How to find interesting clips from a video? Temporal Action Localization (TAL) is a common method.
After using video content for modeling, you can freely search within the entire video.
The joint team of Huazhong University of Science and Technology and the University of Michigan has recently brought new progress to this technology-
In the past, modeling in TAL was at the fragment or even instance level; Now only one frame of
in the video can achieve , and the effect is comparable to that of full supervision.
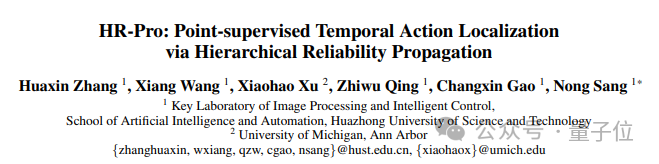
A team from Huazhong University of Science and Technology proposed a new framework called HR-Pro for point-labeled supervised temporal behavior detection.
Through multi-level reliability propagation, HR-Pro can learn more discriminative fragment-level features and more reliable instance-level boundaries online.
HR-Pro consists of two reliability-aware stages, which can effectively propagate high-confidence cues from segment-level and instance-level point annotations, allowing the network to learn more discriminative Fragment representation and more reliable proposals.
Experiments on multiple benchmark datasets show that HR-Pro outperforms existing methods with state-of-the-art results, demonstrating its effectiveness and potential for point annotation.
Performance comparable to full supervision method
The following figure shows the performance comparison of HR-Pro and LACP in temporal behavior detection on the THUMOS14 test video.
HR-Pro demonstrates more accurate action instance detection, specifically:
For the "golf swing" behavior, HR-Pro effectively distinguishes between behavior and context Segments, alleviating LACP's intractable False Positive predictions;- For the discus throwing behavior, HR-Pro detects more complete segments than LACP, which has lower activation values on non-discriminative action segments .
-
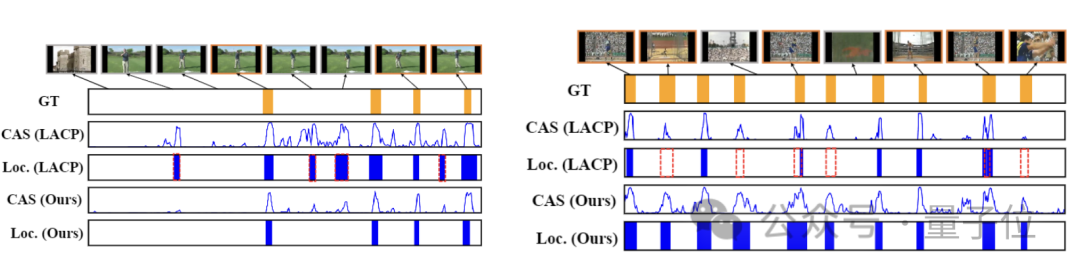
#The test results on the data set also confirm this intuitive feeling.
After visualizing the detection results on the THUMOS14 dataset, it can be observed that after instance-level integrity learning, the difference between high-quality predictions and low-quality predictions increases significantly.
(The left side is the result before instance-level integrity learning, and the right side is the result after learning. The horizontal and vertical axes represent time and reliability scores respectively.)
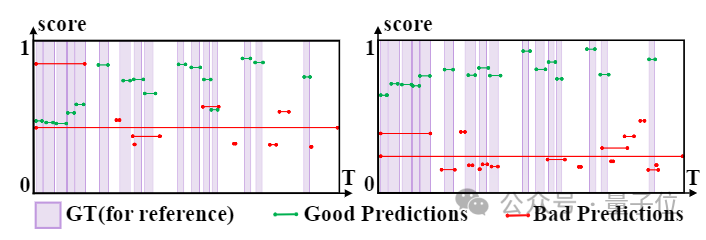
Overall, in the four commonly used data sets, the performance of HR-Pro greatly surpasses the state-of-the-art point supervision method, and the average mAP on the THUMOS14 data set reaches 60.3%. Compared with the previous SoTA method (53.7%), the improvement is 6.5%, and it can achieve comparable results with some fully supervised methods.
Compared to the previous state-of-the-art methods in the table below on the THUMOS14 test set, HR-Pro achieves an average mAP of 60.3% for IoU thresholds between 0.1 and 0.7, which is better than the previous state-of-the-art method CRRC- Net is 6.5% higher.
And HR-Pro is able to achieve comparable performance with competitive fully supervised methods, such as AFSD (average mAP is 51.1% vs. 52.0% for IoU thresholds between 0.3 and 0.7).
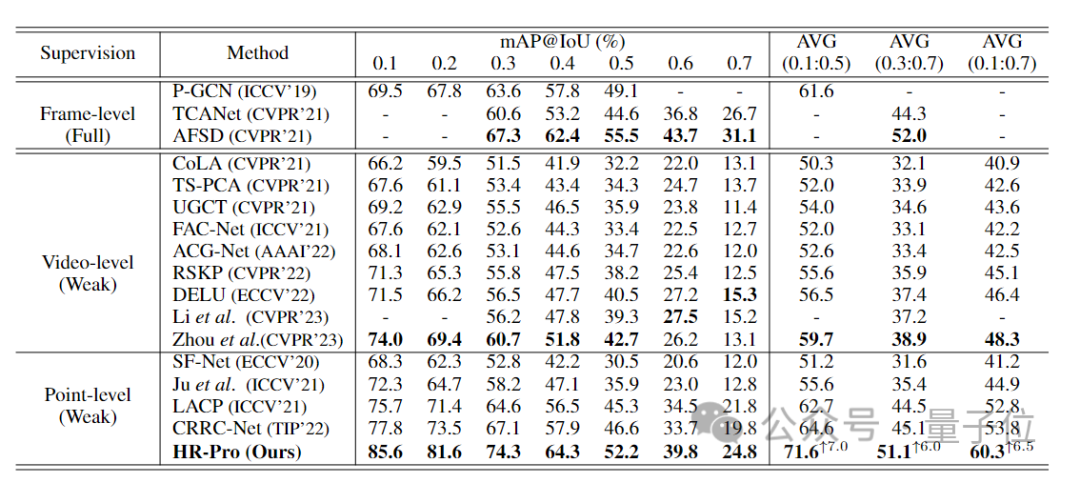
△Comparison of HR-Pro and the former SOTA method on the THUMOS14 data set
In terms of versatility and superiority on various benchmark data sets , HR-Pro also significantly outperforms existing methods, achieving improvements of 3.8%, 7.6% and 2.0% on GTEA, BEOID and ActivityNet 1.3 respectively.

△Comparison between HR-Pro and the former SOTA method on GTEA and other data sets
So, how is HR-Pro implemented?
Learning is carried out in two stages
The research team proposed a multi-level reliable propagation method, introducing a reliable fragment memory module at the fragment level and using the cross-attention method to propagate to other fragments, at the instance level Proposal generation based on point supervision is proposed to associate fragments and instances to generate proposals with different reliability, and further optimize the confidence and boundaries of proposals at the instance level.
The model structure of HR-Pro is shown in the figure below: Temporal behavior detection is divided into a two-stage learning process, namely
fragment-level discriminative learningandinstance-level complete Sexual learning.
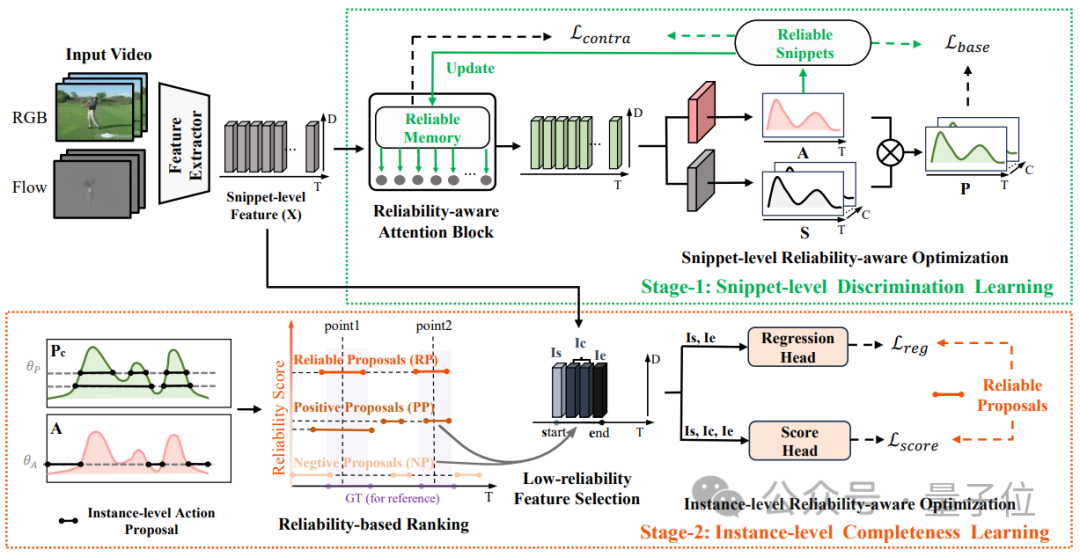
Phase 1: Segment-level discriminative learning
The research team introduces reliability-aware segment-level discriminative learning, proposes to store reliable prototypes for each category, and uses them within the video to and video-to-video methods to propagate high-confidence cues from these prototypes to other clips.
Fragment-level reliable prototype construction
In order to build reliable prototypes at the fragment level, the team created an online updated prototype memory to store reliable prototypes of various behaviors mc (where c = 1, 2, …, C) in order to be able to utilize the feature information of the entire data set.
The research team chose to initialize the prototype with point-labeled segment features:
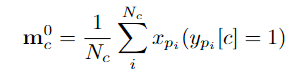
Next, the researchers used pseudo-labeled behavioral segment features to update each Category prototypes are specifically expressed as follows:

Fragment-level reliability awareness optimization
In order to combine the feature information of fragment-level reliable prototypes Passed to other fragments, the research team designed a Reliabilty-aware Attention Block (RAB) to inject reliable information from the prototype into other fragments through cross-attention, thereby enhancing the robustness of fragment features and Increased focus on less discriminative segments.
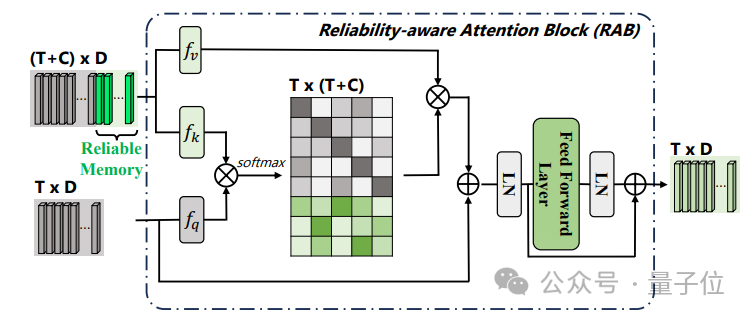
In order to learn more discriminative fragment features, the team also constructed a reliability-aware fragment comparison loss:
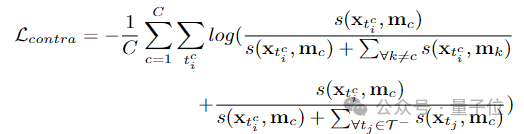
Phase 2: Instance-level integrity learning
In order to fully explore the temporal structure of instance-level behaviors and optimize the proposed score ranking, the team introduced instance-level action integrity learning.
This approach aims to refine the confidence scores and bounds of proposals through instance-level feature learning, guided by reliable instance prototypes.
Instance-level reliable prototype construction
In order to utilize the instance-level prior information of point annotation during the training process, the team proposed a proposal generation method based on point annotation Used to generate proposals with different Reliabilities.
According to their reliability scores and relative point annotated timing positions, these proposals can be divided into two types:
- ##Reliable Proposals (Reliable Proposals, RP ): For each point in each category, the proposal contains this point and has the highest reliability;
- Positive Proposals (Positive Proposals, PP): All the rest Candidate Proposal.
To ensure a balanced number of positive and negative samples, the research team grouped those clips with category-independent attention scores lower than a predefined value into Negative Proposals (NP).
Instance-level reliability-aware optimization
To predict the completeness score of each proposal, the research team inputs the proposal features of sensitive boundaries into the score prediction head φs:

Then use the IoU of positive/negative sample proposals and reliable proposals as a guide to supervise the completeness score prediction of the proposal:

In order to obtain a more accurate boundary behavior proposal, the researcher inputs the start area features and end area features of the proposal in each PP into the regression prediction head φr to predict the offset of the proposal start and end time.
Further calculate the refined proposals, and hope that the refined proposals will coincide with the reliable proposals.


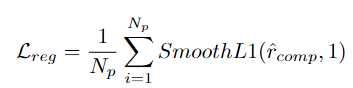
# In short, HR-Pro can achieve great results with only a few annotations. It reduces the cost of obtaining tags and at the same time has strong generalization capabilities, providing favorable conditions for actual deployment applications.
According to this, the author predicts that HR-Pro will have broad application prospects in behavioral analysis, human-computer interaction, driving analysis and other fields.
Paper address: https://arxiv.org/abs/2308.12608
The above is the detailed content of Segment features can be learned by labeling a single frame of video, achieving fully supervised performance! Huake wins new SOTA for sequential behavior detection. For more information, please follow other related articles on the PHP Chinese website!