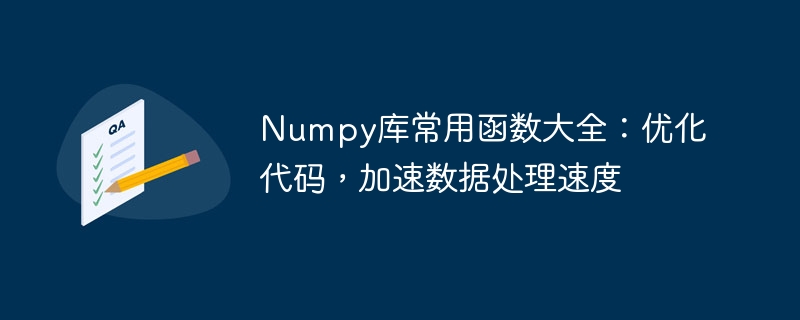
The Numpy library is an important scientific computing library in Python. It provides efficient multi-dimensional array objects and a rich function library, which can help us perform numerical calculations and data processing more efficiently. . This article will introduce a series of commonly used functions in the Numpy library and how to use these functions to optimize code and speed up data processing.
- Create Array
Our commonly used functions to create arrays are:
- np.array(): Convert input data into ndarray objects. You can specify the array by specifying dtype. type of data.
- np.zeros(): Creates an all-zero array of the specified shape.
- np.ones(): Create an all-1 array of the specified shape.
- np.arange(): Create an arithmetic array of the specified range.
- np.linspace(): Create an equally spaced array within the specified range.
- Array operation
Numpy provides many array operation functions, the following are some of the commonly used ones:
- np.reshape(): Change the shape of the array.
- np.concatenate(): Concatenate multiple arrays along the specified axis.
- np.split(): Split the array into multiple sub-arrays along the specified axis.
- np.transpose(): Swap the dimensions of the array.
- np.flatten(): Flatten a multi-dimensional array.
- np.resize(): Reshape the array according to the specified shape.
- Array calculation
Numpy provides a wealth of mathematical functions that can perform various operations on arrays:
- np.add(): Array addition.
- np.subtract(): Array subtraction.
- np.multiply(): Multiply arrays.
- np.divide(): Array division.
- np.exp(): Calculate the index of the array.
- np.sin(), np.cos(), np.tan(): Calculate trigonometric function values.
- Array Statistics
Numpy also provides some functions for statistical analysis, such as:
- np.mean(): Calculate the average of an array.
- np.median(): Calculate the median of the array.
- np.std(): Calculate the standard deviation of the array.
- np.min(), np.max(): Calculate the minimum and maximum values of the array respectively.
- np.sum(): Calculate the sum of all elements of the array.
- np.unique(): Find the unique value in the array.
- Array sorting
The sorting function in Numpy can help us sort the array:
- np.sort(): Sort the array.
- np.argsort(): Returns the sorted index of the array.
- np.argmax(), np.argmin(): Return the index of the maximum value and minimum value of the array respectively.
- np.partition(): Divide the array into two parts at the specified position.
- Data processing
In data processing, the Numpy library also provides many functions to help us quickly perform some common operations:
- np.loadtxt(): Loading from a text file data.
- np.savetxt(): Save data to a text file.
- np.genfromtxt(): Generate an array from a text file.
- np.where(): Return elements that meet the conditions according to the specified conditions.
- np.clip(): Limit the elements in the array to the specified range.
By rationally using the functions provided by the Numpy library, we can greatly optimize the code and improve the data processing speed. Below is a simple example to illustrate.
import numpy as np
# 生成一个100万个元素的随机数组
arr = np.random.rand(1000000)
# 使用Numpy库计算数组的平均值
mean = np.mean(arr)
print("数组平均值:", mean)
# 使用普通的Python循环计算数组的平均值
total = 0
for num in arr:
total += num
mean = total / len(arr)
print("数组平均值:", mean)
In the above example, we used the np.mean() function in the Numpy library to calculate the average of the array and compared it with the ordinary Python loop calculation method. Through comparison, it can be found that the calculation speed using the Numpy library is faster, especially when facing large-scale data, the gap is particularly obvious. Therefore, rational use of functions in the Numpy library can effectively improve code execution efficiency.
In short, the Numpy library provides a wealth of functions and tools that can help us perform numerical calculations and data processing more efficiently. By applying these functions appropriately, we can optimize the code and speed up data processing. I hope the commonly used functions listed in this article will be helpful to everyone.
The above is the detailed content of Comprehensive list of commonly used functions in the Numpy library: optimize code and speed up data processing. For more information, please follow other related articles on the PHP Chinese website!
Statement:The content of this article is voluntarily contributed by netizens, and the copyright belongs to the original author. This site does not assume corresponding legal responsibility. If you find any content suspected of plagiarism or infringement, please contact admin@php.cn