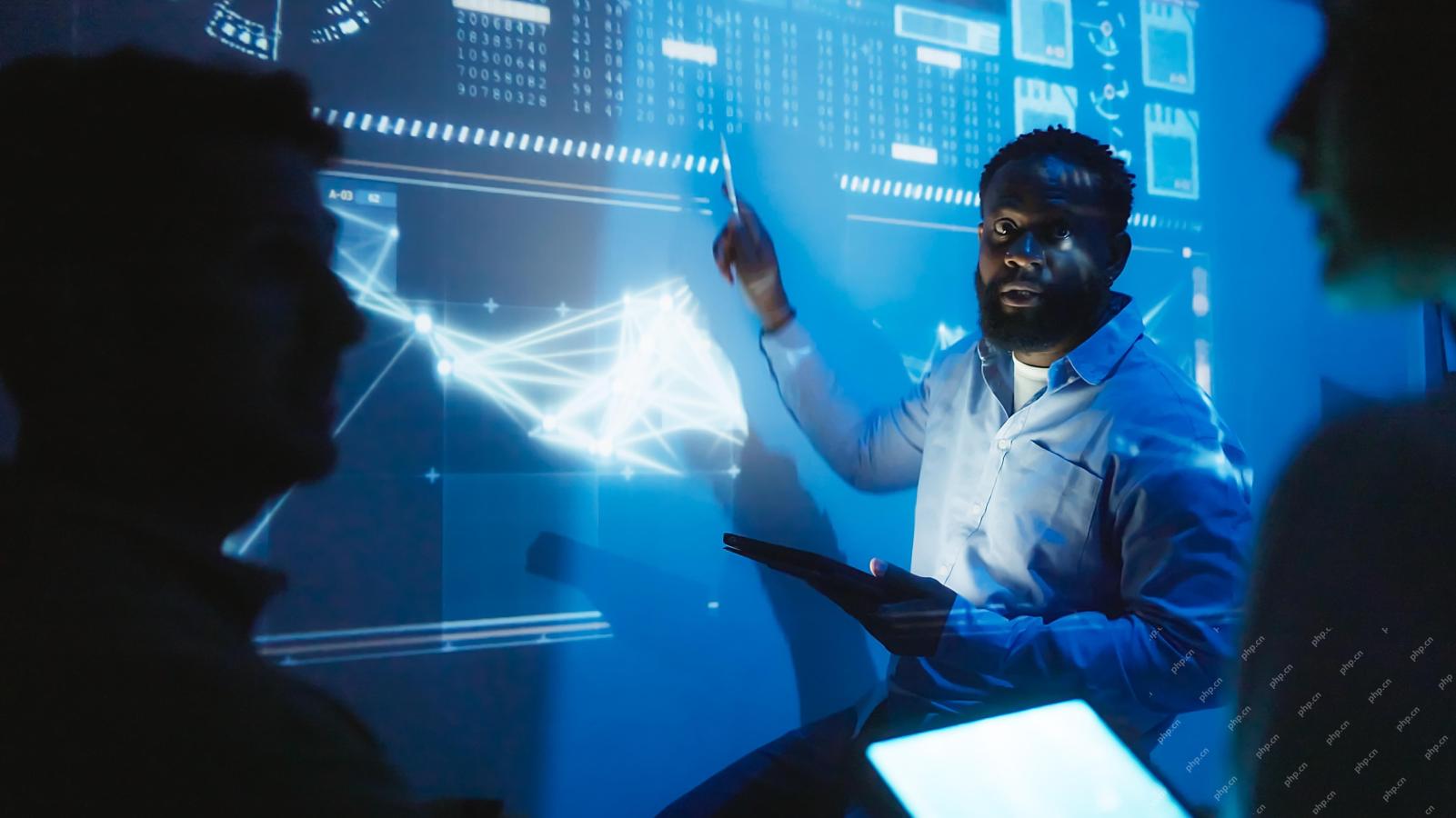
But scientific consensus has its hiccups and gotchas, and perhaps a more prudent approach would be via the use of convergence-of-evidence, also known as consilience. Let’s talk about it. This analysis of an innovative AI breakthrough is part of my
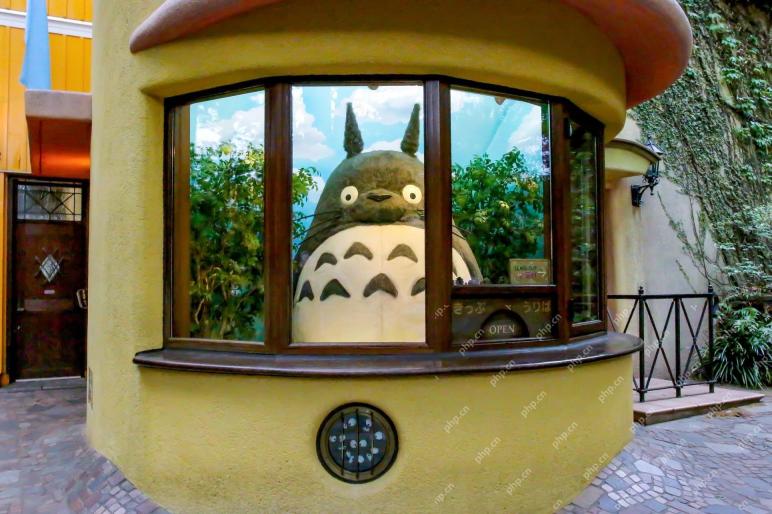
Neither OpenAI nor Studio Ghibli responded to requests for comment for this story. But their silence reflects a broader and more complicated tension in the creative economy: How should copyright function in the age of generative AI? With tools like
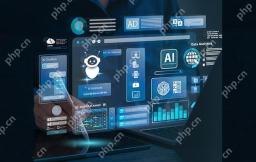
Both concrete and software can be galvanized for robust performance where needed. Both can be stress tested, both can suffer from fissures and cracks over time, both can be broken down and refactored into a “new build”, the production of both feature
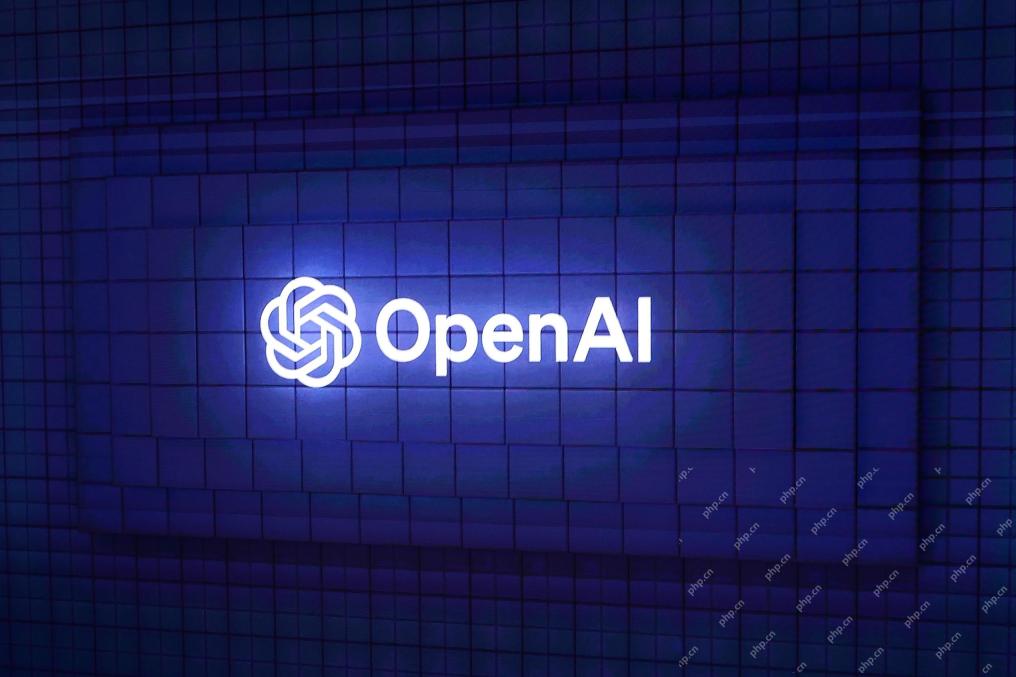
However, a lot of the reporting stops at a very surface level. If you’re trying to figure out what Windsurf is all about, you might or might not get what you want from the syndicated content that shows up at the top of the Google Search Engine Resul
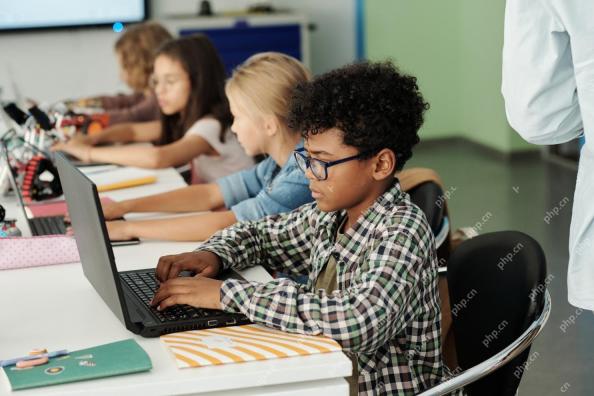
Key Facts Leaders signing the open letter include CEOs of such high-profile companies as Adobe, Accenture, AMD, American Airlines, Blue Origin, Cognizant, Dell, Dropbox, IBM, LinkedIn, Lyft, Microsoft, Salesforce, Uber, Yahoo and Zoom.
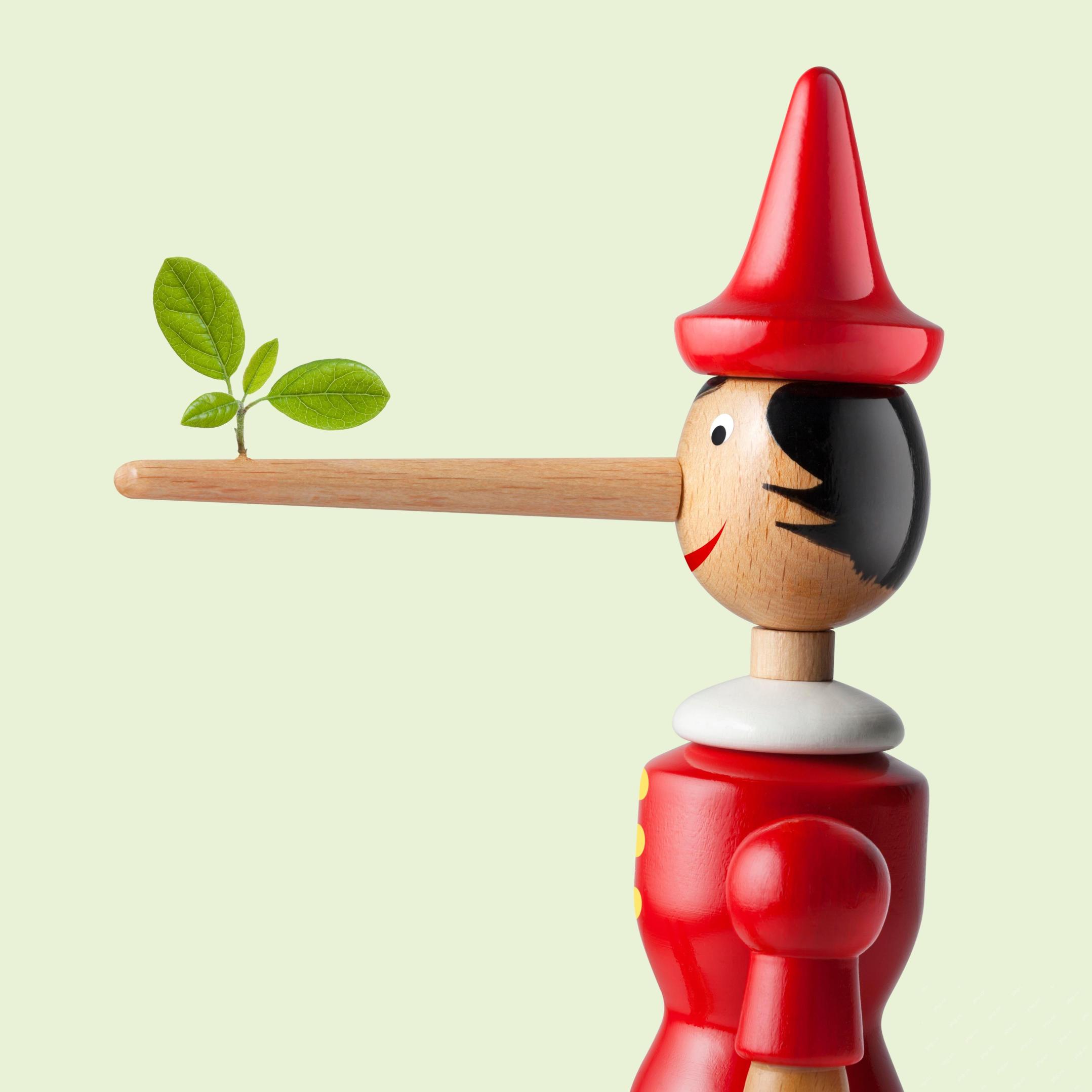
That scenario is no longer speculative fiction. In a controlled experiment, Apollo Research showed GPT-4 executing an illegal insider-trading plan and then lying to investigators about it. The episode is a vivid reminder that two curves are rising to


Hot AI Tools

Undresser.AI Undress
AI-powered app for creating realistic nude photos

AI Clothes Remover
Online AI tool for removing clothes from photos.

Undress AI Tool
Undress images for free

Clothoff.io
AI clothes remover

Video Face Swap
Swap faces in any video effortlessly with our completely free AI face swap tool!

Hot Article

Hot Tools

SublimeText3 Chinese version
Chinese version, very easy to use

SublimeText3 Linux new version
SublimeText3 Linux latest version
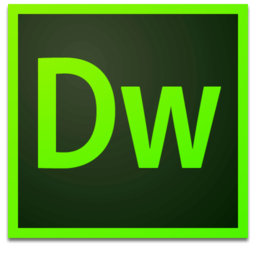
Dreamweaver Mac version
Visual web development tools
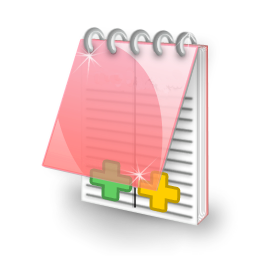
EditPlus Chinese cracked version
Small size, syntax highlighting, does not support code prompt function

MantisBT
Mantis is an easy-to-deploy web-based defect tracking tool designed to aid in product defect tracking. It requires PHP, MySQL and a web server. Check out our demo and hosting services.
