


With just one sentence of description, you can locate the corresponding clip in a large video!
For example, describing "a person drinking water while going down the stairs", by matching the video image and the footsteps, the new method can immediately find out the corresponding start and end timestamps:
Even "laughing", which is semantically difficult to understand, can be accurately located:
The method is called Adaptive dual branch Promotion Network (ADPN), proposed by the Tsinghua University research team.
Specifically, ADPN is used to complete a visual-linguistic cross-modal task called video clip positioning (Temporal Sentence Grounding, TSG) , that is, based on the query text, from the video Locate relevant segments.
ADPN is characterized by its ability to efficiently utilize the consistency and complementarity of visual and audio modalities in the video to enhance video clip positioning performance.
Compared with other TSG work PMI-LOC and UMT that use audio, the ADPN method has achieved more significant performance improvements from the audio mode, and has won new SOTA in multiple tests.
This work has been accepted by ACM Multimedia 2023 and is completely open source.
Let’s take a look at what ADPN is~
Positioning video clips in one sentence
Positioning of video clips(Temporal Sentence Grounding (TSG) is an important visual-linguistic cross-modal task.
Its purpose is to find the start and end timestamps of segments that semantically match it in an unedited video based on natural language queries. It requires the method to have strong temporal cross-modal reasoning capabilities.
However, most existing TSG methods only consider visual information in the video, such as RGB, optical flows, depth(depth) etc., while ignoring the audio information naturally accompanying the video.
Audio information often contains rich semantics and is consistent and complementary with visual information. As shown in the figure below, these properties will help the TSG task.(a) Consistency: The video image and footsteps consistently match "walking down the stairs" in the query Semantics; (b) Complementarity: It is difficult to identify specific behaviors in video images to locate the semantics of "laughing" in the query, but the appearance of laughter provides a strong complementary positioning clue.
Therefore, researchers have deeply studied the audio-enhanced video clip positioning task(Audio-enhanced Temporal Sentence Grounding, ATSG) , aiming to better combine visual and audio Capture positioning clues in the modal, however, the introduction of audio modal also brings the following challenges:
- The consistency and complementarity of audio and visual modalities are associated with the query text, so capturing Audio-visual consistency and complementarity require modeling of the three-modal interaction of text-visual-audio.
- There are significant modal differences between audio and vision. The information density and noise intensity of the two are different, which will affect the performance of audio-visual learning.
Adaptive Dual-branch Prompted Network"(Adaptive Dual-branch Prompted Network, ADPN ).
Through a dual-branch model structure design, this method can adaptively model the consistency and complementarity between audio and vision, and further eliminate the noise using a denoising optimization strategy based on course learning. The interference of audio modal noise reveals the importance of audio signals for video retrieval. The overall structure of ADPN is shown in the figure below:1. Dual-branch network structure design
Considering that the noise of audio is more obvious, and for TSG tasks, audio is usually There is more redundant information, so the learning process of audio and visual modalities needs to be given different importance. Therefore, this article involves a dual-branch network structure that uses audio and vision for multi-modal learning while also dealing with visual information. Be strengthened.Specifically, referring to Figure 2(a), ADPN simultaneously trains a branch (visual branch) that uses only visual information and a branch that uses both visual information and audio information (joint branch ).
The two branches have similar structures, in which the joint branch adds a text-guided clue mining unit(TGCM) Modeling text-visual-audio modal interaction. During the training process, the two branches update parameters simultaneously, and the inference phase uses the result of the joint branch as the model prediction result.
2. Text-Guided Clues Mining Unit(Text-Guided Clues Miner, TGCM)
Considering the consistency of audio and visual modalities Sex and complementarity are conditioned on a given text query, so the researchers designed a TGCM unit to model the interaction between the three modalities of text-visual-audio. Referring to Figure 2(b), TGCM is divided into two steps: "extraction" and "propagation". First, text is used as the query condition, and the associated information is extracted and integrated from the visual and audio modalities; then the visual and audio modalities are used as the query condition, and the integrated information is spread through attention to the respective modes of vision and audio, and finally feature fusion through FFN.3. Course learning optimization strategy
The researchers observed that the audio contained noise, which would affect the effect of multi-modal learning, so they used the intensity of the noise as For reference of sample difficulty, Curriculum Learning (CL)is introduced to denoise the optimization process, refer to Figure 2(c). They evaluate the difficulty of the sample based on the difference in the predicted output of the two branches. They believe that a sample that is too difficult has a high probability that its audio contains too much noise and is not suitable for the TSG task, so they evaluate the difficulty of the sample based on the score. The loss function terms of the training process are reweighted to discard bad gradients caused by noise in the audio.
(Please refer to the original text for the rest of the model structure and training details.)Multiple tests of new SOTA
Benchmark data of researchers on the TSG task Experimental evaluation was conducted on Charades-STA and ActivityNet Captions. The comparison with the baseline method is shown in Table 1.
The ADPN method can achieve SOTA performance; in particular, compared to other TSG work PMI-LOC and UMT that utilize audio, the ADPN method obtains more significant performance improvements from the audio mode, indicating that the ADPN method utilizes audio Modality promotes the superiority of TSG.
△Table 1: Experimental results on Charades-STA and ActivityNet Captions
The researchers further demonstrated the effectiveness of different design units in ADPN through ablation experiments sex, as shown in Table 2.
△Table 2: Ablation experiment on Charades-STA
The researchers selected the prediction results of some samples to visualize, and drew the TGCM " Extract the "text to vision" (T→V) and "text to audio" (T→A) attention weight distribution in the "step," as shown in Figure 3.
It can be observed that the introduction of audio modality improves the prediction results. From the case of "Person laughs at it", we can see that the attention weight distribution of T→A is closer to the Ground Truth, which corrects the misguided guidance of the model prediction by the weight distribution of T→V.
△Figure 3: Case display
In general, the researchers in this article proposed a novel adaptive dual-branch promotion network
(ADPN)to solve the problem of audio enhanced video clip positioning (ATSG) . They designed a dual-branch model structure to jointly train the visual branch and the audiovisual joint branch to resolve the information difference between audio and visual modalities.
They also proposed a text-guided clue mining unit
(TGCM)that uses text semantics as a guide to model text-audio-visual interaction. Finally, the researchers designed a course learning-based optimization strategy to further eliminate audio noise, evaluate sample difficulty as a measure of noise intensity in a self-aware manner, and adaptively adjust the optimization process.
They first conducted an in-depth study of the characteristics of audio in ATSG to better improve the performance improvement effect of audio modalities.
In the future, they hope to build a more appropriate evaluation benchmark for ATSG to encourage more in-depth research in this area.
Paper link: https://dl.acm.org/doi/pdf/10.1145/3581783.3612504Warehouse link: https://github.com/hlchen23 /ADPN-MM
The above is the detailed content of Tsinghua University's new method successfully locates precise video clips! SOTA has been surpassed and open sourced. For more information, please follow other related articles on the PHP Chinese website!
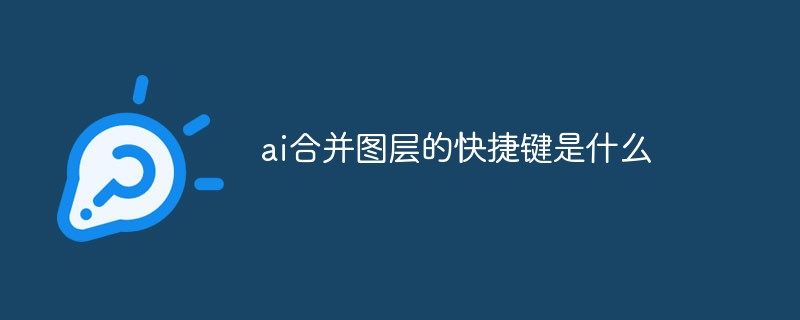
ai合并图层的快捷键是“Ctrl+Shift+E”,它的作用是把目前所有处在显示状态的图层合并,在隐藏状态的图层则不作变动。也可以选中要合并的图层,在菜单栏中依次点击“窗口”-“路径查找器”,点击“合并”按钮。
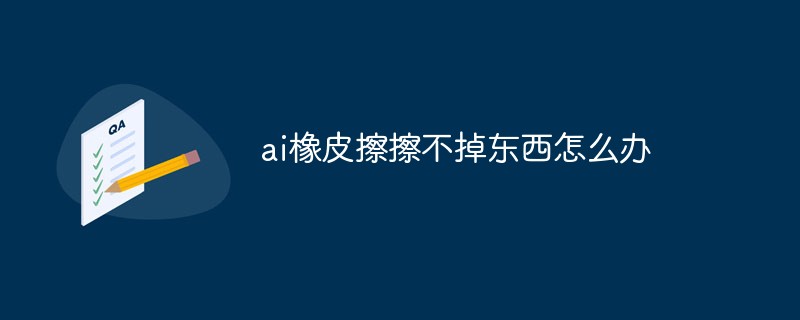
ai橡皮擦擦不掉东西是因为AI是矢量图软件,用橡皮擦不能擦位图的,其解决办法就是用蒙板工具以及钢笔勾好路径再建立蒙板即可实现擦掉东西。
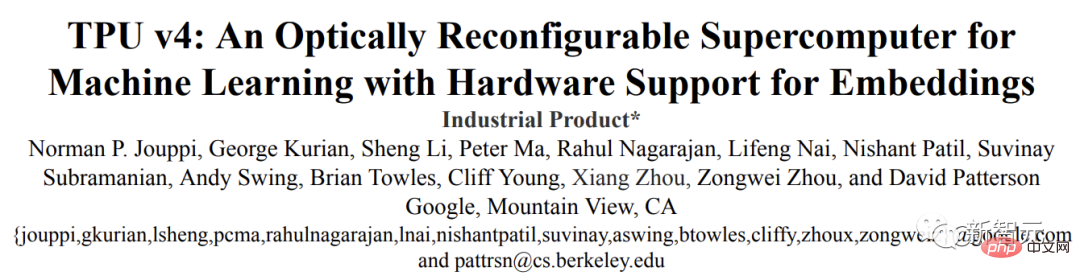
虽然谷歌早在2020年,就在自家的数据中心上部署了当时最强的AI芯片——TPU v4。但直到今年的4月4日,谷歌才首次公布了这台AI超算的技术细节。论文地址:https://arxiv.org/abs/2304.01433相比于TPU v3,TPU v4的性能要高出2.1倍,而在整合4096个芯片之后,超算的性能更是提升了10倍。另外,谷歌还声称,自家芯片要比英伟达A100更快、更节能。与A100对打,速度快1.7倍论文中,谷歌表示,对于规模相当的系统,TPU v4可以提供比英伟达A100强1.
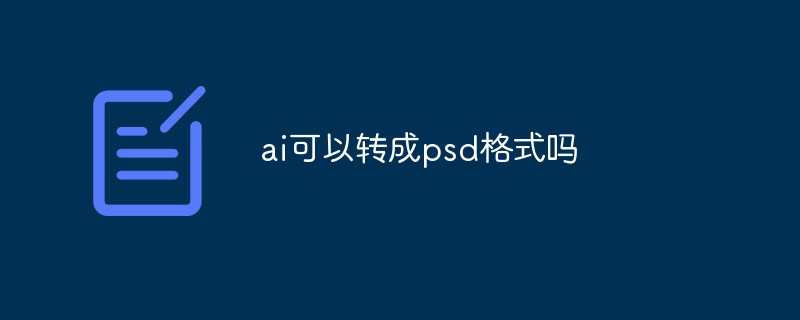
ai可以转成psd格式。转换方法:1、打开Adobe Illustrator软件,依次点击顶部菜单栏的“文件”-“打开”,选择所需的ai文件;2、点击右侧功能面板中的“图层”,点击三杠图标,在弹出的选项中选择“释放到图层(顺序)”;3、依次点击顶部菜单栏的“文件”-“导出”-“导出为”;4、在弹出的“导出”对话框中,将“保存类型”设置为“PSD格式”,点击“导出”即可;
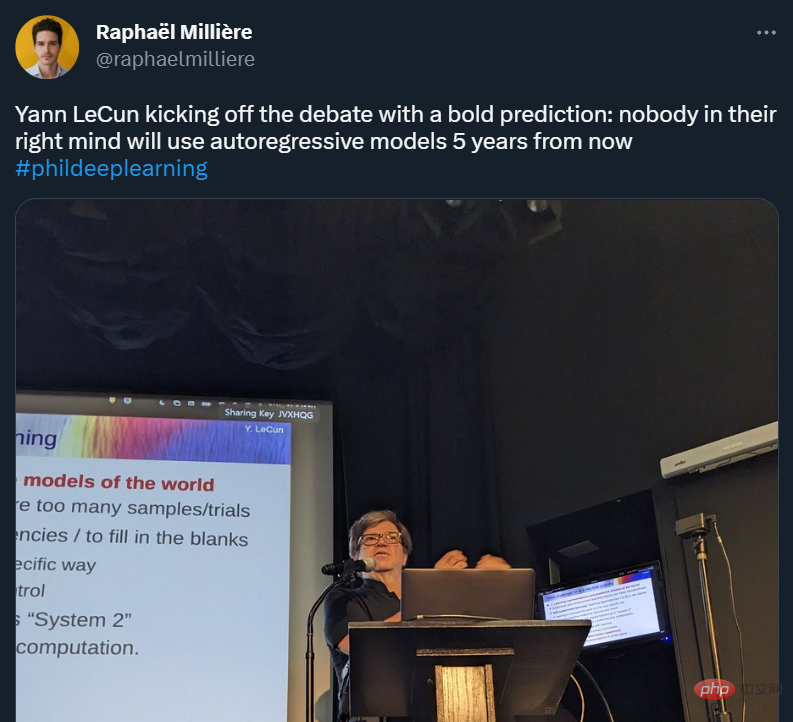
Yann LeCun 这个观点的确有些大胆。 「从现在起 5 年内,没有哪个头脑正常的人会使用自回归模型。」最近,图灵奖得主 Yann LeCun 给一场辩论做了个特别的开场。而他口中的自回归,正是当前爆红的 GPT 家族模型所依赖的学习范式。当然,被 Yann LeCun 指出问题的不只是自回归模型。在他看来,当前整个的机器学习领域都面临巨大挑战。这场辩论的主题为「Do large language models need sensory grounding for meaning and u
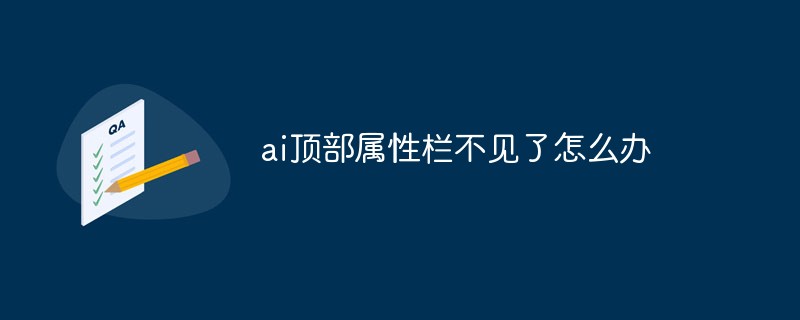
ai顶部属性栏不见了的解决办法:1、开启Ai新建画布,进入绘图页面;2、在Ai顶部菜单栏中点击“窗口”;3、在系统弹出的窗口菜单页面中点击“控制”,然后开启“控制”窗口即可显示出属性栏。
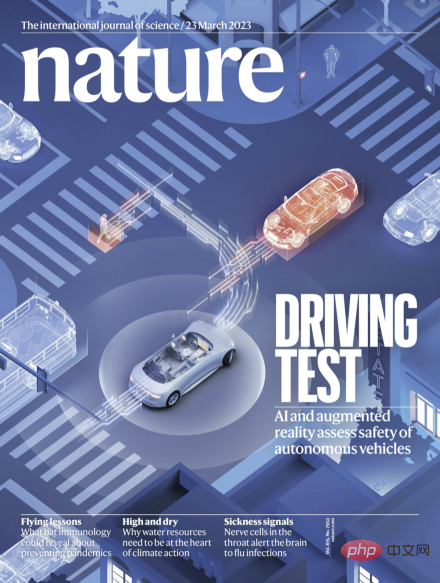
引入密集强化学习,用 AI 验证 AI。 自动驾驶汽车 (AV) 技术的快速发展,使得我们正处于交通革命的风口浪尖,其规模是自一个世纪前汽车问世以来从未见过的。自动驾驶技术具有显着提高交通安全性、机动性和可持续性的潜力,因此引起了工业界、政府机构、专业组织和学术机构的共同关注。过去 20 年里,自动驾驶汽车的发展取得了长足的进步,尤其是随着深度学习的出现更是如此。到 2015 年,开始有公司宣布他们将在 2020 之前量产 AV。不过到目前为止,并且没有 level 4 级别的 AV 可以在市场
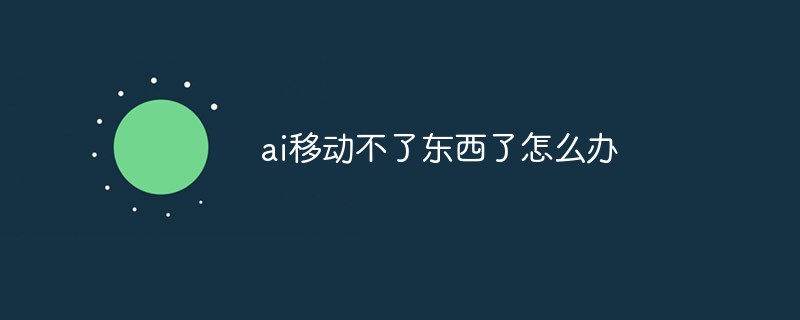
ai移动不了东西的解决办法:1、打开ai软件,打开空白文档;2、选择矩形工具,在文档中绘制矩形;3、点击选择工具,移动文档中的矩形;4、点击图层按钮,弹出图层面板对话框,解锁图层;5、点击选择工具,移动矩形即可。


Hot AI Tools

Undresser.AI Undress
AI-powered app for creating realistic nude photos

AI Clothes Remover
Online AI tool for removing clothes from photos.

Undress AI Tool
Undress images for free

Clothoff.io
AI clothes remover

AI Hentai Generator
Generate AI Hentai for free.

Hot Article

Hot Tools

PhpStorm Mac version
The latest (2018.2.1) professional PHP integrated development tool
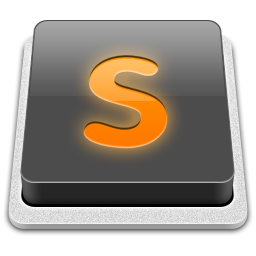
SublimeText3 Mac version
God-level code editing software (SublimeText3)

mPDF
mPDF is a PHP library that can generate PDF files from UTF-8 encoded HTML. The original author, Ian Back, wrote mPDF to output PDF files "on the fly" from his website and handle different languages. It is slower than original scripts like HTML2FPDF and produces larger files when using Unicode fonts, but supports CSS styles etc. and has a lot of enhancements. Supports almost all languages, including RTL (Arabic and Hebrew) and CJK (Chinese, Japanese and Korean). Supports nested block-level elements (such as P, DIV),

Notepad++7.3.1
Easy-to-use and free code editor

Safe Exam Browser
Safe Exam Browser is a secure browser environment for taking online exams securely. This software turns any computer into a secure workstation. It controls access to any utility and prevents students from using unauthorized resources.
