


Alibaba's new mPLUG-Owl upgrade has the best of both worlds, and modal collaboration enables MLLM's new SOTA
OpenAI GPT-4V and Google Gemini have demonstrated very strong multi-modal understanding capabilities, promoting the rapid development of multi-modal large models (MLLM), and MLLM has become The hottest research direction in the industry right now.
MLLM achieves excellent instruction following ability in a variety of visual-linguistic open tasks. Although previous research on multimodal learning has shown that different modalities can collaborate and promote each other, existing MLLM research mainly focuses on improving the ability of multimodal tasks and how to balance the benefits of modal collaboration and the impact of modal interference. remains an important issue that needs to be addressed.
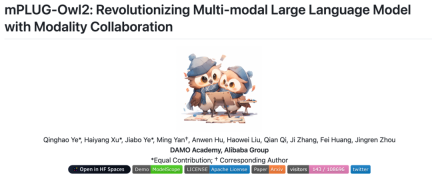
Please click the following link to view the paper: https://arxiv.org/pdf/2311.04257.pdf
-
Please check the following code address: https://github.com/X-PLUG/mPLUG-Owl/tree/main/mPLUG-Owl2
ModelScope experience address: https: //modelscope.cn/studios/damo/mPLUG-Owl2/summary
-
HuggingFace experience address link: https://huggingface.co/spaces/MAGAer13/mPLUG-Owl2
In response to this problem, Alibaba’s multi-modal large model mPLUG-Owl has received a major upgrade. Through modal collaboration, it simultaneously improves the performance of plain text and multi-modality, surpassing LLaVA1.5, MiniGPT4, Qwen-VL and other models, and achieves the best performance in a variety of tasks. Specifically, mPLUG-Owl2 utilizes shared functional modules to promote collaboration between different modalities and introduces a modal adaptation module to retain the characteristics of each modality. With a simple and effective design, mPLUG-Owl2 achieves the best performance in multiple fields including plain text and multi-modal tasks. The study of modal collaboration phenomena also provides inspiration for the future development of multi-modal large models
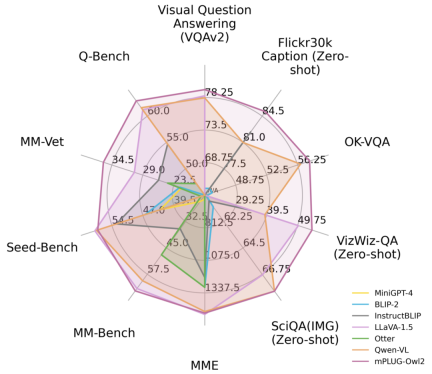
Figure 1 Performance comparison with existing MLLM models
Method introduction In order to achieve the purpose of not changing the original meaning, the content needs to be rewritten into Chinese
mPLUG-Owl2 model mainly consists of three parts:
Visual Encoder: As a visual encoder, ViT-L/14 converts the input image with a resolution of H x W into a sequence of visual tokens of H/14 x W/14 and inputs it into the Visual Abstractor.
Visual Extractor: Extract high-level semantic features by learning a set of available queries while reducing the visual sequence length of the input language model
Language model: LLaMA-2-7B is used as the text decoder, and the modal adaptation module shown in Figure 3 is designed.
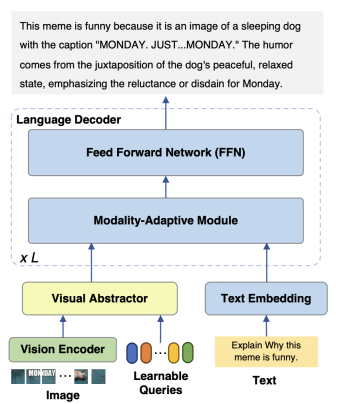
##Figure 2 mPLUG-Owl2 model structure
In order to align the visual and For language modality, existing work usually maps visual features into the semantic space of text. However, this approach ignores the respective characteristics of visual and text information and may affect the performance of the model due to the mismatch of semantic granularity. To solve this problem, this paper proposes a modality-adaptive module (MAM) to map visual and textual features into a shared semantic space, while decoupling visual-linguistic representations to retain the unique properties of each modality. .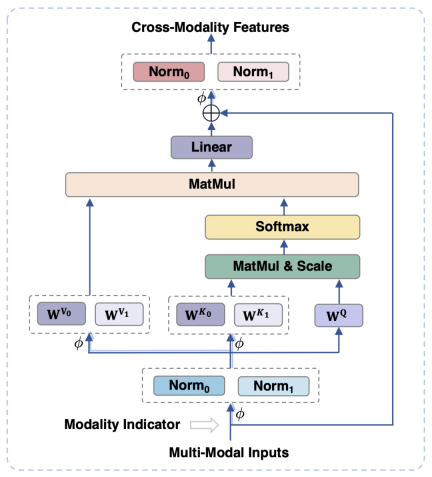
Figure 3 shows the schematic diagram of the modal adaptation module
shown in Figure 3 Yes, compared with the traditional Transformer, the main design of the modal adaptation module is:- In the input and output stages of the module, LayerNorm operations are performed on the visual and language modalities respectively. to adapt to the respective feature distributions of the two modes.
- In the self-attention operation, separate key and value projection matrices are used for visual and language modalities, but a shared query projection matrix is used to decouple the key and value projections. The matrix can avoid interference between the two modalities when the semantic granularity does not match.
- By sharing the same FFN, the two modalities can promote collaboration between each other
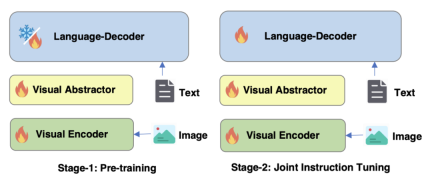
Optimize the training strategy of mPLUG-Owl2 in Figure 4
As shown in Figure 4, the training of mPLUG-Owl2 includes two stages: pre-training and instruction fine-tuning. The pre-training stage is mainly to achieve the alignment of the visual encoder and the language model. At this stage, the Visual Encoder and Visual Abstractor are trainable, and in the language model, only the visual-related model weights added by the Modality Adaptive Module are processed. renew. In the instruction fine-tuning stage, all parameters of the model are fine-tuned based on text and multi-modal instruction data (as shown in Figure 5) to improve the model's instruction following ability.
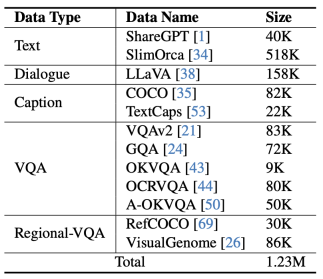
Figure 5 Instruction fine-tuning data used by mPLUG-Owl2
Experiment and results
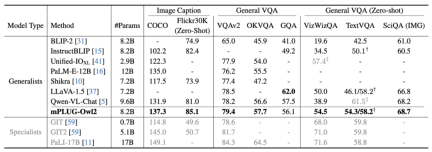
Figure 6 Image description and VQA task performance
Figure 7 MLLM benchmark performance
As shown in Figure 6 and Figure 7, whether it is traditional image description, VQA and other visual-language tasks, or MMBench, Q-Bench, etc. On benchmark data sets for multi-modal large models, mPLUG-Owl2 has achieved better performance than existing work.
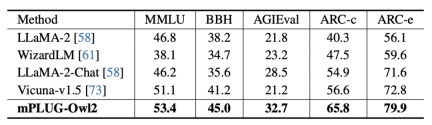
Figure 8 Plain text benchmark performance
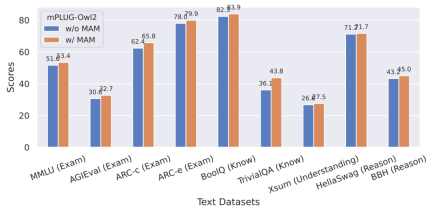
##Figure 9 The impact of modal adaptation module on the performance of plain text tasks
In addition, in order to evaluate the impact of modal collaboration on plain text tasks, the author also tested mPLUG -Owl2 performance in natural language understanding and generation. As shown in Figure 8, mPLUG-Owl2 achieves better performance compared to other instruction-fine-tuned LLMs. Figure 9 shows the performance on the plain text task. It can be seen that since the modal adaptation module promotes modal collaboration, the model's examination and knowledge capabilities have been significantly improved. The author analyzes that this is because multi-modal collaboration enables the model to use visual information to understand concepts that are difficult to describe in language, and enhances the model's reasoning ability through the rich information in the image, and indirectly strengthens the reasoning ability of the text.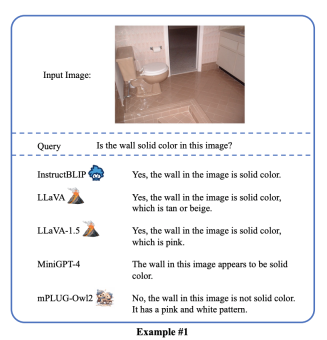
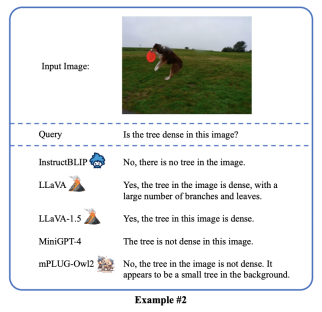
The above is the detailed content of Alibaba's new mPLUG-Owl upgrade has the best of both worlds, and modal collaboration enables MLLM's new SOTA. For more information, please follow other related articles on the PHP Chinese website!
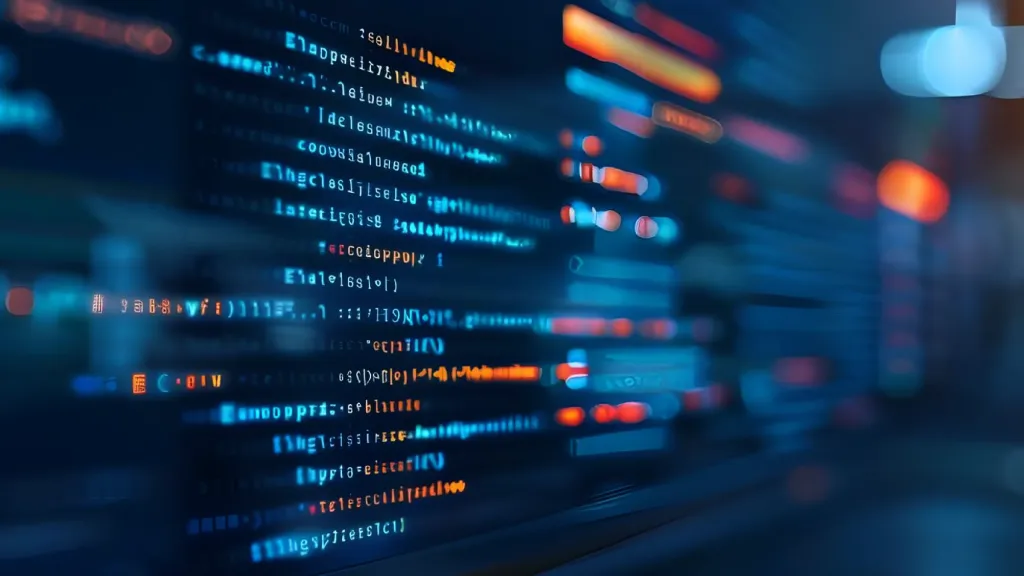
Running large language models at home with ease: LM Studio User Guide In recent years, advances in software and hardware have made it possible to run large language models (LLMs) on personal computers. LM Studio is an excellent tool to make this process easy and convenient. This article will dive into how to run LLM locally using LM Studio, covering key steps, potential challenges, and the benefits of having LLM locally. Whether you are a tech enthusiast or are curious about the latest AI technologies, this guide will provide valuable insights and practical tips. Let's get started! Overview Understand the basic requirements for running LLM locally. Set up LM Studi on your computer
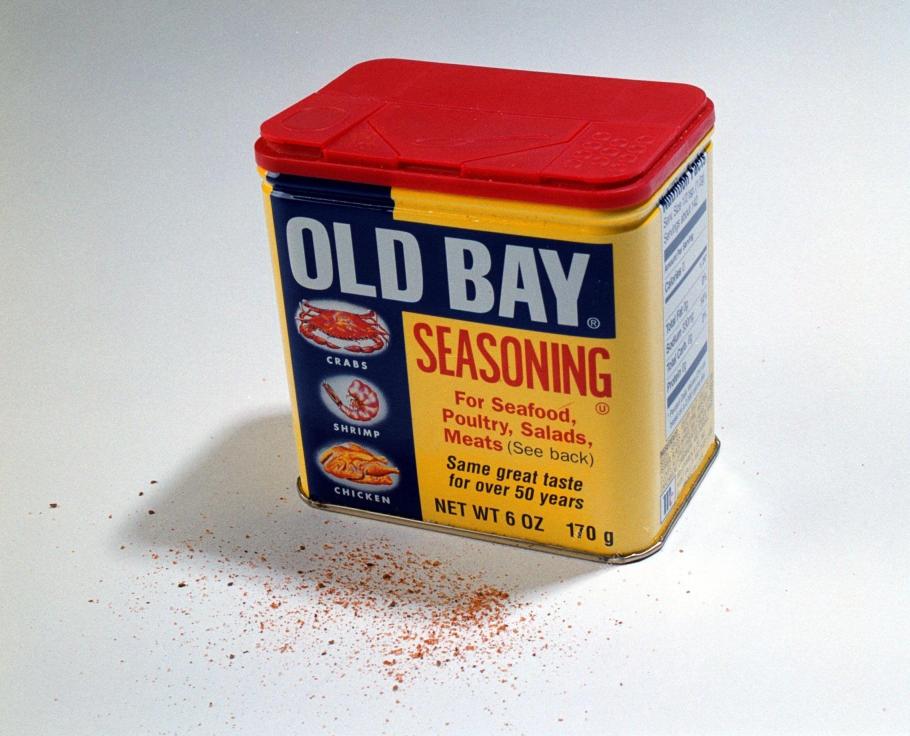
Guy Peri is McCormick’s Chief Information and Digital Officer. Though only seven months into his role, Peri is rapidly advancing a comprehensive transformation of the company’s digital capabilities. His career-long focus on data and analytics informs
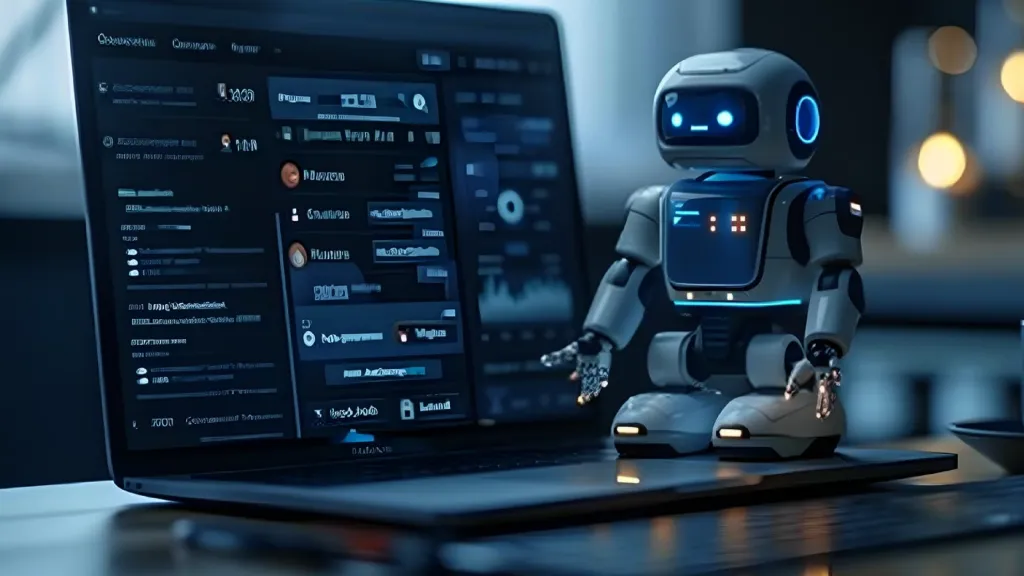
Introduction Artificial intelligence (AI) is evolving to understand not just words, but also emotions, responding with a human touch. This sophisticated interaction is crucial in the rapidly advancing field of AI and natural language processing. Th
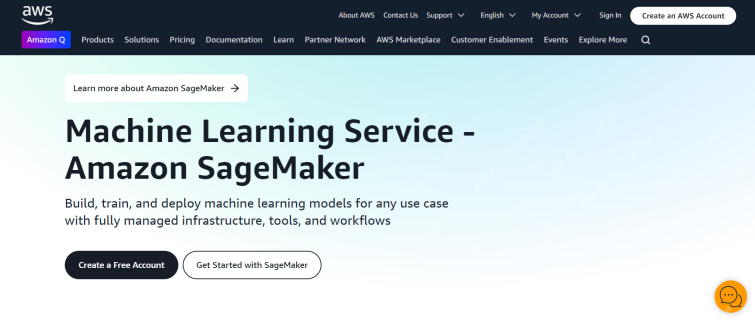
Introduction In today's data-centric world, leveraging advanced AI technologies is crucial for businesses seeking a competitive edge and enhanced efficiency. A range of powerful tools empowers data scientists, analysts, and developers to build, depl
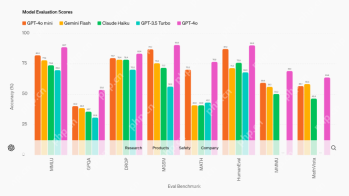
This week's AI landscape exploded with groundbreaking releases from industry giants like OpenAI, Mistral AI, NVIDIA, DeepSeek, and Hugging Face. These new models promise increased power, affordability, and accessibility, fueled by advancements in tr
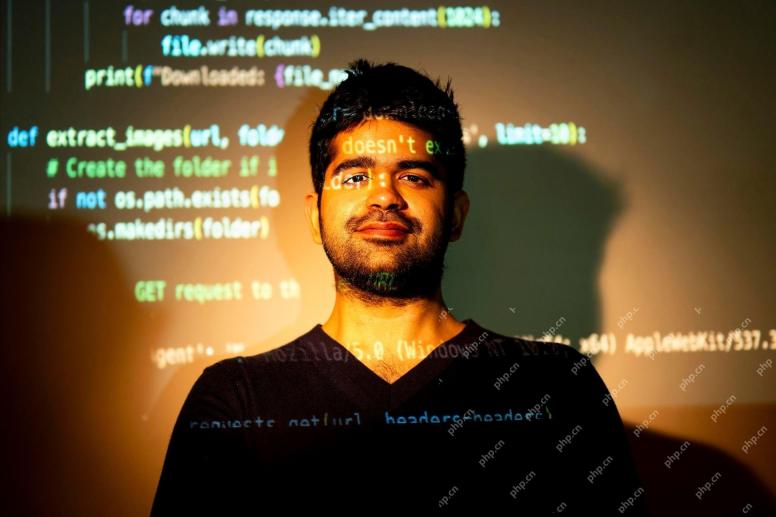
But the company’s Android app, which offers not only search capabilities but also acts as an AI assistant, is riddled with a host of security issues that could expose its users to data theft, account takeovers and impersonation attacks from malicious
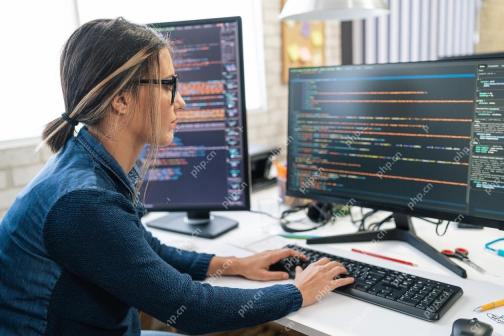
You can look at what’s happening in conferences and at trade shows. You can ask engineers what they’re doing, or consult with a CEO. Everywhere you look, things are changing at breakneck speed. Engineers, and Non-Engineers What’s the difference be
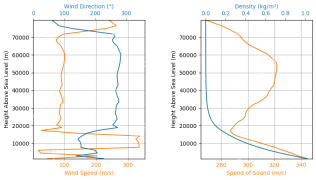
Simulate Rocket Launches with RocketPy: A Comprehensive Guide This article guides you through simulating high-power rocket launches using RocketPy, a powerful Python library. We'll cover everything from defining rocket components to analyzing simula


Hot AI Tools

Undresser.AI Undress
AI-powered app for creating realistic nude photos

AI Clothes Remover
Online AI tool for removing clothes from photos.

Undress AI Tool
Undress images for free

Clothoff.io
AI clothes remover

Video Face Swap
Swap faces in any video effortlessly with our completely free AI face swap tool!

Hot Article

Hot Tools

Atom editor mac version download
The most popular open source editor

SublimeText3 Linux new version
SublimeText3 Linux latest version
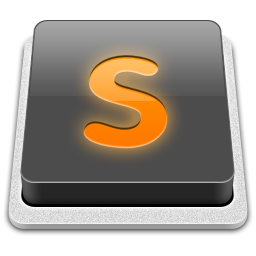
SublimeText3 Mac version
God-level code editing software (SublimeText3)

SublimeText3 English version
Recommended: Win version, supports code prompts!

SAP NetWeaver Server Adapter for Eclipse
Integrate Eclipse with SAP NetWeaver application server.